Abstract
Free full text

Predicting continental-scale patterns of bird species richness with spatially explicit models
Associated Data
Abstract
The causes of global variation in species richness have been debated for nearly two centuries with no clear resolution in sight. Competing hypotheses have typically been evaluated with correlative models that do not explicitly incorporate the mechanisms responsible for biotic diversity gradients. Here, we employ a fundamentally different approach that uses spatially explicit Monte Carlo models of the placement of cohesive geographical ranges in an environmentally heterogeneous landscape. These models predict species richness of endemic South American birds (2248 species) measured at a continental scale. We demonstrate that the principal single-factor and composite (species-energy, water-energy and temperature-kinetics) models proposed thus far fail to predict (r20.05) the richness of species with small to moderately large geographical ranges (first three range-size quartiles). These species constitute the bulk of the avifauna and are primary targets for conservation. Climate-driven models performed reasonably well only for species with the largest geographical ranges (fourth quartile) when range cohesion was enforced. Our analyses suggest that present models inadequately explain the extraordinary diversity of avian species in the montane tropics, the most species-rich region on Earth. Our findings imply that correlative climatic models substantially underestimate the importance of historical factors and small-scale niche-driven assembly processes in shaping contemporary species-richness patterns.
1. Introduction
More than 100 hypotheses have been proposed to explain large-scale spatial variation of species richness (Palmer 1994), but no consensus has yet been reached on the underlying mechanisms (Willig et al. 2003; Colwell et al. 2004; Currie et al. 2004; Pimm & Brown 2004). Studies of continental floras and faunas have repeatedly demonstrated strong relationships between total species richness and measures of temperature, precipitation and net primary productivity (Currie 1991; Rahbek & Graves 2001; Hawkins et al. 2003; Currie et al. 2004). This strong statistical signal has led to the widespread conviction that some aspect of contemporary climate ultimately controls continental species diversity (Hawkins et al. 2003). Nevertheless, despite extensive effort, no mechanistic model has thus far succeeded in explaining the observed correlation between contemporary climate and species richness (Currie et al. 2004). Alternative hypotheses have highlighted the importance of habitat heterogeneity (Guegan et al. 1998), surface area (Rosenzweig 1995), regional and evolutionary history (Ricklefs 2004), and a synergism between climate and evolutionary history (Rahbek & Graves 2001). To date, climate models have been evaluated with curve-fitting procedures, and causal relationships have been suggested for several variables that generate strong statistical signals. However, these interpretations do not provide explicit tests of alternative hypotheses, and curve-fitting procedures are not always a reliable method for discriminating among models (Burnham & Anderson 2002). Traditional correlative studies treat species richness as a ‘black box’ in that they describe the pattern of total species richness, but they do not explicitly model the underlying placement of species' geographical ranges, which actually determines measured richness (Currie 1991; Rahbek & Graves 2001; Hawkins et al. 2003; Currie et al. 2004). Consequently, the repeated demonstration of environmental correlates of species richness has not brought us any closer to resolving the mechanisms that are involved (Currie et al. 2004).
Recent null models for species richness gradients have modelled species ranges more explicitly by randomizing their placement within a spatially bounded one- or two-dimensional geographical domain (Jetz & Rahbek 2001; Colwell et al. 2004). This approach has been controversial because, in its simplest form, it assumes that species' ranges are geographically cohesive (no holes or gaps in the geographical range) and predicts that non-uniform richness patterns would be expected even if geographical ranges were placed randomly with respect to climatic variables. In contrast, climatic hypotheses implicitly assume that there are no such constraints on the placement or on the cohesion of species' geographical ranges within the domain and that species occurrences are limited primarily by climatic factors. To advance beyond this controversy, we developed spatially explicit Monte Carlo models that integrate the influence of climatic variables on the position of ranges in a heterogeneous landscape. We developed models with and without the assumption of range cohesion (hereafter called the range cohesion and range scatter models, respectively). These new ‘hybrid’ models synthesize older environmental explanations for species richness with more recent null models of species range placement (Jetz & Rahbek 2001; Rangel & Diniz-Filho 2005a).
In this paper, we apply this new modelling approach to a high-quality dataset of all South American land birds that is already well established in the literature (Rahbek & Graves 2000, 2001; Graves & Rahbek 2005; Rahbek 2005), by analysing species richness patterns for the endemic avifauna of South America (2248 species), mapped at a spatial scale of 1° latitude–longitude cells. Using these empirical data as a standard, we tested the predictions of 10 models derived from geographical and climatic variables (figure 1), all of which have previously been implicated in influencing species-richness patterns (Currie 1991; Rahbek & Graves 2001; Jetz & Rahbek 2002; Hawkins et al. 2003; Willig et al. 2003; Brown et al. 2004, Jetz et al. 2004; Ruggiero & Kitzberger 2004; Tognelli & Kelt 2004; Allen et al. 2006; Kreft et al. 2006): six single-factor models (mean annual temperature, mean annual precipitation, net primary productivity (NPP), topographic relief, ecosystem diversity and surface area); three composite models (species-energy, water-energy and temperature-kinetics); and one classic null model (geometric constraints).
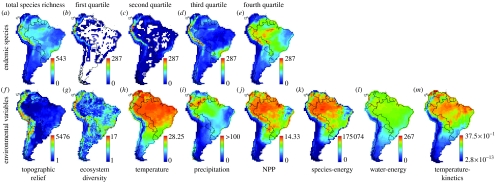
Species richness and environmental variables. (a–e) Species richness of endemic birds of South America (n=2248 species) partitioned into geographical range-size quartiles (first, smallest; fourth, largest ranges), at a scale of 1°×1° (latitude–longitude). (f–m) Environmental maps used to guide species occurrence probabilities in stochastic models. (f–j) Simple variables analogous to traditional single-factor regression analyses. (k–m) Composite variables based on published formal models of species richness: species-energy model (Currie et al. 2004); water-energy model (Hawkins et al. 2003; Currie et al. 2004); and temperature-kinetics model (Brown et al. 2004; see electronic supplementary material for details).
2. Material and methods
(a) Map construction and data template
All data and models were referenced to a gridded map of continental South America, which included 1676 cells (1°×1° latitude–longitude) containing land. Land-bridge islands on the continental shelf were excluded. This is the same template used in the previous analysis of species richness patterns of South American land and fresh-water birds (Rahbek & Graves 2001).
(b) Geographical ranges and species richness
The pattern of regional variation in avian species richness in South America is relatively well known, and the taxonomic inventory is more complete than for any other species-rich group of organisms on the continent. We used an updated version (5 September 2003) of the comprehensive geographical-range database for land and fresh-water birds that are known to have breeding populations in South America (2891 species; 531533 cell records), outlined by Rahbek & Graves (2000, 2001; see also ‘Sources of Museum Specimens’ in the electronic supplementary material for a list of museums from which primary distribution data were derived). Final maps for each species represent a conservative ‘extent of occurrence’ extrapolation at a resolution of 1°×1° cells (latitude–longitude), based on museum specimens, published sight records and spatial distribution of preferred habitat.
We used the Worldmap application (Williams 1996) to overlay the distributional data. Breeding species can be categorized as ‘endemic’ or ‘non-endemic’ depending on whether or not their global breeding ranges occur entirely within South America (and its land-bridge islands). Our analyses focused primarily on the subset of endemic species (n=2248 species; 284517 cell records), which better satisfied the assumptions of the range cohesion model (Jetz & Rahbek 2002; Colwell et al. 2004). However, results were similar when all South American species were analysed (tables 3 and 4 in the electronic supplementary material).
By species richness we mean the number of species occurring in a cell of the 1°×1° map, which approximates species density (Gotelli & Colwell 2001) at the one-degree scale.
(c) Single-factor environmental maps
(i) Climatic variables
For each of the 1676 (1°×1° latitude–longitude) land cells, we estimated the mean annual temperature and mean annual precipitation from the mean monthly climatic database published by New et al. (1999), which was compiled at a 0.5°×0.5° latitude–longitude resolution for the period 1961–1990. This source represents the most accurate published database on contemporary climate of South America available at this time. Temperature and precipitation were calculated for each 1° cell as the mean of the 0.5°×0.5° values within each cell. Data for NPP were obtained from the DOLY global model compiled at a 0.25°×0.25° latitude–longitude resolution (Woodward et al. 1995). NPP was calculated for each 1° cell as the mean of the 0.25°×0.25° values within each cell. Environmental maps for temperature, precipitation and NPP appear in figure 1 (parts h, i and j, respectively).
(ii) Ecosystem diversity
We obtained simple estimates of habitat diversity (figure 1) by counting the number of distinct ecosystems in each cell from a recently published map of global ecosystems (http://edcdaac.usgs.gov/glcc/sadoc1_2.html). This source recognized 94 ecosystem classes derived from 1km Advanced Very High-Resolution Radiometer (AVHRR) data spanning a 12-month period (April 1992 to March 1993).
(iii) Topography
We used topographic relief (maximum minus minimum elevation) in each cell as a surrogate for topographic heterogeneity (figure 1). Elevational data were derived from the Global Land One-kilometre Base Elevation (GLOBE) Digital Elevation Model (http://www.ngdc.noaa.gov/mgg/topo/globe.html). Maximum elevation was truncated at the observed snowline (1200–5700m), which varies as a complex function of environmental variables, including latitude and precipitation. No non-marine bird species recorded in South America is known to breed on glaciers, ice or in snowfields.
(iv) Map cell surface area
Area was calculated as the land surface area within each 1°×1° latitudinal–longitudinal map cell. We retained coastal cells in the analyses because they include a significant fraction of the topographic relief in South America (Rahbek & Graves 2000). The planimetric area of oceans and marine estuaries was subtracted from the area of coastal cells. Area is typically calculated as the planimetric area of map cells rather than as their surface area. Planimetric area calculation ignores the topographic texture within each cell, which can vary significantly for a given domain, often in a spatially ordered fashion (e.g. in South America, the error introduced using planimetric area decreases longitudinally at the Equator, from the topographically rugged cells encompassing the Andean mountains eastward through the relatively planar Amazonian lowlands). Using the GLOBE DEM dataset (http://www.ngdc.noaa.gov/mgg/topo/globe.html), which provides a digital elevational model with a resolution of 30 arc-seconds (1/120°), we calculated the three-dimensional surface area for each of the 1676 1°×1° cells constituting the terrestrial domain of South America, following the protocol of Jennes (2002), adapted to our data and purpose.
On a 30 arc-second scale, each 1°×1° cell encompasses 14400 elevational values, one for each 30 arc-second sub-cell. Surface area was calculated for each 30 arc-second sub-cell within each 1°×1° cell, based on triangular areas derived from eight triangles. Each triangle connected the centre point of the focal cell (pixel) with the centre points of two adjacent cells among the eight cells surrounding the focal cell. These triangles were located in a three-dimensional space, so that the area of each triangle represented the true surface area (given the resolution of our digital elevational model) of the space bounded by its vertices. The total area of eight triangles built around the focal cell area was adjusted, so that it represented only that proportion of the triangles coincident with the focal 1°×1° cell (or, for coastal cells, the proportion overlying land). Finally, the areas of all the 30 arc-second sub-cells that overlapped land or fresh water within a given 1°×1° cell were summed to produce the topographic surface area used in our models.
(d) Environmental maps for composite models
(i) Species-energy model
The species-energy model assumes that the density of individuals depends on both productivity and area and that the number of individuals and species richness are positively correlated. Using the equations and model framework of Currie et al. (2004), we created an environmental map (figure 1) for the following model:
where area is the map cell surface area (see above).
(ii) Water-energy model
An extensive literature has developed implicating the role of precipitation and energy in controlling species richness (Hawkins et al. 2003). However, there is no agreement on the specific form of the relationship between species richness and these variables (Currie et al. 2004). In the absence of a formal theoretical framework, we conducted a principal components analysis (PCA) of temperature, precipitation and NPP to create a multivariate function for establishing cell probabilities. We used the loadings from the first principal component, which accounted for 84% of the variation in these variables among cells, to create an environmental map (figure 1) for the following model:
Probabilities of species occurrence are the highest in cells exhibiting relatively high temperatures, high precipitation and high NPP.
(iii) Temperature-kinetics model
Allen et al. (2002) derived, from first principles, a model of species richness as a function of temperature. Although their model was designed specifically for ectotherms, it may be appropriate for birds as well because the relationship between temperature-corrected population density and body mass is virtually identical for ectotherms and endotherms (fig. 2 of Allen et al. 2002). Brown et al. (2004) subsequently reformulated this model, and we have used this most current formulation of the temperature-kinetics model (Allen et al. 2006). Although this model uses temperature as the only predictor variable, the nonlinear functional form of the relationship is different from our simple temperature model. We created an environmental map (figure 1) based on the functional form of the temperature-kinetics model from Brown et al. (2004):
where T is absolute temperature (K); E is average activation energy of the respiration complex (ca 0.65eV; 1
eV=1.602×10−19
J); and k is the Boltzmann constant (8.62×10−5
eV
K−1; Allen et al. 2006).
(e) Map cell probabilities
For each of the nine environmental maps (cell surface area, topographic relief, NPP, temperature, precipitation, ecosystem diversity, species-energy model, water-energy model and temperature-kinetics model), we prepared a corresponding probability map. Each of the 1676 terrestrial cells for South America (including inland lakes and rivers), arranged in their correct geographical relationship to one another, was assigned a non-zero probability of occurrence, as specified below.
To create the probability map for a simple environmental variable (e.g. temperature) or a derived variable (e.g. temperature-kinetics model value) x, we began with a raw value xij for a cell in row i and column j of the matrix (terrestrial cells only). Maps of these raw values for most of the environmental drivers are illustrated in figure 1. (Surface area is not illustrated.) The modelled probability of cell selection Pij for the cell was then defined as
For the 10th model (geometric constraints), Pij is constant for all map cells at a value of 1/1676.
(f) Stochastic models of species richness
Probability maps based on each of the six single-factor models and three composite models outlined above were used to guide two stochastic models of range location and structure: the range cohesion model and the range scatter model (see the electronic supplementary material for a detailed description of the models).
In both models, the geographical range of each species in the empirical avifauna was stochastically reconstructed in South America using the same number of cells as in its observed range. Each range was reconstructed using the same rules, regardless of the actual identity of the species it represented. Once all ranges had been simulated, predicted species richness (the number of species that occurred in each map cell in the model) was compared statistically with observed species richness. Range simulation for each species began the same way in both models. An initial map cell was chosen stochastically based on the probability map for a particular environmental model (as defined in §2e): the higher the value for a map cell, the more likely was the cell to be chosen. The difference between the two models lies in the placement of cell occurrences for the remaining cells (if any) of the modelled range. In the range scatter model, range cohesion was not enforced. The placement of each range was completed by choosing the second and the subsequent cells from among all cells not already occupied by that species, anywhere in the map, guided only by the pertinent probability map. The range scatter model assumes that the probability that a species occupies a particular grid cell depends only on the environment, not on the proximity of other cells occupied by that species. Thus, although the observed number of occupied cells for each species was preserved in the modelled distributions, the cohesion of each geographical range was unconstrained. Biologically, such a distribution implies a complete absence of intrinsic limits and extrinsic barriers to dispersal (Rangel & Diniz-Filho 2005b).
In contrast, in the range cohesion model, each species' range was completed by choosing the second and the subsequent cells from among cells neighbouring those already occupied by that species, based on the relative values of all adjacent unoccupied cells in the pertinent probability map (see the electronic supplementary material for details). In this way, the cohesion of each species' geographical range is preserved, although the precise placement and shape of the range is guided stochastically by the environmentally determined map cell probabilities. The range cohesion model is appropriate for taxa mapped at a scale coarse enough to yield continuous ranges for most species, based on presence–absence data (Gaston 2003).
The stochastic placement of species occurrences in the two models is a Monte Carlo method for estimating the statistical expectation of species richness (range overlap) in each map cell, with and without range cohesion. In the special case of a uniform environment (all cells are equiprobable), the range cohesion model simplifies to the ‘geometric constraints’ (spreading dye) model of Jetz & Rahbek (2001) and the range scatter model becomes equivalent to their ‘area model’ and to the ‘random placement model’ of Ney-Nifle & Mangel (1999).
(g) Statistical analysis
For each of the 10 environmental maps, we ran a range scatter model and a range cohesion model 300 times each and then regressed cell values for observed species richness on cell values for mean species richness predicted by each model. In addition to simple ordinary least squares (OLS) regressions, we computed generalized least squares (GLS) regressions and (where necessary) simultaneous autoregressive (SAR) models to estimate regression coefficients and intercepts while accounting for spatial autocorrelation. For comparison, we also computed simple (OLS) regressions of observed richness on the raw environmental factors. Model selection was based on the spatially corrected slope and intercept values (a slope of unity with an intercept at zero indicates a perfect fit) and the corrected p-value for the statistical significance of r2 (based on Dutilleul's method). We ran these tests for bird species endemic to South America (284517 cell records for 2248 species). To assess the robustness of the results, we carried out regressions for the six single-factor environmental maps, using range data for all birds breeding in South America (531
533 cell records for 2891 species). Finally, we partitioned the species pool into subsets of species based on their range size (first through fourth quartiles of ranked ranges) and repeated all analyses for each quartile. See electronic supplementary material for a detailed description of statistical analysis.
3. Results
When all species are considered together, the regressions attribute substantial explanatory power to all six climatic models (precipitation, temperature, NPP, species-energy, water-energy and temperature-kinetics; 0.24r2
0.46, table 1, all quartiles column). Similar correlations between climatic factors and species richness are typical of continental studies conducted at comparable spatial resolutions (1° or 2° latitude–longitude cells; Currie 1991; Rahbek & Graves 2001; Jetz & Rahbek 2002; Hawkins et al. 2003; Currie et al. 2004; Ruggiero & Kitzberger 2004; Kreft et al. 2006). At our scale of analysis, the three models related to spatial heterogeneity (surface area, ecosystem diversity and topographic relief) and the pure geometric constraints model were less successful than climate-based models in explaining aggregate species richness (0.00
r2
0.27, table 1, all quartiles column).
Table 1
Explanatory models for species richness of endemic birds of South America (n=2248). Rows represent environmental models and columns represent species range quartiles and stochastic range placement procedures (RS, range scatter model; RC, range cohesion model). In each table cell, the first entry is the simple r2 value for the correlation between observed and predicted species richness. The second entry is the slope of the relationship, based on an appropriately fitted spatial autocorrelation model (see Rangel et al. (2006) and supplementary table 1 in the electronic supplementary material for full model details). A successful model should explain a significant proportion of the variation in species richness and have a slope that is close to 1.0. Unshaded cells indicate non-explanatory models, for which the r2-value does not differ significantly from 0, based on the effective number of degrees of freedom using Dutilleul's method to adjust for spatial autocorrelation (Dutilleul 1993). Light grey cells indicate models for which the r2-value was significantly different from 0, but for which the 95% confidence interval of the slope for the best-fitting spatial model did not bracket 1.0. (Note that some models in this category have negative slopes.) Dark grey cells (which have italic type) indicate models for which both the r2 and the slope criterion were satisfied. Within each quartile, the model for which the slope is closest to 1.0 is boldfaced, indicating the best-fitting model for that quartile. For the fourth quartile species, the slope values for the water-energy, temperature-kinetics and temperature models were virtually equidistant from the expected slope of 1.0, but the water-energy model was selected as best fitting because it had a slightly higher r2-value and a better fitting intercept (see table 1 in the electronic supplementary material). Comparable results based on all birds of South America (n=2891) are shown in table 3 in the electronic supplementary material. Results of simple (OLS) regressions of observed species richness on raw environmental variables appear in table 2 in the electronic supplementary material for endemic species and in table 4 in the electronic supplementary material for all species. Note that, for species with the largest ranges (fourth quartile), incorporating range cohesion substantially improved the fit of all environmental models to observed richness (RS versus RC for fourth quartile). When species of all range size quartiles were considered together (all quartiles column), the effect of range cohesion on model fit was quantitatively weaker, but remained consistent (only the species-energy model had a slightly lower r2 for RC than for RS).
quartile | first quartile | second quartile | third quartile | fourth quartile | all quartiles | |||||
---|---|---|---|---|---|---|---|---|---|---|
factor | RS | RC | RS | RC | RS | RC | RS | RC | RS | RC |
geometric constraints | n/a | 0.01 | n/a | 0.00 | n/a | 0.01 | n/a | 0.37 | n/a | 0.16 |
0.00 | 3.77 | 0.00 | 0.99 | 0.00 | 1.18 | 0.00 | 0.74 | 0.00 | 1.19 | |
species-energy | 0.01 | 0.01 | 0.00 | 0.02 | 0.00 | 0.00 | 0.66 | 0.72 | 0.46 | 0.43 |
−0.72 | −1.15 | −0.39 | −1.01 | 0.21 | −0.18 | 0.42 | 0.38 | 0.24 | 0.49 | |
water-energy | 0.00 | 0.00 | 0.01 | 0.02 | 0.00 | 0.01 | 0.55 | 0.71 | 0.33 | 0.39 |
−5.15 | −3.40 | −3.46 | −2.14 | −1.27 | −0.61 | 0.57 | 1.03 | −0.07 | 0.61 | |
temperature-kinetics | 0.01 | 0.02 | 0.03 | 0.05 | 0.04 | 0.05 | 0.46 | 0.66 | 0.21 | 0.30 |
−5.33 | −4.16 | −3.90 | −2.57 | −1.60 | −0.98 | 0.25 | 0.99 | −0.51 | −0.14 | |
precipitation (mm![]() | 0.00 | 0.00 | 0.00 | 0.00 | 0.02 | 0.01 | 0.49 | 0.65 | 0.39 | 0.42 |
−0.89 | −0.83 | −0.30 | −0.49 | 0.11 | 0.01 | 0.36 | 0.88 | 0.04 | 0.53 | |
temperature (mean annual, °C) | 0.01 | 0.01 | 0.02 | 0.03 | 0.02 | 0.02 | 0.47 | 0.68 | 0.25 | 0.34 |
−4.91 | −3.09 | −3.77 | −2.07 | −1.52 | −0.61 | 0.41 | 0.98 | −0.17 | 0.51 | |
net primary productivity | 0.00 | 0.01 | 0.00 | 0.02 | 0.01 | 0.00 | 0.63 | 0.74 | 0.44 | 0.44 |
(tons carbon per hectare per year) | −1.51 | −1.58 | −0.80 | −1.17 | −0.12 | −0.41 | 0.58 | 0.89 | 0.27 | 0.63 |
topographic surface area (km2) | 0.01 | 0.01 | 0.00 | 0.00 | 0.01 | 0.00 | 0.22 | 0.48 | 0.20 | 0.27 |
1.92 | 1.39 | 1.17 | 0.55 | 0.79 | 0.90 | 0.05 | 0.12 | 0.21 | 0.34 | |
ecosystem diversity | 0.21 | 0.23 | 0.22 | 0.19 | 0.19 | 0.11 | 0.00 | 0.11 | 0.06 | 0.12 |
(number of ecosystems in cell) | 3.01 | 2.93 | 1.88 | 1.80 | 0.68 | 0.75 | 0.06 | 0.23 | 0.29 | 0.51 |
topographic relief | 0.34 | 0.33 | 0.42 | 0.38 | 0.22 | 0.17 | 0.22 | 0.24 | 0.00 | 0.02 |
(elevational range, m.a.s.l.) | 1.53 | 1.40 | 1.10 | 0.99 | 0.47 | 0.53 | 0.04 | 0.07 | 0.21 | 0.29 |
The predictive power of our climate-based models was not sustained, however, when the species pool was partitioned into quartiles of species' geographical range sizes (first quartile, smallest ranges; fourth quartile, largest ranges). For the first three range-size quartiles (all but the largest ranges), all models based on climate variables (precipitation, temperature, NPP, species-energy, water-energy and temperature-kinetics), as well as those based on geometric constraints and surface area, failed completely to predict endemic species richness (0.00r2
0.05, table 1; figure 2). Qualitatively, the same result is obtained for simple regressions of observed species richness on raw environmental variables (table 2 in the electronic supplementary material), and for corresponding analyses of the entire avifauna (n=2891 species), which includes an additional 643 non-endemic species whose ranges extend beyond the boundaries of South America (tables 3 and 4 in the electronic supplementary material). In contrast, fourth quartile species (those with the largest ranges) yielded strong correlations with the predictions of the climate models (0.46
r2
0.74, table 1; figure 2) and with the untransformed environmental variables (table 2 in the electronic supplementary material), although the latter yielded substantially poorer fits for fourth quartile species than the corresponding range cohesion models.
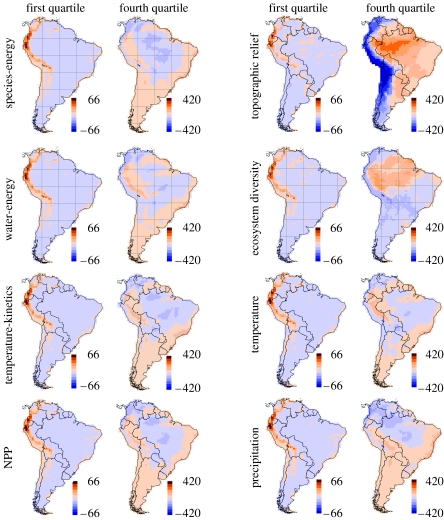
Spatial distribution of residuals (observed minus expected bird species richness) from range cohesion models. Results are shown for subsets of endemic species partitioned into geographical range-size quartiles for the first (smallest ranges) and fourth (largest ranges) quartiles for eight environmentally driven models. Note that the colour scale differs for the quartiles.
For first quartile species, the accumulation of species richness with topographic relief (as measured by elevational range) was significantly steeper than our models predicted (slope>1.0; table 1). For second quartile species, the model based on topographic relief accurately predicted species richness, whereas, for third and fourth quartiles, the models based on topographic relief and ecosystem diversity overestimated species richness.
A previous correlative analysis of African birds also found that the effects of productivity decreased and topographic heterogeneity increased at small range sizes (Jetz & Rahbek 2002). However, our study is the first to document a complete lack of correlation between species richness and the predictions of climate-based models for all but the largest-ranged species.
4. Discussion
Our results and those of some previous analyses (Jetz & Rahbek 2001, 2002; Lennon et al. 2004; Ruggiero & Kitzberger 2004; Kreft et al. 2006) suggest that statistical associations between total species richness and environmental predictor variables may be misleading owing to the dominating influence of widespread species. The geographical distribution of South American bird species with the largest geographical ranges (fourth quartile) was successfully explained only by the version of the water-energy model that incorporates geographical range cohesion as well as precipitation, temperature and NPP (table 1). In contrast, all models based on contemporary climate variables were unsuccessful in predicting species richness of taxa with smaller ranges (first to third quartiles). These results are not artefacts of sample size dilution caused by range size partitioning (tables 2 and 4 in the electronic supplementary material). Species with relatively small geographical ranges constitute the bulk of the South American avifauna, and they contribute heavily to the peaks of species richness observed in the Andes and other montane regions of South America (Graves & Rahbek 2005; figures 1 and and22).
Without exception, the inclusion of the range cohesion assumption improved the fit of the data to the model predictions for the widest ranging species (fourth quartile). Moreover, the hybrid models (range cohesion plus climate factors) always did a better job of predicting species richness of wide-ranging species than simple climate models that ignore range cohesion or classic null models that enforce range cohesion but assume a homogeneous spatial environment. As an example, figure 3 illustrates the effects of incorporating or omitting the range cohesion assumption for the NPP model.
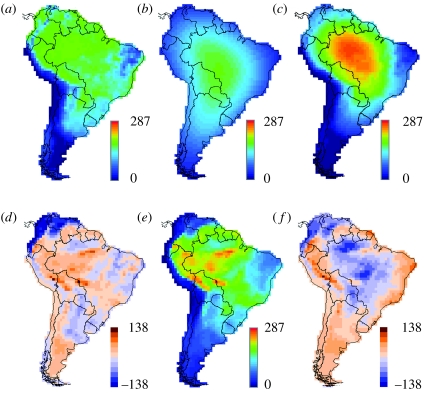
Effect of range cohesion on predicted bird species richness for fourth quartile (largest) ranges. Expected richness driven by: (a) NPP in the range scatter model (geographical range cohesion not enforced; r2=0.63); (b) simple geometric constraints (geographical range cohesion enforced, but environment uniform, producing the mid-domain peak expected for such models; r2=0.37); (c) NPP in the range cohesion model (necessarily influenced by geometric constraints; r2=0.74). (d) Spatial distribution of residuals (observed minus expected species richness) for the range scatter NPP model, (a). (e) Observed richness. (f) Residuals for the range cohesion NPP model (c).
In contrast to single-factor and composite climate-driven models, the two models that directly incorporate habitat heterogeneity (topographic relief and ecosystem diversity) were stronger predictors of species richness for taxa with small to moderately large ranges (first to third quartiles: 0.11<r2<0.42, p<0.05; table 1) than for species with the largest ranges (fourth quartile: 0.00<r2<0.24, p>0.05; table 1). However, even these models could not account entirely for the species richness peaks in humid montane regions, especially at equatorial latitudes (figure 2).
The extraordinary species richness of the Andean region is thought to be caused by elevated rates of speciation, which are promoted by highly dissected topography, narrow homothermous elevational zones, linear geographical ranges and disjunct habitat distributions (Vuilleumier & Simberloff 1980; Graves 1985, 1988; Fjeldså 1995; Rahbek & Graves 2001). At the continental scale, the large residual variance (more than 97% for the first three range-size quartiles) generated by our contemporary climate models (table 1) may reflect historical events associated with the Pleistocene–Holocene distribution and diversity of habitats (Haffer 1969) as well as species-specific habitat preferences (Graves & Rahbek 2005) and niche conservatism (Peterson et al. 1999; Wiens & Donoghue 2004). Although some of these mechanisms undoubtedly interact with climatic factors, they are largely uncoupled from measures of contemporary climate at large spatial scales, and the modern distribution of avian species in South America at the 1° scale is poorly predicted by measures of contemporary climate.
Three caveats apply to our models and interpretations. First, no specific functional relationships have been proposed in the literature, based on the mechanistic principles, for species richness as a function of the environmental variables that drive the single-factor models. In the absence of such functional forms and given the infinite number of more complex possibilities, the probability maps for single-factor models assume parsimoniously that a simple proportional mapping is an adequate representation of the relationship between the measured environmental variable and the probability of occurrence. Simple (1:
1) proportionality will not obviously hold over a very large range of values (e.g. temperature), but as an initial assumption for the restricted range of contemporary climate values observed in South America (and the even smaller scope of variation in these variables within the local neighbourhoods of cohesive ranges), proportionality should capture the essence of any strongly determined relationships with species occurrence. Moreover, when range cohesion is enforced, the modelled spatial pattern of range overlap (and thus of species richness) is not a simple transformation of environmental variables, but it reflects the complexities of spatial pattern in the environment as well. We did not find evidence of strong nonlinearities in avian species richness as a function of any of the single-factor environmental variables over their actual range of values, and all relationships proved to be monotonic, including richness as a function of NPP (as illustrated in supplementary figure 1 in the electronic supplementary material), which assumes a humped form at some spatial scales (Rosenzweig 1995). To date, only the temperature-kinetics and species-energy models provide a priori nonlinear functional forms for modelling the probability of species occurrence, and we modelled them accordingly. Other macroecological models are simply verbal descriptions of the effects of variables that have been measured with conventional curve-fitting techniques.
Second, the results we have presented here are likely to be scale dependent (Rahbek & Graves 2000, 2001; Willis & Whittaker 2002; Rahbek 2005). The resolution of peaks and troughs of species richness in South American birds varies with macroecological scale as do the correlations of environmental variables with spatial gradients of species richness, as demonstrated by Rahbek & Graves (2000, 2001). In those studies, the predictive power of stepwise regression models incorporating simple climatic and topographic variables exhibited a roughly monotonic increase with increasing cell size, although the ranking of variables depended on spatial scale. In particular, the variance in species richness explained by topography increased dramatically when cell size increased from 1°×1° to 10°×10°. The critical question is what statistical patterns will emerge at finer spatial scales (e.g. ca 10–1km2). Although finer scales of resolution may be impossible to achieve for the entire continent, we expect that avian species richness will show stronger associations with local climate when cell size is progressively reduced to the point where few distinctive habitats are sampled by a single map cell. In any case, the scaling effects fall outside the scope of the present study.
Finally, by using Model I spatial regressions, our statistical analysis has modelled error and spatial autocorrelation in the Y variable (observed species richness), but it has not modelled the effect of errors in underlying climatic and environmental data on predicted richness (the X variable). In the simplest case, errors in X variables bias measured slopes downward (Mesplé et al. 1996; Farrell-Gray & Gotelli 2005). Ideally, a Model II regression approach should be applied, testing the slope and intercept of the reduced major axis (Mesplé et al. 1996), but unfortunately, spatial regression methods for Model II regression are not yet available. Meanwhile, the error introduced using Model I regression (underestimation of regression slopes, compared with Model II) is likely to be substantially less serious and unpredictable than the likely consequences of ignoring spatial autocorrelation, which is known to be important in generating ecological patterns (Legendre 1993; Lichstein et al. 2002; Diniz-Filho et al. 2003).
Questions about linearity, scale dependence and sources of measurement error are not unique to our analysis. Rather, they are common to all macroecological analyses, although not always explicitly discussed.
In summary, our models and analyses suggest that history, topography and niche-driven assembly processes may be more important than large-scale contemporary climate in shaping present-day patterns of species richness in South American birds. Future modelling efforts should incorporate phylogeny (Davies et al. 2005), range-size evolution (Rangel & Diniz-Filho 2005b), dispersal dynamics (Hubbell 2001; Leibold 2004) and community assembly processes (Graves & Gotelli 1993; Graves & Rahbek 2005). Such models may provide better insights into the proximate and ultimate causes of species richness patterns for taxa with smaller geographical ranges, which are often the prime focus of conservation efforts.
Acknowledgments
We thank M. Bjerrum and F. Skov for their assistance in recompiling GIS data as necessary. P. Williams kindly provided the Worldmap software used to manage the distributional data and to generate figures 1–3. Support for travel to a joint work session in Sweden at which the first draft of this paper was written was provided by The Danish National Science Foundation (J. no. 21-03-0221). C.R. acknowledges the Danish National Science Foundation (J. no. 21-03-0221) for support of macroecological research. N.J.G. and G.L.E. acknowledge NSF grants DEB-0107403 and DEB 05-41936 for support of modelling and null model research. R.K.C. was supported by NSF grant DEB-0072702. G.R.G. was supported by the Alexander Wetmore fund of the Smithsonian Institution. T.F.L.V.B.R was supported by CNPq graduate studentship 133745/2005-8.
Supplementary Material
This file contains museum sources that were consulted for the primary data, details of the simulation algorithm, spatial regression analyses, and expanded regression results.
References
- Allen A.P, Brown J.H, Gillooly J.F. Global biodiversity, biochemical kinetics, and the energetic-equivalence rule. Science. 2002;297:1545–1548. 10.1126/science.1072380 [Abstract] [Google Scholar]
- Allen A.P, Gillooly J.F, Savage V.M, Brown J.H. Kinetic effects of temperature on rates of genetic divergence and speciation. Proc. Natl Acad. Sci. USA. 2006;103:9130–9135. 10.1073/pnas.0603587103 [Europe PMC free article] [Abstract] [Google Scholar]
- Brown J.H, Gillooly J.F, Allen A.P, Savage V.M, West G.B. Toward a metabolic theory of ecology. Ecology. 2004;85:1771–1789. [Google Scholar]
- Burnham K.P, Anderson D.R. Springer; New York, NY: 2002. Model selection and multimodel inference: a practical information-theoretical approach. [Google Scholar]
- Colwell R.K, Rahbek C, Gotelli N.J. The mid-domain effect and species richness patterns: what have we learned so far? Am. Nat. 2004;163:E1–E23. 10.1086/382056 [Abstract] [Google Scholar]
- Currie D.J. Energy and large-scale patterns of animal-species and plant-species richness. Am. Nat. 1991;137:27–49. 10.1086/285144 [Google Scholar]
- Currie D.J, et al. Predictions and tests of climate-based hypotheses of broad-scale variation in taxonomic richness. Ecol. Lett. 2004;7:1121–1134. 10.1111/j.1461-0248.2004.00671.x [Google Scholar]
- Davies T.J, Grenyer R, Gittleman J.L. Phylogeny can make the mid-domain effect an inappropriate null model. Biol. Lett. 2005;1:143–146. 10.1098/rsbl.2005.0297 [Europe PMC free article] [Abstract] [Google Scholar]
- Diniz-Filho J.A.F, Bini L.M, Hawkins B.A. Spatial autocorrelation and red herrings in geographical ecology. Global Ecol. Biogeogr. 2003;12:53–64. 10.1046/j.1466-822X.2003.00322.x [Google Scholar]
- Dutilleul P. Modifying the t-test for assessing the correlation between 2 spatial processes. Biometrics. 1993;49:305–314. 10.2307/2532625 [Google Scholar]
- Farrell-Gray C.C, Gotelli N.J. Allometric exponents support a ¾ power scaling law. Ecology. 2005;86:2083–2087. [Google Scholar]
- Fjeldså J. Geographical patterns of neoendemic and older relict species of Andean forest birds: the significance of ecologically stable areas. In: Churchill S.P, editor. Biodiversity and conservation of neotropical montane forests. New York Botanical Garden; New York, NY: 1995. [Google Scholar]
- Gotelli N.J, Colwell R.K. Quantifying biodiversity: procedures and pitfalls in the measurement and comparison of species richness. Ecol. Lett. 2001;4:379–391. 10.1046/j.1461-0248.2001.00230.x [Google Scholar]
- Gaston K.J. Blackwell Science; Oxford, UK: 2003. The structure and dynamics of geographical ranges. [Google Scholar]
- Graves G.R. Elevational correlates of speciation and intraspecific geographic-variation in plumage in Andean forest birds. Auk. 1985;102:556–579. [Google Scholar]
- Graves G.R. Linearity of geographic range and its possible effect on the population structure of Andean birds. Auk. 1988;105:47–52. [Google Scholar]
- Graves G.R, Gotelli N.J. Assembly of avian mixed-species flocks in Amazonia. Proc. Natl Acad. Sci. USA. 1993;90:1388–1391. 10.1073/pnas.90.4.1388 [Europe PMC free article] [Abstract] [Google Scholar]
- Graves G.R, Rahbek C. Source pool geometry and the assembly of continental avifaunas. Proc. Natl Acad. Sci. USA. 2005;102:7871–7876. 10.1073/pnas.0500424102 [Europe PMC free article] [Abstract] [Google Scholar]
- Guegan J.F, Lek S, Oberdorff T. Energy availability and habitat heterogeneity predict global riverine fish diversity. Nature. 1998;391:382–384. 10.1038/34899 [Google Scholar]
- Haffer J. Speciation in Amazonian forest birds. Science. 1969;165:131–137. 10.1126/science.165.3889.131 [Abstract] [Google Scholar]
- Hawkins B.A, et al. Energy, water, and broad-scale geographic patterns of species richness. Ecology. 2003;84:3105–3117. [Google Scholar]
- Hubbell S.P. Princeton University Press; Princeton, NJ: 2001. The unified neutral theory of biodiversity and biogeography. [Abstract] [Google Scholar]
- Jennes, J. 2002 Surface areas and ratios from elevation grid (surgrids.avx) extension for ArcView 3.x, v. 1.2. (Available at http://www.jennessent.com/arcview/surface_areas.htm.)
- Jetz W, Rahbek C. Geometric constraints explain much of the species richness pattern in African birds. Proc. Natl Acad. Sci. USA. 2001;98:5661–5666. 10.1073/pnas.091100998 [Europe PMC free article] [Abstract] [Google Scholar]
- Jetz W, Rahbek C. Geographic range size and determinants of avian species richness. Science. 2002;297:1548–1551. 10.1126/science.1072779 [Abstract] [Google Scholar]
- Jetz W, Rahbek C, Colwell R.K. The coincidence of rarity and richness and the potential signature of history in centres of endemism. Ecol. Lett. 2004;7:1180–1191. 10.1111/j.1461-0248.2004.00678.x [Google Scholar]
- Kreft H, Sommer J.H, Barthlott W. The significance of geographic range size for spatial diversity patterns in Neotropical palms. Ecography. 2006;29:21–30. 10.1111/j.2005.0906-7590.04203.x [Google Scholar]
- Legendre P. Spatial autocorrelation—trouble or new paradigm? Ecology. 1993;74:1659–1673. 10.2307/1939924 [Google Scholar]
- Leibold M.A, et al. The metacommunity concept: a framework for multi-scale community ecology. Ecol. Lett. 2004;7:601–613. 10.1111/j.1461-0248.2004.00608.x [Google Scholar]
- Lennon J.J, Koleff P, Greenwood J.J.D, Gaston K.J. Contribution of rarity and commonness to patterns of species richness. Ecol. Lett. 2004;7:81–87. 10.1046/j.1461-0248.2004.00548.x [Google Scholar]
- Lichstein J.W, Simons T.R, Shriner S.A, Franzreb K.E. Spatial autocorrelation and autoregressive models in ecology. Ecol. Monogr. 2002;72:445–463. [Google Scholar]
- Mesplé F, Troussellier M, Casellas C, Legendre P. Evaluation of simple statistical criteria to qualify a simulation. Ecol. Model. 1996;88:9–18. 10.1016/0304-3800(95)00033-X [Google Scholar]
- New M, Hulme M, Jones P. Representing twentieth-century space—time climate variability. Part I: Development of a 1961–90 mean monthly terrestrial climatology. J. Climate. 1999;12:829–856. 10.1175/1520-0442(2000)013<2217:RTCSTC>2.0.CO;2 [Google Scholar]
- Ney-Nifle M, Mangel M. Species-area curves based on geographic range and occupancy. J. Theor. Biol. 1999;196:327–342. 10.1006/jtbi.1998.0844 [Google Scholar]
- Palmer M.W. Variation in species richness—towards a unification of hypotheses. Folia Geobot. Phytotaxon. 1994;29:511–530. [Google Scholar]
- Peterson A.T, Soberon J, Sanchez-Cordero V. Conservatism of ecological niches in evolutionary time. Science. 1999;285:1265–1267. 10.1126/science.285.5431.1265 [Abstract] [Google Scholar]
- Pimm S.L, Brown J.H. Domains of diversity. Science. 2004;304:831. 10.1126/science.1095332 [Abstract] [Google Scholar]
- Rahbek C. The role of spatial scale and the perception of large-scale species richness patterns. Ecol. Lett. 2005;8:224–239. 10.1111/j.1461-0248.2004.00701.x [Google Scholar]
- Rahbek C, Graves G.R. Detection of macro-ecological patterns in South American hummingbirds is affected by spatial scale. Proc. R. Soc. B. 2000;267:2259–2265. 10.1098/rspb.2000.1277 [Europe PMC free article] [Abstract] [Google Scholar]
- Rahbek C, Graves G.R. Multiscale assessment of patterns of avian species richness. Proc. Natl Acad. Sci. USA. 2001;98:4534–4539. 10.1073/pnas.071034898 [Europe PMC free article] [Abstract] [Google Scholar]
- Rangel T.F.L.V.B, Diniz-Filho J.A.F. Neutral community dynamics, the mid-domain effect and spatial patterns in species richness. Ecol. Lett. 2005;8:783–790. 10.1111/j.1461-0248.2005.00786.x [Google Scholar]
- Rangel T.F.L.V.B, Diniz-Filho J.A.F. An evolutionary tolerance model explaining spatial patterns in species richness under environmental gradients and geometric constraints. Ecography. 2005b;28:253–263. 10.1111/j.0906-7590.2005.04038.x [Google Scholar]
- Rangel T.F.L.V.B, Diniz-Filho J.A.F, Bini L.M. Towards an integrated computational tool for spatial analysis in macroecology and biogeography. Global Ecol. Biogeogr. 2006;15:321–327. 10.1111/j.1466-822X.2006.00237.x [Google Scholar]
- Ricklefs R.E. A comprehensive framework for global patterns in biodiversity. Ecol. Lett. 2004;7:1–15. 10.1046/j.1461-0248.2003.00554.x [Google Scholar]
- Rosenzweig M.L. Cambridge University Press; Cambridge, UK: 1995. Species diversity in space and time. [Google Scholar]
- Ruggiero A, Kitzberger T. Environmental correlates of mammal species richness in South America: effects of spatial structure, taxonomy and geographic range. Ecography. 2004;27:401–416. 10.1111/j.0906-7590.2004.03801.x [Google Scholar]
- Tognelli M.F, Kelt D.A. Analysis of determinants of mammalian species richness in South America using spatial autoregressive models. Ecography. 2004;27:427–436. 10.1111/j.0906-7590.2004.03732.x [Google Scholar]
- Vuilleumier F, Simberloff D. Ecology versus history as determinants of patchy and insular distributions in high Andean birds. Evol. Biol. 1980;12:235–379. [Google Scholar]
- Wiens J.J, Donoghue M.J. Historical biogeography, ecology and species richness. Trends Ecol. Evol. 2004;19:639–644. 10.1016/j.tree.2004.09.011 [Abstract] [Google Scholar]
- Williams, P. H. 1996 WORLDMAP 4 WINDOWS: Software and Help Document 4.19 (Privately distributed and available at: http://www.nhm.ac.uk/science/projects/worldmap)
- Willig M.R, Kaufman D.M, Stevens R.D. Latitudinal gradients of biodiversity: pattern, process, scale, and synthesis. Annu. Rev. Ecol. Syst. 2003;34:273–309. 10.1146/annurev.ecolsys.34.012103.144032 [Google Scholar]
- Willis K.J, Whittaker R.J. Species diversity—scale matters. Science. 2002;295:1245. 10.1126/science.1067335 [Abstract] [Google Scholar]
- Woodward F.I, Smith T.M, Emmanuel W.R. A global land primary productivity and phytogeography model. Global Biogeochem. Cycles. 1995;9:471–490. 10.1029/95GB02432 [Google Scholar]
Articles from Proceedings of the Royal Society B: Biological Sciences are provided here courtesy of The Royal Society
Full text links
Read article at publisher's site: https://doi.org/10.1098/rspb.2006.3700
Read article for free, from open access legal sources, via Unpaywall:
https://europepmc.org/articles/pmc1685854?pdf=render
Citations & impact
Impact metrics
Citations of article over time
Alternative metrics

Discover the attention surrounding your research
https://www.altmetric.com/details/102665196
Smart citations by scite.ai
Explore citation contexts and check if this article has been
supported or disputed.
https://scite.ai/reports/10.1098/rspb.2006.3700
Article citations
Unraveling habitat-driven shifts in alpha, beta, and gamma diversity of hummingbirds and their floral resource.
PeerJ, 12:e17713, 10 Jul 2024
Cited by: 1 article | PMID: 39006017 | PMCID: PMC11246024
Exploring the causes underlying the latitudinal variation in range sizes: Evidence for Rapoport's rule in spiny lizards (genus Sceloporus).
PLoS One, 19(7):e0306832, 09 Jul 2024
Cited by: 0 articles | PMID: 38980894 | PMCID: PMC11233011
Idiosyncratic patterns of local species richness and turnover define global biodiversity hotspots.
Proc Natl Acad Sci U S A, 121(3):e2313106121, 08 Jan 2024
Cited by: 2 articles | PMID: 38190521 | PMCID: PMC10801871
Seasonal variation in dragonfly assemblage colouration suggests a link between thermal melanism and phenology.
Nat Commun, 14(1):8427, 19 Dec 2023
Cited by: 1 article | PMID: 38114459 | PMCID: PMC10730518
The geography of climate and the global patterns of species diversity.
Nature, 622(7983):537-544, 27 Sep 2023
Cited by: 5 articles | PMID: 37758942 | PMCID: PMC10584679
Go to all (73) article citations
Other citations
Data
Data behind the article
This data has been text mined from the article, or deposited into data resources.
BioStudies: supplemental material and supporting data
Similar Articles
To arrive at the top five similar articles we use a word-weighted algorithm to compare words from the Title and Abstract of each citation.
Plant and animal endemism in the eastern Andean slope: challenges to conservation.
BMC Ecol, 12:1, 27 Jan 2012
Cited by: 30 articles | PMID: 22284854 | PMCID: PMC3311091
Elevational gradients in bird diversity in the Eastern Himalaya: an evaluation of distribution patterns and their underlying mechanisms.
PLoS One, 6(12):e29097, 13 Dec 2011
Cited by: 27 articles | PMID: 22174953 | PMCID: PMC3236786
Geographic coincidence of richness, mass, conservation value, and response to climate of U.S. land birds.
Ecol Appl, 24(4):791-811, 01 Jun 2014
Cited by: 1 article | PMID: 24988777
Humboldt's enigma: What causes global patterns of mountain biodiversity?
Science, 365(6458):1108-1113, 01 Sep 2019
Cited by: 137 articles | PMID: 31515383
Review