Abstract
Background
Epigenetic changes are emerging as one of the most important events in carcinogenesis. Two alterations in the pattern of DNA methylation in breast cancer (BC) have been previously reported; active estrogen receptor-alpha (ER-alpha) is associated with decreased methylation of ER-alpha target (ERT) genes, and polycomb group target (PCGT) genes are more likely than other genes to have promoter DNA hypermethylation in cancer. However, whether DNA methylation in normal unrelated cells is associated with BC risk and whether these imprints can be related to factors which can be modified by the environment, is unclear.Methodology/principal findings
Using quantitative methylation analysis in a case-control study (n = 1,083) we found that DNA methylation of peripheral blood cell DNA provides good prediction of BC risk. We also report that invasive ductal and invasive lobular BC is characterized by two different sets of genes, the latter particular by genes involved in the differentiation of the mesenchyme (PITX2, TITF1, GDNF and MYOD1). Finally we demonstrate that only ERT genes predict ER positive BC; lack of peripheral blood cell DNA methylation of ZNF217 predicted BC independent of age and family history (odds ratio 1.49; 95% confidence interval 1.12-1.97; P = 0.006) and was associated with ER-alpha bioactivity in the corresponding serum.Conclusion/significance
This first large-scale epigenotyping study demonstrates that DNA methylation may serve as a link between the environment and the genome. Factors that can be modulated by the environment (like estrogens) leave an imprint in the DNA of cells that are unrelated to the target organ and indicate the predisposition to develop a cancer. Further research will need to demonstrate whether DNA methylation profiles will be able to serve as a new tool to predict the risk of developing chronic diseases with sufficient accuracy to guide preventive measures.Free full text

Epigenotyping in Peripheral Blood Cell DNA and Breast Cancer Risk: A Proof of Principle Study
Associated Data
Abstract
Background
Epigenetic changes are emerging as one of the most important events in carcinogenesis. Two alterations in the pattern of DNA methylation in breast cancer (BC) have been previously reported; active estrogen receptor-α (ER-α) is associated with decreased methylation of ER-α target (ERT) genes, and polycomb group target (PCGT) genes are more likely than other genes to have promoter DNA hypermethylation in cancer. However, whether DNA methylation in normal unrelated cells is associated with BC risk and whether these imprints can be related to factors which can be modified by the environment, is unclear.
Methodology/Principal Findings
Using quantitative methylation analysis in a case-control study (n=
1,083) we found that DNA methylation of peripheral blood cell DNA provides good prediction of BC risk. We also report that invasive ductal and invasive lobular BC is characterized by two different sets of genes, the latter particular by genes involved in the differentiation of the mesenchyme (PITX2, TITF1, GDNF and MYOD1). Finally we demonstrate that only ERT genes predict ER positive BC; lack of peripheral blood cell DNA methylation of ZNF217 predicted BC independent of age and family history (odds ratio 1.49; 95% confidence interval 1.12–1.97; P
=
0.006) and was associated with ER-α bioactivity in the corresponding serum.
Conclusion/Significance
This first large-scale epigenotyping study demonstrates that DNA methylation may serve as a link between the environment and the genome. Factors that can be modulated by the environment (like estrogens) leave an imprint in the DNA of cells that are unrelated to the target organ and indicate the predisposition to develop a cancer. Further research will need to demonstrate whether DNA methylation profiles will be able to serve as a new tool to predict the risk of developing chronic diseases with sufficient accuracy to guide preventive measures.
Introduction
Each year, more than 1.15 million new cases of breast cancer are diagnosed worldwide [1]. Currently the Gail model, based on epidemiological risk factors, is the best we have of predicting overall risk of invasive breast cancer and the concordance statistics for correctly classifying women with respect to this outcome is 58–59% [2].
Epigenetic changes, in particular DNA methylation, are emerging as one of the most important events in carcinogenesis [3]–[6]. Recently, an increasing body of evidence from animal studies demonstrated that environmental factors can result in epigenetic modifications and subsequent changes in the risk of developing disease [7], [8]. There is preliminary evidence from small studies that peripheral blood cell DNA contains epigenetic information, which is a valuable predictive marker of an individual's risk of developing cancer [9], [10], [11]. Only the largest of these studies, involving 172 individuals and analyzing one locus, demonstrated that epigenetic alterations are able to predict the risk of colorectal cancer independently of family history [9].
We have undertaken a large scale study to test the hypothesis that methylation in peripheral blood cell DNA may be a predictor of breast cancer risk. Recently, two alterations in the pattern of DNA methylation in breast cancer have been described. First, active estrogen receptor-α (ER-α), which reflects breast cancer risk, is associated with decreased methylation of ER-α target genes (ERT) [12], [13]. Second, polycomb group target genes (PCGT), which play a key role in stem cell biology, are more likely to have promoter DNA hypermethylation in cancer than other genes [14], [15], [16]. These observations led to our hypothesis that the pattern of peripheral blood cell DNA methylation of ERT genes and PCGT genes is an important predictor of breast cancer risk. Based on our previous data we hypothesized that as a function of time and dose, cumulative estrogen exposure during lifetime leaves an epigenetic signature in peripheral white blood cell DNA (depending on a woman's genetic and lifestyle background), which is associated with a postmenopausal risk to develop breast cancer. Physiologically, aging and decrease of estrogens in the postmenopause may lead to methylation of ERT genes. However, lack of methylation may be an indicator for a long-term high estrogen exposure, thus the expectation would be that ERT genes have a lower frequency of methylation in peripheral blood cell DNA of women with breast cancer compared to controls. Furthermore, embryonic stem cells rely on polycomb group proteins to reversibly repress genes required for differentiation. We recently reported that stem cell polycomb group targets are up to 12-fold more likely to have cancer-specific promoter DNA hypermethylation [14], supporting a stem cell origin of cancer where reversible gene expression is replaced by permanent silencing, locking the cell into a perpetual state of self-renewal that predisposes to subsequent malignant transformation. Based on this, our expectation would be that PCGT genes have a higher frequency of methylation in peripheral blood cell DNA of women with breast cancer compared to controls.
In this study we have demonstrated that methylation in peripheral blood cell DNA is related to breast cancer risk. This observation opens opportunities for progress in risk assessment and prevention of breast cancer and is a model for investigation in other chronic diseases.
Results and Discussion
In order to test our hypothesis that peripheral blood cell DNA methylation is predictor of breast cancer risk, we took a three step approach. In step 1 a total of 49 genes were selected as shown in Table 1. These include (a) 19 ERT genes, previously reported to be associated with decreased DNA methylation [12], [13], (b) 4 genes which are not known to be ER-α target genes, but have been demonstrated to be differently methylated depending on hormone receptor status (DMHR) [12], (c) 20 PCGT genes, which play a key role in stem cell biology and are more likely to have promoter DNA hypermethylation in cancer [14], [15], [16] and (d) 6 genes which are known to be methylated in breast cancer (MBC) [12] and are not a member of the other three groups.
Table 1
Gene group | Gene loci | Gene ID Accession # | % methylated | Gene group | Gene loci | Gene ID Accession # | % methylated |
(n![]() ![]() | (n![]() ![]() | ||||||
ERT | BCL2 | 596 | 0 | PCGT | ITGA4 | 3676 | 0 |
MGA (II) | 23269 | 0 | CDKN2C | 1031 | 0 | ||
NUP155 (II) | 9631 | 1 | TWIST1 | 7291 | 1 | ||
BRIP1 (II) | 83990 | 6 | SLC6A20* | 54716 | 5 | ||
ZC3H4 (C19orf7) | 23211 | 7 | CYP1B1* | 1545 | 11 | ||
MGA (I) | 23269 | 11 | NEUROG1* | 4762 | 14 | ||
BRIP1 (I)* | 83990 | 13 | HOXA1* | 3198 | 23 | ||
ESR1* | 2099 | 33 | TITF1* | 7080 | 23 | ||
SIRT3* | 23410 | 36 | GDNF* | 2668 | 24 | ||
NUP155 (I)* | 9631 | 41 | NEUROD1* | 4760 | 31 | ||
PITX2 (II)* | 5308 | 41 | SFRP1* | 6422 | 41 | ||
PITX2 (I)* | 5308 | 42 | MYOD1* | 4654 | 59 | ||
DCC* | 1630 | 46 | CALCA | 796 | 64 | ||
ZNF217 (II)* | 7764 | 54 | HOXA10 | 3206 | 64 | ||
FLJ39739* | 388685 | 58 | SFRP4 | 6424 | 69 | ||
PGR* | 5241 | 77 | ZBTB16 | 7704 | 71 | ||
TRIP10 | 9322 | 95 | SLIT2 | 9353 | 72 | ||
ZNF217 (I) | 7764 | 99 | HIC1 | 3090 | 82 | ||
TFF1 | 7031 | 100 | HOXA11 | 3207 | 82 | ||
MBC | TERT | 7015 | 0 | SFRP5 | 6425 | 83 | |
SYK* | 6850 | 7 | |||||
SEZ6L* | 23544 | 45 | DMHR | TIMP3* | 7078 | 12 | |
GATA5 | 140628 | 61 | CDH13* | 1012 | 20 | ||
SFRP2 | 6423 | 76 | HSD17B4* | 3295 | 27 | ||
CCND2 | 894 | 82 | PTGS2* | 5743 | 77 |








In step 2 we used MethyLight [17] to analyze the 49 genes (Table 1; additional information see Table S1a–b) in peripheral blood cell DNA of 83 healthy postmenopausal women (participating in UKOPS; see Materials and Methods) blinded for any history of cancer. DNA methylation at each locus (expressed as percentage of methylated reference; PMR) was divided into methylated (PMR>0) and unmethylated (PMR=
0), given that methylation was present only in a minority of women for most genes. Based on the hypothesis that lack of methylation of ERT (and DMHR) and methylation of PCGT genes predict breast cancer risk, for further analysis in the case-control study (step 3), we selected those ERT loci with a higher frequency in methylation and those PCGT loci with a lower frequency of methylation in the healthy postmenopausal women analyzed. All 4 DHMR and the 2 MBC gene loci with a lower frequency of methylation in the healthy individuals were also selected for further analysis. On this basis a total of 25 genes were further analyzed in a case-control population (indicated with an asterisk in Table 1).
Step 3 involved conducting a carefully designed case-control study involving 1,083 samples from both healthy women and breast cancer patients provided from the ESTHER study (see Material and Methods). 353 cases and 730 age-matched controls, recruited in a state-wide study in Saarland, Germany, were included in the study. Cases were more likely to have first-degree relatives with breast cancer (odds ratio (OR) 1.90 (95% confidence interval (CI) 1.27–2.85; P=
0.002). In addition, there was a trend towards increased breast cancer risk for women with late menopause (50+ vs. <50 yrs. OR 1.31 (95% CI 0.98–1.75; P
=
0.10). There was no significant difference between cases and controls regarding other features (Table 2). Results were essentially unchanged when all continuous covariates were entered as such in the model. A multiple logistic regression model based on the risk factors addressed in table 2 showed a concordance statistic [18] of 0.585, which is identical with the concordance statistics demonstrated for the Gail model [2]. Jointly, the various risk factors contributed significantly to the prediction of disease status (P-value for likelihood ratio test
=
0.007, 12 degrees of freedom). Clinicopathological features of the cases are equivalent to an average cohort of women with breast cancer (Table S2).
Table 2
Characteristics | Cases | Controls | OR*** | 95% CI | P-value | ||||
n | % | n | % | from | to | ||||
Age (matching variable) | 50–54 | 48 | 13.6 | 100 | 13.7 | ||||
55–59 | 65 | 18.4 | 129 | 17.7 | |||||
60–64 | 99 | 28.1 | 211 | 28.9 | |||||
65–69 | 88 | 24.9 | 182 | 24.9 | |||||
70–74 | 53 | 15.0 | 108 | 14.8 | |||||
1st degree relative with breast cancer | no | 302 | 85.6 | 671 | 91.9 | 1.00 | |||
yes | 51 | 14.4 | 59 | 8.1 | 1.90 | 1.27 | 2.85 | 0.002** | |
Age at menarche* | <12 | 29 | 8.9 | 85 | 12.5 | 1.00 | |||
12–13 | 132 | 40.6 | 264 | 38.8 | 1.45 | 0.91 | 2.31 | ||
14+ | 164 | 50.5 | 331 | 48.7 | 1.44 | 0.88 | 2.36 | 0.39 | |
Age at 1st childbirth* | <20 | 34 | 9.8 | 77 | 10.9 | 1.00 | |||
20–24 | 155 | 44.8 | 361 | 51.3 | 0.95 | 0.61 | 1.49 | ||
25–29 | 77 | 22.3 | 145 | 20.6 | 1.11 | 0.67 | 1.84 | ||
30+ | 80 | 23.1 | 121 | 17.2 | 1.28 | 0.76 | 2.15 | 0.18 | |
Age at menopause* | <50 | 172 | 52.3 | 403 | 58.8 | 1.00 | |||
50+ | 157 | 47.7 | 283 | 41.2 | 1.31 | 0.98 | 1.75 | 0.10 | |
BMI at age 20* | <18.5 | 61 | 17.9 | 101 | 15.1 | 1.00 | |||
18.5−<22.5 | 190 | 55.7 | 369 | 55.3 | 0.88 | 0.61 | 1.28 | ||
22.5+ | 90 | 26.4 | 197 | 29.5 | 0.86 | 0.54 | 1.23 | 0.34 | |
Ever breastfed* | no | 148 | 42.5 | 263 | 36.9 | 1.00 | |||
yes | 200 | 57.5 | 449 | 63.1 | 0.85 | 0.64 | 1.13 | 0.20 | |
Ever use of hormone replacement therapy* | no | 152 | 46.8 | 348 | 50.4 | 1.00 | |||
yes | 173 | 53.2 | 343 | 49.6 | 1.15 | 0.87 | 1.51 | 0.27 |








Using PMR values, 7 of the 25 genes analyzed in stage 3 demonstrated significant differences between the 353 cases and 730 controls (Table S3). 6 of these 7 genes retained their significant differences after dividing each locus into methylated (PMR>0) or unmethylated (PMR=
0) and adjusting for age (Table 3). 5 of those were significantly associated with breast cancer risk even after adjusting for family history of breast cancer (Table 3), and after additional control for all the other established breast cancer risk factors (ZNF217: OR 1.48, 95% CI 1.12–1.97, P
=
0.006; NEUROD1: OR 1.49, 95% CI 1.11–2.02, P
=
0.009; SFRP1: OR 1.44, 95% CI 1.07–1.92, P
=
0.015; TITF1: OR 1.49, 95% CI 1.02–2.18, P
=
0.038; NUP155: OR 1.40, 95% CI 1.02–1.94, P
=
0.040). A model solely based on the methylation markers of all 25 loci (methylated vs. unmethylated) showed a concordance statistic of 0.628. Jointly, the methylation status of the 25 genes was significantly related to disease risk (P-value for likelihood ratio test with 25 degrees of freedom
=
0.048). The combination of both risk factors and methylation status increased the concordance statistic to 0.647. The likelihood ratio test for adding traditional risk factors (family history, age at menarche, age at 1st childbirth, age at menopause, BMI at age 20, history of breastfeeding, history of hormone replacement therapy, 7 degrees of freedom) to a model including methylation status of the 25 genes and the matching factor age yielded a P-value of 0.011. Conversely, the likelihood ratio test for adding methylation status of the 25 genes (25 degrees of freedom) to a model including traditional risk factors yielded a P-value of 0.057.
Table 3
Gene group | Gene loci | Presence of methylation in cases (all) | Presence of methylation in controls | Adjusted for age | Adjusted for age and family history | ||
OR (95% CI) | P-value | OR (95% CI) | P-value | ||||
ERT | BRIP1 (I) | 31%(95/306) | 30.5%(199/653) | 0.97(0.72–1.31) | 0.852 | 0.97(0.72–1.31) | 0.85 |
ESR1 | 12.2%(39/320) | 13.5%(91/676) | 1.1(0.73–1.65) | 0.645 | 1.09(0.73–1.64) | 0.678 | |
SIRT3 | 20.7%(61/294) | 16.3%(102/627) | 0.74(0.52–1.06) | 0.1 | 0.74(0.52–1.06) | 0.099 | |
NUP155 (I) | 21.9%(67/306) | 28.6%(187/653) | 1.42(1.03–1.96) | 0.031* | 1.4(1.02–1.94) | 0.038* | |
PITX2 (I) | 33.1%(106/320) | 38%(257/676) | 1.24(0.94–1.64) | 0.133 | 1.23(0.92–1.63) | 0.157 | |
PITX2 (II) | 48.1%(154/320) | 48.5%(328/676) | 1.01(0.78–1.33) | 0.916 | 1.02(0.78–1.33) | 0.913 | |
DCC | 35.1%(106/302) | 41.7%(266/638) | 1.32(0.99–1.75) | 0.059 | 1.29(0.96–1.71) | 0.086 | |
ZNF217 (II) | 39.4%(119/302) | 48.9%(312/638) | 1.49(1.13–1.97) | 0.005* | 1.49(1.12–1.97) | 0.006* | |
FLJ39739 | 52%(153/294) | 49.4%(310/627) | 0.9(0.68–1.19) | 0.474 | 0.9(0.68–1.19) | 0.454 | |
PGR | 69.7%(223/320) | 70.7%(478/676) | 1.05(0.78–1.4) | 0.754 | 1.04(0.77–1.39) | 0.808 | |
DMHR | TIMP3 | 12.5%(40/320) | 14.2%(96/676) | 1.14(0.77–1.7) | 0.511 | 1.13(0.76–1.69) | 0.537 |
CDH13 | 13.8%(44/320) | 15.4%(104/676) | 1.15(0.78–1.68) | 0.485 | 1.15(0.79–1.69) | 0.462 | |
HSD17B4 | 17.5%(56/320) | 14.3%(97/676) | 0.79(0.55–1.13) | 0.19 | 0.78(0.55–1.13) | 0.189 | |
PTGS2 | 71.3%(228/320) | 77.1%(521/676) | 1.35(1–1.83) | 0.049* | 1.31(0.97–1.78) | 0.078 | |
PCGT | SLC6A20 | 0.9%(3/320) | 1.8%(12/676) | 1.94(0.54–6.94) | 0.307 | 2.2(0.61–7.96) | 0.23 |
NEUROG1 | 4.3%(13/302) | 4.7%(30/638) | 1.1(0.56–2.14) | 0.783 | 1.08(0.55–2.11) | 0.822 | |
HOXA1 | 11.1%(34/306) | 13.9%(91/653) | 1.28(0.84–1.96) | 0.247 | 1.27(0.83–1.94) | 0.272 | |
TITF1 | 13.8%(44/320) | 19.5%(132/676) | 1.53(1.05–2.22) | 0.025* | 1.52(1.05–2.21) | 0.028* | |
GDNF | 14.7%(45/306) | 18.7%(122/653) | 1.32(0.91–1.92) | 0.147 | 1.3(0.89–1.89) | 0.176 | |
NEUROD1 | 30.5%(91/298) | 38.9%(250/642) | 1.45(1.08–1.94) | 0.014* | 1.47(1.09–1.98) | 0.011* | |
SFRP1 | 29.4%(94/320) | 37.4%(253/676) | 1.44(1.08–1.91) | 0.014* | 1.44(1.08–1.93) | 0.013* | |
MYOD1 | 60%(192/320) | 63.5%(429/676) | 1.18(0.89–1.55) | 0.244 | 1.17(0.89–1.54) | 0.261 | |
MBC | SYK | 2.2%(7/320) | 2.4%(16/676) | 1.07(0.43–2.62) | 0.889 | 1.06(0.43–2.62) | 0.9 |
CYP1B1 | 7.5%(24/320) | 4.9%(33/676) | 0.63(0.37–1.09) | 0.096 | 0.62(0.36–1.08) | 0.09 | |
SEZ6L | 50.3%(154/306) | 52.8%(345/653) | 1.1(0.84–1.45) | 0.482 | 1.1(0.83–1.44) | 0.517 |








Taken together, these patterns suggest exactly what we had expected based on the selection of genes we used for this study: The prediction of risk by traditional risk factors and methylation patterns are not entirely independent. This again supports our hypothesis that DNA methylation, on one hand may act as a surrogate for genetic risk and lifelong environmental exposure, but on the other hand, independently reflects the individual response of women to these factors. In this context the results of the first genome-wide-breast cancer susceptibility study which was recently reported are relevant. The study identified 5 novel breast cancer susceptibility loci after a three stage procedure, which started with analysis of 227,876 single nucleotide polymorphisms (SNPs). The per allele odds ratio for an association with breast cancer for the top five SNPs was between 1.07 and 1.26 [19]. This study suggests that it is unlikely that genetic tests alone will achieve the sensitivity and specificity required for risk assessment to guide preventive measures.
Furthermore, the two main histological subtypes in breast cancer are invasive ductal and invasive lobular. It is known that there are specific carcinogenic pathways for these two breast cancer groups. There is growing evidence suggesting that a change in tumor tissue architecture takes place in the genesis of invasive lobular breast cancer known as epithelial-mesenchymal transition [20]. To study whether this is also reflected in the epigenotype of peripheral blood cells, we calculated separate ORs which predict each histological subtype (Table S4a–b). The genes which predicted invasive ductal breast cancer (ZNF217, NEUROD1, SFRP1) were completely different from the genes associated with invasive lobular cancer (PITX2, TITF1, GDNF, MYOD1, DCC). Interestingly genes which predicted invasive lobular cancer are involved in diseases that affect the mesenchyme like Hirschsprung disease or are known to be involved in epithelial-mesenchymal transition [21]–[25].
Beside histology, estrogen receptor status is the most important feature in breast cancer. It is known that estrogen exposure (e.g. serum estradiol in postmenopausal women) is only associated with ER positive breast cancer [26]. Therefore we tested which of the 25 genes predict ER positive and which predict ER negative breast cancer. Interestingly only the ERT gene loci (ZNF217 OR 1.48 (95% CI 1.01–2.16; P=
0.042) and NUP155 OR 1.46 (95% CI 1.05–2.02; P
=
0.023) predicted ER positive breast cancer (Table 4), whereas a PCGT gene locus (SFRP1 OR 2.37 (95% CI 1.23–4.57; P
=
0.01) predicted ER negative breast cancer (Table 5).
Table 4
Gene group | Gene loci | Presence of methylation in cases (ER positive) | Presence of methylation in controls | Adjusted for age | Adjusted for age and family history | ||
OR (95% CI) | P- value | OR (95% CI) | P- value | ||||
ERT | BRIP1 (I) | 30% (61/203) | 30.5% (199/653) | 1.01 (0.72–1.43) | 0.943 | 1.01 (0.72–1.43) | 0.942 |
ESR1 | 11.3% (24/212) | 13.5% (91/676) | 1.23 (0.76–1.99) | 0.404 | 1.22 (0.75–1.98) | 0.419 | |
SIRT3 | 18.6% (36/194) | 16.3% (102/627) | 0.84 (0.55–1.27) | 0.405 | 0.83 (0.54–1.27) | 0.384 | |
NUP155 (I) | 21.2% (43/203) | 28.6% (187/653) | 1.48 (1.01–2.16) | 0.042* | 1.46 (1–2.13) | 0.052 | |
PITX2 (I) | 32.5% (69/212) | 38% (257/676) | 1.27 (0.92–1.77) | 0.151 | 1.25 (0.9–1.74) | 0.189 | |
PITX2 (II) | 49.1% (104/212) | 48.5% (328/676) | 0.99 (0.73–1.35) | 0.951 | 0.97 (0.71–1.33) | 0.865 | |
DCC | 37.5% (75/200) | 41.7% (266/638) | 1.19 (0.86–1.66) | 0.291 | 1.15 (0.82–1.6) | 0.41 | |
ZNF217 (II) | 40% (80/200) | 48.9% (312/638) | 1.46 (1.05–2.02) | 0.023* | 1.44 (1.04–1.99) | 0.029* | |
FLJ39739 | 52.6% (102/194) | 49.4% (310/627) | 0.88 (0.63–1.21) | 0.43 | 0.87 (0.63–1.21) | 0.413 | |
PGR | 69.3% (147/212) | 70.7% (478/676) | 1.07 (0.76–1.5) | 0.69 | 1.05 (0.75–1.47) | 0.789 | |
DMHR | TIMP3 | 11.3% (24/212) | 14.2% (96/676) | 1.27 (0.79–2.05) | 0.327 | 1.26 (0.78–2.04) | 0.347 |
CDH13 | 12.7% (27/212) | 15.4% (104/676) | 1.28 (0.81–2.01) | 0.296 | 1.28 (0.81–2.03) | 0.288 | |
HSD17B4 | 17% (36/212) | 14.3% (97/676) | 0.81 (0.53–1.23) | 0.323 | 0.8 (0.53–1.23) | 0.31 | |
PTGS2 | 72.2% (153/212) | 77.1% (521/676) | 1.31 (0.92–1.87) | 0.129 | 1.26 (0.88–1.79) | 0.205 | |
PCGT | SLC6A20 | 0.9% (2/212) | 1.8% (12/676) | 1.98 (0.44–8.92) | 0.376 | 2.31 (0.5–10.61) | 0.28 |
NEUROG1 | 5% (10/200) | 4.7% (30/638) | 0.91 (0.43–1.9) | 0.794 | 0.89 (0.43–1.87) | 0.762 | |
HOXA1 | 11.3% (23/203) | 13.9% (91/653) | 1.26 (0.77–2.06) | 0.349 | 1.25 (0.76–2.04) | 0.378 | |
TITF1 | 15.6% (33/212) | 19.5% (132/676) | 1.34 (0.88–2.04) | 0.173 | 1.33 (0.87–2.02) | 0.189 | |
GDNF | 15.8% (32/203) | 18.7% (122/653) | 1.23 (0.8–1.89) | 0.339 | 1.2 (0.78–1.85) | 0.406 | |
NEUROD1 | 32% (63/197) | 38.9% (250/642) | 1.35 (0.96–1.9) | 0.085 | 1.37 (0.97–1.94) | 0.071 | |
SFRP1 | 32.1% (68/212) | 37.4% (253/676) | 1.26 (0.91–1.76) | 0.165 | 1.27 (0.91–1.77) | 0.161 | |
MYOD1 | 59% (125/212) | 63.5% (429/676) | 1.24 (0.91–1.71) | 0.177 | 1.23 (0.89–1.69) | 0.203 | |
MBC | SYK | 2.8% (6/212) | 2.4% (16/676) | 0.82 (0.32–2.14) | 0.691 | 0.82 (0.31–2.15) | 0.688 |
CYP1B1 | 8% (17/212) | 4.9% (33/676) | 0.58 (0.31–1.06) | 0.075 | 0.57 (0.31–1.06) | 0.076 | |
SEZ6L | 50.2% (102/203) | 52.8% (345/653) | 1.11 (0.81–1.53) | 0.514 | 1.1 (0.8–1.51) | 0.569 |














Table 5
Gene group | Gene loci | Presence of methylation in cases (ER negative) | Presence of methylation in controls | Adjusted for age | Adjusted for age and family history | ||
OR (95% CI) | P-value | OR (95% CI) | P- value | ||||
ERT | BRIP1 (I) | 31.6% (18/57) | 30.5% (199/653) | 0.95 (0.53–1.72) | 0.875 | 0.95 (0.53–1.71) | 0.866 |
ESR1 | 11.7% (7/60) | 13.5% (91/676) | 1.07 (0.47–2.43) | 0.88 | 1.06 (0.47–2.43) | 0.881 | |
SIRT3 | 24.6% (14/57) | 16.3% (102/627) | 0.64 (0.34–1.22) | 0.174 | 0.64 (0.34–1.23) | 0.18 | |
NUP155 (I) | 21.1% (12/57) | 28.6% (187/653) | 1.51 (0.78–2.92) | 0.224 | 1.51 (0.78–2.92) | 0.225 | |
PITX2 (I) | 33.3% (20/60) | 38% (257/676) | 1.24 (0.7–2.18) | 0.457 | 1.23 (0.7–2.17) | 0.468 | |
PITX2 (II) | 46.7% (28/60) | 48.5% (328/676) | 1.04 (0.61–1.78) | 0.873 | 1.04 (0.61–1.78) | 0.876 | |
DCC | 29.8% (17/57) | 41.7% (266/638) | 1.67 (0.92–3.01) | 0.091 | 1.66 (0.91–3) | 0.096 | |
ZNF217 (II) | 36.8% (21/57) | 48.9% (312/638) | 1.59 (0.91–2.8) | 0.106 | 1.61 (0.91–2.83) | 0.1 | |
FLJ39739 | 49.1% (28/57) | 49.4% (310/627) | 1.02 (0.59–1.77) | 0.934 | 1.03 (0.59–1.78) | 0.926 | |
PGR | 73.3% (44/60) | 70.7% (478/676) | 0.9 (0.49–1.64) | 0.735 | 0.9 (0.49–1.64) | 0.732 | |
DMHR | TIMP3 | 16.7% (10/60) | 14.2% (96/676) | 0.87 (0.42–1.79) | 0.708 | 0.87 (0.42–1.79) | 0.706 |
CDH13 | 15% (9/60) | 15.4% (104/676) | 1.03 (0.49–2.17) | 0.933 | 1.03 (0.49–2.16) | 0.941 | |
HSD17B4 | 21.7% (13/60) | 14.3% (97/676) | 0.59 (0.31–1.14) | 0.119 | 0.59 (0.31–1.14) | 0.119 | |
PTGS2 | 71.7% (43/60) | 77.1% (521/676) | 1.3 (0.72–2.36) | 0.38 | 1.3 (0.72–2.35) | 0.387 | |
PCGT | SLC6A20 | 0% (0/60) | 1.8% (12/676) | ||||
NEUROG1 | 0% (0/57) | 4.7% (30/638) | |||||
HOXA1 | 14% (8/57) | 13.9% (91/653) | 0.97 (0.44–2.14) | 0.947 | 0.97 (0.44–2.12) | 0.932 | |
TITF1 | 8.3% (5/60) | 19.5% (132/676) | 2.53 (0.99–6.48) | 0.053 | 2.53 (0.99–6.48) | 0.053 | |
GDNF | 10.5% (6/57) | 18.7% (122/653) | 1.85 (0.77–4.43) | 0.168 | 1.84 (0.77–4.4) | 0.172 | |
NEUROD1 | 28.1% (16/57) | 38.9% (250/642) | 1.66 (0.91–3.04) | 0.098 | 1.66 (0.91–3.04) | 0.099 | |
SFRP1 | 20% (12/60) | 37.4% (253/676) | 2.37 (1.23–4.57) | 0.01* | 2.37 (1.23–4.57) | 0.01* | |
MYOD1 | 65% (39/60) | 63.5% (429/676) | 0.94 (0.54–1.65) | 0.839 | 0.94 (0.54–1.65) | 0.828 | |
MBC | SYK | 1.7% (1/60) | 2.4% (16/676) | 1.28 (0.16–9.91) | 0.816 | 1.26 (0.16–9.79) | 0.826 |
CYP1B1 | 6.7% (4/60) | 4.9% (33/676) | 0.71 (0.24–2.09) | 0.529 | 0.71 (0.24–2.1) | 0.534 | |
SEZ6L | 50.9% (29/57) | 52.8% (345/653) | 1.07 (0.62–1.85) | 0.799 | 1.07 (0.62–1.84) | 0.815 |














Given the relationship between ERT genes and breast cancer risk we considered the possibility that current serum estrogen activity may have an impact on DNA methylation of these genes. To assess this possibility we analyzed ER-α bioactivity in the serum of the cases and controls using a functional assay, which measures the potential for binding to ER-α and transactivating the estrogen responsive elements (EREs). This ER-α ERE-GFP (green fluorescent protein) reporter test system in Saccharomyces cerevisiae has been recently described [27], [28]. Only peripheral blood cell DNA methylation of ZNF217, one of the 10 ERT genes, demonstrated a significant inverse correlation (ρ=
−0.112; P
=
0.046) with ER-α bioactivity in the corresponding serum (Figure 1). The possibility that this correlation could be triggered by the current use of hormone replacement therapy was considered. After excluding women currently taking hormone replacement therapy, the inverse correlation remained significant (ρ
=
−0.166; P
=
0.013). Our data suggest that lack of ZNF217 methylation is a long-term surrogate marker of estrogen exposure, indicating a woman's risk of breast cancer. This observation fits with evidence for changes in the activity of ZNF217 in breast carcinogenesis. ZNF217 encodes a transcription factor which mainly represses genes involved in differentiation [29]. Introduction of ZNF217 into human mammary epithelial cells leads to immortalization [30]. In addition, ZNF217 is amplified and overexpressed in 40% and 18% of breast cancer cell lines and tissues, respectively [31], [32].
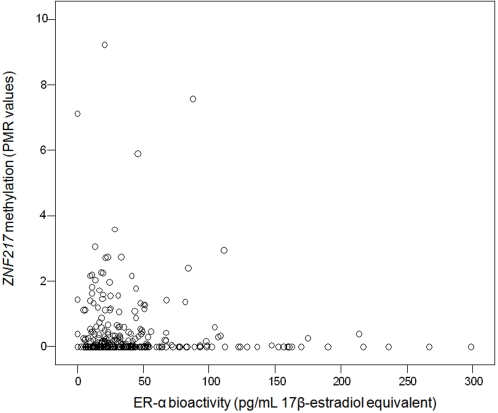
Peripheral blood cell DNA methylation of ZNF217, one of the estrogen receptor target genes studied, demonstrated a significant inverse correlation with ER-α bioactivity in the corresponding serum (ρ=
−0.112; P
=
0.046).
Interestingly, analyzing solely women currently taking hormone replacement therapy, PGR, which is also an ERT gene, was the only locus demonstrating significant inverse correlation with corresponding serum ER-α bioactivity (ρ=
−0.242; P
=
0.044). Peripheral blood cell DNA methylation of PGR, the gene coding for the progesterone receptor whose expression is strongly regulated by ER-α, was not linked to breast cancer risk. PGR methylation is not significantly different between non-neoplastic breast tissue and breast cancer in premenopausal women [14], but PGR methylation analyzed in breast cancer tissue adjusted for age and expression of progesterone receptor is the most significant predictor of ER status in breast cancer [12]. Using logistic regression and after adjustment for age and progesterone receptor status, lack of peripheral blood cell PGR methylation predicted the ER status of the corresponding breast cancer (OR 1.52; 95% CI 1.10–2.11; P
=
0.012), whereas none of the other 24 gene loci's methylation status was an ER status predictor. This finding indicates that the epigenetic pathways and interactions, which have previously only been identified in cancer tissue, are also reflected in peripheral blood, a source of DNA completely unrelated to the breast cancer tissue.
The results did not however confirm the hypothesis that peripheral blood cell DNA methylation of PCGT genes is associated with an increased breast cancer risk. One possible explanation for this could be that lack of methylation at specific gene loci is only a surrogate for general global hypomethylation. This hypothesis is supported by data demonstrating that lack of folic acid supply (a condition leading to depletion of available methyl groups and subsequent global hypomethylation) is associated with some forms of cancer [33]. In order to study global methylation we analyzed peripheral blood cell DNA methylation of ALU repetitive elements, which has recently been demonstrated to be an excellent marker for global methylation [34]. None of the single predictive loci was associated with global methylation in the 349 samples analyzed for methylation of ALU (Table 6). ALU methylation did not differ between cases and controls (controls (n=
180), median PMR 54.8 and interquartile range 34.0–84.0; cases (n
=
169), median PMR 51.1 and interquartile range 34.7–73.0; Wilcoxon-Mann-Whitney test P
=
0.25). This again indicates that the predictive potential of DNA methylation is only reflected by specific gene loci rather than by a surrogate marker which reflects global single-carbon metabolism.
Table 6
Gene group | Gene loci | Cases & Controls | Cases only | Controls only | |||
n![]() ![]() |
n![]() ![]() |
n![]() ![]() | |||||
rho | P-value | rho | P-value | rho | P-value | ||
ERT | BRIP1 (I) | 0.037 | 0.501 | 0.035 | 0.657 | 0.047 | 0.543 |
ESR1 | 0.125 | 0.0204* | 0.162 | 0.0361* | 0.105 | 0.164 | |
SIRT3 | 0.062 | 0.272 | 0.001 | 0.987 | 0.115 | 0.142 | |
NUP155 (I) | 0.004 | 0.946 | 0.031 | 0.692 | −0.026 | 0.732 | |
PITX2 (I) | 0.017 | 0.752 | 0.031 | 0.691 | 0.006 | 0.938 | |
PITX2 (II) | 0.019 | 0.720 | −0.087 | 0.263 | 0.120 | 0.111 | |
DCC | 0.040 | 0.477 | 0.026 | 0.740 | 0.054 | 0.493 | |
ZNF217 (II) | 0.023 | 0.681 | −0.012 | 0.880 | 0.041 | 0.601 | |
FLJ39739 | −0.048 | 0.395 | −0.080 | 0.319 | −0.012 | 0.875 | |
PGR | −0.061 | 0.255 | −0.149 | 0.055 | 0.014 | 0.851 | |
DMHR | TIMP3 | 0.028 | 0.780 | −0.023 | 0.772 | 0.058 | 0.441 |
CDH13 | −0.060 | 0.267 | 0.045 | 0.560 | −0.146 | 0.052 | |
HSD17B4 | 0.012 | 0.828 | 0.115 | 0.138 | −0.095 | 0.206 | |
PTGS2 | 0.094 | 0.082 | 0.119 | 0.126 | 0.070 | 0.356 | |
PCGT | SLC6A20 | −0.030 | 0.578 | −0.063 | 0.416 | −0.006 | 0.937 |
NEUROG1 | 0.062 | 0.261 | 0.034 | 0.692 | 0.109 | 0.162 | |
HOXA1 | −0.049 | 0.373 | 0.042 | 0.598 | −0.131 | 0.087 | |
TITF1 | −0.015 | 0.780 | −0.019 | 0.804 | −0.011 | 0.884 | |
GDNF | −0.029 | 0.599 | −0.051 | 0.518 | −0.020 | 0.799 | |
NEUROD1 | 0.037 | 0.509 | 0.064 | 0.423 | 0.001 | 0.986 | |
SFRP1 | −0.046 | 0.396 | −0.148 | 0.056 | 0.045 | 0.553 | |
MYOD1 | 0.043 | 0.431 | −0.026 | 0.742 | 0.099 | 0.189 | |
MBC | SYK | 0.007 | 0.901 | 0.057 | 0.465 | −0.031 | 0.682 |
CYP1B1 | 0.074 | 0.267 | 0.080 | 0.306 | 0.068 | 0.364 | |
SEZ6L | −0.129 | 0.018* | −0.167 | 0.034* | −0.093 | 0.227 |












It is worth noting that the samples used in this study were obtained after the diagnosis of breast cancer. In women with metastatic breast cancer, tumor cells (5 tumor cells in 7.5 mL blood) can be identified in the peripheral blood [35]. This means that on average 1 out of 40 million cells in the peripheral blood of women with metastatic (less in non-metastatic) are breast cancer cells. Although MethyLight is very sensitive, this assay is only capable of detecting methylated alleles in the presence of a 10,000 -fold excess of unmethylated alleles [17]. The possibility that the methylation signal we detect in peripheral blood cell DNA is influenced by tumor cell DNA can therefore be discounted.
In this first large scale epigenotyping study, we were able to demonstrate that particular DNA methylation patterns in peripheral blood may serve as a surrogate marker for breast cancer risk. The current report also provides a basis for further research to assess the role of a combination of genotyping and epigenotyping as a clinical tool to predict an individual's risk of developing breast cancer, other cancers and chronic diseases with sufficient accuracy to guide preventive measures.
Materials and Methods
Samples
UKOPS (United Kingdom Ovarian Cancer Population Study) study is being carried out in 10 regional centers in England, Wales and Northern Ireland. Recruitment has started since January 2006 and is aiming to include 2,000 ovarian cancer patients, 1,500 benign and 5,000 controls. Recruitment of cancer cases has been carried out during visits to the Gynecological Oncology wards. Control participants have been recruited from the UKCTOCS (United Kingdom Collaborative Trial of Ovarian Cancer Screening) trial centers (www.ukctocs.org.uk). Detailed information about the medical history was obtained by a standardized questionnaire. Diagnosis data from histology and cytology reports has also been included. Furthermore, serum and whole blood were collected from all subjects. Written consent was obtained from each participant. Ethical approval was received by the joint University College London Committees on the Ethics of Human Research (Committee A). In the current study 83 healthy postmenopausal women (blinded for cancer history) were selected for step 2.
ESTHER (www.esther.dkfz.org/esther/) is a population-based study carried out in the state of Saarland (located in South West Germany). In the clinical arm of the study, 1,981 cancer patients age 50 to 75, including 380 women with breast cancer, were recruited during their first stay in the hospital for primary cancer treatment. In the community arm, of the study, 9,953 women and men age 50–75 were recruited during routine health examinations by their general practitioners. Recruitment and baseline examinations were carried out in 2000–2003. In both study arms, detailed information about medical history, including family history, sociodemographic and lifestyle factors and current health status was obtained by standardized questionnaire. In addition, serum and whole blood samples were collected. Written informed consent was obtained from all subjects. The protocol was approved by the Ethics Committee of the Medical Faculty of the University of Heidelberg. Germany. In this study, all 353 cases with postmenopausal invasive breast cancer were included, and a stratified random subset of 730 age-matched postmenopausal women were selected as controls.
DNA isolation and storage of DNA
A standard chloroform-based DNA isolation protocol has been used to extract DNA from whole blood from the UKOPS samples. DNA was extracted from whole blood samples of the ESTHER study participants by Invisorb extraction kits (Invitek; www.invitek.de). DNA from both sample collections have been dissolved in distilled water and stored at −80°C until analysis.
Analysis of DNA Methylation
Sodium bisulfite conversion (Zymo Research; www.zymoresearch.com), MethyLight analysis (Applied Biosystems; www.appliedbiosystems.com) and nucleotide sequences for most MethyLight primers and probes in the promoter or 5′ end region was described recently [12], [14], [34], [36]. Each MethyLight reaction at a specific locus covers on average 5–7 CpG dinucleotides. A detailed list of primer and probes (Metabion; www.metabion.com) for all analyzed loci is provided in Table S1. Briefly two sets of primers and probes, designed specifically for bisulfite-converted DNA, have been used: a methylated set for the gene of interest and a reference set (COL2A1) to normalize for input DNA. Specificity of the reactions for methylated DNA was confirmed separately using SssI (New England Biolabs; www.newenglandbiolabs.co.uk) treated human white blood cell DNA (heavily methylated). The percentage of fully methylated molecules at a specific locus were calculated by dividing the GENECOL2A1 ratio of a sample by the GENE
COL2A1 ratio of the SssI-treated human white blood cell DNA and multiplied by 100. The abbreviation PMR (Percentage of Methylated Reference) indicates this measurement.
The analysis was performed blinded and cases and controls were randomly mixed for bisulfite treatment and real-time PCR. The concentration of bisulfite modified DNA (assessed by the level of the reference gene COL2A1) was the same between cases and controls.
ER-α serum bioactivity assay
ER-α bioactivity has been as recently described [27]. Each serum has been tested blindly in quadruplicates (20 µL for each reaction in a total of 100 µL) and a mean value has been calculated based on four independent measures (done on two different days).
Statistical Analysis
Descriptive analyses were performed on age and known risk factors for breast cancer among cases and controls and on clinical features among breast cancer cases. Percent methylated, mean and median values of PMR were calculated for the selected gene loci. Differences in PMR values between cases and controls were analyzed by means of Wilcoxon-Mann-Whitney test. In addition, odds ratios for breast cancer (overall and according to estrogen receptor status) associated with absence of methylation, adjusted for age (matching factor) and for positive family history (at least one first degree relative with breast cancer) as well as for other established breast cancer risk factors were calculated by multiple logistic regression. Multiple imputation was employed to deal with missing covariate data in multivariable analyses. Initially, a linear term for percent methylation in addition to the dichotomous term for absence of methylation was included in the models to address a potential dose-response relationship. As this term did not significantly improve prediction for any of the 25 genes assessed, it was dropped from the final models. Joint contribution of methylation status of all 25 genes to the prediction of breast cancer risk was evaluated by concordance statistics [18] and likelihood ratio tests. Spearman's rho was calculated to assess the correlation of global methylation (ALU repetitive elements) with methylation at single gene loci. All statistical analyses were done using SAS Software, version 8.2.
Supporting Information
Table S1
Information on genes analyzed. S1A: Primers and probe sequences for MethyLight. S1B: General gene information. Gene alternative names, chromosomal location and amplicons' position relative to the transcription start site are indicated.
(0.14 MB DOC)
Table S2
Clinicopathological characteristics of breast cancer cases used for the study. UICC=
Union internationale contre le cancer; *Among those with information; n/a
=
not applicable.
(0.05 MB DOC)
Table S3
Mean and median percentage of methylated reference (PMR) value of the 25 different loci. 7 genes of those analyzed demonstrated significant differences in the level of methylation between cases and controls, based on the quantitative results on peripheral blood cell DNA. ERT=
estrogen receptor-α target; DMHR
=
differently methylated depending on hormone receptor status; PCGT
=
stem cell polycomb group target; MBC
=
methylated in breast cancer; * indicates P-values <0.05
(0.08 MB DOC)
Table S4
Odds ratios for invasive ductal and lobular breast cancer risk associated with peripheral blood DNA methylation. S4A: Risk for invasive ductal breast cancer. S4B: Risk for invasive lobular breast cancer.
(0.14 MB DOC)
Acknowledgments
We thank A. Jones for technical support and H. Lichtenberg-Frate and her team for performing the serum ER-α bioactivity assays.
Footnotes
Competing Interests: The authors have declared that no competing interests exist.
Funding: The work described in this manuscript was supported by The Eve Appeal and The Oak Foundation and grants from the European Union and the FWF Austrian Science Fund (awarded to M. Widschwendter) and by a grant from the Baden-Württemberg Ministry of Research, Science and Arts (awarded to H. Brenner). The funders did not play any role in the design or conduct of the study.
References
Articles from PLOS ONE are provided here courtesy of PLOS
Full text links
Read article at publisher's site: https://doi.org/10.1371/journal.pone.0002656
Read article for free, from open access legal sources, via Unpaywall:
https://journals.plos.org/plosone/article/file?id=10.1371/journal.pone.0002656&type=printable
Citations & impact
Impact metrics
Citations of article over time
Alternative metrics
Smart citations by scite.ai
Explore citation contexts and check if this article has been
supported or disputed.
https://scite.ai/reports/10.1371/journal.pone.0002656
Article citations
Novel DNA methylation-based epigenetic signatures in colorectal cancer from peripheral blood leukocytes.
Am J Cancer Res, 14(5):2253-2271, 15 May 2024
Cited by: 0 articles | PMID: 38859857
DNA methylation in necrotizing enterocolitis.
Expert Rev Mol Med, 26:e16, 01 Apr 2024
Cited by: 1 article | PMID: 38557638 | PMCID: PMC11140546
Review Free full text in Europe PMC
The impact of physical activity on promoter-specific methylation of genes involved in the redox-status and disease progression: A longitudinal study on post-surgery female breast cancer patients undergoing medical treatment.
Redox Biol, 70:103033, 05 Jan 2024
Cited by: 3 articles | PMID: 38211440 | PMCID: PMC10821067
DNA Methylation Biomarkers as Prediction Tools for Therapeutic Response and Prognosis in Intermediate-Stage Hepatocellular Carcinoma.
Cancers (Basel), 15(18):4465, 07 Sep 2023
Cited by: 2 articles | PMID: 37760434 | PMCID: PMC10527266
A multiplex blood-based assay targeting DNA methylation in PBMCs enables early detection of breast cancer.
Nat Commun, 14(1):4724, 07 Aug 2023
Cited by: 12 articles | PMID: 37550304 | PMCID: PMC10406825
Go to all (99) article citations
Data
Data behind the article
This data has been text mined from the article, or deposited into data resources.
BioStudies: supplemental material and supporting data
Similar Articles
To arrive at the top five similar articles we use a word-weighted algorithm to compare words from the Title and Abstract of each citation.
Clinicopathological significance and potential drug target of CDH1 in breast cancer: a meta-analysis and literature review.
Drug Des Devel Ther, 9:5277-5285, 18 Sep 2015
Cited by: 13 articles | PMID: 26425077 | PMCID: PMC4583122
Review Free full text in Europe PMC
Epigenetic Changes of the ESR1 Gene in Breast Tissue of Healthy Women: A Missing Link with Breast Cancer Risk Factors?
Genet Test Mol Biomarkers, 21(8):464-470, 14 Jul 2017
Cited by: 10 articles | PMID: 28708432
Promoter hypermethylation of TMS1, BRCA1, ERalpha and PRB in serum and tumor DNA of invasive ductal breast carcinoma patients.
Life Sci, 81(4):280-287, 25 May 2007
Cited by: 66 articles | PMID: 17599361
Single CpG hypermethylation, allele methylation errors, and decreased expression of multiple tumor suppressor genes in normal body cells of mutation-negative early-onset and high-risk breast cancer patients.
Int J Cancer, 143(6):1416-1425, 25 Apr 2018
Cited by: 11 articles | PMID: 29659014 | PMCID: PMC6099327