Abstract
Free full text

Dopamine-dependent prediction errors underpin reward-seeking behaviour in humans
Theories of instrumental learning are centred on understanding how success and failure are used to improve future decisions1. These theories highlight a central role for reward prediction errors in updating the values associated with available actions2. In animals, substantial evidence indicates that the neurotransmitter dopamine might have a key function in this type of learning, through its ability to modulate cortico-striatal synaptic efficacy3. However, no direct evidence links dopamine, striatal activity and behavioural choice in humans. Here we show that, during instrumental learning, the magnitude of reward prediction error expressed in the striatum is modulated by the administration of drugs enhancing (3,4-dihydroxy-L-phenylalanine; L-DOPA) or reducing (haloperidol) dopaminergic function. Accordingly, subjects treated with L-DOPA have a greater propensity to choose the most rewarding action relative to subjects treated with haloperidol. Furthermore, incorporating the magnitude of the prediction errors into a standard action-value learning algorithm accurately reproduced subjects' behavioural choices under the different drug conditions. We conclude that dopamine-dependent modulation of striatal activity can account for how the human brain uses reward prediction errors to improve future decisions.
Dopamine is closely associated with reward-seeking behaviours, such as approach, consummation and addiction3-5. However, exactly how dopamine influences behavioural choice towards available rewards remains poorly understood. Substantial evidence from experiments on primates has led to the hypothesis that midbrain dopamine cells encode errors in reward prediction, the ‘teaching signal’ embodied in modern computational reinforcement learning theory6. Accumulating data indicate that different aspects of the dopamine signal incorporate information about the time, context, probability and magnitude of an expected reward7-9. Furthermore, dopamine terminal projections are able to modulate the efficacy of cortico-striatal synapses10,11, providing a mechanism for the adaptation of striatal activities during learning. Thus, dopamine-dependent plasticity could explain how striatal neurons learn to represent both upcoming reward and optimal behaviour12-16. However, no direct evidence is available that links dopamine, striatal plasticity and reward-seeking behaviour in humans. More specifically, although striatal activity has been closely associated with instrumental learning in humans17,18, there is no evidence that this activity is modulated by dopamine. Here we establish this link by using combined behavioural, pharmacological, computational and functional magnetic resonance imaging techniques.
We assessed the effects of haloperidol (an antagonist of dopamine receptors) and L-DOPA (a metabolic precursor of dopamine) on both brain activity and behavioural choice in groups of healthy subjects. Subjects performed an instrumental learning task involving monetary gains and losses, which required choosing between two novel visual stimuli displayed on a computer screen, so as to maximize payoffs (Fig. 1a). Each stimulus was associated with a certain probability of gain or loss: one pair of stimuli was associated with gains (£1 or nothing), a second pair was associated with loss (2£1 or nothing), and a third pair was associated with no financial outcomes. Thus, the first pair was designed to assess the effects of the drugs on the ability to learn the most rewarding choice. The second pair was a control condition for the specificity of drug effects, because it required subjects to learn from punishments (losses) instead of rewards (gains), with the same relative financial interests. The third pair was a neutral condition allowing further control, in which subjects could indifferently choose any of the two stimuli, because they involved no monetary gain or loss. The probabilities were reciprocally 0.8 and 0.2 in all three pairs of stimuli, which were randomly displayed in different trials within the same learning session. Subjects had to press a button to select the upper stimulus, or do nothing to select the lower stimulus, as they appeared on the display screen. This Go/NoGo mode of response offers the possibility of identifying brain areas related to motor execution of the choice, by contrasting Go and NoGo trials.
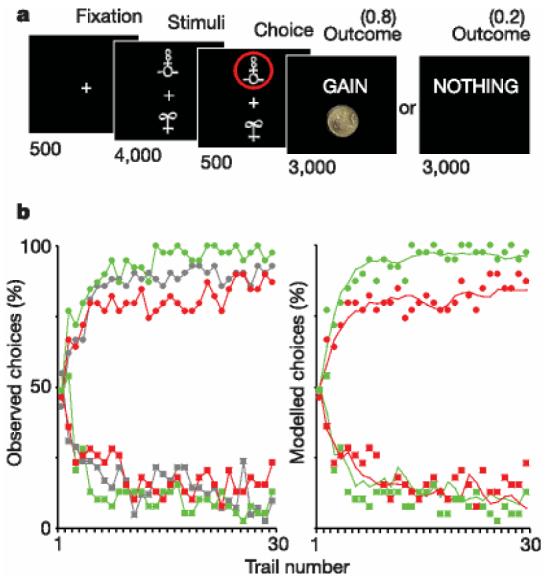
Experimental task and behavioural results. a, Experimental task. Subjects selected either the upper or lower of two abstract visual stimuli presented on a display screen, and subsequently observed the outcome. In this example, the chosen stimulus is associated with a probability of 0.8 of winning £1 and a probability of 0.2 of winning nothing. Durations of the successive screens are given in milliseconds. b, Behavioural results. Left: observed behavioural choices for initial placebo (grey), superimposed over the results from the subsequent drug groups: L-DOPA (green) and haloperidol (red). The learning curves depict, trial by trial, the proportion of subjects that chose the ‘correct’ stimulus (associated with a probability of 0.8 of winning £1) in the gain condition (circles, upper graph), and the ‘incorrect’ stimulus (associated with a probability of 0.8 of losing £1) in the loss condition (squares, lower graph). Right: modelled behavioural choices for L-DOPA (green) and haloperidol (red) groups. The learning curves represent the probabilities predicted by the computational model. Circles and squares representing observed choices have been left for the purpose of comparison. All parameters of the model were the same for the different drug conditions, except the reinforcement magnitude R, which was estimated from striatal BOLD response.
We first investigated the performance of a placebo-treated group, which showed that subjects learn within a 30-trial session to select the high-probability gain and avoid the high-probability loss. The overall performance was similar across the gain and loss trials, but with significantly lower inter-trial consistency and longer response time for the loss condition (Supplementary Table 1). This result indicates the possible existence of physiological differences between selecting actions to achieve rewards and selecting actions to avoid losses, possibly corresponding to additional processes being recruited during the avoidance condition. In the subsequent pharmacological study, L-DOPA-treated subjects won more money than haloperidol-treated subjects (£66.7 ± 1.00 versus £61.0 ± 2.10 (errors indicate s.e.m.), P , 0.05), but did not lose less money (£26.7 ± 1.50 versus £28.9 ± 1.40). Thus, relative to haloperidol, L-DOPA increased the frequency which subjects chose high-probability gain but not the frequency which they chose low-probability loss (Fig. 1b). In other words, enhancing central dopaminergic activity improved choice performance towards monetary gains but not avoidance of monetary losses. Neither drug significantly influenced response times, percentages of Go responses or subjective ratings of mood, feelings and sensations (Supplementary Table 2 and Supplementary Fig. 1).
For the analysis of brain activity, we first examined the representation of outcome prediction errors across all groups (placebo, L-DOPA and haloperidol). Corresponding brain regions were identified in a linear regression analysis, conducted across all trials, sessions and subjects, with the prediction errors generated from a standard action-value learning model. The parameters were adjusted to maximize the likelihood of the subjects' choices under the model. For each trial the model calculated choice probabilities according to action values. After each trial the value of the chosen action was updated in proportion to the prediction error, defined as the difference between expected value and actual outcome.
Statistical parametric maps (SPMs) revealed large clusters that were positively correlated with reward prediction error, all located in the striatum: predominantly the bilateral ventral striatum and left posterior putamen (Fig. 2a). This appetitive prediction error was observed across both gain and loss conditions, indicating that the striatum might represent successfully avoided outcomes as relative rewards. In addition, we observed a cluster showing significant negative correlation with an appetitive prediction error during the loss (but not gain) trials in the right anterior insula. This corresponds to an aversive prediction error, indicating that the loss condition might engage opponent appetitive and aversive processes, an idea in keeping with an experimental psychological literature on the dual excitatory and inhibitory mechanisms involved in signalled avoidance learning19.
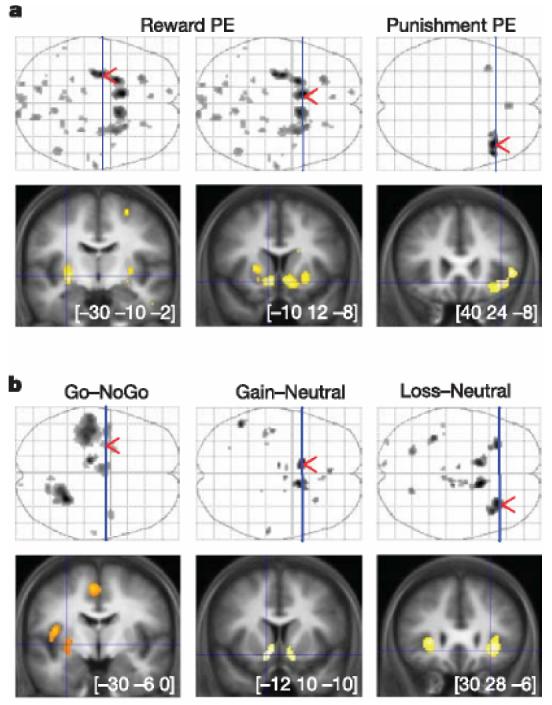
I Statistical parametric maps of prediction error and stimulus-related activity. Coronal slices (bottom) were taken at local maxima of interest indicated by red arrows on the axial projection planes (top). Areas shown in grey on axial planes and in orange or yellow on coronal slices showed significant effect after family-wise error correction for multiple comparisons (P , 0.05). a, Brain activity correlated with prediction errors derived from the computational model. Reward prediction errors (positive correlation) were found by conjunction of gain and loss conditions (left panels), whereas punishment prediction errors (negative correlation) were found in the loss condition alone (right panel). From left to right, MNI (Montreal Neurological Institute) coordinates are given for the maxima found in the left posterior putamen, left ventral striatum and right anterior insula. b, Statistical parametric maps resulting from main contrasts between stimuli conditions. Go and NoGo refer to stimuli position requiring, or not requiring, a button press to get the optimal outcome. Gain, neutral and loss correspond to the different pairs of stimuli. As above, the maxima shown are located in the left posterior putamen, left ventral striatum and right anterior insula, from left to right.
To characterize further the brain activity involved in behavioural choice, we next examined the main contrasts between trial types at the time of stimuli display (Fig. 2b). Bilateral ventral striatum was significantly activated in the contrast between gain and neutral stimuli, and also in the contrast between loss and neutral stimuli. This activity is consistent with a learned value reflecting the distinction between stimuli predicting gains or losses on the one hand, and those predicting mere neutral outcomes on the other. Again, the similarity of the signal across both gain and loss trials might indicate a comparable appetitive representation of stimuli predicting reward and punishment avoidance. The left posterior putamen was significantly activated when the optimal stimulus was on the top of the screen rather than the bottom. This indicates that this region might be involved specifically when the optimal choice requires a Go (button press) and not a NoGo response. The left lateralization of posterior putamen activity is consistent with the fact that the right hand was employed for pressing the button. These findings are in line with a body of literature implicating the anterior ventral striatum in reward prediction20,21 and the posterior putamen in movement execution22,23. The distinct functional roles that we ascribe to these striatal regions are also supported by their principal afferents24,25: amygdala, orbital and medial prefrontal cortex for the ventral striatum versus somatosensory, motor and premotor cortex for the posterior putamen. The bilateral anterior insula was activated in the contrast between loss and neutral pairs alone, again providing support for the existence of an opponent aversive representation of stimulus value during avoidance learning. This same region of anterior insula has been shown to encode aversive cue-related prediction errors during pavlovian learning of physical punishment26.
Last, we explored the effects of drugs (L-DOPA and haloperidol) on the representation of outcome prediction errors. We averaged the blood-oxygen-level-dependent (BOLD) responses over clusters reflecting prediction errors (derived from the above analysis), separately for the different drugs and outcomes (Fig. 3). Note that in striatal clusters the average amplitude of the negative BOLD response was about fourfold that of positive BOLD response, which was consistent with the expression of appetitive prediction error (converging towards +0.2 and −0.8). The right anterior insula showed the opposite pattern (during the loss trials), which was consistent with the expression of an aversive prediction error (converging towards +0.8 and −0.2). Comparing between drugs, there was a significant difference (P < 0.05) in the gain condition alone, with positive and negative BOLD responses being enhanced under L-DOPA in comparison with haloperidol. There was no significant effect in the loss condition, either in the striatum or in the anterior insula, in accord with the absence of drug effects on behavioural choices.
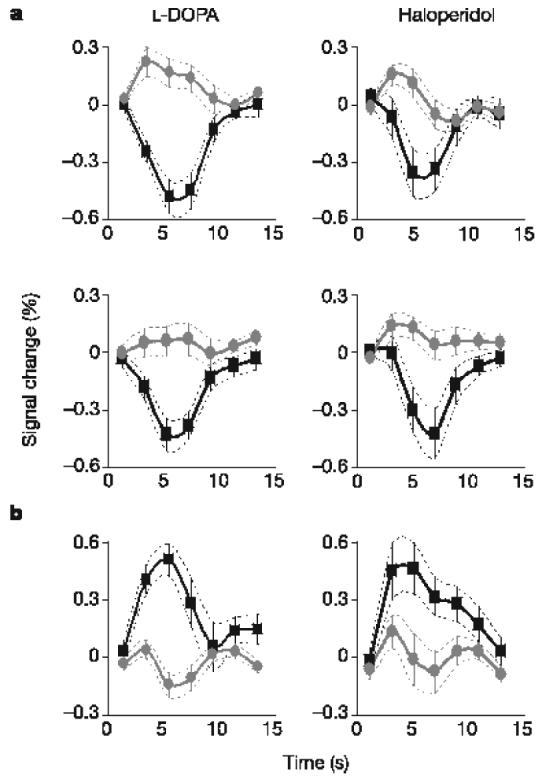
Time course of brain responses reflecting prediction errors. Time courses were averaged across trials throughout the entire learning sessions. Error bars are inter-subject s.e.m. a, Overlaid positive (grey circles) and negative (black squares) reward prediction errors in the striatum for both L-DOPA-treated and haloperidol-treated groups, and in both gain and loss trials. b, Overlaid positive (black squares) and negative (grey circles) punishment prediction errors in the right anterior insula, during the loss trials.
The asymmetry of drug effects between gain and loss conditions supports the hypothesis that striatal dopamine has a specific involvement in reward learning, providing new insight into the debate over its relative reward selectivity27 given evidence implicating dopamine involvement in salient and aversive behaviours28. In some paradigms, such as in the aversive component of various cognitive procedural learning tasks, dopamine depletion improves performance13. In others, however, such as conditioned avoidance response learning, dopamine blockade impairs performance29, probably as a result of interference with appetitive processes underlying the opponent ‘safety state’ of the avoided outcome. Although our data support the expression of distinct appetitive and aversive prediction errors during avoidance learning, the fact that neither of these opponent signals was affected by the dopamine-modulating drugs leaves it still unclear precisely what function dopamine has in aversive instrumental learning. This uncertainty is confounded to some extent by the fact that we do not know unequivocally how the drugs affect the different components of dopaminergic function, for example with regard to tonic versus phasic firing, or D1 versus D2 receptors. Thus, although we can assert that dopamine has a selective effect on gain-related striatal prediction errors, we have to be cautious about inferring the precise mechanism at a cellular level.
We then investigated whether there was any relationship between dopamine-modulated striatal activity and behaviour, during the gain condition. We first estimated the effective monetary reward value from the amplitude of the striatal BOLD responses, for the drug conditions in comparison with the placebo group. By taking the difference between positive and negative BOLD responses as equivalent to £1.00 for the placebo group, we estimated an effective reward value of £1.29 ± 0.07 under L-DOPA and £0.71 ± 0.12 under haloperidol. These values were within the 95% confidence interval of those provided by the maximum-likelihood estimate of the observed choices under our computational model (see Supplementary Fig. 2). In other words, when we incorporated the reward magnitudes estimated from striatal BOLD responses into the computational model, it accurately and specifically reproduced the effects of the drugs on behavioural choices (Fig. 1b).
Our results support a key functional link between dopamine, striatal activity and reward-seeking behaviour in humans. We have shown first that dopamine-related drugs modulate reward prediction errors expressed in the striatum, and second that the magnitude of this modulation is sufficient for a standard action-value learning model to explain the effects of drugs on behavioural choices. These findings suggest that humans use dopamine-dependent prediction errors to guide their decisions, and, more specifically, that dopamine modulates the apparent value of rewards as represented in the striatum. Furthermore, the findings might provide insight into models of clinical disorders in which dopamine is implicated, and for which L-DOPA and haloperidol are used as therapeutic agents, such as Parkinson's disease and schizophrenia. For example, it offers a potential mechanism for the development of compulsive behaviours (such as overeating, hypersexuality and pathological gambling) induced by dopamine replacement therapy in patients with Parkinson's disease30.
METHODS
For a detailed and referenced description of the experimental and analytical techniques, see Supplementary Methods and Results.
Experimental procedure
The study was approved by the local ethics committee. In all, 39 healthy subjects were scanned (19–37 years old; 23 males), including a single-blind initial study of 13 subjects treated with a placebo only (lactose) and a double-blind test study of 26 subjects, half treated with Haldol (haloperidol, 1mg) and half with Madopar (L-DOPA, 100mg, plus benserazide, 25mg). After a short practice, subjects had to perform three sessions of the same instrumental learning task, each proposing three new pairs of abstract stimuli. Each of the pairs of stimuli (gain, loss and neutral) was associated with pairs of outcomes (‘gain’ £1/nil, ‘loss’ £1/nil, ‘look’ £1/nil), the two stimuli corresponding to reciprocal probabilities (0.8/0.2 and 0.2/0.8). On each trial, one pair was randomly presented and the two stimuli were displayed on the screen, above and below a central fixation cross, their relative position being counterbalanced across trials. The subject was required to choose the upper stimulus by pressing a button (Go response), or the lower stimulus by doing nothing (NoGo response). The choice was then circled in red and the outcome was displayed on the screen. To win money the subjects had to learn, by trial and error, the stimulus–outcome associations. They were told that their winnings would be their remuneration for participation, but they all left with the same fixed amount. To assess for side effects of the drug, they were finally asked to rate their subjective feelings, using visual analogue scales. Behavioural performance was compared directly between the L-DOPA and haloperidol groups, with two-sample t-tests.
Computational model
A standard algorithm of action-value learning was then fitted to the observed behaviour. For each pair of stimuli A and B, the model estimates the expected values of choosing A (Qa) and choosing B (Qb), on the basis of individual sequences of choices and outcomes. The expected values were set at zero before learning, and after every trial t> 0 the value of the chosen stimulus (sayA) was updated according to the rule Qa(t + 1) = Qa(t) + a*d(t). The outcome prediction error, d(t), is the difference between the actual and the expected outcome, d(t) = R(t) − Qa(t), the reinforcement R(t) being either +£1, £0 or −£1. Given the expected values, the probability (or likelihood) of the observed choice was estimated with the softmax rule: Pa(t) = exp(Qa(t)=$)={exp[Qa(t)=$] + exp[Qb(t)=$]}: The constants a (learning rate) and $ (temperature) were adjusted to maximize the likelihood of the actual choices under the model, across all groups of subjects. Outcome prediction errors estimated by the model were then used as a statistical regressor in the imaging data.
Image acquisition and analysis
T*2 -weighted echo planar images (EPIs) were acquired with BOLD contrast on a 3.0-T Siemens Allegra magnetic resonance scanner, using a tilted plane acquisition sequence covering the whole brain. T1-weighted structural images were normalized and averaged across subjects to allow group-level anatomical localization. EPIs were analysed in an event-related manner, with the statistical parametric mapping software SPM5. Preprocessing consisted of spatial realignment, normalization to a standard EPI template, and spatial smoothing with a 6-mm gaussian kernel. To correct for motion artefacts, subject-specific realignment parameters were modelled as covariates of no interest. Onsets of stimuli and outcomes were modelled as separate delta functions and convolved with a canonical haemodynamic response function. Prediction errors generated by the computational model were used as parametric modulation of additional regressors modelled at outcome onsets. Linear contrasts of regression coefficients were computed at the individual subject level and then taken to group-level t-tests. All group-level SPMs are reported with a threshold of P, 0.05 after family-wise error correction for the entire brain. For large clusters (more than 64 voxels) showing statistical covariation with the theoretical prediction error, the response time courses were estimated, with the use of a flexible basis set of finite impulse responses (FIRs), separated from the next by one scan (1.95 s). The area between positive and negative FIRs, over 3–9s after outcome, were used to estimate effective reward values under the drug conditions.
References
Full text links
Read article at publisher's site: https://doi.org/10.1038/nature05051
Read article for free, from open access legal sources, via Unpaywall:
https://pure.mpg.de/pubman/item/item_2614402_3/component/file_2621853/RD_Dopamine_2006.pdf
Citations & impact
Impact metrics
Article citations
Higher Striatal Dopamine is Related With Lower Physical Performance Fatigability in Community-Dwelling Older Adults.
J Gerontol A Biol Sci Med Sci, 79(11):glae209, 01 Nov 2024
Cited by: 0 articles | PMID: 39208421
Roles and interplay of reinforcement-based and error-based processes during reaching and gait in neurotypical adults and individuals with Parkinson's disease.
PLoS Comput Biol, 20(10):e1012474, 14 Oct 2024
Cited by: 0 articles | PMID: 39401183 | PMCID: PMC11472932
Pre-choice midbrain fluctuations affect self-control in food choice: A functional magnetic resonance imaging (fMRI) study.
Cogn Affect Behav Neurosci, 08 Oct 2024
Cited by: 0 articles | PMID: 39379768
Dopamine has no direct causal role in the formation of treatment expectations and placebo analgesia in humans.
PLoS Biol, 22(9):e3002772, 24 Sep 2024
Cited by: 1 article | PMID: 39316644 | PMCID: PMC11421806
Revisiting the role of computational neuroimaging in the era of integrative neuroscience.
Neuropsychopharmacology, 50(1):103-113, 06 Sep 2024
Cited by: 0 articles | PMID: 39242921 | PMCID: PMC11525590
Review Free full text in Europe PMC
Go to all (834) article citations
Data
Data behind the article
This data has been text mined from the article, or deposited into data resources.
BioStudies: supplemental material and supporting data
Similar Articles
To arrive at the top five similar articles we use a word-weighted algorithm to compare words from the Title and Abstract of each citation.
L-DOPA reduces model-free control of behavior by attenuating the transfer of value to action.
Neuroimage, 186:113-125, 28 Oct 2018
Cited by: 32 articles | PMID: 30381245
Dopamine Modulates Adaptive Prediction Error Coding in the Human Midbrain and Striatum.
J Neurosci, 37(7):1708-1720, 01 Feb 2017
Cited by: 41 articles | PMID: 28202786 | PMCID: PMC5320604
Pharmacological modulation of subliminal learning in Parkinson's and Tourette's syndromes.
Proc Natl Acad Sci U S A, 106(45):19179-19184, 22 Oct 2009
Cited by: 70 articles | PMID: 19850878 | PMCID: PMC2776465
A computational substrate for incentive salience.
Trends Neurosci, 26(8):423-428, 01 Aug 2003
Cited by: 216 articles | PMID: 12900173
Review
Funding
Funders who supported this work.
Wellcome Trust (1)
The role of emotion in decision making
Prof Raymond Dolan, University College London
Grant ID: 078865