Abstract
Free full text

Cingulate activation increases dynamically with response speed under stimulus unpredictability
Abstract
FMRI studies of cognition require repeated and consistent engagement of the cognitive process under investigation. Activation is generally averaged across trials that are assumed to tax a specific mental operation or state, while intra-individual variability in performance between trials is usually considered error variance. A more recent analysis approach postulates that these fluctuations can reflect variation in the very process taxed by the particular trial type. In the present study, participants responded to targets presented randomly in one of four peripheral locations. By employing a function of reaction time (RT) of individual trials as a linear regressor, brain regions were identified whose activation varied with RT on a trial-by-trial basis. Whole-brain analysis revealed that the anterior cingulate, posterior cingulate and left angular/superior temporal gyrus were more active in trials with faster RT, but only when the target location was unpredictable. No such association was seen in trials where the target location was predicted by a central cue. These results suggest a role for the cingulate and angular gyrus in the dynamic regulation of attention to unpredictable events. This is in accordance with the function of a default network that is active in the absence of top-down focused attention and is thought to continuously provide resources for broad and spontaneous information gathering. Exploiting inter-trial performance variability may be particularly suitable for capturing such spontaneous and elusive phenomena as stimulus-driven processes of attention.
Introduction
Functional Magnetic Resonance Imaging (fMRI) studies of cognition (see Aguirre, 1996 for a review of designs) require repeated engagement of the cognitive process under investigation. Activation is typically averaged across trials that are assumed to evoke a specific (set of) mental operation(s) upon each presentation. The approach relies heavily on the consistent engagement of this operation across trials. Both inter- and intra-individual variability in performance between trials of the same type are usually considered error variance. If this variance is large, it results in a loss of sensitivity and reduces the likelihood that neural correlates of the process of interest can be detected.
However, performance variability has also been exploited to obtain deeper insight into associations between cognitive and brain functions. For this approach, it is assumed that variation in performance in part reflects variation in the cognitive process under investigation. Thus, variability in a task-relevant mental operation is used as a probe to identify brain structures engaged by this process. On an inter-individual level, an approach is to correlate subjects’ average performance with activation in predefined regions, or to perform a median split on individual performance values and test for group differences in brain activation or vice versa (e.g. Lawrence and others, 2003; Hester and others, 2004). In contrast, an intra-individual approach postulates that trial-by-trial fluctuations in performance co-vary with fluctuations in the very cognitive process(es) taxed by this trial type. Thus, a measure of individual trial performance is employed as a regressor, a common example being discrete performance events such as errors (e.g. Carter and others, 1998; Hester and others, 2004). Furthermore, recent studies successfully employed trial-by-trial reaction time (RT) as a regressor to verify regional engagement by external stimulus processing demands (Gilbert et al. 2006) or by specific functions of language processing and generation (Binder and others, 2005; Desai and others, 2006). Another recent study related trial-by-trial performance accuracy to the BOLD time course to demonstrate that cue-induced regional activity dynamically predicted target detection (Sapir et al. 2005).
The differing approaches of averaging activation across trials or of exploiting inter-trial variability may be optimal for capturing different phenomena. If some cognitive processes can be evoked more consistently than others, inter-trial fluctuations in these processes may tend to be of different magnitudes. In the present study, it was hypothesized that a simple operation initiated by a clear instructive cue, such as attending to a specific spatial location in anticipation of a stimulus, would be evoked more reliably than a more spontaneous phenomenon such as shifting attention to an unpredicted stimulus. Thus, exploiting the inter-trial variability could be a more sensitive approach for detecting those brain regions that regulate more elusive cognitive phenomena that unfold in the absence of clear top-down control signals and that are involved in dynamic and spontaneous behavioral adjustments.
The inter-trial variability of RT was used as the basis for analyzing data from a stimulus detection paradigm designed to differentiate neural substrates of top-down and bottom-up processes of visuospatial selective attention. Top-down or endogenous attentional control is intentional and directed by knowledge, expectation and current goals. Bottom-up or exogenous attention, in contrast, is stimulus-driven, i.e. attention is spontaneously oriented towards an oncoming stimulus (Desimone and Duncan, 1995; Egeth and Yantis, 1997). In the current paradigm, by manipulating the predictability of the location of a target stimulus, attention could be either allocated endogenously to this location in advance of target onset or had to be oriented rapidly upon target onset. The purpose of the present study was to identify those brain regions whose activation tended to coincide with fast RT, either under conditions of stimulus predictability that allowed top-down regulation of attention, or stimulus unpredictability where attention had to be shifted spontaneously.
Methods
Subjects
Subjects were 22 healthy, right-handed adults (13 females, 27.5±7.55 years of age), who were recruited from the general population through advertising and referrals and gave informed consent for a protocol approved by the NIDA-IRP Institutional Review Board. All participants were non-smokers and had been asked to consume not more than half a cup of coffee in the 12 hours preceding MR scans and no alcohol in the preceding 24 hours. Subjects were screened for major medical illnesses, claustrophobia, history of neurological or psychiatric disorders and drug abuse, pregnancy and appropriateness for MRI.
Procedure
The protocol required three separate visits. During the first visit, participants gave written informed consent and were trained on two cognitive tasks, initially on a bench computer and then in a mock scanner that mimicked all properties of the MRI scanner. Training in the mock scanner was equal in length to when the tasks were performed in the real scanner. Sessions 2 and 3 were identical and served as time controls for a pharmacological experiment reported elsewhere. Prior to both MR scans, participants were tested for recent drug use (TRIAGE®) and for alcohol intake or smoking via breath analysis; a pregnancy test was given to female participants. Subjects then received a 10 min reminder task training on a bench computer, during which their eye-position was recorded. MR scans started with a brief central executive task (data not reported). Eight blocks of the Spatial Attentional Resource Allocation Task (SARAT) were then performed, separated by one-minute rest periods, followed by anatomical scans. A previous report analyzed the same dataset based on trial averages (Hahn and others, 2006).
Paradigm
The SARAT required participants to detect a target signal (500 ms) that could occur at any of four peripheral locations marked by empty circles (Figure 1). Subjects were asked to keep their eyes fixated on a central circle containing a fixation cross during performance of the task. Target locations were positioned at 10–12.5° of visual angle. Targets consisted of a circle filling with a checkerboard of grey and white squares of 3×3 pixels each. Two different target intensities (high: 80% grey; low: 20% grey) were tested. Upon presentation of a target, subjects were instructed to press a button with their right index finger as quickly as possible.
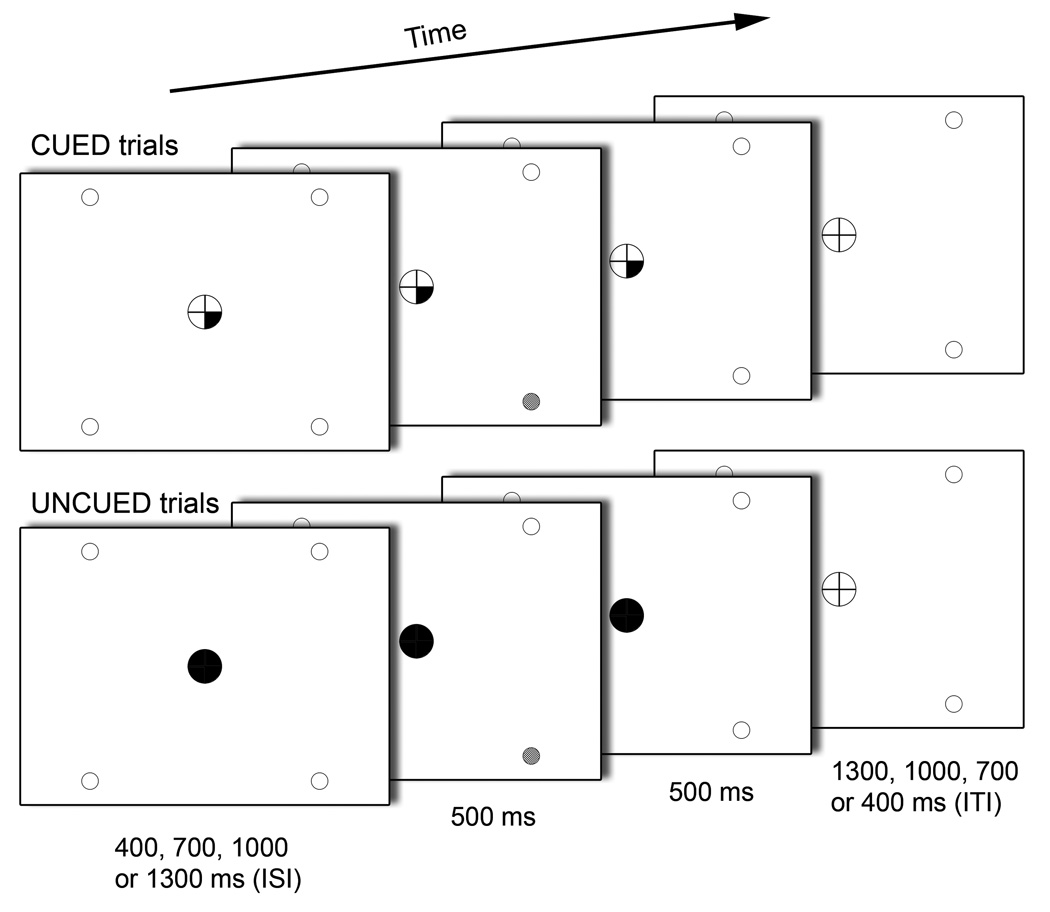
Components of a CUED and an UNCUED target trial in the Spatial Attentional Resource Allocation Task (SARAT). Onset of a central cue preceded target onset by a variable SOA of 400, 700, 1000 or 1300 ms. The target was presented for 500 ms in the continuing presence of the cue, which remained on display until 500 ms after target offset. Only screen background was then presented for an intertrial interval that varied in length such that total trial duration was always 2700 ms.
Cues appeared in the central circle 400, 700, 1000 or 1300 ms (variable stimulus-onset-asynchrony - SOA) prior to target onset and remained on display until 1000 ms after target onset, i.e. until 500 ms after target termination. Cues consisted of quarters of the fixation circle turning black with their location indicating the probable location of the peripheral target. One, two, three or four quarters could turn black simultaneously. Thus, predictability of the target location varied across trials with one cued location providing the most precise advance information and four cued locations leaving the target location completely unpredictable. The cue provided invalid information in 20% of trials with one, two or three cued locations. In some trials, the cue was not followed by a target. Valid trials with high-intensity targets, valid trials with low-intensity targets and no-target trials were presented with equal frequency. All trial types were randomized within runs. To create sufficient temporal jitter for event-related analysis, no-event trials, where no cues or targets were presented, were randomly interspersed. Trial duration was 2.7 s (Figure 1). Each of the eight runs consisted of 81 trials; 32 valid target trials, 16 no-target trials, 6 invalid target trials and 27 no-event trials, resulting in a run duration of 3:39 min.
For the current study, only valid target trials with one cued location (referred to as “CUED trials”) or four cued locations (referred to as “UNCUED trials”) were analyzed. Only those trials where a subject response occurred were analyzed. Thus, the study focused on two conditions: (a) a target is detected at a location to which attention was allocated endogenously prior to target onset, and (b) the target location was unknown until target onset, at which point attention is drawn to this location by the physical appearance of the target stimulus.
Eye-tracking
Eye-position on the screen was recorded during performance of the 10 min refresher training that preceded each MR scan, using a remote eye-tracking system consisting of a video camera and infrared light source (IVIEW, Sensomotoric Instruments Inc, Needham, Massachusetts). The purpose was to verify that the task was not performed by re-focusing the gaze to where the target was expected. This could have resulted in eye-movement related brain activation that differed systematically between CUED and UNCUED trials, thus potentially confounding results. Eye-tracking was not performed in eight subjects due to equipment unavailability. As described previously (Hahn and others, 2006), participants for whom eye-position was recorded spent 98.2±2.8% of the time that eyes were directed at the screen fixating no further than 3° from its center. No participant displayed any indication that fixations outside this radius were systematically oriented towards target locations. Furthermore, the absolute distance of subjects’ gaze position from the center of the screen did not differ between CUED and UNCUED trials, neither for the vertical nor horizontal direction [|t|(12)<1 for each direction].
Magnetic resonance imaging
Scanning was performed on a 3 Tesla Siemens Allegra scanner (Erlangen, Germany). Whole brain functional EPI images were acquired for measurement of T2*-weighted BOLD effects (4mm sagital slices, 64×64 matrix, FOV=22×22cm, TR=2.7s, TE=27ms, FA=85°). In each scanning session, a whole-brain sagital T1-weighted structural image (MPRAGE) was acquired for anatomical reference (1mm3 isotropic voxels, TR=2.5s, TE=4.38 ms, FA=8°).
Analysis of fMRI data
Data were processed using the AFNI software package version 2.55j (Cox, 1996). Motion correction was performed by volume registering each 3D volume to a base volume. The time series was then analyzed by voxel-wise multiple regression, where the main regressor of interest was related to the RT of individual trials. To ensure that RT variability did not reflect shifts in response speed with time on task due to practice or fatigue, RT averaged across all valid target trials was compared between the eight runs by paired t-tests, with Bonferroni-adjustments made for 28 comparisons. RT was significantly faster in run1 than in run 2, 4, 5 and 7 [t(21)>3.9, P<0.05 for each comparison], while RT in runs 2–8 did not differ significantly from each other. Thus, the regression analyses were based only on trials from runs 2–8, throughout which average RT appeared stable over time. Regressors were expressed as a simple delta function convolved with a model hemodynamic response function and its temporal derivative.
The RT regressor was a function of individual trial RT, i.e. its amplitude was scaled according to these trial-by-trial values. For this regressor, the RT of individual trials i was expressed as
Additional regressors corresponded to 18 different trial types (1/2/3/4 validly cued locations * high/low/no target + 1/2/3 invalidly cued locations * high/low target intensity). The beta-weights from these regressors reflect signal amplitude as in standard fMRI experiments, henceforward termed Betamean. Only data from valid target trials with one and four cued locations, i.e. from CUED and UNCUED trials, were further analyzed. All other regressors were included only to account for related signal variation. Further nuisance regressors corresponded to the six head motion parameters to help account for residual motion, and if applicable, to target trials in which no response was registered.
For the four trial types under investigation (CUED/UNCUED × high/low target intensity), voxel-wise Betaslope and Betamean values were normalized to baseline for each subject and each test session. The resulting maps were resampled to a higher (1µl) resolution, converted to standard stereotaxic space (Talairach and Tournoux, 1988) and spatially blurred using a Gaussian 4.2 mm FWHM isotropic kernel.
Functional regions of interest (ROIs) were derived by performing voxel-wise one-sample, random-effects t-tests against zero on Betaslope, separately for CUED and UNCUED trials averaged over target intensity. One-tailed t-tests were performed, testing only for voxels with a significantly positive Betaslope, i.e. an increase in activation with faster RT. Increases in activation with slower RT could simply reflect a longer period of neuronal activation. A range of previous studies employing simple sensory stimulation paradigms reported that the BOLD signal increased in amplitude with prolonged or repeated stimulation (e.g. Boynton and others, 1996; Dale and Buckner, 1997), even on a millisecond scale (Robson and others, 1998). Thus, in trials where more time is spent on signal processing or response preparation, voxels of underlying brain regions may display higher signal amplitude as a direct result of their longer engagement. Such effects were not of interest in the present investigation. Areas that increased in activation with slower responding were extensive and are listed in Supplementary Table S1. A voxel-wise threshold of p<0.005 was applied to the t-test activation maps which, combined with a cluster volume threshold of 515 µl, yielded an overall false positive p<0.05 as determined by Monte Carlo simulation. Betaslope and Betamean values were each averaged over voxels within each ROI for each subject, session, cue condition and target intensity. These average values were subjected to repeated measures ANOVA with session, cue condition and target intensity as within-subject factors, followed by t-tests where appropriate.
Results
Performance of the SARAT
Detailed behavioral analyses across all trial types can be found in Hahn et al. (2006). Briefly, RT decreased parametrically with increasing cue precision and was faster with high than low target intensity. Three-factor repeated measures ANOVA of the four trial types included in the present study (CUED and UNCUED, each with high or low target intensity) revealed main effects of cue condition [F(1,21)=67.4, P<0.001] and target intensity [F(1,21)=164.4, P<0.001] but no cue x target interaction, confirming that average RT was overall faster in CUED than UNCUED trials (430±81 vs. 457±83 ms) and faster with high than low intensity targets (421±80 vs. 466±85 ms). The main effect of SOA [F(3,63)=12.7, P<0.001] was due to shorter RT at the two intermediate SOA (Figure 2). Also the SOA x cue interaction was significant [F(3,63)=4.0, P<0.05]; the RT difference between CUED and UNCUED trials was particularly large with SOA 700 ms, where CUED RT was substantially lower than in all other conditions. Accordingly, the effect of SOA in 1-way ANOVA was more pronounced in CUED [F(3,63)=13.1, P<0.001] than in UNCUED trials [F(3,63)=4.77, P<0.01]. The fact that RT differed with SOA necessitated controls for the possibility that associations between RT and BOLD signal we1re secondary to regional responsivity to SOA.
fMRI
One-sample t-tests performed on the voxel-wise average Betaslope of CUED trials did not identify any region that activated with faster responding. In contrast, one-sample t-tests performed on voxel-wise Betaslope of UNCUED trials identified three regions whose activation was positively related to response speed: rostral anterior cingulate gyrus, posterior cingulate gyrus and left inferior parietal lobule in the area of the angular gyrus, possibly reaching into superior temporal gyrus (STG) (Table 1 and Figure 3). Anterior cingulate cortex (ACC) activation was located mostly in areas 24a, b, c and 32, as defined by Vogt and others (1995), with little spread into areas 24a’ and b’ and no overlap with areas 24c’ and 32’. Activation within the posterior cingulate cortex (PCC) comprised regions 23a and b, but not c, with spread also into areas 31 and 30.
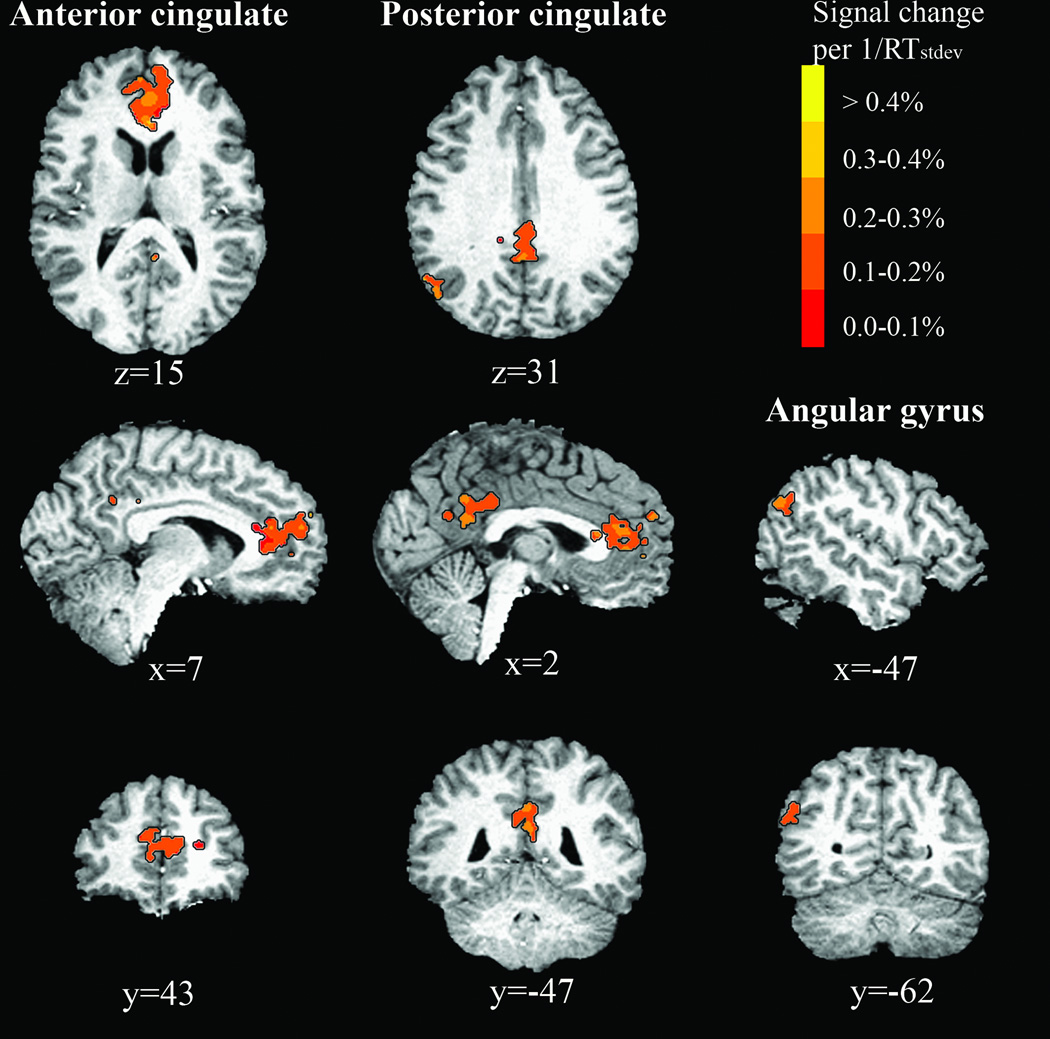
Brain regions whose activation in UNCUED trials increased with
Table 1
Brain regions identified by analysis of UNCUED trials
Brain Region | Side | Center of Mass (mm) X, Y, Z | Brodmann Area(s) | Volume (µl) |
---|---|---|---|---|
Anterior cingulate gyrus | B | 1.8, 41.2, 13.2 | 24, 32 | 5427 |
Posterior cingulate gyrus | B | −0.2, −43.6, 29.3 | 23,31,30 | 2131 |
Angular gyrus/STG | L | −47.4, −64.5, 31.1 | 39 | 541 |
Regions in Talairach space where the BOLD signal intensity increased linearly with
In all three regions, 3-factor ANOVA for repeated measures revealed a significant main effect of cue condition [F(1,21)>8.27, P<0.01 in each region]. One-sample t-tests against zero confirmed significantly positive Betaslope in UNCUED trials [t(21)>4.56, P<0.001 in each region], but not in CUED trials [t(21)<1.47, NS]. Figure 4 displays the association between
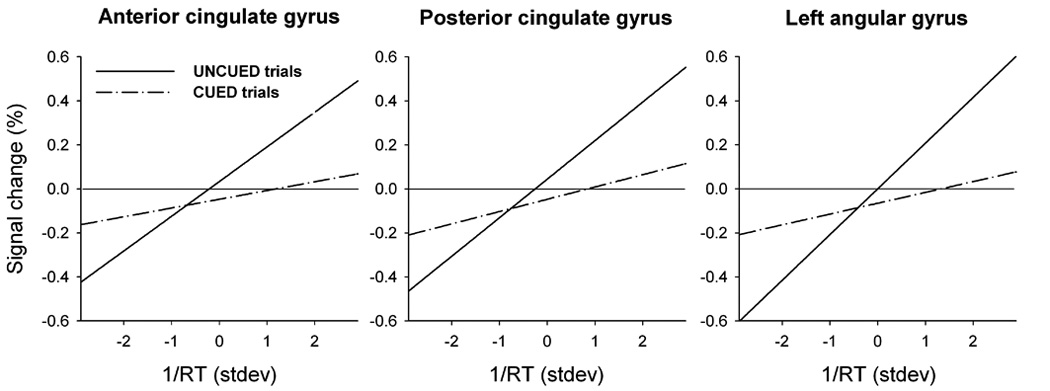
Activation in the anterior and posterior cingulate and in the left angular gyrus plotted against
The differential BOLD-RT association in UNCUED trials could not have reflected regional responsivity to SOA. First, SOA effects on RT were more pronounced in CUED trials, where the three ROIs did not display any such brain-behavior relationship. Second, these regions were not responsive to SOA; the same dataset had previously been analyzed for separate SOAs (see Hahn and others, 2006, for methods), and these activation values were averaged across voxels of each of the present ROIs. Two-factor ANOVA for repeated measures identified no effect of SOA [F(3,63)<1.5, NS, for each of the three regions] and no interaction of SOA with cue condition [F(3,63)<2.62, NS].
Next, the average activation Betamean was determined within each region for each cue condition, session and target intensity, and analyzed by three-factor repeated measures ANOVA. A significant main effect of cue condition was identified in the ACC and PCC [F(1,21)>5.0, P<0.05], but not in the left angular gyrus [F(1,21)=1.79, n.s.]. Figure 5 shows that in both structures this was due to negative mean activation values in CUED trials and positive values in UNCUED trials. However, in one-sample t-tests, average activation did not differ from zero in either CUED or UNCUED trials [t(21)<1 for both trial types and regions]. The effects of cue did not interact with session or with target intensity in any region.
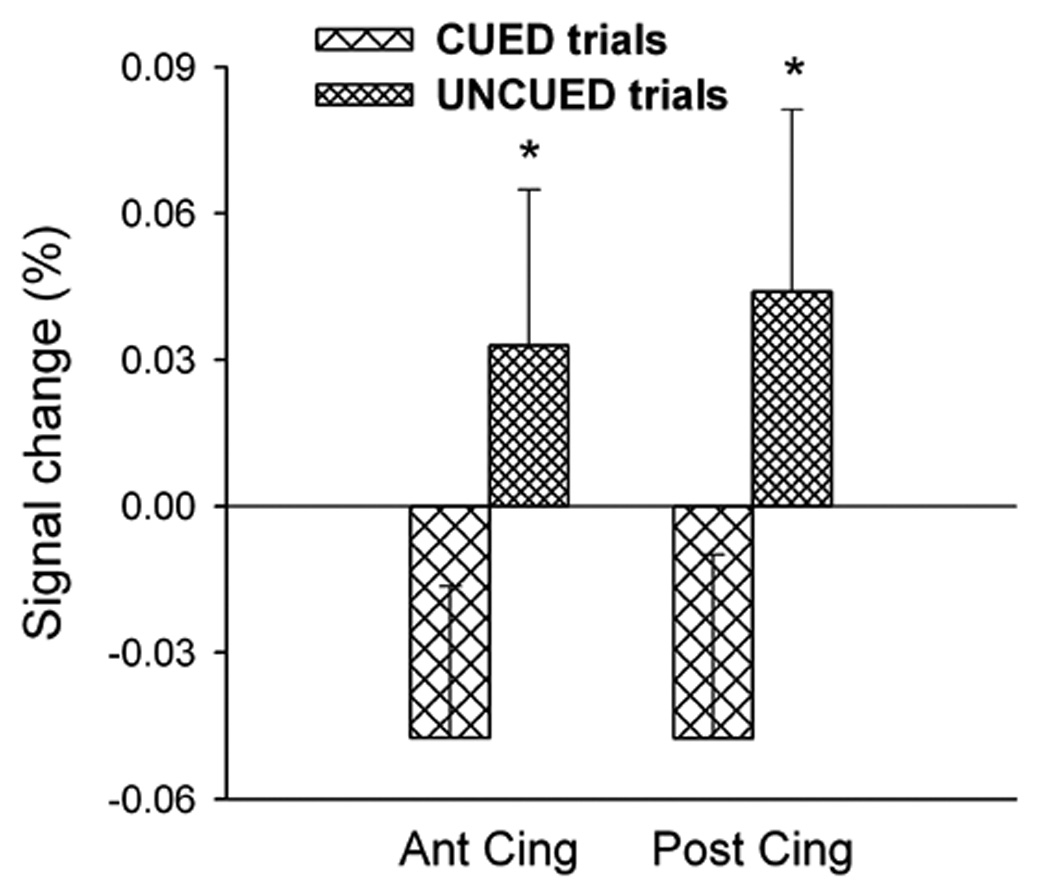
Mean percentage of signal change in the anterior and posterior cingulate gyrus across trials. These values differed (*P<0.05) between CUED and UNCUED trials, but no condition displayed a significant overall activation or deactivation relative to baseline.
To test whether each region’s average activation was associated with average RT on an inter-individual basis, Pearson correlations were determined between Betamean and average RT, separately in CUED and UNCUED trials. There were no significant correlations [P>0.2 in each case].
Discussion
The present study identified three brain structures whose signal amplitude was related to performance of a visuospatial stimulus detection task on a trial-by-trial basis. Activation in the ACC, PCC and left angular gyrus/STG was positively associated with response speed, and this association occurred only in the condition where stimuli were detected at unpredictable locations. When the target location was predicted by a central cue, no region was found to be more active in trials with faster RT. Thus, while RT variability in UNCUED trials in part reflected fluctuation rooted in the function of specific brain areas, variability of RT in CUED trials, despite being of equal magnitude, may have constituted variance not based on task-specific brain processing.
Although we hypothesized that co-variation between regional activation and trial-by-trial performance reflects fluctuation in an underlying cognitive function, the ACC is also known as an effector region that controls output to skeletomotor systems (Vogt and others, 1992). As such, activation that accompanies faster responding could also be a reflection of more efficient response initiation. In monkeys, one area of ACC and one of PCC were activated by voluntary hand movements (Shima and others, 1991). They were located in area 24c (mostly c’) of the anterior and 23c of the posterior cingulate sulcus, consistent with cingulate motor areas localized by retrograde labeling and intracortical microstimulation (Dum and Strick, 1991; Morecraft and Van Hoesen, 1992; Nimchinsky and others, 1996; Luppino and others, 1991; Wang and others, 2001). Notably, these cingulate sub-regions did not overlap with those identified in the present study. Similarly, in a human PET study, the area of ACC that displayed a rise in blood flow when subjects made manual responses (but not when responding verbally or by saccades) was located in posterior parts of the anterior cingulate sulcus (Paus and others, 1993), remote from the current regions. Thus, the current association between cingulate activation and RT most likely is not based on variation in a motor output but in a stimulus processing function.
Co-variation between RT and regional activation does not however answer the question of causality, i.e. if activation facilitated fast responding or if it constituted a response to fast RT, for example reflecting rapid performance evaluation. In ACC, rapid (250–300 ms) neuronal responding to performance feedback is a well-studied phenomenon (Ridderinkhof and others, 2004), but such responses are larger in amplitude for negative than positive feedback (Holroyd and Coles, 2002; Ullsperger and von Cramon, 2004). Thus, assuming that the brain is indeed able to differentially process responses with different RTs, one would expect increasing ACC activation with “unsuccessful” slow responding (the instruction was to respond as fast as possible) instead of the increase with faster responding according to which the current regions were identified. Another possibility is that faster responding created more post-response conflict or uncertainty, i.e. it may have unfolded without complete certainty of having detected a target. If this was the case, the association between RT and the BOLD signal would have been stronger in trials with low-intensity targets where such uncertainty must have been more pronounced. However, there was no difference in Betaslope between trials with high and low target intensity despite large behavioral differences. Overall, regional activation affecting performance appears to be the more likely alternative.
The regions may have fulfilled a preparatory function, mobilizing resources in response to the cue signaling unpredictability of the target location, or they may have constituted the actual resource that supported the spontaneous, rapid orienting towards the target stimulus. Adopting the latter interpretation, the selectivity of co-variation between regional activation and RT for the condition of stimulus unpredictability may suggest that exploiting inter-trial variability of performance is a particularly suitable approach to capture phenomena that unfold spontaneously in the absence of voluntary cognitive control, like stimulus-driven orienting of attention. Such processes can be expected to be more difficult to detect with analyses based on trial averages because they are less likely to be engaged in a consistent manner across trials. Indeed, within the regions identified with the inter-trial approach, the average activation across trials did not significantly differ from zero in either CUED or UNCUED trials and did not correlate with average RT on an inter-individual basis. Thus, by analyzing trial averages, an association of BOLD signal in these regions with UNCUED trial performance would not likely have been detected.
Results from the current analytical approach that evade clear interpretation are increases in regional activity with slower responding (Supplementary Table S1). As mentioned, such a pattern may simply reflect longer neuronal engagement in response-relevant cognitive processes in trials with longer RT. Sensory stimulation experiments showed that prolonging the stimulation duration or adding further stimuli within the time frame of the hemodynamic response to a prior stimulus resulted in fMRI responses that were not only longer but also reached a higher peak (e.g. Boynton and others, 1996; Dale and Buckner, 1997; Robson and others, 1998). Larger response amplitudes were seen even with adding only one 100-ms auditory stimulus after a 100-ms off-period (Robson and others, 1998). Although trial-by-trial fluctuations in the present study are based on an even shorter timescale, it is likely that in trials with slower RT, more time is spent on signal processing or response preparation, and it is possible that corresponding brain regions displayed higher signal amplitude as a direct result of their longer engagement. Areas that displayed this pattern indeed included regions involved in functions such as these. It is also possible that lower activation with faster responding reflected an increasing automaticity of responding. A shortfall of the trial-by-trial analysis approach is that increases in activation with longer RT are currently not interpretable.
The present results suggest a role of the cingulate and angular gyrus in the dynamic regulation of processing unpredictable, behaviorally relevant signals. For the ACC, this is in agreement with studies reporting activation when performance was not under clear top-down control and there was conflict regarding which stimulus aspect should control performance (Carter and others, 1998, 2000; Botvinick and others, 1999; McDonald and others, 2000; Kerns and others 2004). These studies supported the notion that the ACC responds to conflict, specifically to response competition, i.e. conflict between evoked motor action plans (Carter and others, 2000; Milham and others, 2001, 2003; Matsumoto and Tanaka, 2004). This was consistent with cingulate projections to motor areas discussed above, and with the proximity of regions identified by these studies to cingulate motor areas. Few studies reported ACC responsiveness to perceptual or semantic conflict, i.e. conflict in the input domain (Weissman and others, 2003; van Veen and Carter, 2005).
The current behavioral paradigm differed from those typically used to probe the ACC in that it did not evoke any conflict in the response domain. Responses were always made to the same type of stimulus by pressing the same response button with the right index finger, and stimuli were always of the same temporal predictability. Thus, CUED and UNCUED trials enabled the same degree of response preparation. However, only UNCUED trials evoked conflict in the input domain in the form of uncertainty regarding the stimulus location. ACC activation was associated with task performance in these but not in CUED trials that reduced this uncertainty by evoking a visuospatial processing bias in preparation for target detection. Thus, the current findings may extend theories of a late evaluative function of ACC in the absence of advance top-down selection for action, specifically in the input domain of information processing.
Further, there is evidence that some areas of ACC are responsive to the perceived likelihood of committing an error on a given trial (Brown and Braver 2005). In the current paradigm, the percentage of omission errors was slightly higher in UNCUED than CUED trials (11 vs. 14%, Hahn and others, 2006), but it is unlikely that participants were aware of this small difference in success rate, or even of having missed a target (almost 30% of trials were cue-only trials). A global description of ACC function may be a more general responsiveness to conditions where behavior is not under tight stimulus control and there is uncertainty, be it regarding stimulus processing, response selection or outcome.
The ACC’s well-known functional heterogeneity (Devinsky and others, 1995) beckons the suggestion that different sub-regions respond to different types of conflict or uncertainty. Typically, the ACC has been probed with cognitively demanding tasks that involve high levels of control. These studies report activation in dorsal areas of ACC, such as 24a’, b’, c’ and 32’ (Carter and others, 1998, 2000; Botvinick and others, 1999; MacDonald and others, 2000; Milham and others, 2001; Weissman and others, 2003; van Veen and Carter, 2005), termed its cognitive subdivision (Bush and others, 2000). ACC-responses in these, but not rostral areas, are frequently accompanied by activation in dorsolateral prefrontal cortex (Paus, 2001), where they appear to recruit control processes (Kerns and others, 2004; Ridderinkhof and others, 2004). It is striking that the current area of ACC associated with simple stimulus detection performance in the absence of top-down selection fell so clearly outside this area and into a rostral region that has been reported to even deactivate during cognitively demanding tasks (Bush and others, 2000). We suggest that functions of dorsal and rostral ACC are complementary in that dorsal ACC responds to conflict that demands increased top-down control by other regions, while rostral ACC exerts influence over performance when such control would not reduce uncertainty.
An association of rostral ACC activation with RT performance in simple stimulus detection tasks has been observed previously (Gilbert and others, 2006). Notably, ACC along with PCC activation occurred when task demands were restricted to remaining alert to external events, and was reduced in the presence of higher order cognitive engagement. Generally, rostral ACC displayed preference for simple task conditions involving minimal stimulus processing. The conclusion that rostral ACC plays a role in maintaining attention toward the external environment and all incoming visual information, specifically during low-demand tasks in the absence of internal processing requirements, appears compatible with its current association with unbiased visuospatial stimulus detection.
The association of PCC activation with RT performance likely reflects involvement in fast visuospatial orienting. The current activation largely overlapped with the PCC’s “visuospatial area”, which is involved in spatial orienting and monitoring of visual events, especially in the periphery (reviewed by Vogt and others, 1992; Gusnard and Raichle, 2001). Importantly, Vogt and others (1992) report PCC responses to previously unattended but salient peripheral stimuli, but not to attended foveal stimuli, indicating a role in stimulus-driven orienting of attention in space. Gusnard and Raichle (2001) suggested that the PCC provides resources for the rapid and automatic allocation of attention to previously unattended input, enabling the detection of unforeseen events that may be relevant for survival. With regard to the present results, this would be in agreement with PCC activation supporting fast responding to targets when their location was unpredictable, although, as mentioned, the question of causality remains open.
The left angular gyrus/STG activation may be located in the vicinity of an area termed temporoparietal junction (TPJ) shown to be activated by target detection, especially at unexpected locations (Corbetta and others, 2000), and suggested to form part of a ventral frontoparietal exogenous orienting system (Corbetta and Shulman, 2002). Thus, activation with faster responding selectively under conditions of target unpredictability may be explained by a role in stimulus-driven orienting of attention, for this region, as well. In the above-cited studies, TPJ activation occurred predominantly in the right hemisphere, whereas the current activation was left lateralized, consistent with Hahn and others (2006). This report, based on the same dataset as the current study, also demonstrated that activation in or near TPJ was dependent on the presence of a target, suggesting it reflected target-induced activity in accordance with stimulus-driven attentional processes.
One commonality between all three anatomical regions is their overlap with areas that consistently displayed higher activation during rest than during various goal-directed behavioral activities (Shulman and others, 1997; Mazoyer and others, 2001). These areas form part of a network that displayed elevated blood flow and oxygen metabolism under resting conditions and that has been suggested to maintain an organized baseline default mode of brain function (Raichle and others, 2001). Thus, these regions display sustained and inter-correlated (Greicius and others, 2003) activity at rest that is attenuated by focused attention and that may reflect, among other functions, the continuous provision of resources for spontaneous, broad and exogenously driven information gathering (Gusnard and Raichle, 2001). The present association with performance exclusively in the absence of top-down focused attention supports involvement in regulating attentional resource allocation when the individual is not engaged in a specific endogenous task. UNCUED trials with many possible target locations appear to have created a condition that taps into the broad information gathering function that may be a default mode of these regions.
In summary, employing trial-by-trial performance variability as a regressor is a sensitive approach to identifying brain regions involved in spontaneous orienting in space. A brain structure with known responsiveness to uncertainty and to the absence of clear top-down control (ACC) was identified, as well as two regions with likely involvement in processes of stimulus-driven orienting (PCC, angular gyrus/STG). The present findings expand the view of the ACC’s conflict monitoring function to include responsiveness to uncertainty in the input domain of information processing and to demands on maintaining broad unbiased attention to the external environment.
Acknowledgments
This research was supported by the Intramural Research Program of the NIH, National Institute on Drug Abuse.
References
- Aguirre GK. Functional imaging in behavioral neurology and neuropsychology. In: Feinberg TE, Farah MJ, editors. Behavioral Neurology and Neuropsychology. 2nd edition. New York: McGraw-Hill; 2003. pp. 85–96. [Google Scholar]
- Binder JR, Medler DA, Desai R, Conant LL, Liebenthal E. Some neurophysiological constraints on models of word naming. Neuroimage. 2005;27:677–693. [Abstract] [Google Scholar]
- Botvinick M, Nystrom LE, Fissell K, Carter CS, Cohen JD. Conflict monitoring versus selection-for-action in anterior cingulate cortex. Nature. 1999;402:179–181. [Abstract] [Google Scholar]
- Box GEP, Cox DR. An Analysis of Transformations. Journal of the Royal Statistical Society (B) 1964;26:211–252. [Google Scholar]
- Boynton GM, Engel SA, Glover GH, Heeger DJ. Linear systems analysis of functional magnetic resonance imaging in human V1. J Neurosci. 1996;16:4207–4221. [Europe PMC free article] [Abstract] [Google Scholar]
- Brown JW, Braver TS. Learned predictions of error likelihood in the anterior cingulate cortex. Science. 2005;307:1118–1121. [Abstract] [Google Scholar]
- Bush G, Luu P, Posner MI. Cognitive and emotional influences in anterior cingulate cortex. Trends Cogn Sci. 2000;4:215–222. [Abstract] [Google Scholar]
- Carter CS, Braver TS, Barch DM, Botvinick MM, Noll D, Cohen JD. Anterior cingulate cortex, error detection, and the online monitoring of performance. Science. 1998;280:747–749. [Abstract] [Google Scholar]
- Carter CS, Macdonald AM, Botvinick M, Ross LL, Stenger VA, Noll D, Cohen JD. Parsing executive processes: strategic vs. evaluative functions of the anterior cingulate cortex. Proc Natl Acad Sci USA. 2000;97:1944–1948. [Europe PMC free article] [Abstract] [Google Scholar]
- Corbetta M, Kincade JM, Ollinger JM, McAvoy MP, Shulman GL. Voluntary orienting is dissociated from target detection in human posterior parietal cortex. Nat Neurosci. 2000;3:292–297. [Abstract] [Google Scholar]
- Corbetta M, Shulman GL. Control of goal-directed and stimulus-driven attention in the brain. Nat Rev Neurosci. 2002;3:201–215. [Abstract] [Google Scholar]
- Dale AM, Buckner RL. Selective averaging of rapidly presented individual trials using fMRI. Human Brain Mapping. 1997;5:329–340. [Abstract] [Google Scholar]
- Desai R, Conant LL, Waldron E, Binder JR. FMRI of past tense processing: the effects of phonological complexity and task difficulty. J Cogn Neurosci. 2006;18:278–297. [Europe PMC free article] [Abstract] [Google Scholar]
- Desimone R, Duncan J. Neural mechanisms of selective visual attention. Annu Rev Neurosci. 1995;18:193–222. [Abstract] [Google Scholar]
- Devinsky O, Morrell MJ, Vogt BA. Contributions of anterior cingulate cortex to behaviour. Brain. 1995;118:279–306. [Abstract] [Google Scholar]
- Dum RP, Strick PL. The origin of corticospinal projections from the premotor areas in the frontal lobe. J Neurosci. 1991;11:667–689. [Europe PMC free article] [Abstract] [Google Scholar]
- Egeth HE, Yantis S. Visual attention: control, representation, and time course. Annu Rev Psychol. 1997;48:269–297. [Abstract] [Google Scholar]
- Frith C, Dolan R. The role of the prefrontal cortex in higher cognitive functions. Brain Res Cogn Brain Res. 1996;5:175–181. [Abstract] [Google Scholar]
- Gilbert SJ, Simons JS, Frith CD, Burgess PW. Performance-related activity in medial rostral prefrontal cortex (area 10) during low-demand tasks. J Exp Psychol Hum Percept Perform. 2006;32:45–58. [Abstract] [Google Scholar]
- Greicius MD, Krasnow B, Reiss AL, Menon V. Functional connectivity in the resting brain: a network analysis of the default mode hypothesis. Proc Natl Acad Sci USA. 2003;100:253–258. [Europe PMC free article] [Abstract] [Google Scholar]
- Gusnard DA, Raichle ME. Searching for a baseline: functional imaging and the resting human brain. Nat Rev Neurosci. 2001;2:685–694. [Abstract] [Google Scholar]
- Hahn B, Ross TJ, Stein EA. Neuroanatomical dissociation between bottom-up and top-down processes of visuospatial selective attention. Neuroimage. 2006;32:842–853. [Europe PMC free article] [Abstract] [Google Scholar]
- Hester R, Fassbender C, Garavan H. Individual differences in error processing: a review and reanalysis of three event-related fMRI studies using the GO/NOGO task. Cereb Cortex. 2004;14:986–994. [Abstract] [Google Scholar]
- Holroyd CB, Coles MG. The neural basis of human error processing: reinforcement learning, dopamine, and the error-related negativity. Psychol Rev. 2002;109:679–709. [Abstract] [Google Scholar]
- Kerns JG, Cohen JD, MacDonald AW, III, Cho RY, Stenger VA, Carter CS. Anterior cingulate conflict monitoring and adjustments in control. Science. 2004;303:1023–1026. [Abstract] [Google Scholar]
- Lawrence NS, Ross TJ, Hoffmann R, Garavan H, Stein EA. Multiple neuronal networks mediate sustained attention. J Cogn Neurosci. 2003;15:1028–1038. [Abstract] [Google Scholar]
- Luppino G, Matelli M, Camarda RM, Gallese V, Rizzolatti G. Multiple representations of body movements in mesial area 6 and the adjacent cingulate cortex: an intracortical microstimulation study in the macaque monkey. J Comp Neurol. 1991;311:463–482. [Abstract] [Google Scholar]
- MacDonald AW, III, Cohen JD, Stenger VA, Carter CS. Dissociating the role of the dorsolateral prefrontal and anterior cingulate cortex in cognitive control. Science. 2000;288:1835–1838. [Abstract] [Google Scholar]
- Matsumoto K, Tanaka K. Conflict and cognitive control. Science. 2004;303:969–970. [Abstract] [Google Scholar]
- Mazoyer B, Zago L, Mellet E, Bricogne S, Etard O, Houde O, Crivello F, Joliot M, Petit L, Tzourio-Mazoyer N. Cortical networks for working memory and executive functions sustain the conscious resting state in man. Brain Res Bull. 2001;54:287–298. [Abstract] [Google Scholar]
- Milham MP, Banich MT, Webb A, Barad V, Cohen NJ, Wszalek T, Kramer AF. The relative involvement of anterior cingulate and prefrontal cortex in attentional control depends on nature of conflict. Brain Res Cogn Brain Res. 2001;12:467–473. [Abstract] [Google Scholar]
- Milham MP, Banich MT, Barad V. Competition for priority in processing increases prefrontal cortex's involvement in top-down control: an event-related fMRI study of the stroop task. Brain Res Cogn Brain Res. 2003;17:212–222. [Abstract] [Google Scholar]
- Morecraft RJ, Van Hoesen GW. Cingulate input to the primary and supplementary motor cortices in the rhesus monkey: evidence for somatotopy in areas 24c and 23c. J Comp Neurol. 1992;322:471–489. [Abstract] [Google Scholar]
- Nimchinsky EA, Hof PR, Young WG, Morrison JH. Neurochemical, morphologic, and laminar characterization of cortical projection neurons in the cingulate motor areas of the macaque monkey. J Comp Neurol. 1996;374:136–160. [Abstract] [Google Scholar]
- Paus T, Petrides M, Evans AC, Meyer E. Role of the human anterior cingulate cortex in the control of oculomotor, manual, and speech responses: a positron emission tomography study. J Neurophysiol. 1993;70:453–469. [Abstract] [Google Scholar]
- Paus T. Primate anterior cingulate cortex: where motor control, drive and cognition interface. Nat Rev Neurosci. 2001;2:417–424. [Abstract] [Google Scholar]
- Raichle ME, MacLeod AM, Snyder AZ, Powers WJ, Gusnard DA, Shulman GL. A default mode of brain function. Proc Natl Acad Sci USA. 2001;98:676–682. [Europe PMC free article] [Abstract] [Google Scholar]
- Ridderinkhof KR, Ullsperger M, Crone EA, Nieuwenhuis S. The role of the medial frontal cortex in cognitive control. Science. 2004;306:443–447. [Abstract] [Google Scholar]
- Robson MD, Dorosz JL, Gore JC. Measurements of the temporal fMRI response of the human auditory cortex to trains of tones. Neuroimage. 1998;7:185–198. [Abstract] [Google Scholar]
- Sapir A, d'Avossa G, McAvoy M, Shulman GL, Corbetta M. Brain signals for spatial attention predict performance in a motion discrimination task. Proc Natl Acad Sci USA. 2005;102:17810–17815. [Europe PMC free article] [Abstract] [Google Scholar]
- Shima K, Aya K, Mushiake H, Inase M, Aizawa H, Tanji J. Two movement-related foci in the primate cingulate cortex observed in signal-triggered and self-paced forelimb movements. J Neurophysiol. 1991;65:188–202. [Abstract] [Google Scholar]
- Shulman GL, Fiez JA, Corbetta M, Buckner RL, Miezin FM, Raichle ME, Petersen SE. Common blood flow changes across visual tasks: II. Decreases in cerebral cortex. Journal of Cognitive Neuroscience. 1997;9:648–663. [Abstract] [Google Scholar]
- Talairach J, Tournoux P. Co-planar stereotaxic atlas of the human brain. New York: Thieme; 1988. [Google Scholar]
- Ullsperger M, von Cramon DY. Neuroimaging of performance monitoring: error detection and beyond. Cortex. 2004;40:593–604. [Abstract] [Google Scholar]
- van Veen V, Carter CS. Separating semantic conflict and response conflict in the Stroop task: a functional MRI study. Neuroimage. 2005;27:497–504. [Abstract] [Google Scholar]
- Vogt BA, Finch DM, Olson CR. Functional heterogeneity in cingulate cortex: the anterior executive and posterior evaluative regions. Cereb Cortex. 1992;2:435–443. [Abstract] [Google Scholar]
- Wang Y, Shima K, Sawamura H, Tanji J. Spatial distribution of cingulate cells projecting to the primary, supplementary, and pre-supplementary motor areas: a retrograde multiple labeling study in the macaque monkey. Neurosci Res. 2001;39:39–49. [Abstract] [Google Scholar]
- Weissman DH, Giesbrecht B, Song AW, Mangun GR, Woldorff MG. Conflict monitoring in the human anterior cingulate cortex during selective attention to global and local object features. Neuroimage. 2003;19:1361–1368. [Abstract] [Google Scholar]
Full text links
Read article at publisher's site: https://doi.org/10.1093/cercor/bhl075
Read article for free, from open access legal sources, via Unpaywall:
https://europepmc.org/articles/pmc2693262?pdf=render
Citations & impact
Impact metrics
Citations of article over time
Smart citations by scite.ai
Explore citation contexts and check if this article has been
supported or disputed.
https://scite.ai/reports/10.1093/cercor/bhl075
Article citations
Distinct connectivity patterns in bipolar and unipolar depression: a functional connectivity multivariate pattern analysis study.
BMC Neurosci, 25(1):46, 27 Sep 2024
Cited by: 0 articles | PMID: 39333843 | PMCID: PMC11428473
Music Listening as Exploratory Behavior: From Dispositional Reactions to Epistemic Interactions with the Sonic World.
Behav Sci (Basel), 14(9):825, 16 Sep 2024
Cited by: 0 articles | PMID: 39336040 | PMCID: PMC11429034
Review Free full text in Europe PMC
Neurocognitive mechanisms of emotional interference in native and foreign languages: evidence from proficient bilinguals.
Front Behav Neurosci, 18:1392005, 07 Aug 2024
Cited by: 0 articles | PMID: 39170641 | PMCID: PMC11337870
Association of chronotype with language and episodic memory processing in children: implications for brain structure.
Front Integr Neurosci, 18:1437585, 07 Aug 2024
Cited by: 0 articles | PMID: 39170667 | PMCID: PMC11335642
Time-varying functional connectivity predicts fluctuations in sustained attention in a serial tapping task.
Cogn Affect Behav Neurosci, 24(1):111-125, 22 Jan 2024
Cited by: 5 articles | PMID: 38253775
Go to all (130) article citations
Data
Data behind the article
This data has been text mined from the article, or deposited into data resources.
BioStudies: supplemental material and supporting data
Similar Articles
To arrive at the top five similar articles we use a word-weighted algorithm to compare words from the Title and Abstract of each citation.
Unavoidable errors: a spatio-temporal analysis of time-course and neural sources of evoked potentials associated with error processing in a speeded task.
Neuropsychologia, 46(10):2545-2555, 03 Jun 2008
Cited by: 103 articles | PMID: 18533202
Neuroanatomical dissociation between bottom-up and top-down processes of visuospatial selective attention.
Neuroimage, 32(2):842-853, 06 Jun 2006
Cited by: 154 articles | PMID: 16757180 | PMCID: PMC2652125
Heterogeneity of cingulate contributions to spatial attention.
Neuroimage, 13(6 pt 1):1065-1072, 01 Jun 2001
Cited by: 114 articles | PMID: 11352612
Spatio-temporal dynamics of visual selective attention identified by a common spatial pattern decomposition method.
Brain Res, 1282:84-94, 06 Jun 2009
Cited by: 8 articles | PMID: 19501069
Funding
Funders who supported this work.
Intramural NIH HHS (1)
Grant ID: Z99 DA999999