Abstract
Free full text

Protein Identification False Discovery Rates for Very Large Proteomics Data Sets Generated by Tandem Mass Spectrometry*
Abstract
Comprehensive characterization of a proteome is a fundamental goal in proteomics. To achieve saturation coverage of a proteome or specific subproteome via tandem mass spectrometric identification of tryptic protein sample digests, proteomics data sets are growing dramatically in size and heterogeneity. The trend toward very large integrated data sets poses so far unsolved challenges to control the uncertainty of protein identifications going beyond well established confidence measures for peptide-spectrum matches. We present MAYU, a novel strategy that reliably estimates false discovery rates for protein identifications in large scale data sets. We validated and applied MAYU using various large proteomics data sets. The data show that the size of the data set has an important and previously underestimated impact on the reliability of protein identifications. We particularly found that protein false discovery rates are significantly elevated compared with those of peptide-spectrum matches. The function provided by MAYU is critical to control the quality of proteome data repositories and thereby to enhance any study relying on these data sources. The MAYU software is available as standalone software and also integrated into the Trans-Proteomic Pipeline.
An explicit goal of proteomics is the complete description of a proteome and the measurement of its response to perturbations (1). Over the last few years advances in mass spectrometry-based proteomics have achieved a tremendous increase in proteome coverage (2–11). The volume and heterogeneity of proteomics data required to substantially map out a proteome pose considerable challenges to assess the confidence of peptides and proteins that are inferred from the collected fragment ion spectra (12). Although a number of statistical tools and strategies have been developed to assess the error rate of peptide-spectrum matches (PSMs),1 estimation of the false discovery rate (FDR) of protein identifications in large data sets remains an unresolved problem. This study presents a probabilistic framework and software that addresses this issue.
The most extensive proteome coverage has generally been realized by a strategy typically referred to as shotgun proteomics. Briefly, proteins are extracted from their biological source, enzymatically digested, and optionally fractionated. The resulting peptide mixtures are then analyzed by MS/MS. Peptide and protein identities are inferred by computational analyses of the acquired tandem mass spectra. The data generated by shotgun proteomics experiments are highly redundant, i.e. a subset of the peptides present is repeatedly and preferentially selected for fragmentation and identified. In contrast, other subsets of peptides, e.g. those derived from low abundance proteins, are more difficult to detect, and a large number of fragment ion spectra have to be acquired to increase the likelihood of their detection (2, 13, 14). Consequently, proteomics studies aiming at extensive proteome coverage generate very large data sets consisting of up to millions of fragment ion spectra.
Shotgun proteomics experiments essentially aim at the compilation of a set of reliable protein identifications covering the proteome as extensively as possible. This is achieved by first inferring a set of protein identifications (inference) and second assessing the reliability of these identifications (FDR estimation) (Fig. 1). Briefly, fragment ion spectra are assigned to peptide sequences by generating PSMs using one of a range of database search engines (e.g. Mascot, Sequest, and X!Tandem) (15). Then protein identifications are inferred from the PSMs by assembling the identified peptide sequences into proteins (12, 16). Protein identifications are thus defined as assemblies of PSMs whose peptide sequences map to the same protein (Fig. 1). Neither PSMs nor protein identifications are perfect. Therefore it is essential to control the reliability of PSMs and protein identifications. Various approaches have been developed to estimate the reliability of PSMs (17–20). The FDR (21), i.e. the expected fraction of false positive assignments, has become a widely used measure for reliability of PSMs. The FDR for PSMs can be confidently estimated by means of decoy database search strategies in which the acquired fragment ion spectra are searched against a chimeric protein database containing all (target) protein sequences possibly present in the sample analyzed and an equal number of nonsense (decoy) sequences. Target-decoy strategies are particularly appealing because they constitute a generic and independent approach to validate PSMs generated by any type of identification strategy.
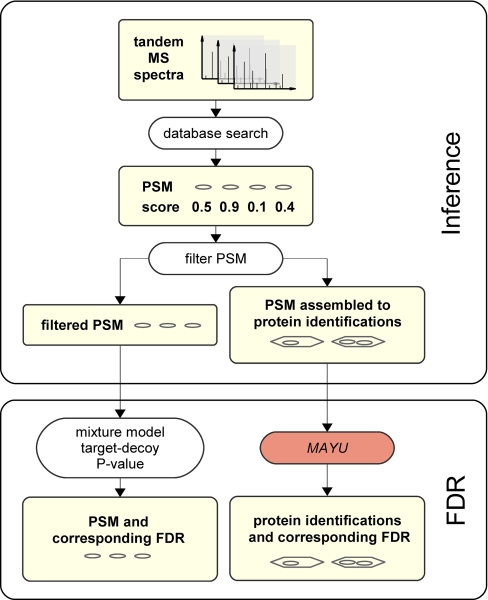
Protein inference and false discovery rate estimation. Tandem mass spectra are searched against a sequence database where each spectrum is assigned to the best matching, i.e. highest scoring, peptide sequence. These assignments are referred to as PSMs. The PSMs can then be filtered according to their score. The quality of the filtered PSMs is usually specified in terms of PSM FDRs. Score cutoffs for PSMs are usually selected according to a user-defined maximal PSM FDR. Alternatively the filtered PSMs can first be assembled to protein identifications. The quality of the assignments is then assessed on the level of protein identifications. MAYU provides a strategy to quantify this quality in terms of the protein identification FDR. Compared with the PSM FDR, the protein identification FDR is a more informative quality measure because it operates on biological entities of interest, i.e. proteins.
Protein identifications, i.e. assemblies of PSMs, are the biologically relevant outcome of a shotgun experiment. Therefore, it is highly desirable to directly control the quality of protein identifications, for example in terms of FDR. Deriving FDR for protein identifications, however, is not as obvious as determining the FDR for PSMs. Because protein identifications are defined by assemblies of PSMs, errors determined at the PSM level propagate to the protein identification level in a non-trivial manner. Therefore, controlling quality on the level of PSMs does not ensure quality at the (biologically relevant) level of protein identifications. This issue has so far not been appropriately appreciated because the distinction between PSMs and protein identifications is frequently blurred in the literature. An estimate of the protein identification FDR, i.e. the expected proportion of false positive protein identifications, has to account for false positive and true positive PSMs distributing differently across the protein database. Although false positive PSMs comparably distribute over all entries in the database (17), true positive PSMs map exclusively to the smaller subset of proteins present in the biological sample. As a result, the protein identification FDR in practice is larger than the PSM FDR (22).
Number, frequency, size, and heterogeneity of proteomics data sets steadily increase (2–10). Available approaches for protein identification focus on the protein inference task and provide reasonable to good error estimates for individual experiments (typically 10–100 LC-MS/MS runs), the complexity level at which most proteomics studies operate (22–26). However, none of these approaches reliably quantifies the confidence in protein identifications in very large, integrated data sets (typically 100 or more LC-MS/MS runs), e.g. in terms of quantifying FDR for protein identifications (Fig. 1). To date, protein identifications in large proteomics data sets have been compiled according to heuristic criteria for which so far no quantitative confidence measures like FDR have been derived at the protein identification level (2, 3, 7, 27, 28).
To close this gap, we developed a generic strategy enabling, for the first time, the quantification of the confidence in protein identifications obtained from a wide range of inference methods (Fig. 1) in data sets of all sizes, especially in large to very large data sets. We refer to this approach as MAYU (no acronym). The approach extends the well established target-decoy strategy designed to estimate FDR at the PSM level (17, 18) to the level of protein identifications, i.e. defined assemblies of PSMs (Fig. 1 ). We applied MAYU to three different data sets varying in instrumentation and species. We found that data set size has a previously underestimated impact on the protein identification FDR. The strategy developed and the tool that implements it could therefore be of critical importance for the generation and quality control of large proteome data sets and databases. The MAYU software and a manual are publicly available for download as standalone software and also implemented in the Trans-Proteomic Pipeline (29) (supplemental Note 1).
EXPERIMENTAL PROCEDURES
Spectral Data and Database Searching
We analyzed three different data sets from studies varying in MS instrumentation and underlying organism. All studies were based on multidimensional fractionation techniques and comprised samples from Caenorhabditis elegans (10), Leptospira interrogans, and Schizosaccharomyces pombe. Although the first data set was acquired on a low resolution LTQ instrument, the latter two were acquired on a high mass accuracy LTQ-FT instrument. The C. elegans project is part of the Center for Model Organism Proteomes initiative; the C. elegans proteome data are available on PeptideAtlas (30). We searched each data set against a composite target-decoy database using Turbo Sequest (31) and Sequest on a Sorcerer machine (Sorcerer™-SEQUEST®, 3.10.4 release). The search results were transformed to the pepXML format and further processed using the Trans-Proteomic Pipeline (29) to the level of PeptideProphet (19) in units of experiments. The pepXML files were then further analyzed with the MAYU software. If a peptide existed in more than one protein sequence the hit was associated with one protein representing the gene locus (Ref. 10; see also Refs. (2) and (8)). We performed all the database searches using a concatenated target-decoy database (17). As target database for the C. elegans data set we chose wormpep170 (WormBase). For the L. interrogans data set we used NC_005824 (National Center for Biotechnology Information), and for the S. pombe data set we used 78.S_pombe (European Bioinformatics Institute). As decoy databases we used the reversed sequences of the target database.
Estimate of Protein Identification FDR
The set of PSMs produced in the course of a proteomics experiment gives rise to protein identifications. A set of PSMs mapping to the same protein sequence defines a protein identification. A protein identification is considered to be true positive if it contains at least one true positive PSM and false positive if all of its PSMs are false positive. This particularly implies that a protein identification that contains false positive PSMs is not necessarily false positive. To estimate the protein identification FDR we estimate the expected number of false positive identifications within a set of protein identifications that has been assembled from a user-defined set of PSMs, e.g. from the set of PSMs at FDR = 0.01.
Based on the well established assumption that false positive PSMs are equally likely to map to either the target or decoy database, we used the number of PSMs mapping to the decoy database as an estimate for the number of false positive PSMs mapping to the target database. The PSM FDR is then estimated as the ratio of the number of PSMs pointing to the decoy and target databases, respectively. Considering that target and decoy databases share the same protein length distribution, the expected number of protein identifications containing false positive PSMs can be estimated analogously using the number of protein identifications mapping to the decoy database (Fig. 2b).
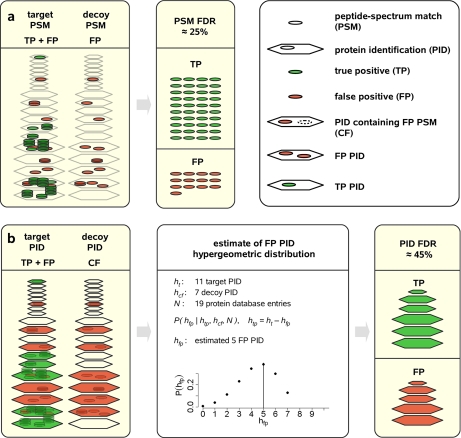
MAYU protein identification false discovery rate estimation. Estimation of the PSM FDR using a target-decoy strategy (a) and the protein identification (PID) FDR by MAYU (b) is shown. PSMs in the target database can be FP or TP. The PSM FDR (the expected fraction of false positive target PSMs) can be estimated with the number of decoy PSMs that are false positive by definition. The PSM FDR is currently the major measure used for quality control of mass spectrometric data sets (a). The derivation of the protein identification FDR has to account for protein identifications containing false positive PSMs (CF) although not being false positive protein identifications (b; two proteins). To estimate the expected number of true positive (htp) and false positive (hfp) protein identifications, MAYU implements a hypergeometric model that takes the number of target (ht) and decoy (hcf) protein identifications and the total number of protein entries in the database (N) as input. The hypothetical example illustrates that the PSM FDR (25%) and the protein identification FDR (45%) can differ largely.
We then estimate the expected number of false positive protein identifications given the inferred number of protein identifications containing false positive PSMs. If we assume that protein identifications containing false positive PSMs uniformly distribute over the target database, then the number of false positive protein identifications is hypergeometrically distributed (Fig. 2b, middle panel). See also supplemental Method/Note 2 for details.
This relation can be seen by regarding the protein database as an urn containing balls, each representing a protein entry. Those balls that correspond to the true positive protein identifications are green, whereas the remaining balls are white. In the urn analogy, observing k false positive protein identifications then corresponds to hitting k white balls after drawing (without replacement) as many times from the urn as we have protein identifications containing false positive PSMs.
Having specified the probability distribution of the number of false positive protein identifications as the hypergeometric distribution, the expected number of false positive protein identifications then follows as the probability weighted average (expectation value). The estimate of the protein identification FDR is computed as the ratio of the expected number of false positive protein identifications and the total amount of protein identifications mapping to the target database.
We also estimated the single hit FDR based on the FDR estimate for the complete set of protein identifications by applying Bayes’ law. The single hit FDR is thus obtained by multiplying the FDR of the complete set of protein identifications by the fraction of single hits among the decoy protein identifications divided by the fraction of single hits among the target protein identifications. In supplemental Method 2 we provide a formal statement of the underlying assumptions and a formal derivation of the individual estimates.
Simulation of Non-uniformly Distributed Protein Identifications Containing False Positive PSM
We performed simulation studies to assess the robustness of the MAYU FDR estimates. We simulated the outcome of proteomics experiments with varying types of distributions for false positive PSMs. For each simulation we first distributed a fixed number of true positive protein identifications across the protein database (comprising N entries). We distributed false positive PSMs according to a truncated exponential distribution (~λe−λx). The rate parameter λ = 1/(u·N) was chosen for different degrees of “uniformity” u. For each simulation we determined the true protein identification FDR and its MAYU estimate. For each seed of distributed true positive protein identifications we performed 50 simulations and report the average relative FDR deviation.
Validation of Single Hit FDR Using Isoelectric Point Information
To validate our model we independently derived an FDR estimate for single hits and compared this value with the estimation of MAYU. We used 67 LC-MS/MS runs of experiment 15 of the C. elegans data set where peptides were fractionated by isoelectric focusing according to their pI (10). We used the standard deviation σΔpI of isoelectric point deviations (ΔpI) as a quality measure for a set H of PSMs,


where pIpr(i) is the isoelectric point of a PSM i predicted by Bioperl (32). pIex(i) corresponds to the experimentally measured isoelectric point of a PSM i determined as the mean isoelectric point of the high confidence peptides of the respective LC-MS/MS run (PSM FDR 0.01). mΔpI(H) denotes the mean of pIpr(i) for PSM i in H.
To specify the correspondence of PSM FDR and σΔpI, we generated a calibration curve with sets Hc,x of PSMs of defined PSM FDR x. These sets were compiled from high confidence target hits with an estimated FDR of zero complemented with an appropriate amount of decoy hits to yield the designated PSM FDR. The corresponding decoy hits were sampled from a set of target-decoy PSMs featuring the designated PSM FDR. Standard deviations were computed using 20 bootstrap samples.
We estimated FDR for the set Hs,x of single PSM protein identifications (single hits) with PSM FDR x by computing σΔpI(Hs,x) and reading out the corresponding FDR by linear interpolation of the calibration curve. For a very small PSM FDR x we observed a significant shift of σΔpI(Hs,x) compared with the calibration curve. Arguing that true positive (TP) single hit peptides focus “better” (see Fig. 4a) in the isoelectric focusing step, we adjust σΔpI(Hs,x) to read out the FDR. The unadjusted initial FDR estimate (FDRini) is used to weight the adjustment according to the initially estimated TP single hits.
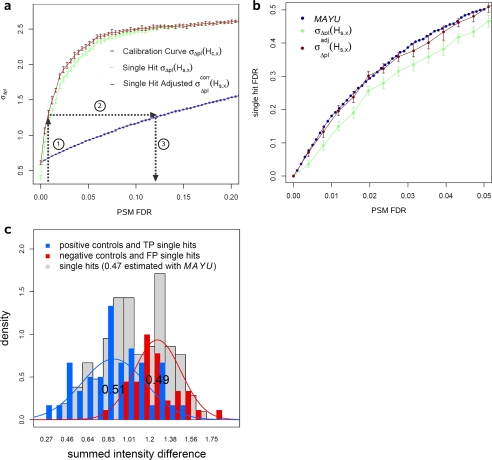
Validation of the false discovery rate estimates of MAYU. We validated the MAYU FDR using two data sets of different size and with two distinct methods. We used experiment 15 (67 LC-MS/MS runs) of the C. elegans data set where experimental pI information of peptides was available (a and b), and we generated synthetic peptides to validate the FDRs of the complete C. elegans data set (1,305 LC-MS/MS runs) (c). Using experiment 15 we derived a measure of the discrepancy between the measured and the computationally predicted pI values of peptides, σΔpI (see “Experimental Procedures”). Sets of PSMs filtered with increasing PSM FDR up to 0.2 show an increase in σΔpI (a, blue curve). σΔpI for only the single hits is significantly higher than for all PSMs over the complete range indicating that the single hit FDR is much higher compared with the PSM FDR (a, green and blue curves). The error bars specify standard deviations from 20 bootstraps. Using σΔpI of all PSMs as a calibration curve we could estimate the single hit FDR assuming that TP single hits are not generally different from the rest of PSMs in terms of pI (b). We also calculated a corrected single hit FDR (a and b, brown curve) by making the reasonable assumption that TP single hit peptides focused better in the isoelectric focusing experiment (a; see offset of σΔpI at zero PSM FDR between the single hits and all PSMs). We found strong consistency between MAYU and the independent method based on peptide pI information (b). We ordered three sets of synthetic peptides corresponding to randomly picked PSMs of three different classes from the complete C. elegans data set (see “Experimental Procedures”). We recorded tandem mass spectra of the synthetic peptides in a directed way using inclusion lists and compared them with the corresponding spectra of the C. elegans data set (c). 35 peptides of the negative control (c, red), 42 peptides of the positive control (c, blue), and 114 peptides of our peptides of interest (c, gray) were identified with a stringent cutoff. We could nicely separate the distributions of positive and negative controls using the summed intensity difference (see “Experimental Procedures”). Based on a Gaussian mixture model of the positive and negative controls we estimated the fraction of false positives of our peptides of interest as 0.49, which is very consistent with the estimated 0.47 of MAYU.

Validation of Single Hit FDR Using Synthetic Peptides
We generated three different sets of synthetic peptides synthesized on a microscale using the SPOT synthesis technology (33, 34). These sets were compiled as follows. 1) As a positive control, we randomly selected 50 peptide sequences that were identified with at least 100 PSMs with a PSM FDR of zero in the search results of the complete C. elegans data set. 2) As a negative control, we randomly selected 50 peptide sequences from decoy proteins with a PSM FDR of 0.01 in the search results of the complete C. elegans data set. 3) As peptides of interest, we randomly selected 150 peptide sequences whose PSMs in the search results of the complete C. elegans data set were single hits.
The search results of the complete C. elegans data set were processed as follows. The PSMs of the complete C. elegans data set were extracted. Ambiguous peptides, peptides longer than 18 amino acids, and cysteine-containing peptides were removed. MAYU was run on the remaining PSMs, and all PSMs corresponding to a PSM FDR of 0.01 were extracted. From these PSMs the three sets were selected as described above.
For all 250 synthetic peptides an inclusion list was generated (35) and measured on an LTQ-FT instrument such that the precursors corresponding to the selected PSMs were targeted. The spectra were searched using Sequest on a Sorcerer machine (Sorcerer-SEQUEST, 3.10.4 release) and filtered for an FDR of 0.01 (a protein identification FDR of 0.01 estimated by MAYU). The resulting tandem mass spectra were then normalized to total ion current and compared with the analogously processed tandem mass spectra of the C. elegans data set. Each peptide was attributed to a score comparing the corresponding C. elegans data set spectrum and inclusion list fragment ion spectrum, i.e. the summed difference of normalized intensities. We trained a Gaussian mixture model for TP/false positive (FP) score distributions by fitting each component to the positive and respective negative controls and then used the mixture model to estimate the expected number of FP single hits for the peptides of interest.
MAYU Analysis on ProteinProphet Protein Identifications
ProteinProphet was run on the pepXML files using runprophet from the Trans-Proteomic Pipeline (29), and target/decoy protein identifications of ProteinProphet were used as input for the MAYU protein identification FDR calculation.
RESULTS
MAYU: FDR for Protein Identifications
MAYU implements a target-decoy strategy to estimate the FDR for a set of protein identifications compiled from a selection of PSMs. Target-decoy strategies to estimate the FDR of PSMs rely on the well established assumption that false positive PSMs uniformly distribute between the target and decoy databases. Consequently, the PSM FDR is estimated as the ratio of PSMs mapping to the decoy and target databases, respectively (Fig. 2 a) (17). MAYU extends this approach to estimate the FDR for protein identifications, i.e. assemblies of PSMs (Fig. 2b).
Prior to MAYU analysis, PSMs are gathered by a target-decoy database search and processed by a protein inference engine, finally yielding a set of target and decoy protein identifications (Fig. 1). Note that MAYU analysis solely aims to estimate the false discovery rate of a set of already inferred protein identifications. MAYU analysis is applicable to the results of any search and protein inference engine (Fig. 5 and supplemental Fig. 2). The following describes the MAYU work flow.
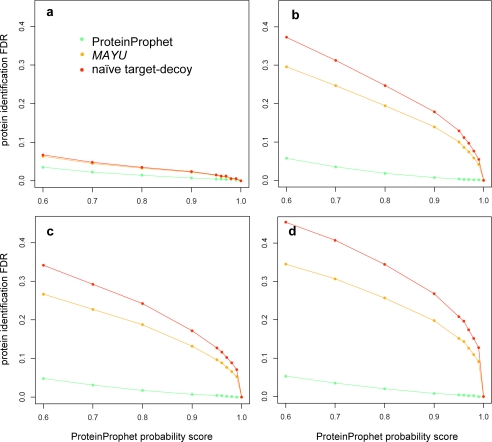
Comparison of different protein identification false discovery rate estimation strategies. We compared the protein identification FDR estimates of MAYU, ProteinProphet, and the naïve target-decoy strategy for four different data set sizes (1, 5, 10, and 20 experiments of the C. elegans data set; a–d). The discrepancy of the alternative FDR estimates and the MAYU estimates grows with data set size.
MAYU processes the supplied list of protein identifications to estimate their FDR. We define a false positive protein identification as being exclusively supported by false positive PSMs and no true positive PSMs. Assuming that false positive PSMs distribute uniformly over the chimeric database, the number of the decoy protein identifications provides an estimate of target protein identifications containing false positive PSMs (seven in the example shown in Fig. 2b). However, the actual number of false positive protein identifications (five in Fig. 2b) is lower than this (naïve target-decoy) estimate as some proteins (two in Fig. 2b) in the target database will contain both true and false positive PSMs.
MAYU uses the number of protein identifications in the target and decoy databases and the total number of protein entries in the database (11, 7, and 19, respectively, in Fig. 2b) to estimate the expected number of false positive protein identifications in the target database (see “Experimental Procedures”, supplemental Method 2, and supplemental Note 2). In summary, starting from a shotgun proteomics data set searched against a target-decoy database, the MAYU work flow provides comprehensive and quantitative error analysis for protein identifications.
Validation of Protein Identification FDR Estimate
We validated the MAYU approach in various ways. First, we assessed the robustness of the FDR estimates under violations of the underlying assumptions. Second, we validated the MAYU FDR estimates by comparing them with an independent approach that estimates the single PSM protein identification (single hit) FDR based on pI information from an isoelectric focusing experiment (67 LC-MS/MS runs, C. elegans data set). Third, we validated the MAYU FDR estimates by confirming the single hit FDR using synthesized peptides corresponding to single hits in the complete C. elegans data set (1,305 LC-MS/MS runs).
We studied the robustness of our FDR estimates under deviations from the assumptions underlying the hypergeometric model. The MAYU protein identification FDR relies on statistics gathered from a target-decoy search, most importantly the number of protein identifications mapping to the decoy database. Following Elias and Gygi (17), we assume this number to equal the number of target protein identifications containing false positive PSMs. To estimate the protein identification FDR with the hypergeometric model, we further assume that protein identifications containing false positive PSMs uniformly distribute over the protein database. To closely meet this assumption, MAYU partitions the protein database into subsets whose entries feature similar size. The protein identification FDR estimate is obtained by applying the hypergeometric model to each of these subsets (see “Experimental Procedures”). The granularity of the partition does not affect the FDR estimate as long as more than 10 size bins are considered (Fig. 3 a). We further conducted simulation studies to assess how deviations from the uniformity assumption influence the MAYU FDR estimate. For each simulation we assumed a fixed number of true positive protein identifications and distributed false positive PSMs according to a truncated geometric distribution. For each simulation we determined the true protein identification FDR and compared it with the MAYU estimate (Fig. 3b). We observed that the MAYU estimates are not compromised, even for considerable deviations from the uniformity assumption.
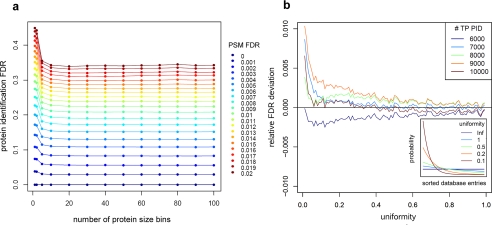
Robustness of the false discovery rate estimates of MAYU. MAYU imposes the assumption that protein identifications containing false positive PSMs uniformly distribute over the protein database. To closely meet this assumption MAYU operates on a partition of the protein database into subsets comprising proteins of similar size. The figure depicts how the size of the partition affects the protein identification FDR estimates for different sets of PSMs defined over the complete C. elegans data set (a). Partitions with more than 10 size bins yield stable FDR estimates and therefore seem to yield the desired protein size homogeneity. b, simulation studies for the complete C. elegans set where we explicitly distributed false positive PSMs according to distributions increasingly deviating from uniformity (see “Experimental Procedures”). We assessed the accuracy of the MAYU estimate in terms of relative deviation from the true FDR depending on the degree of uniformity of the false positive PSM distribution. The inset plot exemplarily depicts four distributions of varying uniformity. We observed that the MAYU estimates do not deviate more than 1% from the true FDR (e.g. 0.2 ± 0.002%) even for considerable deviations from the uniformity assumption. PID, protein identification.
We further validated the MAYU FDR estimates for (non-simulated) experimental data. The MAYU protein identification FDR estimates are ideally validated on a test data set derived from a well defined mixture of proteins. To capture the relevant phenomena complicating protein identification FDR estimates, a protein reference sample of defined composition covering a significant proportion of the entire protein database (e.g. 10%) would be required. Unfortunately, such a test data set is not available and would be exceedingly difficult to construct. We therefore validated MAYU on a large data set providing additional information that allows us to independently derive the single hit FDR gathered from an experiment of the C. elegans data set where peptides were separated by pI using isoelectric focusing (experiment 15, 67 LC-MS/MS runs).
We used the standard deviation of PSM pI deviations as a quality measure for a set of PSMs. This measure grows with the fraction of false positive PSMs because their pI values distribute over the complete pI range in contrast to those of true positive PSMs clustering closely around the measured pI. By exploiting this phenomenon, we related pI information associated to PSMs evidencing single hits to their quality in terms of the FDR (see “Experimental Procedures” and Fig. 4, a and b). Because for single hits the PSM FDR is equivalent to the single hit FDR, we can obtain a protein identification FDR estimate for the set of single hits.
MAYU analysis yielded a single hit FDR about 10-fold higher than the corresponding PSM FDR of the complete set of protein identifications. We found the surprisingly high single hit FDRs obtained by MAYU analysis to be independently confirmed by the pI deviation method (Fig. 4b). We argue that the protein identification FDR estimates produced by MAYU are accurate in the context of typical proteomics studies in the range of 50 LC-MS/MS runs.
We also wanted to validate the MAYU FDR applied to the complete C. elegans data set where the error propagation effects from the PSM FDR to the protein identification FDR are most pronounced. Because there was no pI information available for all 20 experiments, we used a different strategy. We used synthetic peptides and compared their tandem mass spectra with the tandem mass spectra from the C. elegans data set (see “Experimental Procedures”). We generated three sets of peptides: positive controls, negative controls, and peptides of interest. The analysis was performed on the complete data set filtered with a PSM FDR of 0.01.
We recorded tandem mass spectra of the synthetic peptides in a targeted way using inclusion lists and compared them to the corresponding spectra of the C. elegans data set. 35 peptides of the negative control (Fig. 4c, red), 42 peptides of the positive control (blue), and 114 peptides of our peptides of interest (gray) were identified.
We report the summed intensity difference distributions and observed that the peptides of interest show a bimodal distribution with the two apexes very close to the apexes of the positive and negative controls. Based on a Gaussian mixture model for positive and negative controls, we estimated the fraction of false positives of our peptides of interest as 0.49, which is very consistent with the estimated 0.47 of MAYU. Other recent studies confirm this considerable error accumulation among single hits (11).
We conclude that the MAYU estimates are accurate in the context of a very large data set (1,305 LC-MS/MS runs). Considering the results obtained from the pI deviation method, we conclude that MAYU achieves accurate protein FDR estimates that scale well with data set size.
Comparison of Protein Identification FDR Estimation Procedures
We compared protein identification FDR estimates of MAYU, ProteinProphet, and the naïve target-decoy approach. We studied four different subsets of the C. elegans data set varying in size (1, 5, 10, and 20 cumulative experiments). Protein identifications were inferred with ProteinProphet. Protein identification FDRs for these identifications were then determined with MAYU with the built-in functionality of ProteinProphet and the naïve target-decoy strategy.
The naïve target-decoy strategy estimates protein identification FDR analogously to PSM FDR, i.e. by approximating the expected number of FP protein identifications by the number of decoy protein identifier (Table I). We observed that the naïve target-decoy strategy estimate is overly pessimistic (Fig. 5). This is due to true positive (TP) protein identifications contain FP PSMs and thus do not contribute to the pool of FP protein identifications. In contrast, the ProteinProphet FDR estimates are too optimistic. For typically sized data sets of up to 50 LC-MS/MS runs, ProteinProphet and the naïve target-decoy strategy still yield reasonable protein identification FDR estimates. However, the larger the data set size, the more pronounced we found its discrepancy to the MAYU estimates to be. Note the difference between the FDR estimate and protein inference. The foregoing comparison only aims to compare different protein identification FDR estimates; it is not suitable to assess the protein inference functionality of ProteinProphet that provides an effective prioritization of protein identifications using the principle of parsimony.
Table I
The number of target and decoy peptide-spectrum matches, peptide identifications, and protein identifications for three different PSM FDRs are shown. For peptides mapping to several protein sequences only the alphabetically first protein identification was considered. For any PSM FDR, the ratio of decoy to target hits is higher for peptides and again higher for proteins. Unlike for the PSMs, this ratio is not to be mistaken for the FDR for peptide or protein identifications.
PSM FDR | PSMs | Peptide identifications | Protein identifications | ||||||
---|---|---|---|---|---|---|---|---|---|
Target | Decoy | Decoy/Target | Target | Decoy | Decoy/Target | Target | Decoy | Decoy/Target | |
0.05 | 954,661 | 47,725 | 0.05 | 117,293 | 36,419 | 0.310 | 16,459 | 14,354 | 0.872 |
0.01 | 795,502 | 7,947 | 0.01 | 82,628 | 6,394 | 0.077 | 11,089 | 4,974 | 0.449 |
0.001 | 614,486 | 614 | 0.001 | 65,779 | 519 | 0.008 | 8,477 | 506 | 0.060 |
Protein Identification FDR for Various Data Sets
Proteomics studies typically report lists of protein identifications and specify confidence in terms of the FDR at the PSM level. We used various data sets to study how well the PSM FDR reflects the relevant confidence measure for these lists, i.e. the protein identification FDR. To this end, we applied MAYU to several shotgun proteomics data sets, varying in MS instrumentation and studied organism (Fig. 6, a–c). We analyzed isoelectric focusing experiments of C. elegans (10), L. interrogans, and S. pombe samples. The first data set was acquired on a low resolution LTQ instrument, the latter two were acquired on a high mass accuracy LTQ-FT instrument. Protein identifications were compiled by lexicographical protein inference including all PSMs above a score threshold (see “Experimental Procedures”). We observed that protein identification FDR behaves similarly for any of the data sets. Most importantly, we noted that the protein identification FDR is significantly elevated compared with the PSM FDR. We conclude that the PSM FDR is not generally an appropriate confidence measure for lists of protein identifications.
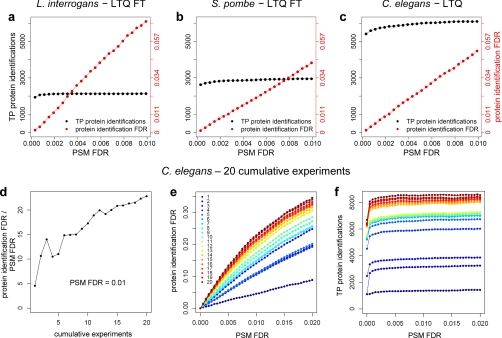
Protein identification false discovery rates behave similarly for data sets of different species and instruments and largely depend on the size of the data set. We applied MAYU to three different data sets of similar size but from different organisms and instruments (59,918 (a), 40,008 (b), and 65,553 (c) target PSMs for a PSM FDR of 0.01). In all three data sets the protein identification FDR is roughly 5 times higher than the PSM FDR. The number of estimated TP protein identifications reaches an apparent maximal number of identifications for a very low PSM FDR (a–c and f). We investigated the influence of data set size using 20 compilations from the C. elegans data set representing 1–20 cumulative experiments. The ratio of the protein identification FDR to PSM FDR (protein identification FDR/PSM FDR) shows clear dependence on data set size (d). In the complete data set (1,305 LC-MS/MS runs) the protein identification FDR is more than 20-fold higher than the PSM FDR. For all data set sizes the protein identification FDR is elevated compared with the PSM FDR over the whole range of PSM FDR (e), and the apparent maximal number of TP protein identifications is reached for a very stringent PSM FDR of roughly 0.005 (f). These data suggest that increasing the PSM FDR beyond 0.005 mainly entails an accumulation of FP protein identifications.
Accumulation of False Positive Protein Identifications for Data Sets of Increasing Size
Using MAYU, we assessed the impact of data set size on the protein identification FDR. For this purpose, we analyzed the currently largest shotgun proteomics data set for C. elegans (10) generated at the Center for Model Organism Proteomes. We subsampled this data set (5,897,279 tandem mass spectra, 1,305 LC-MS/MS runs) into 20 data units of increasing size (Fig. 6, d–f). For each of these units we estimated the FDR of the protein identifications defined for varying PSM FDR cutoffs.
Our analysis revealed that protein identification FDR is strongly influenced by the chosen FDR of PSMs and the size of the respective data set (Fig. 6, d and e). For the 20 data units, the protein identification FDR increased dramatically with the growing PSM FDR (Fig. 6d). In the largest data unit, the protein identification FDR was more than 20 times the corresponding PSM FDR (Fig. 6e).
For all data sets shown, the apparent maximal number of true positive protein identifications achievable by the respective data unit is approached already at a very low PSM FDR in the range of 0.005 (Fig. 6, a–c and f). This quick convergence of the expected number of TP protein identifications suggests that including less reliable PSMs mainly entails accumulation of FP protein identifications without gaining new TP protein identifications. We conclude that to achieve an acceptable protein identification FDR PSMs have to be selected exceedingly stringently with increasing data set size.
DISCUSSION
MAYU is a generic strategy to estimate false discovery rates for protein identifications inferred from shotgun proteomics data sets. An implementation of MAYU is publicly available as standalone software and also integrated into the Trans-Proteomic Pipeline (29) (supplemental Note 1).
Unlike other well established strategies, which quantify the uncertainty of PSMs (frequently also referred to as peptide identifications), MAYU evaluates quality at the level of protein identifications. MAYU implements a novel and generic strategy that generalizes the established target-decoy database search approach for PSMs to estimate the FDR for protein identifications. This approach constitutes a shift from assessing confidence of proteomics data sets at the PSM level by providing instead a confidence measure at the protein level. It should be noted that MAYU is not designed for protein inference, i.e. for the assembly of protein identifications. Instead, MAYU generically assesses the reliability of protein identifications already inferred by any sequence database-driven identification strategy (e.g. search engines such as Sequest and Mascot or protein inference strategies such as ProteinProphet). Besides exemplarily showing the compatibility of MAYU to applications such as lexicographical and ProteinProphet protein inference, we also applied MAYU to non-ambiguous protein inference (supplemental Fig. 2). With regard to conceptual as well as computational issues, MAYU scales well with data set size and is particularly suited for the analysis of very large integrated data sets comprising millions of tandem mass spectra. This concept is also expected to be applicable to other high throughput experiments in biology and medicine that are characterized by indirect observations.
In this study, we assessed MAYU on three heterogeneous data sets including the largest shotgun proteomics data set for C. elegans available to date (10). FDR estimation for protein identifications on data sets of this size has not been solved satisfactorily prior to MAYU. Widely used protein inference tools like ProteinProphet (24) have proven to yield reliable error estimates on data sets at the experiment level (typically 10–50 LC-MS/MS runs) but fail to estimate an accurate protein identification FDR for large data sets (Fig. 5). Current approaches to assemble protein identification from such large data sets rely on common sense criteria for which no quantitative confidence measure at the protein identification level has yet been reported. MAYU overcomes this limitation by providing the FDR for protein identifications in arbitrarily large data sets.
We found that data set size critically influences the protein identification FDR. For the integrated data set (1,305 LC-MS/MS runs), the discrepancy in FDR rose to a more than 20-fold difference even when stringent PSM FDR thresholds were used. Besides these results obtained for protein inference as described under “Experimental Procedures,” we found the same trend toward a larger protein identification FDR for various other protein inference strategies.
This study aimed to quantify the uncertainty of protein identifications in the context of a large scale data set. To the best of our knowledge, this is the first study that independently confirmed the scale of FDR estimates. More precisely, we showed that the scale of FDR estimates for a subset of single hits is in very good agreement with independent estimation methods (Fig. 4). We also showed that the MAYU protein identification FDRs are reproducible regardless of the underlying decoy database (supplemental Fig. 1).
Other approaches like the protein inference engine ProteinProphet have been successfully applied to estimate confidence measures for protein identifications in the context of smaller data sets. ProteinProphet relies on probability estimates of given PSMs to be false to compute the probability of the cognate protein identification to be false. Our results show that in large data sets certain classes of PSMs are enriched in false positive PSMs. This particularly applies to PSMs defining single hits: their actual proportion of false positive instances was nearly 2 orders of magnitude larger than the average FDR for the complete set of PSMs (data not shown). This discrepancy is not a contradiction: because false positive PSMs randomly map to a very large target-decoy database, they are prone to map to a previously unoccupied protein entry and therefore give rise to a single hit. Phenomena like these complicate a reasonable estimate for false positive probabilities for single PSMs and thus challenge approaches like ProteinProphet to estimate FDRs at the protein level in the context of large scale data sets (Fig. 5). In contrast, MAYU estimates protein identification FDR without relying on false positive probabilities for single hit PSMs because FDR estimates are derived solely from statistics gathered at the protein identification level.
In a similar spirit, a Poisson model has been proposed to estimate the proportion of false positive protein identifications given the number of supporting PSMs (22). The parametric model requires the Poisson distribution parameter to be estimated. This estimate is obtained in a heuristic way by assuming different scenarios for the validity of single hits. This model implicitly assumes statistical independence of all PSMs. Our results indicate that this assumption does not hold in general (data not shown), confirming the coarse approximate nature of the Poisson model.
MAYU circumvents the shortcomings of such parametric assumptions. MAYU exploits the underlying target-decoy database search strategy and particularly addresses the phenomenon of true positive protein identifications containing false positive PSMs. This clearly distinguishes MAYU from naïve target-decoy strategies that approximate the number of false positive protein identifications with the number of decoy protein identifications (26). These strategies overestimate the protein identification FDR because they implicitly assume that all protein identifications containing false positive PSMs are false positive (Table I). In particular, the degree of protein identification FDR overestimation grows with data set size (Fig. 5) (26).
Consider the following example where all proteins of a proteome (e.g. Escherichia coli) have been truly identified. The correct protein identification FDR would thus be zero. Due to the accumulation of false positives, i.e. decoy PSMs (not invalidating the true evidence for the protein identifications), the naïve target-decoy strategy will falsely estimate an FDR differing significantly from zero. Furthermore, the naïve target-decoy estimate has the undesired property of being more pessimistic, the more experiments will be carried out.
The MAYU FDR builds on an estimate of the number of protein identifications containing false positive PSMs. In this study we estimated this quantity by the number of decoy protein identifications. Although in principle there are other means to estimate the number of protein identifications containing false positive PSMs, MAYU uses target-decoy database-searched data sets to estimate protein identification FDRs because this represents a well understood and well accepted strategy.
In addition, we found the assumptions underlying the target-decoy search strategy to be well met. The central assumption is that false positive PSMs uniformly distribute between the target and decoy databases. Foregoing studies have discussed and shown the general validity of the target-decoy search strategy (17). Recurrently occurring chemical entities (e.g. unusually modified peptides), which are not represented by the protein database, could potentially challenge the validity of target-decoy strategies because each of these gives rise to a false positive PSM preferably mapping to the same false peptide sequence. However, the overall balanced distribution of all false positive PSMs as well as protein identifications containing false positive PSMs is not compromised because of the large number of such entities.
We saw that protein length has a small and controllable effect on the MAYU FDR estimates (Fig. 3a). We observed that deviations from the uniformity assumption regarding the distribution of protein identifications containing false positive PSMs do not compromise the FDR estimates (Fig. 3b). We furthermore observed that the MAYU FDR estimates are not dependent on the underlying type of decoy database, i.e. reversed or Markov model type (supplemental Fig. 1). Most importantly, we were able to independently reproduce a single hit FDR (Fig. 4), altogether providing a strong indication that the assumptions underlying MAYU analysis are reasonable and provide reliable estimates of the protein identification FDR.
Throughput and sensitivity of mass spectrometers applied to proteomics are steadily increasing. Data repositories have been created to store the vast amount of mass spectrometric data (4, 30, 36, 37). These repositories constitute a cornerstone for proteomics contributing to a wide range of genome-wide studies. Well curated data repositories are a prerequisite of the success of applications like spectrum library searching (38–40), protein expression estimates by spectral counting (41), and targeted proteomics approaches based on the selection of proteotypic peptides (42). MAYU enables more efficient utilization of existing and upcoming data sets in this context by allowing a quantitative quality control of the of protein identifications. MAYU is the first approach to quantify the uncertainty of protein identifications in the context of large scale data sets, thereby allowing automatic curation of proteomics repositories of steadily increasing size. We conclude that approaches like MAYU will significantly enhance genome-wide studies based on shotgun proteomics strategies.
Acknowledgments
We thank Vinzenz Lange, Christian Müller, Lukas Müller, Thomas Fuchs, and Bernd Bodenmiller for careful reading of the manuscript. Furthermore we thank James Eddes, Christian Panse, the Center for Model Organism Proteomes, and the Functional Genomics Center Zurich for support. We also thank the Institute for Systems Biology in Seattle, especially Terry Farrah, Natalie Tasman, and Eric Deutsch for software hosting and implementation into the Trans-Proteomic Pipeline.
Footnotes
* This work was supported, in whole or in part, by National Institutes of Health Contract N01-HV-28179 from the NHLBI. This work was also supported by grants from the Forschungskredit of the University of Zurich, the University of Zurich Research Priority Program in Systems Biology and Functional Genomics, the GEBERT-RÜF Stiftung, Swiss National Science Foundation Grant 31000-10767, and SystemsX.ch, the Swiss initiative for systems biology.
The on-line version of this article (available at http://www.mcponline.org) contains supplemental Notes 1 and 2, Figs. 1 and 2, and Methods 1–3.
1 The abbreviations used are:
- PSM
- peptide-spectrum match
- FDR
- false discovery rate
- single hit
- single peptide-spectrum match protein identification
- TP
- true positive
- FP
- false positive
- LTQ
- linear trap quadrupole.
REFERENCES
Articles from Molecular & Cellular Proteomics : MCP are provided here courtesy of American Society for Biochemistry and Molecular Biology
Full text links
Read article at publisher's site: https://doi.org/10.1074/mcp.m900317-mcp200
Read article for free, from open access legal sources, via Unpaywall:
http://www.mcponline.org/article/S1535947620339293/pdf
Open access at www.mcponline.org
http://www.mcponline.org/cgi/content/abstract/8/11/2405
Open access at www.mcponline.org
http://www.mcponline.org/cgi/reprint/8/11/2405.pdf
Open access at www.mcponline.org
http://www.mcponline.org/cgi/content/full/8/11/2405
Citations & impact
Impact metrics
Citations of article over time
Alternative metrics
Article citations
Exploring the Alternative Proteome with OpenProt and Mass Spectrometry.
Methods Mol Biol, 2836:3-17, 01 Jan 2024
Cited by: 0 articles | PMID: 38995532
Proteomic approach towards identification of seminal fluid biomarkers from individuals with severe oligozoospermia, cryptozoospermia and non-obstructive azoospermia: a pilot study.
Transl Androl Urol, 12(10):1497-1510, 24 Oct 2023
Cited by: 1 article | PMID: 37969768 | PMCID: PMC10643378
Improving quantitation accuracy in isobaric-labeling mass spectrometry experiments with spectral library searching and feature-based peptide-spectrum match filter.
Sci Rep, 13(1):14119, 29 Aug 2023
Cited by: 0 articles | PMID: 37644119 | PMCID: PMC10465558
Mzion enables deep and precise identification of peptides in data-dependent acquisition proteomics.
Sci Rep, 13(1):7056, 29 Apr 2023
Cited by: 1 article | PMID: 37120666 | PMCID: PMC10148867
Rapid Profiling of Protein Complex Reorganization in Perturbed Systems.
J Proteome Res, 22(5):1520-1536, 14 Apr 2023
Cited by: 4 articles | PMID: 37058003 | PMCID: PMC10167687
Go to all (195) article citations
Other citations
Data
Data behind the article
This data has been text mined from the article, or deposited into data resources.
BioStudies: supplemental material and supporting data
Similar Articles
To arrive at the top five similar articles we use a word-weighted algorithm to compare words from the Title and Abstract of each citation.
iProphet: multi-level integrative analysis of shotgun proteomic data improves peptide and protein identification rates and error estimates.
Mol Cell Proteomics, 10(12):M111.007690, 29 Aug 2011
Cited by: 295 articles | PMID: 21876204 | PMCID: PMC3237071
High-throughput database search and large-scale negative polarity liquid chromatography-tandem mass spectrometry with ultraviolet photodissociation for complex proteomic samples.
Mol Cell Proteomics, 12(9):2604-2614, 21 May 2013
Cited by: 21 articles | PMID: 23695934 | PMCID: PMC3769333
Improved False Discovery Rate Estimation Procedure for Shotgun Proteomics.
J Proteome Res, 14(8):3148-3161, 27 Jul 2015
Cited by: 23 articles | PMID: 26152888 | PMCID: PMC4533616
Tandem Mass Spectrum Sequencing: An Alternative to Database Search Engines in Shotgun Proteomics.
Adv Exp Med Biol, 919:217-226, 01 Jan 2016
Cited by: 2 articles | PMID: 27975219
Review
Funding
Funders who supported this work.
NHLBI NIH HHS (2)
Grant ID: N01-HV-28179
Grant ID: N01HV28179