Abstract
Background
Self-identified race or ethnic group is used to determine normal reference standards in the prediction of pulmonary function. We conducted a study to determine whether the genetically determined percentage of African ancestry is associated with lung function and whether its use could improve predictions of lung function among persons who identified themselves as African American.Methods
We assessed the ancestry of 777 participants self-identified as African American in the Coronary Artery Risk Development in Young Adults (CARDIA) study and evaluated the relation between pulmonary function and ancestry by means of linear regression. We performed similar analyses of data for two independent cohorts of subjects identifying themselves as African American: 813 participants in the Health, Aging, and Body Composition (HABC) study and 579 participants in the Cardiovascular Health Study (CHS). We compared the fit of two types of models to lung-function measurements: models based on the covariates used in standard prediction equations and models incorporating ancestry. We also evaluated the effect of the ancestry-based models on the classification of disease severity in two asthma-study populations.Results
African ancestry was inversely related to forced expiratory volume in 1 second (FEV(1)) and forced vital capacity in the CARDIA cohort. These relations were also seen in the HABC and CHS cohorts. In predicting lung function, the ancestry-based model fit the data better than standard models. Ancestry-based models resulted in the reclassification of asthma severity (based on the percentage of the predicted FEV(1)) in 4 to 5% of participants.Conclusions
Current predictive equations, which rely on self-identified race alone, may misestimate lung function among subjects who identify themselves as African American. Incorporating ancestry into normative equations may improve lung-function estimates and more accurately categorize disease severity. (Funded by the National Institutes of Health and others.)Free full text

Genetic Ancestry in Lung-Function Predictions
Abstract
BACKGROUND
Self-identified race or ethnic group is used to determine normal reference standards in the prediction of pulmonary function. We conducted a study to determine whether the genetically determined percentage of African ancestry is associated with lung function and whether its use could improve predictions of lung function among persons who identified themselves as African American.
METHODS
We assessed the ancestry of 777 participants self-identified as African American in the Coronary Artery Risk Development in Young Adults (CARDIA) study and evaluated the relation between pulmonary function and ancestry by means of linear regression. We performed similar analyses of data for two independent cohorts of subjects identifying themselves as African American: 813 participants in the Health, Aging, and Body Composition (HABC) study and 579 participants in the Cardiovascular Health Study (CHS). We compared the fit of two types of models to lung-function measurements: models based on the covariates used in standard prediction equations and models incorporating ancestry. We also evaluated the effect of the ancestry-based models on the classification of disease severity in two asthma-study populations.
RESULTS
African ancestry was inversely related to forced expiratory volume in 1 second (FEV1) and forced vital capacity in the CARDIA cohort. These relations were also seen in the HABC and CHS cohorts. In predicting lung function, the ancestry-based model fit the data better than standard models. Ancestry-based models resulted in the reclassification of asthma severity (based on the percentage of the predicted FEV1) in 4 to 5% of participants.
CONCLUSIONS
Current predictive equations, which rely on self-identified race alone, may misestimate lung function among subjects who identify themselves as African American. Incorporating ancestry into normative equations may improve lung-function estimates and more accurately categorize disease severity. (Funded by the National Institutes of Health and others.)
The use of racial or ethnic classification in medical practice and research has been the subject of debate.1–3 Race and ethnicity are complex constructs incorporating social, cultural, and genetic factors. Currently, pulmonary-function testing is one of the few clinical applications in which self-reported race or ethnic group is used to define a normal range for a test outcome. Normative equations of lung function have been developed by testing large populations categorized on the basis of self-reported race or ethnic group.4 However, many populations are racially admixed, and self-identified racial and ethnic categories are crude descriptors of individual genetic ancestry.5–8 Use of self-reported race or ethnic group may misclassify persons with respect to the normal range for physiological measures, if the measures are dependent on ancestry. Such errors could lead to inaccuracies in evaluating individual pulmonary function and in determining population-specific disease prevalence and severity.
Advances in genetics have led to the development of ancestry informative markers, which allow genetic ancestry to be easily and inexpensively estimated in admixed populations such as self-identified African Americans and Latinos.9–11 Ancestry may serve as a proxy for differentially distributed genetic factors that vary according to historical geographic separations.12 Quantitative traits may vary with ancestry13–15; thus, ancestry may have relevance to lung function, which has a genetic component.16–19 We hypothesized that incorporating measures of individual genetic ancestry into models would improve predictions of pulmonary function in self-identified African Americans.
METHODS
STUDY POPULATIONS
Self-identified African-American participants from five independent study populations were included in our analysis. We first analyzed data for participants in the Coronary Artery Risk Development in Young Adults (CARDIA) study (ClinicalTrials.gov number, NCT00005130).20,21 We subsequently studied the cohorts from the Health, Aging, and Body Composition (HABC) study22 and the Cardiovascular Health Study (CHS) (NCT00005133).23 Finally, we evaluated the effect of ancestry-based models on the classification of disease severity in two study populations of participants with asthma, from the Study of Asthma Phenotypes and Pharmacogenomic Interactions by Race–Ethnicity (SAPPHIRE) (NCT01142947) and the Study of African Americans, Asthma, Genes, and Environments (SAGE).24 For the CARDIA, HABC, and CHS cohorts, participants who had asthma or pulmonary disease at the time of the baseline examination were excluded. See the Supplementary Appendix (available with the full text of this article at NEJM.org) for details of these five study populations.
The CARDIA, HABC, CHS, SAPPHIRE, and SAGE cohorts consisted of 777, 813, 579, 698, and 95 study participants, respectively; the age ranges at baseline were 18 to 30 years, 68 to 81 years, 65 to 93 years, 18 to 56 years, and 18 to 40 years, respectively. In all studies, spirometry was performed in accordance with American Thoracic Society recommendations.25 All subjects provided written informed consent, and each study was approved by the institutional review boards at all participating institutions.
ESTIMATION OF ANCESTRAL ADMIXTURE AND GENOTYPING
To estimate the ancestral admixture in CARDIA participants, we used genomewide genotyping data from the microarray platform (Genome-Wide Human SNP Array 6.0, Affymetrix) used in the Candidate Gene Association Resource (CARe) study. We removed single-nucleotide polymorphisms (SNPs) with more than 5% missing values and those that were not in Hardy–Weinberg equilibrium (P<10−5) as well as SNP pairs in high linkage disequilibrium (r2≥0.8). This left a final sample of 631,243 autosomal SNPs from which to estimate ancestry with the use of the Admixture software program26 (see the Supplementary Appendix for further details). We genotyped 1332 ancestry informative markers in samples obtained from participants in the HABC study and used the software program Structure to estimate the percent African and European ancestry,27 assuming two ancestral populations.28,29 We estimated the percentage of African ancestry in CHS participants by using a maximum-likelihood method incorporating 24 ancestry informative markers,30 and in SAPPHIRE participants by using a maximum-likelihood estimation31 and 107 previously described ancestry informative markers.7 We estimated the percent African ancestry in SAGE participants by using the Structure program and 104 ancestry informative markers.32
STATISTICAL ANALYSIS
In the CARDIA study, we examined the effect of genetic ancestry on measures of lung function (i.e., the forced expiratory volume in 1 second [FEV1], the forced vital capacity [FVC], and the FEV1:FVC ratio) ascertained at the baseline examination. Linear regression models, stratified on the basis of sex, included several covariates: percent African ancestry, age, lifetime pack-years of smoking, body-mass index (BMI), height, square of the height, and study site. Age was centered at 25 years, by which time the majority of lung growth has occurred but the onset of lung-function decline in association with age has not.33,34 Other continuous variables were centered around their study-population means. We also examined differences in the effect of ancestry between the sexes, by adding both sex and interactions between sex and ancestry as covariates to a model analyzing pooled data for men and women.
We evaluated the association between baseline lung function and African ancestry in two additional cohorts, from the HABC study and the CHS. We used linear regression models to assess the associations of genetic ancestry with measures of lung function, after controlling for age, sex, lifetime pack-years of smoking, BMI, height, square of the height, and study site.
To ascertain whether the use of data on genetic ancestry could improve the accuracy of pulmonary-function predictions, we compared models based on self-identified race or ethnic group with models incorporating ancestry as determined by genetic means. To do so, we made use of the age- and sex-specific covariates used by Hankinson and colleagues4 to estimate FEV1 in persons identifying themselves as African Americans. These covariates were selected according to their fit in the third National Health and Nutrition Examination Survey (NHANES III) study population, and the models that included them are referred to herein as standard race-based models. In other models, here called ancestry-based models, we used the age- and sex-specific covariates but also added a term for individual ancestry to assess whether ancestry remained independently associated with FEV1 and FVC and whether the additional covariate improved the overall fit of the model. Model fit was assessed with the use of the mean squared error, the coefficient of determination (R2), and an adjusted coefficient of determination (adjusted R2). The adjusted coefficient is a measure of the proportion of variance in FEV1 explained by the model, accounting for the number of covariates used. We tested these models in the three population-based cohorts: the CARDIA, HABC, and CHS cohorts.
We also categorized asthma severity on the basis of the percentage of the predicted FEV1 cut-off values specified in current U.S. guidelines.35 We assessed the number of participants whose asthma-severity categorization differed when the predicted FEV1 value was obtained from models that incorporated genetic ancestry rather than from models that did not. We derived parameter estimates for these models from data for the CARDIA cohort, using the covariates from Hankinson et al.4 for self-identified African-American men 20 years of age or older and women 18 years of age or older. We then applied these models to the same age groups in the SAPPHIRE and SAGE cohorts.
RESULTS
STUDY POPULATIONS
The CARDIA cohort consisted of 777 participants who identified themselves as African American, with a mean age of 24.5 years at the time of enrollment (Table 1). The mean percentage of African ancestry did not differ significantly between men and women (85.0% and 85.1%, respectively). Participants in the CHS and HABC cohorts were older (Table 2).
Table 1
Baseline Characteristics of CARDIA Study Participants, According to Sex.*
Characteristic | Men (N = 309) | Women (N = 468) |
---|---|---|
Age (yr) | 24.5±3.7 | 24.4±3.9 |
BMI† | 24.5±4.1 | 26.4±6.3 |
Education (yr) | 13.1±2.0 | 13.2±1.8 |
Median income category ($) | 25,000–34,999 | 16,000–24,999 |
No. of lifetime pack-years of smoking | 1.8±3.4 | 1.7±3.5 |
Pulmonary function (liters) | ||
![]() | 3.83±0.56 | 2.85±0.42 |
![]() | 4.63±0.70 | 3.34±0.50 |
African ancestry (%) | 85.0±10.1 | 85.1±9.6 |
Table 2
Characteristics of the CHS and HABC Study Participants.*
Characteristic | CHS (N = 579) | HABC (N = 813) |
---|---|---|
Age — yr | ||
![]() | 72.5 | 73.8 |
![]() | 65–93 | 68–81 |
Female sex — no. (%) | 367 (63.4) | 435 (53.5) |
BMI† | 28.4±5.0 | 28.5±5.2 |
Education — yr | 13.0±5.2 | 11.6±3.5 |
Median income category — $ | 12,000–15,999 | 10,000–25,000 |
Smoking status — no./total no. (%) | ||
![]() | 81/576 (14.1) | 126/811 (15.5) |
![]() | 209 (36.3) | 336 (41.4) |
![]() | 286 (49.7) | 349 (43.0) |
Pulmonary function — liters | ||
![]() | 1.79±0.6 | 2.0±0.6 |
![]() | 2.52±0.8 | 2.7±0.7 |
African ancestry — % | 72.8±20.7 | 78.0±12.8 |
RELATION BETWEEN ANCESTRY AND LUNG FUNCTION
Figure 1 shows the distribution of, and considerable variation in, the percentage of genetically determined African ancestry among male and female CARDIA participants (Panels A and B, respectively). Table 3 presents the results of the test for association between pulmonary-function variables (FEV1, FVC, and the FEV1:FVC ratio) and African ancestry among CARDIA subjects, with adjustment for the following potential confounders: age, lifetime pack-years of smoking, BMI, height, square of the height, and study site. The coefficient for African ancestry represents the change in each lung-function variable for each percentage-point increase in African ancestry, with adjustment for all other variables shown. In both men and women, there were significant inverse relations between African ancestry and FEV1 and between African ancestry and FVC. The association of ancestry with FEV1 among men and among women in the CARDIA cohort is shown in Figures 1C and 1D, respectively. In an analysis of the pooled data for men and women, interaction terms for sex and ancestry neared significance (P = 0.09 for FEV1:FVC and P = 0.07 for FVC), suggesting that the negative relation between ancestry and FVC was greater for men than for women (data not shown).
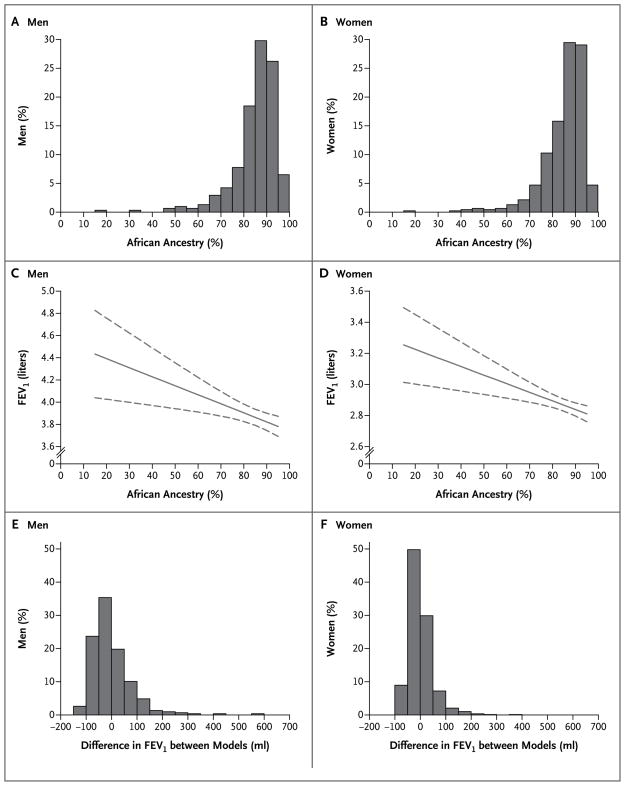
The percentage of African ancestry, as estimated by means of genetic markers, in men and women who identified themselves as African American is shown in Panels A and B, respectively. The relation between African ancestry and FEV1 (indicated as a solid curve, with 95% confidence intervals as dashed curves) is also shown for men and women, in Panels C and D, respectively. The extent and distribution of the differences in predicted FEV1 between the ancestry-based models and the standard race-based models (not including ancestry) in men and women are shown in Panels E and F, respectively.
Table 3
Associations between Lung Function and Covariates of Ancestry-Based Models among Participants in the CARDIA Study, According to Sex.*
Covariate | Men (N = 309) | Women (N = 468) | ||||
---|---|---|---|---|---|---|
FEV1 | FVC | FEV1:FVC | FEV1 | FVC | FEV1:FVC | |
milliliters | milliliters | |||||
Intercept† | 3885.6 | 4763.9 | 81.8 | 2892.0 | 3368.4 | 86.1 |
Age (per yr)‡ | 3.75 | 20.57 | −0.29 | −9.55 | 0.23 | −0.31 |
African ancestry (per percentage point)§ | −8.14 | −12.12 | 0.04 | −5.54 | −5.41 | −0.03 |
Lifetime smoking (per pack-yr)¶ | −9.13 | −7.82 | 0.00 | −14.95 | −8.72 | −0.22 |
BMI (per unit)|| | 9.11 | 20.17 | −0.15 | −0.69 | −0.12 | −0.03 |
Height (per cm)** | 38.53 | 53.70 | −0.12 | 33.62 | 42.58 | −0.07 |
Square of height (per cm2)†† | −0.37 | 0.06 | −0.01 | 0.004 | 0.16 | −0.01 |
Study site | ||||||
![]() | −91.64 | −206.26 | 1.61 | 4.12 | 60.64 | −1.67 |
![]() | 56.15 | −22.97 | 1.46 | −56.82 | −57.75 | −0.18 |
![]() | −44.11 | −193.85 | 2.29 | −58.77 | −91.08 | 0.57 |
We also performed a post hoc analysis in the CARDIA cohort, incorporating additional measures of smoking exposure, including smoking status (current smoker, former smoker, or never smoked), the number of cigarettes smoked per day, and the number of years of smoking. The effect size and the significance of the association between ancestry and lung function were not substantively changed in this analysis (data not shown).
We evaluated the difference in the CARDIA cohort between the predicted FEV1 estimated from the ancestry-based models (Table 3) and the predicted FEV1 estimated from the standard race-based models, which do not include genetically determined ancestry (Table 1 in the Supplementary Appendix). Figure 1 shows the differences in predicted FEV1 between the standard race-based and ancestry-based models for men and women (Panels E and F, respectively). The mean (±SD) absolute difference in predicted FEV1 between the two models for each participant was 55.5±58.3 ml for men and 34.1±36.9 ml for women.
ANALYSES OF ADDITIONAL COHORTS
We did not observe a significant interaction between sex and ancestry with respect to the effect on lung-function outcomes in either the HABC cohort or the CHS cohort (data not shown). Therefore, in each cohort, we analyzed the data for men and women together. In both cohorts, we found that African ancestry was significantly associated with both FEV1 and FVC (Table 4). For each percentage-point increase in African ancestry, the associated changes in FEV1 and FVC were −3.99 ml and −5.50 ml, respectively, among HABC participants, and −2.39 ml and −3.46 ml, respectively, among CHS participants. We performed an additional analysis of the HABC data wherein the height while seated was used in lieu of the height while standing. There was minimal attenuation in the relation between African ancestry and the lung-function variables FEV1 and FVC, and these associations remained significant (Table 2 in the Supplementary Appendix).
Table 4
Associations between Lung Function and Covariates of Ancestry-Based Models among Participants in the CHS and HABC Studies.*
Covariate | CHS (N = 579) | HABC (N = 813) | ||||
---|---|---|---|---|---|---|
FEV1 | FVC | FEV1:FVC | FEV1 | FVC | FEV1:FVC | |
milliliters | milliliters | |||||
Intercept† | 2050.7 | 2902.2 | 70.3 | 2165.8 | 2755.3 | 77.8 |
Age (per yr)‡ | −19.78 | −22.71 | −0.09 | −17.27 | −19.86 | −0.13 |
Sex§ | −398.04 | −640.25 | 3.24 | −348.66 | −442.68 | −0.29 |
African ancestry (per percentage point)¶ | −2.39 | −3.46 | 0.02 | −3.99 | −5.50 | 0.01 |
Lifetime smoking (per pack-yr)|| | −3.66 | −1.75 | −0.09 | −4.80 | −3.45 | −0.06 |
BMI (per unit)** | −1.46 | −4.56 | 0.06 | −1.36 | −10.25 | 0.21 |
Height (per cm)†† | 19.73 | 28.94 | −0.01 | 21.12 | 33.96 | −0.07 |
Square of height (per cm2) | 0.07 | 0.02 | 0.01 | −0.15 | 0.09 | −0.004 |
Study site | ||||||
![]() | 26.74 | 54.55 | −0.12 | 65.86 | 121.31 | −0.28 |
![]() | 54.33 | 50.67 | 1.13 | |||
![]() | 59.88 | 71.89 | 0.53 |
PREDICTING FEV1 WITH THE USE OF STANDARD RACE-BASED MODELS AND ANCESTRY-BASED MODELS
To determine whether the inclusion of ancestry improves the fit of predictive models of pulmonary function, we regressed data for FEV1 on the covariates used in the standard race-based models (i.e., those used elsewhere4 for self-identified African-American women ≥18 years of age and men ≥20 years of age) in the three population-based cohorts (CARDIA, HABC, and CHS). We also used ancestry-based models containing the age-, sex-, and race-specific covariates as well as an additional term for individual genetic African ancestry. The term for ancestry was significantly associated with both FVC and FEV1 in all groups, with the exception of men in the CHS cohort (Tables 3 and 4 in the Supplementary Appendix). The ancestry-based models explained more of the variance than the standard race-based models (except for FEV1 among women in the CHS cohort), even after we had accounted for the number of variables included in the model (reflected in the adjusted R2).
CLASSIFYING ASTHMA SEVERITY
We assessed the severity of asthma in 698 SAPPHIRE participants and 95 SAGE participants. Severity was categorized according to the percent of the predicted FEV1 cutoff values specified in current U.S. guidelines,35 and these percentages were derived from predicted FEV1 values from models with and those without a term for genetic African ancestry. A total of 28 participants (4.0%) in SAPPHIRE and 5 (5.3%) in SAGE would have had a change in their asthma-severity category had ancestry been included in the model used to predict the FEV1. Specifically, the asthma would have been classified as less severe in 14 (2.0%) and more severe in 14 (2.0%) of SAPPHIRE participants, with corresponding reclassifications in 1 (1.1%) and 4 (4.2%) of SAGE participants.
DISCUSSION
We found an association between genetic ancestry and lung function among subjects who identified themselves as African American; specifically, the percentage of African ancestry was inversely associated with lung function in three independent cohorts across a wide range of ages. However, there were differences in the magnitude of the effect across the cohorts, possibly because of the older age of subjects in the HABC and CHS cohorts. The relative contribution of genetic ancestry is likely to be smaller among older persons who have had a greater cumulative exposure to environmental factors that adversely affect lung function.
Extrapolation of the distribution of percentage of African ancestry among the men and women in the CARDIA study to persons of the same sex and age group (18 to 34 years) suggests that for approximately 6.4% of persons in the United States who identify themselves as African Americans (i.e., 0.65 million persons, according to 2008 U.S. Census estimates)36 the percentage of African ancestry would be 15% higher or lower than the mean, suggesting they would be misrepresented by the use of standard race-based models. In these persons, the degree of misestimation of FEV1 could be greater than 122.1 ml in men and 83.1 ml in women, the equivalent of an age-associated loss of lung function of approximately 15 ml per year over a 5-to-8-year period.4
The inverse association between lung function and African ancestry may be especially important when predicted lung-function values are used to assess the severity of lung disease.35,37,38 Current clinical practice guidelines use the FEV1:FVC ratio or percentages of predicted lung function in the assessment of the severity of chronic obstructive pulmonary disease (COPD),39,40 the severity of asthma,35 and the degree of overall lung impairment.41 Accordingly, inaccurate estimates of percentages of predicted lung function may result in the misclassification of disease severity and impairment.41 For example, an estimated 2.1 million self-identified African Americans have asthma.42 On the basis of the percentage of the predicted FEV1 value, the severity of the asthma would be misclassified for approximately 4% of these patients (i.e., 84,000 patients), were ancestry not taken into account. Misclassification of severity might affect even more patients with COPD, a condition more common than asthma. An improvement in the accuracy of predicted lung function may lead to more appropriate treatment for the level of impairment, resulting in more effective and efficient care.
There are some important limitations of our study. First, our analysis does not address population groups other than self-identified African Americans, such as Latinos, who have more complex patterns of ancestral admixture. Second, the association between lung function and ancestry found in our study may be the result of factors other than genetic variation, such as premature birth, prenatal nutrition, socioeconomic status, and other environmental factors.43,44 Third, we did not study a replication population with the same age range as that of the CARDIA cohort. Thus, we may have overestimated the association between ancestry and lung function in the CARDIA participants, who were young adults. Finally, some researcher groups used different statistical approaches to estimate ancestry in their respective study populations. We have found previously, however, that different approaches (e.g., Markov models and maximum-likelihood estimation) produce highly correlated results from the same set of markers.8,45 The consistency of our findings across three cohorts, despite the different methods for estimating ancestry, underscores the robustness of the association with ancestry.
In conclusion, our study shows that incorporating measures of individual genetic ancestry into normative equations of lung function in persons who identify themselves as African Americans may provide more accurate predictions than formulas based on self-reported ancestry alone. The same argument may apply to other ancestrally defined groups; further studies in this area are necessary. Further studies are also needed to determine whether estimates informed by genetic ancestry are associated with health outcomes. It remains to be seen whether differences associated with race or ethnic group in the response to medications that control asthma46,47 are more tightly associated with estimates of ancestry. Although measures of individual genetic ancestry may foster the development of personalized medicine, large clinical trials and cohort studies that include assessments of genetic ancestry are needed to determine whether measures of ancestry are more useful clinically than a reliance on self-identified race alone.
Acknowledgments
Supported by contracts (N01-HC-48047 through 48050 and N01-HC-95095) from the National Heart, Lung, and Blood Institute (NHLBI), National Institutes of Health (NIH), as well as grants from the NIH (HL078885, HL088133, AI077439, and ES015794, to Dr. Burchard; K23HL093023-01, to Dr. Kumar; R01 HL71862, R01 HL71017, and R01 AG032136, to Dr. Reiner; and R01 AI79139, R01 AI61774, and R01 HL79055, to Dr. Williams), the Robert Wood Johnson Foundation Amos Medical Faculty Development Program and the Flight Attendant Medical Research Institute (to Dr. Burchard), the American Asthma Foundation (to Dr. Williams), and the Tobacco-Related Disease Research Program (New Investigator Award 15KT-0008, to Dr. Choudhry). The CHS was supported by NHLBI grants (N01-HC-85079 through N01-HC-85086, N01-HC-35129, N01 HC-15103, N01 HC-55222, N01-HC-75150, N01-HC-45133, and U01 HL080295), with additional funding from the National Institute of Neurological Disorders and Stroke and a grant from the National Institute on Aging (NIA) (U19 AG023122 from the Longevity Consortium). The CARe Consortium was supported in part by a grant from the NHLBI (with a full description of the funding available at http://public.nhlbi.nih.gov/GeneticsGenomics/home/care.aspx). Health ABC was supported in part by the Intramural Research Program of the NIH and by the NIA, through contracts (N01-AG-6-2101, N01-AG-6-2103, and N01-AG-6-2106), and by a grant from the National Center for Research Resources (U54-RR020278) for the genotyping.
We thank the participants in the studies (CARDIA, HABC, CHS, SAGE, and SAPPHIRE) for their involvement; the study coordinators and staff for their dedication and commitment; the research institutions, study investigators, field staff, and study participants for their contributions in creating the CARe Consortium, a resource for biomedical research; the UCSF Biostatistics High Performance Computing System, which was used to perform some computations; and Dr. Albert Levin for his input.
Footnotes
Disclosure forms provided by the authors are available with the full text of this article at NEJM.org.
References
Full text links
Read article at publisher's site: https://doi.org/10.1056/nejmoa0907897
Subscription required at content.nejm.org
http://content.nejm.org/cgi/reprint/363/4/321.pdf
Free to read at content.nejm.org
http://content.nejm.org/cgi/content/abstract/363/4/321
Subscription required at content.nejm.org
http://content.nejm.org/cgi/content/full/363/4/321
Citations & impact
Impact metrics
Article citations
Impact of race-neutral global reference equations on spirometry interpretation in healthy children in The Gambia.
IJTLD Open, 1(9):418-421, 01 Sep 2024
Cited by: 0 articles | PMID: 39301136 | PMCID: PMC11409167
Implications of Race Adjustment in Lung-Function Equations.
N Engl J Med, 390(22):2083-2097, 19 May 2024
Cited by: 6 articles | PMID: 38767252 | PMCID: PMC11305821
FEV1Q: a race-neutral approach to assessing lung function.
Eur Respir J, 63(4):2301622, 04 Apr 2024
Cited by: 6 articles | PMID: 38485146
Association of Preoperative Lung Function with Complications after Lobectomy Using Race-Neutral and Race-Specific Normative Equations.
Ann Am Thorac Soc, 21(1):38-46, 01 Jan 2024
Cited by: 3 articles | PMID: 37796618
Spirometry in Mixed-Race Civil War Veterans.
Ann Am Thorac Soc, 20(8):1217-1219, 01 Aug 2023
Cited by: 0 articles | PMID: 37159952 | PMCID: PMC10405614
Go to all (157) article citations
Data
Data behind the article
This data has been text mined from the article, or deposited into data resources.
BioStudies: supplemental material and supporting data
Clinical Trials (3)
- (1 citation) ClinicalTrials.gov - NCT01142947
- (1 citation) ClinicalTrials.gov - NCT00005130
- (1 citation) ClinicalTrials.gov - NCT00005133
Similar Articles
To arrive at the top five similar articles we use a word-weighted algorithm to compare words from the Title and Abstract of each citation.
Meta-analysis across Cohorts for Heart and Aging Research in Genomic Epidemiology (CHARGE) consortium provides evidence for an association of serum vitamin D with pulmonary function.
Br J Nutr, 120(10):1159-1170, 12 Sep 2018
Cited by: 5 articles | PMID: 30205856 | PMCID: PMC6263170
Race- and Ethnicity-Based Spirometry Reference Equations: Are They Accurate for Genetically Admixed Children?
Chest, 162(1):184-195, 13 Jan 2022
Cited by: 2 articles | PMID: 35033507 | PMCID: PMC9279648
"Race correction" in pulmonary-function testing.
N Engl J Med, 363(4):385-386, 07 Jul 2010
Cited by: 13 articles | PMID: 20647189
Race Adjustment of Pulmonary Function Tests in the Diagnosis and Management of COPD: A Scoping Review.
Int J Chron Obstruct Pulmon Dis, 19:969-980, 29 Apr 2024
Cited by: 1 article | PMID: 38708410 | PMCID: PMC11067926
Review Free full text in Europe PMC
Funding
Funders who supported this work.
Intramural NIH HHS
NCRR NIH HHS (2)
Grant ID: U54-RR020278
Grant ID: U54 RR020278
NHLBI NIH HHS (38)
Grant ID: N01 HC-55222
Grant ID: N01-HC-85081
Grant ID: N01HC95095
Grant ID: R01 HL079055
Grant ID: K23HL093023-01
Grant ID: N01-HC-48048
Grant ID: N01-HC-48049
Grant ID: N01-HC-85085
Grant ID: N01-HC-95095
Grant ID: R01 HL71862
Grant ID: K23 HL093023
Grant ID: N01HC85079
Grant ID: R01 HL071017
Grant ID: HL088133
Grant ID: N01HC55222
Grant ID: N01 HC045133
Grant ID: N01-HC-85083
Grant ID: N01-HC-85086
Grant ID: N01 HC015103
Grant ID: N01-HC-75150
Grant ID: N01-HC-85079
Grant ID: N01-HC-85084
Grant ID: N01HC48047
Grant ID: R01 HL071862
Grant ID: R01 HL078885
Grant ID: R01 HL71017
Grant ID: HL078885
Grant ID: N01-HC-48047
Grant ID: N01-HC-85082
Grant ID: N01HC85086
Grant ID: R01 HL088133
Grant ID: R01 HL79055
Grant ID: U01 HL080295
Grant ID: K23 HL093023-03
Grant ID: N01 HC035129
Grant ID: N01-HC-48050
Grant ID: N01-HC-85080
Grant ID: N01HC75150
NIA NIH HHS (6)
Grant ID: N01-AG-6-2103
Grant ID: R01 AG032136
Grant ID: N01-AG-6-2106
Grant ID: P30 AG021332
Grant ID: N01-AG-6-2101
Grant ID: U19 AG023122
NIAID NIH HHS (6)
Grant ID: R01 AI061774
Grant ID: R01 AI61774
Grant ID: U19 AI077439
Grant ID: R01 AI79139
Grant ID: AI077439
Grant ID: R01 AI079139
NIEHS NIH HHS (2)
Grant ID: R01 ES015794
Grant ID: ES015794
NIGMS NIH HHS (1)
Grant ID: T32 GM007546