Abstract
Free full text

Metagenomic Analysis of the Human Distal Gut Microbiome
Abstract
The human intestinal microbiota is composed of 1013 to 1014 microorganisms whose collective genome (“microbiome”) contains at least 100 times as many genes as our own genome. We analyzed ~78 million base pairs of unique DNA sequence and 2062 polymerase chain reaction–amplified 16S ribosomal DNA sequences obtained from the fecal DNAs of two healthy adults. Using metabolic function analyses of identified genes, we compared our human genome with the average content of previously sequenced microbial genomes. Our microbiome has significantly enriched metabolism of glycans, amino acids, and xenobiotics; methanogenesis; and 2-methyl-d-erythritol 4-phosphate pathway–mediated biosynthesis of vitamins and isoprenoids. Thus, humans are superorganisms whose metabolism represents an amalgamation of microbial and human attributes.
Our body surfaces are home to microbial communities whose aggregate membership outnumbers our human somatic and germ cells by at least an order of magnitude. The vast majority of these microbes (10 to 100 trillion) inhabit our gastrointestinal tract, with the greatest number residing in the distal gut, where they synthesize essential amino acids and vitamins and process components of otherwise indigestible contributions to our diet such as plant polysaccharides (1). The most comprehensive 16S ribosomal DNA (rDNA) sequence-based enumeration of the distal gut and fecal microbiota published to date underscores its highly selected nature. Among the 70 divisions (deep evolutionary lineages) of Bacteria and 13 divisions of Archaea described to date, the distal gut and fecal microbiota of the three healthy adults surveyed was dominated by just two bacterial divisions, the Bacteroidetes and the Firmicutes, which made up >99% of the identified phylogenetic types (phylotypes), and by one prominent methanogenic archaeon, Methanobrevibacter smithii (2). The human distal gut microbiome is estimated to contain ≥100 times as many genes as our 2.85–billion base pair (bp) human genome (1). Therefore, superorganismal view of our genetic landscape should include genes embedded in our human genome and the genes in our affiliated microbiome, whereas a comprehensive view of our metabolome would encompass the metabolic networks based in our microbial communities.
Progress made with 16S rDNA-based enumerations has disclosed significant differences in community membership between healthy adults (2, 3), differences that may contribute to variations in normal physiology between individuals or that may predispose to disease. For example, studies of humans and gnotobiotic mouse models indicate that our mutualistic relations with the gut microbiota influence maturation of the immune system (4), modulate responses to epithelial cell injury (5), affect energy balance (6), and support biotransformations that we are ill-equipped to perform on our own, including processing of xenobiotics (7). However, we are limited by our continued inability to cultivate the majority of our indigenous microbial community members, biases introduced by preferential polymerase chain reaction (PCR) amplification of 16S rDNA genes and by our limited ability to infer organismal function from these gene sequences.
As with soil (8) and ocean (9), metagenomic analysis of complex communities offers an opportunity to examine in a comprehensive manner how ecosystems respond to environmental perturbations, and in the case of humans, how our microbial ecosystems contribute to health and disease. In the current study, we use a metagenomics approach to reveal microbial genomic and genetic diversity and to identify some of the distinctive functional attributes encoded in our distal gut microbiome.
Sequencing the microbiome
Although whole-genome shotgun sequencing and assembly have historically been applied to the study of single organisms, recent reports from Venter et al. (9) and Baker et al. (10) have demonstrated the utility of this approach for studying mixed microbial communities. Variations in the relative abundance of each member of the microbial community and their respective genome sizes determine the final depth of sequence coverage for any organism at a particular level of sequencing. This means that the genome sequences of abundant species will be well represented in a set of random shotgun reads, whereas lower abundance species may be represented by a small number of sequences. In fact, the size and depth of coverage (computed as the ratio between the total length of the reads placed into contigs and the total size of the contigs) of genome assemblies generated from a metagenomics project can provide information on relative species abundance.
A total of 65,059 and 74,462 high-quality sequence reads were generated from random DNA libraries created with fecal specimens of two healthy humans (subjects 7 and 8). These two subjects, ages 28 and 37, female and male, respectively, had not used antibiotics or any other medications during the year before specimen collection (11). The combined sequenced distal gut “microbiome” of subjects 7 and 8 consisted of 17,668 contigs that assembled into 14,572 scaffolds, totaling 33,753,108 bp. The scaffolds ranged in size from 1000 to 57,894 bp and the contigs from 92 to 44,747 bp. The average depth of sequence coverage in contigs was 2.13-fold. Forty percent of the reads (56,292 total) could not be assembled into contigs, most likely because of a combination of low depth of coverage and low abundance of some organisms within the specimens. Together, these singletons accounted for an additional 45,078,063 bp of DNA.
A total of 50,164 open reading frames (ORFs) were predicted from the data set (25,077 for subject 7 and 25,087 for subject 8). These ORFs correspond to 19,866 unique database matches (13,293 for subject 7; 12,273 for subject 8; 5700 that were present in both). ORF-based alignments against public databases identified 259 contigs in subject 7 and 330 in subject 8 that could be assigned to members of Archaea, plus 5992 contigs from subject 7 and 7138 from subject 8 assignable to members of Bacteria (table S1). The remaining contigs either did not match any known ORFs or were ambiguously assigned.
Insight into the diversity within our samples was obtained by comparison of a subset of the shotgun reads to the completed sequence of Bifidobacterium longum, a member of the lactic acid bacteria present in the distal gut of healthy humans (12). A total of 1965 reads from the combined data set from subjects 7 and 8 could be aligned to the genome sequence of B. longum. These reads represented a total of 1,617,706 bp of DNA sequence, which corresponds to ~0.7-fold coverage of the B. longum genome. There was a great deal of heterogeneity in nucleotide sequence in the 1965 reads that aligned with the B. longum genome sequence (80 to 100% identity) with 52% of the reads aligned at less than 95% identity (Fig. 1A). These data suggest that these reads are not derived from a single discrete strain of B. longum in subjects 7 and 8, but instead, reflect the presence of multiple strains, as well as other Bifidobacterium phylotypes in the distal gut microbiota.
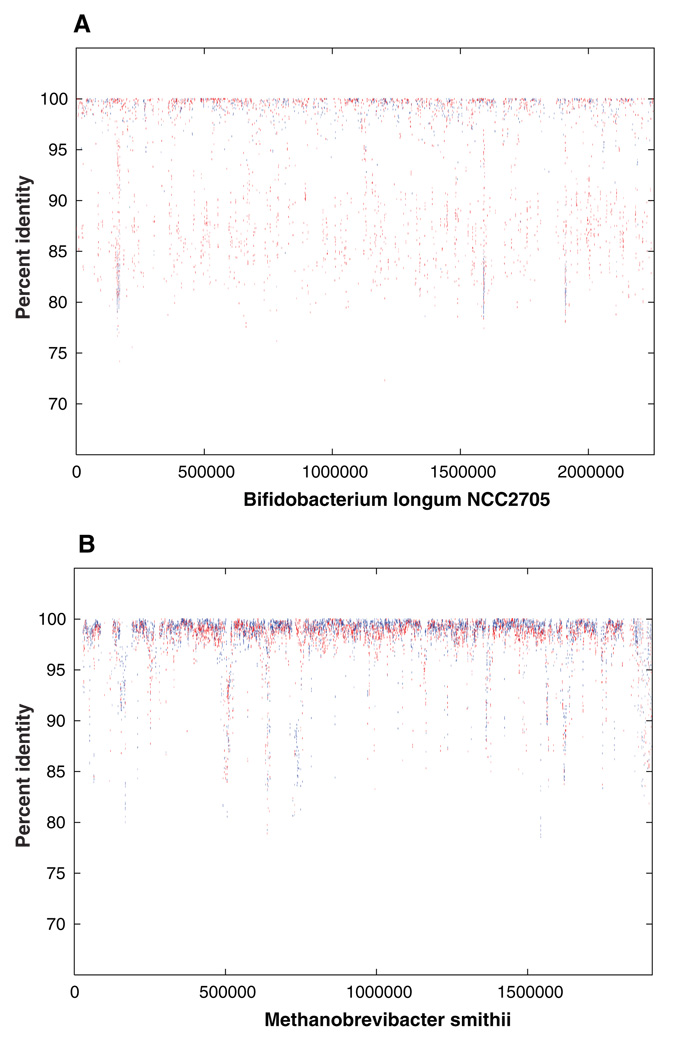
Comparison of random metagenome reads with completed genome of Bifidobacterium longum and Methanobrevibacter smithii. (A) Percent identity plot (PIP) of alignments of shotgun reads along the genome of B. longum strain NCC2705. The x axis represents the coordinate along the genome, and the y axis represents the percent identity of the match. (B) Percent identity plot (PIP) of the alignment of shotgun reads along the draft genome of M. smithii. The x axis represents the coordinate along a pseudomolecule formed by concatenating all contigs in the M. smithii draft assembly. The y axis represents the percent identity of the match. The variation in the percent identity of the matches between the shotgun reads from subjects 7 and 8 as compared with the genome sequences of B. longum NCC2705 suggests considerable diversity among Bifidobacterium-like organisms within our samples. Alignments of the reads to the draft genome of M. smithii exhibit a much narrower range of percent identity (89% of alignments were at 95% or better identity as compared with 48% for B. longum), consistent with lower levels of diversity among archaeal members of the gastrointestinal tract.
Previous work (2) has shown that archaeal species, in particular M. smithii, are also major players in the human distal gut ecosystem. M. smithii was represented in our data set at ~3.5-fold coverage, as indicated by the 7955 shotgun reads that matched this draft assembly (Fig. 1B). The presence of M. smithii is also supported by the identification of eight partial-length 16S rDNA sequences with 99.65 to 100% identity to M. smithii. Unlike B. longum, the majority (89%) of alignments to M. smithii had 95% or better sequence identity to the draft assembly, indicating low divergence between Methanobrevibacter strains present in our samples. More than half of the archaeal contigs in our data set had significant similarity to M. smithii: 145 of 259 archaeal contigs in subject 7 and 174 of 330 archaeal contigs from subject 8 had matches ≥100 bases, and ≥80% identity to a deep draft assembly of this genome (13), consistent with previous reports on the abundance of this species in the human gut.
Identifying phylotypes
We explored bacterial diversity in both stool samples with analysis of 16S rDNA sequences from the random shotgun assemblies and from libraries of cloned, PCR-amplified 16S rDNA. Phylogenetic assessments of the local microbial community census provide a benchmark for interpreting the functional predictions from metagenomic data. Of the 237 partial bacterial-length 16S rDNA sequences identified in the shotgun assemblies, we selected 132 bacterial sequences for further analysis (2, 11). Using a 97% minimum pair-wise similarity definition, 72 bacterial phylotypes were identified. Only one archaeal phylotype was identified (i.e., M. smithii). Sixteen bacterial phylotypes (22.2%) were novel, and 60 (83.3%) represented uncultivated species. The bacterial phylotypes were assigned to only two divisions, the Firmicutes (62 phylotypes, 105 sequences) and the Actinobacteria (10 phylotypes, 27 sequences). Sixty of the Firmicute phylotypes belonged to the class Clostridia, including Clostridia cluster XIV and Faecalibacteria. Analysis of 2062 near–full-length PCR-amplified 16S rDNA sequences (1024 from subject 7 and 1038 from subject 8) revealed a similar phylogenetic distribution among higher-order taxa, but a more diverse population at the species level. Using a ≥97% similarity phylotype threshold, 151 phylotypes were identified (23% novel; 150 Firmicutes; 1 Actinobacteria) (fig. S1A). Similar analyses based on a ≥99% similarity threshold are provided (11).
Although there were no Bacteroidetes 16S rDNA sequences identified in the random assemblies and clone libraries, amplification with species-specific 16S rDNA primers yielded sequences from Bacteroides fragilis and Bacteroides uniformis. This relative paucity of Bacteroidetes sequences is in conflict with data from other studies (2, 3). This discrepancy may have been caused by the known biases associated with the fecal lysis and DNA extraction methods used in the current study with respect to Bacteroides spp. (14); although less likely, it is also possible that members of the Bacteroidetes division are less abundant in the feces of subjects 7 and 8. In addition, with respect to the PCR-amplified 16S rDNA sequence data, there may be biases associated with the primers or PCR reaction conditions. Similar arguments may apply to other underrepresented taxa as well, such as the Actinobacteria and Proteobacteria phyla. Estimates of diversity indicated that at least 300 unique bacterial phylotypes would be detected with continued sequencing from these stool samples (fig. S1, B to D).
Comparative functional analysis of the distal gut microbiome
To delineate how the human distal gut microbiome endows us with physiological properties that we have not had to evolve on our own, we explored the metabolic potential of the microbiota in subjects 7 and 8 using KEGG (Kyoto Encyclopedia of Genes and Genomes, version 37) pathways and COGs (Clusters of Orthologous Groups) (15, 16). Both annotation schemes contain categories of metabolic functions organized in multiple hierarchical levels: KEGG analysis maps enzymes onto known metabolic pathways; COG analysis uses evolutionary relations (orthologs) to group functionally related genes. Odds ratios were used to rank the relative enrichment or underrepresentation of COG and KEGG categories. An odds ratio of one indicates that the community DNA has the same proportion of hits to a given category as the comparison data set; an odds ratio greater than one indicates enrichment (more hits to a given category than expected), whereas an odds ratio less than one indicates underrepresentation (fewer hits to a given category than expected). Odds ratios for the KEGG pathway involved in biosynthesis of peptidoglycan (table S3), a major component of the bacterial cell wall, are consistent with expectations: The human gut microbiome is highly enriched relative to the human genome (77.88), similar to all sequenced bacteria (1.83), and moderately enriched relative to all sequenced Archaea (7.06).
Because we have not obtained saturation (see below), we cannot be confident that a given COG or KEGG pathway component is not present in the human distal gut microbiome. Therefore, we have focused our analysis on identified functional categories that are enriched relative to previously sequenced genomes.
BLAST comparisons of all sequences yielded 62,036 hits to the COG database, corresponding to 2407 unique COGs. ACE and Chao1 estimates of community richness were 2558 and 2553 COGs, respectively. This observed degree of community COG diversity is greater than that described for an acid mine drainage (1824 COGs), but less than that described for whale fall (3332), soil (3394), and Sargasso Sea samples (3714) (17). The number of KEGG pathways and COG terms enriched in the human distal gut microbiomes of subjects 7 and 8 is listed in table S2. KEGG maps and COG assignments can be found at (11, 18, 19).
The metabolome of the human distal gut microbiota
Both human subjects showed similar patterns of enrichment for each COG (Fig. 2) and KEGG (Fig. 3) category involved in metabolism. However, compared with subject 7, subject 8 was enriched for energy production and conversion; carbohydrate transport and metabolism; amino acid transport and metabolism; coenzyme transport and metabolism; and secondary metabolites biosynthesis, transport, and catabolism (Fig. 2). At this time, it is not clear whether these differences reflect limited coverage of their microbiomes or other factors such as host diet, genotype, and life-style. The analysis presented below combines the genes identified in the fecal microbiotas of both subjects to create an aggregate “human distal gut microbiome.”
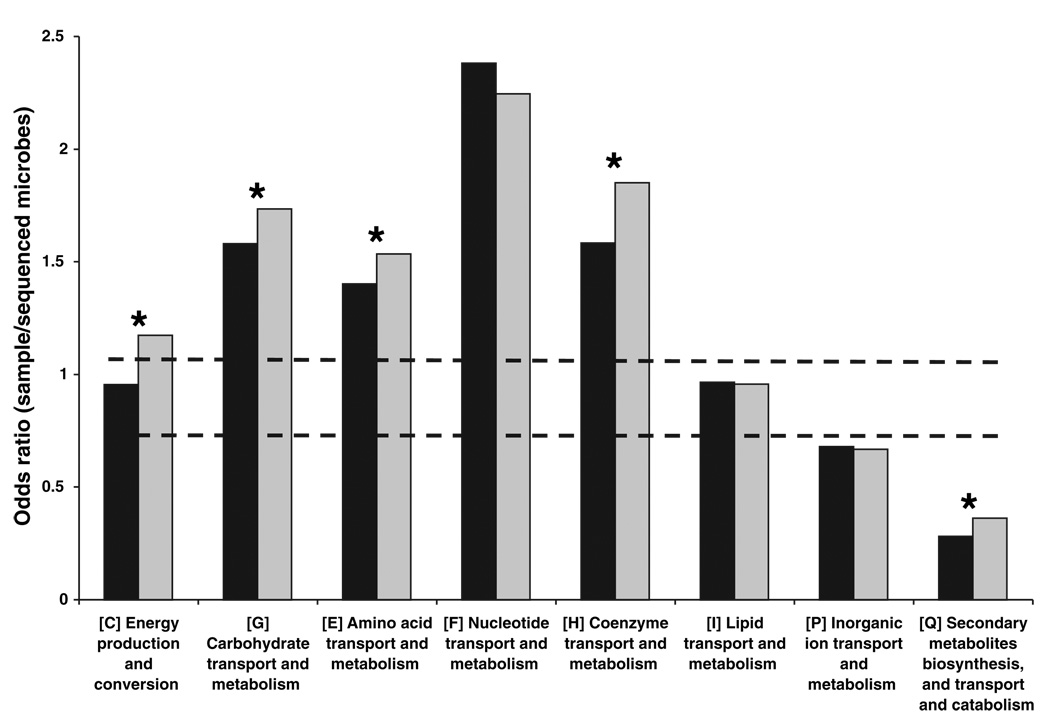
COG analysis reveals metabolic functions that are enriched or underrepresented in the human distal gut microbiome (relative to all sequenced microbes). Color code: black, subject 7; gray, subject 8. Bars above both dashed lines indicate enrichment, and bars below both lines indicate underrepresentation (P < 0.05). Asterisks indicate categories that are significantly different between the two subjects (P < 0.05). Secondary metabolites biosynthesis includes antibiotics, pigments, and nonribosomal peptides. Inorganic ion transport and metabolism includes phosphate, sulfate, and various cation transporters.
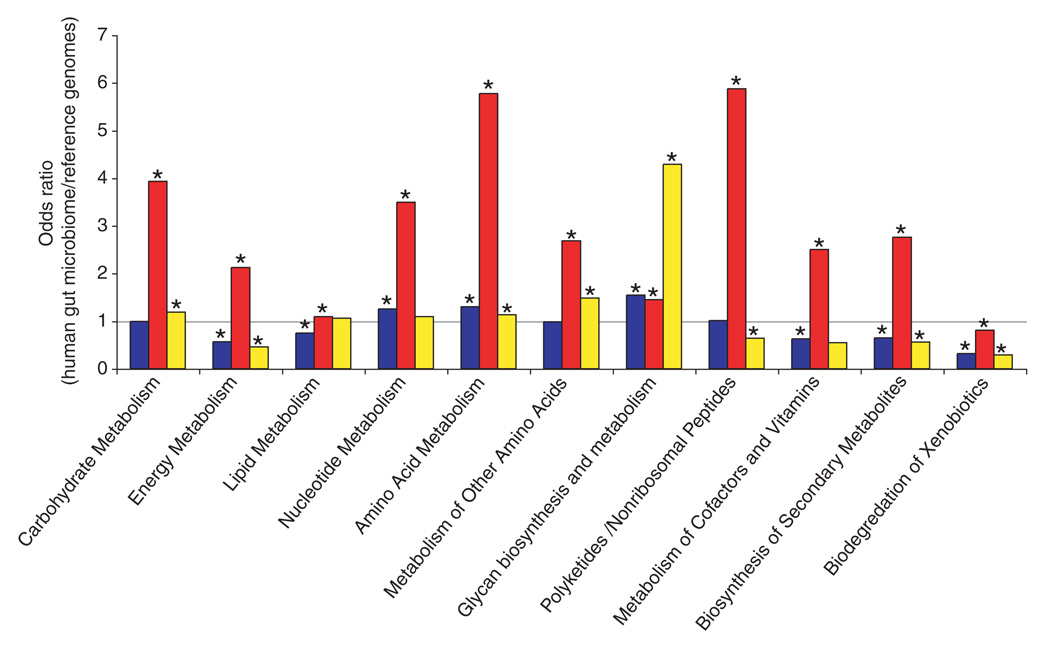
KEGG pathway reconstructions reveal metabolic functions that are enriched or underrepresented in the human distal gut microbiome as follows: both samples compared with all sequenced bacterial genomes in KEGG (blue), the human genome (red), and all sequenced archaeal genomes in KEGG (yellow). Asterisks indicate enrichment (odds ratio > 1, P < 0.05) or underrepresentation (odds ratio < 1, P < 0.05). The KEGG category, “metabolism of other amino acids,” includes amino acids that are not incorporated into proteins, such as β-alanine, taurine, and glutathione. Odds ratios are a measure of relative gene content based on the number of independent hits to enzymes present in a given KEGG category.
The plant polysaccharides that we commonly consume are rich in xylan-, pectin-, and arabinose-containing carbohydrate structures. The human genome lacks most of the enzymes required for degrading these glycans (20). However, the distal gut microbiome provides us with this capacity (1) (Fig. 3 and tables S3 and S4). The human gut microbiome is enriched for genes involved in starch and sucrose metabolism (fig. S2) plus the metabolism of glucose, galactose, fructose, arabinose, mannose, and xylose (table S4). At least 81 different glycoside hydrolase families are represented in the microbiome, many of which are not present in the human “glycobiome” (table S5).
Host mucus provides a consistent reservoir of glycans for the microbiota and thus, in principle, can serve to mitigate the effects of marked changes in the availability of dietary polysaccharides (1). Gnotobiotic mouse models of the human gut microbiota have indicated that α-linked terminal fucose in host glycans is an attractive and accessible source of energy for members of the microbiota such as the Bacteroidetes (1, 6). Several COGs responsible for fucose utilization are enriched in the human gut microbiome relative to all microbial genomes (table S4).
Fermentation of dietary fiber or host-derived glycans requires cooperation of groups of microorganisms linked in a trophic chain. Primary fermenters process glycans to short-chain fatty acids (SCFAs), mainly acetate, propionate, and butyrate, plus gases (i.e., H2 and CO2). The bulk of SCFAs are absorbed by the host: Together, they account for ~10% of calories extracted from a Western diet each day (21). COG analyses demonstrated enrichment of key genes involved in generating acetate, butyrate, lactate, and succinate in the gut microbiome compared with all microbial genomes in the COG database (table S6). The most enriched COG was related to butyrate kinase (odds ratio of 9.30), an enzyme that facilitates formation of butyryl-coenzyme A by phosphorylating butyrate. This enrichment underscores the important commitment of the distal gut microbiota to generating this biologically significant SCFA, which serves as the principal energy source for colonocytes and may fortify the intestinal mucosal barrier by stimulating their growth (22).
Accumulation of H2, an end product of bacterial fermentation, reduces the efficiency of processing of dietary polysaccharides (23). Production of methane by mesophilic methanogenic archaeons is a major pathway for removing H2 from the human distal gut (23), although sulfate reduction and homoacetogenesis serve as alternate pathways. Enhancement of bacterial growth rates, fermentation of polysaccharides, and SCFA production have been observed when bacteria (e.g., Fibrobacter succinogenes and Ruminococcus flavefaciens) are cocultured with a Methanobrevibacter species (24). The distal gut microbiome is enriched for many COGs representing key genes in the methanogenic pathway (Fig. 4, C and D), consistent with the importance of H2 removal from the distal gut ecosystem via methanogenesis.
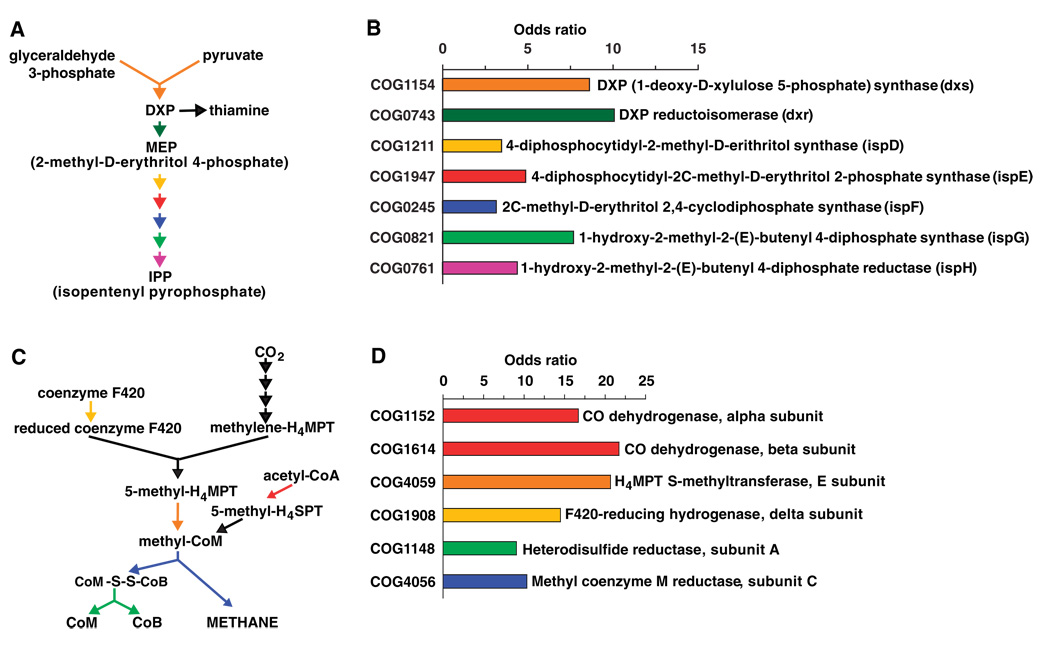
Isoprenoid biosynthesis via the MEP pathway and methanogenesis are highly enriched in the distal gut microbiome. (A) MEP pathway for isoprenoid biosynthesis. (B) Odds ratio for each COG in the MEP pathway. All enzymes necessary to convert DXP to IPP and thiamine are enriched (P < 0.0001 relative to all sequenced microbes). (C) Location and role of key enzymes in methanogenesis. (D) Odds ratio for each COG highlighted in (C).
The distal gut microbiome is enriched for a variety of COGs involved in synthesis of essential amino acids and vitamins (tables S7 and S8). COGs representing enzymes in the MEP (2-methyl-d-erythritol 4-phosphate) pathway, used for biosynthesis of deoxyxylulose 5-phosphate (DXP) and isopenteryl pyrophosphate (IPP), are notably enriched (P < 0.0001; relative to all sequenced microbes) (Fig. 4, A and B). DXP is a precursor in the biosynthesis of vitamins essential for human health, including B1 (thiamine) and B6 (pyridoxal form) (25). IPP is found in all known prokaryotic and eukaryotic cells and can give rise to at least 25,000 known derivatives, including archaeal membrane lipids (26), carotenoids (27), and cholesterol (28). Together, these results indicate that the MEP pathway is much better represented in the distal human gut microbiome than was previously known. The MEP pathway has been proposed as a target for developing new antibiotics, because some pathogenic bacteria use the MEP pathway instead of the mevanolate pathway for IPP biosynthesis (29). However, our metagenomic study indicates that this approach may be detrimental to the microbiota and, in turn, the host.
Detoxification of xenobiotics could impact the host in a variety of ways, ranging from susceptibility to cancer to the efficiency of drug metabolism. Dietary plant–derived phenolics, such as flavonoids and cinnamates, have pronounced effects on mammalian cells (30–32). Hydrolysis of phenolic glycosidic or ester linkages occurs in the distal gut by microbial β-glucosidases, β-rhamnosidases, and esterases (33). The human distal gut microbiome is enriched for β-glucosidase (COG1472, COG2723 in table S4; P < 0.0005; glycosidase families GH3 and GH9 in table S5). Glucuronide conjugates of xenobiotics and bile salts induce microbial β-glucuronidase activity (34). The microbiome is enriched in this enzyme activity (i.e., COG3250; table S4). KEGG analysis also indicates enrichment for pathways involved in degradation of tetrachloroethene, dichloroethane, caprolactam, and benzoate (table S3).
Conclusion
This metagenomics analysis begins to define the gene content and encoded functional attributes of the gut microbiome in healthy humans. Future studies are needed to provide deeper coverage of the microbiome and to assess the effects of age, diet, and pathologic states (e.g., inflammatory bowel diseases, obesity, and cancer) on the distal gut microbiome of humans living in different environments. Periodic sampling of the distal gut microbiome (and of our other microbial communities) may provide insights into the effects of environmental change on our “microevolution.” The results should provide a broader view of human biology, including new biomarkers for defining our health; new ways for optimizing our personal nutrition; new ways for predicting the bio-availability of orally administered drugs; and new ways to forecast our individual and societal predispositions to disorders such as infections with pathogens, obesity, and misdirected or maladapted host immune responses of the gut.
Footnotes
Supporting Online Material
www.sciencemag.org/cgi/content/full/312/5778/1355/DC1
Materials and Methods
Figs. S1 and S2
Tables S1 to S8
References
References and Notes
Full text links
Read article at publisher's site: https://doi.org/10.1126/science.1124234
Read article for free, from open access legal sources, via Unpaywall:
https://europepmc.org/articles/pmc3027896?pdf=render
Citations & impact
Impact metrics
Citations of article over time
Alternative metrics

Discover the attention surrounding your research
https://www.altmetric.com/details/101774227
Article citations
Exploring the gut microbiome's role in colorectal cancer: diagnostic and prognostic implications.
Front Immunol, 15:1431747, 17 Oct 2024
Cited by: 0 articles | PMID: 39483461 | PMCID: PMC11524876
Review Free full text in Europe PMC
Regular Exercise Training Induces More Changes on Intestinal Glucose Uptake from Blood and Microbiota Composition in Leaner Compared to Heavier Individuals in Monozygotic Twins Discordant for BMI.
Nutrients, 16(20):3554, 20 Oct 2024
Cited by: 0 articles | PMID: 39458548 | PMCID: PMC11510543
Thai Fermented Soybean (Thua-Nao) Prevents Early Stages of Colorectal Carcinogenesis Induced by Diethylnitrosamine and 1,2-Dimethylhydrazine Through Modulations of Cell Proliferation and Gut Microbiota in Rats.
Nutrients, 16(20):3506, 16 Oct 2024
Cited by: 0 articles | PMID: 39458500 | PMCID: PMC11510544
A systematic framework for understanding the microbiome in human health and disease: from basic principles to clinical translation.
Signal Transduct Target Ther, 9(1):237, 23 Sep 2024
Cited by: 0 articles | PMID: 39307902 | PMCID: PMC11418828
Review Free full text in Europe PMC
Effects of Interactions between Feeding Patterns and the Gut Microbiota on Pig Reproductive Performance.
Animals (Basel), 14(18):2714, 19 Sep 2024
Cited by: 0 articles | PMID: 39335303 | PMCID: PMC11428678
Go to all (2,400) article citations
Other citations
Data
Data behind the article
This data has been text mined from the article, or deposited into data resources.
BioStudies: supplemental material and supporting data
Similar Articles
To arrive at the top five similar articles we use a word-weighted algorithm to compare words from the Title and Abstract of each citation.
Phylogenetic analysis of the human gut microbiota using 16S rDNA clone libraries and strictly anaerobic culture-based methods.
Microbiol Immunol, 46(8):535-548, 01 Jan 2002
Cited by: 248 articles | PMID: 12363017
The comparative genomics of Bifidobacterium callitrichos reflects dietary carbohydrate utilization within the common marmoset gut.
Microb Genom, 4(6), 15 Jun 2018
Cited by: 11 articles | PMID: 29906260 | PMCID: PMC6096940
Metagenomic reconstructions of gut microbial metabolism in weanling pigs.
Microbiome, 7(1):48, 26 Mar 2019
Cited by: 52 articles | PMID: 30914068 | PMCID: PMC6436221
Human gut microbiota and bifidobacteria: from composition to functionality.
Antonie Van Leeuwenhoek, 94(1):35-50, 13 Mar 2008
Cited by: 124 articles | PMID: 18338233
Review
Funding
Funders who supported this work.
NIAID NIH HHS (3)
Grant ID: AI51259
Grant ID: R01 AI051259
Grant ID: R01 AI051259-04
NIDDK NIH HHS (1)
Grant ID: DK70977