Abstract
Purpose
The authors' previous models predicted local formation of diabetic retinopathy (DR) in adults with diabetes and existing retinopathy. Here they derived a multivariate model for local prediction of DR onset in patients with no previous retinopathy.Methods
Seventy-eight eyes from 41 diabetes patients were tested annually for several years. The presence or absence of DR at the last study visit was the outcome measure, and measurements of risk factors from the previous visit were used for prediction. Logistic regression was used to assess the relationship between DR development and 7 factors: multifocal ERG (mfERG) implicit time (IT) Z-score, sex, diabetes duration, blood glucose, HbA1c, age, and diabetes type. Thirty-five retinal zones, spanning 45°, were constructed from the mfERG stimulus elements. The maximum IT Z-score for each zone was calculated based on data from 50 control subjects. ROC curve analysis, using fivefold cross-validation, was used to determine the model's predictive properties.Results
Mild DR developed in 80 of 2730 retinal zones (3%) in 29 of 78 eyes (37%). Multivariate analysis showed mfERG IT to be predictive for DR development in a zone after adjusting for diabetes type. The multivariate model has a sensitivity of 80% and a specificity of 74%.Conclusions
mfERG IT is a good predictor of DR onset, 1 year later, in patients with diabetes without DR. It can be used to assess the risk for DR development in these patients and may be a valuable outcome measure in evaluation of novel prophylactic therapeutics directed at impeding DR.Free full text

Multifocal Electroretinograms Predict Onset of Diabetic Retinopathy in Adult Patients with Diabetes
Abstract
Purpose.
The authors' previous models predicted local formation of diabetic retinopathy (DR) in adults with diabetes and existing retinopathy. Here they derived a multivariate model for local prediction of DR onset in patients with no previous retinopathy.
Methods.
Seventy-eight eyes from 41 diabetes patients were tested annually for several years. The presence or absence of DR at the last study visit was the outcome measure, and measurements of risk factors from the previous visit were used for prediction. Logistic regression was used to assess the relationship between DR development and 7 factors: multifocal ERG (mfERG) implicit time (IT) Z-score, sex, diabetes duration, blood glucose, HbA1c, age, and diabetes type. Thirty-five retinal zones, spanning 45°, were constructed from the mfERG stimulus elements. The maximum IT Z-score for each zone was calculated based on data from 50 control subjects. ROC curve analysis, using fivefold cross-validation, was used to determine the model's predictive properties.
Results.
Mild DR developed in 80 of 2730 retinal zones (3%) in 29 of 78 eyes (37%). Multivariate analysis showed mfERG IT to be predictive for DR development in a zone after adjusting for diabetes type. The multivariate model has a sensitivity of 80% and a specificity of 74%.
Conclusions.
mfERG IT is a good predictor of DR onset, 1 year later, in patients with diabetes without DR. It can be used to assess the risk for DR development in these patients and may be a valuable outcome measure in evaluation of novel prophylactic therapeutics directed at impeding DR.
The number of patients with diabetes in the United States is expected to drastically increase, nearly doubling in the next 25 years.1 Because diabetes is the leading cause of new cases of preventable blindness in Americans of working age (20–74 years old),2 the ongoing search for better and earlier treatments for diabetic eye disease has become even more important. The gold standard treatment, laser photocoagulation,3 is aimed only at the end stage of eye disease and has many side effects, including decreases in peripheral and night vision.4 Despite much research there is still nothing more effective for saving vision in the late stages of this disease.5 Furthermore, even with several efforts, no successful ocular treatments for mild to moderate nonproliferative diabetic retinopathy (NPDR) have been found. However, patients at this stage of disease often report visual symptoms such as difficulty driving at night.6
Currently, patients with early NPDR are counseled only on blood sugar and blood pressure control and monitored. Although better blood sugar and blood pressure control have been shown to be effective at reducing retinopathy progression at early stages of disease,5 they are often difficult for patients to accomplish and are not successful in all patients. Some patients still progress to worsening retinopathy even with improved blood sugar control.7–9 The vision of many patients could be preserved, at least for a longer period, if earlier treatments were available.
Previous studies that have examined candidate predictive factors for diabetic eye disease have focused primarily on those risk factors leading to the most severe NPDR and treatable sight-threatening proliferative diabetic retinopathy (PDR). They have found an association between severe NPDR and many factors, including duration of diabetes, blood pressure, and smoking.10 Furthermore, PDR has been linked to neuropathy, decreased visual acuity, elevated triglycerides, type 1 diabetes, and previous levels of retinopathy.11,12 PDR has also been linked with higher HbA1c percentage levels,13 and a reduction in HbA1c percentage reduces the need for and risks associated with laser photocoagulation.14
Changes in the retina are, however, detectable at much earlier stages, and studies have begun to focus on candidate predictive factors for earlier retinopathy development. The UK Prospective Diabetes Study Group study group found that even one or two microaneurysms are predictive of future worsening of retinopathy and should not be ignored.15 Other factors, such as microalbuminuria, hypertension, and neuropathy, have also been found to increase the risk for earlier retinopathy.16,17 There are also many indications that neural changes take place in the retina during diabetes and that these changes take place before vascular changes are apparent.18–21 These changes have been identified using several different electrophysiological tests and have been shown to worsen as diabetic retinal disease progresses. Several possible mechanisms for this progressive change have been suggested.22 Neural changes are thus obvious candidates as risk factors for predicting retinopathy. Electrophysiological tests of neural function are fast, objective, and noninvasive.
We have previously developed multivariate models using the multifocal electroretinogram (mfERG) implicit time (IT), a local measure of retinal neural function, along with other diabetes health measures, to predict new local retinopathy development over 1 to 3 years in patients with diabetes and some retinopathy at baseline.23–26 The present study derives a new model to predict retinopathy development, within a 1-year window, in a cohort of subjects who have no diabetic retinopathy at baseline. Prediction of the earliest clinically visible diabetic changes in the eye has implications for clinical care and tracking eye health. Perhaps more important, the relatively short-term predictive measures allow rapid clinical trials of new drugs targeting the earlier stages of DR, whereas the alternative, visual acuity outcomes, demand more protracted studies.
Subjects and Methods
Subjects
Forty-one adult subjects with diabetes completed the study. Both eyes of each subject were used in the analysis, with the exception of four eyes that were excluded at the start of the study because of media opacities, intraocular lens implants, and myopic degeneration, leaving a total of 78 eyes. All subjects were between 25 and 65 years of age (mean age, 52.4 ± 10.8 years). Eight subjects had type 1 diabetes, and 33 subjects had type 2 diabetes. Some subjects in this study were part of our earlier predictive models, but their data here are from more recent follow-up visits not previously reported. In addition, 50 healthy nondiabetic control subjects, between 21 and 67 years of age (mean age, 43.7 ± 13.0 years) participated, and their data were used for normalization to create Z-scores and local templates for the mfERG analysis.
All subjects had 20/25 or better acuity, refractive error between −6D and +4D, and no retinopathy at the start of the study. Subject demographic data are shown in Table 1. All subjects provided informed written consent, and the procedures were in compliance with the Declaration of Helsinki and the University of California Berkeley Committee for the protection of Human Subjects.
Table 1.
Demographic Data
Group | Patients (n) | Sex (Male/Female Ratio) | Age (y) | Duration (y) | Blood Glucose (mg/dL) | HbA1c (%) | Average mfERG Implicit Time (Z-scores) | Retinopathy Development |
---|---|---|---|---|---|---|---|---|
All diabetes patients | 41 | 22:19 | 52.4 ± 10.4 | 9.1 ± 4.4 | 181.0 ± 86.0 | 8.44 ± 1.7 | 0.87 ± 1.66 | 20 yes, 21 no |
Type 1 diabetes | 8 | 3:5 | 38.3 ± 10.6 | 13.0 ± 6.3 | 118.0 ± 46.9 | 7.8 ± 0.9 | −0.30 ± 0.78 | 5 yes, 3 no |
Type 2 diabetes | 33 | 19:14 | 55.8 ± 7.7 | 8.0 ± 3.2 | 183.3 ± 91.8 | 8.6 ± 1.8 | 1.17 ± 1.65 | 15 yes, 18 no |
Controls | 50 | 21:29 | 43.7 ± 13.0 | N/A | 103.2 ± 20.8 | N/A | 0.00 ± 0.67 | N/A |
Study Timeline and Testing Procedures
All subjects with diabetes were followed up over time, and study visits occurred annually until either retinopathy developed (n = 20) or the study ended (n = 21). Recruitment was continuous; the average time in the study was 3 years (range, 1–6 years.) Patients with and without retinopathy at the end of the study had the same range and mean for time followed. The mean for patients in whom retinopathy developed was 3.35 ± 1.2 years, and in those in whom it did not it was 3.23 ± 1.3 years.
Every study visit included a full medical history, nonfasting blood glucose reading (One Touch Ultra; Lifescan, Milpitas, CA), HbA1c percentage (At Home A1c; FlexSite Diagnostics, Palm City, FL) measurement, dilated fundus examination with photographs covering the central 50° (Carl Zeiss Meditec, Dublin, CA), and mfERG (VERIS; EDI, Redwood City, CA).
The mfERGs were recorded as described in Ng et al.,25 Han et al.,24 and Bearse et al.26 Briefly, subjects' pupils were fully dilated with 1% tropicamide and 2.5% phenylephrine, and a Burian-Allen contact lens electrode was used. A ground electrode was placed on to the right earlobe, and the contralateral eye was occluded during the recording. An mfERG (VERIS 4.3; EDI) system was used with a scaled 103 hexagon stimulus array displayed on a CRT at a frame rate of 75 Hz. The stimulus array subtended 45° on the retina. An eye camera display refractor unit was used. This allowed the patient to self-adjust a cross in the center of the display to best focus. The monitor was calibrated every 6 months to ensure quality measures over time. It remained stable between calibrations. Preamplifier filters were set to 10 to 100 Hz, and retinal signals were amplified 100,000 times. The contrast of the stimulus display was set to 98%, with the white elements at 200 cd/m2 and the dark elements at <2 cd/m2. Seventeen percent spatial averaging was used with a single iteration of artifact removal.
First-order P1 kernel mfERG implicit times were measured with the template scaling method previously described.27 For this method local templates were constructed from the mean local waveforms of the 50 control subjects. The template is scaled in both amplitude and time to fit a subject's corresponding local waveform by minimizing the least squares difference between the subject's local waveform and the local template. This information is then used to derive the P1 implicit time and the N1-P1 amplitude. A parameter indicating the goodness-of-fit (statfit) was generated for each response. Although none of the local response fits reached this criterion, a fit (statfit) parameter of more than 0.8 would have been rejected. Each local implicit time (IT) measure for the diabetic subjects was converted to a Z-score using the mean and SD obtained from the controls. For our instrumentation and control data, one mfERG IT Z-score is equal to 0.9 ms when averaged over all measured retinal locations.28
To be spatially conservative, 35 retinal zones (each of which contained two or three neighboring hexagons) were constructed from the 103 stimulus elements. For each zone, a maximum IT Z-score was assigned from the two or three Z-scores from hexagons in that zone. All fundus photographs were graded in a detailed and masked fashion by a retinal specialist for the presence or absence of retinopathy without knowledge of other study results. The mfERG array was overlaid onto the digital photographs to match the location of retinopathy with any applicable mfERG zones (Fig. 1).
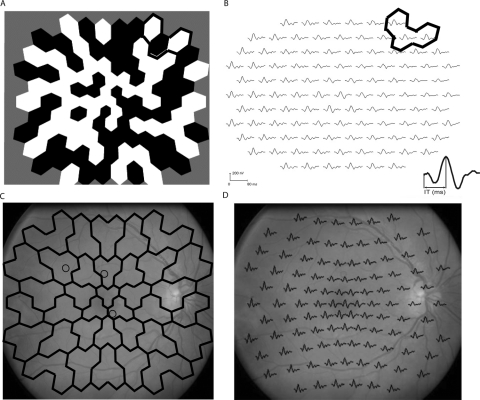
(A) mfERG array with a sample three-hexagon zone highlighted. (B) Array results in 103 mfERG traces, grouped by zone. A sample zone is again shown. The inset waveform shows the measurement of the P1 implicit time. (C) Zones were also overlaid over fundus photographs to determine which zones had retinopathy. Circles: the locations where the retinopathy has developed. (D) The same photograph of one of our type 1 patients with the mfERG trace array overlaid.
Statistical Analysis
Logistic regression29 was performed to examine associations between new retinopathy development and seven baseline risk factors (measured 1 year before the retinopathy outcome for subjects who developed retinopathy and 1 year before the last visit for those who remained retinopathy free): mfERG IT Z-score, diabetes duration, diabetes type, sex, blood glucose level, HbA1c, and age. Given that correlations might have existed between mfERG IT zones within the eye of any subject and across eyes of the same subject, generalized estimating equations (GEEs) were used to estimate model coefficients. With the GEE approach, estimates allow for covariance between zones in the same subject while assuming independence across subjects.30 Observations on both eyes of a single subject were combined into a single “cluster” to permit correlations across eyes. Robust variances were used for inference to accommodate any disparity between the assumed and true covariance structures.
For the logistic regression analysis, we first performed a univariate analysis of every risk factor, determining which factors were most likely to be predictive. Next, possible confounders of mfERG IT were identified and evaluated. Last, a final model was derived using a stepwise forward regression approach to determine whether other factors strengthened the predictive power of the model.
Probabilities of new retinopathy development in a zone were calculated from the final model and used to construct receiver operating characteristic (ROC) curves.31 A fivefold cross-validation procedure was used, randomly dividing the data (grouped by eye) into five subsets. Each subset is used to validate a model created by the other four sets of data together. The five validations were averaged to determine the generalized predictive accuracy of the model.32,33
Results
Retinopathy Development and Comparison of Type 1 and Type 2 Subjects
Retinopathy developed in 20 of the 41 subjects, 29 of the 78 eyes and 80 of the 2730 retinal zones (3%). In each subject, the retinopathy was mild and was either a microaneurysm or a dot hemorrhage. No subject had retinopathy in more than five zones in the same eye. Seventeen of the 80 zones (21%) that developed retinopathy were in the eight subjects with type 1 diabetes. When comparing type 1 and type 2 subjects, it was found that the type 1 subjects were younger and had longer durations of diabetes, faster mfERG implicit times, and better blood glucose control than did type 2 subjects (Table 1).
Model Creation
First we evaluated each potential risk factor in univariate models. The mfERG IT was found to be the most significant factor in the prediction of future retinopathy. Univariate analysis found that mfERG IT Z-score alone had an odds ratio of 1.16 (1.02–1.33). Diabetes duration was the only other factor that was significant in the univariate analyses. Duration of diabetes had an odds ratio of 1.07 (1.00–1.15; Table 2), which meant that mfERG IT Z-score alone and duration of diabetes alone were predictive of retinopathy. Odds ratios approximated relative risks; in other words, for every unit increase in mfERG IT Z-score, the risk for retinopathy onset increased by 16%, and for every 1-year increase in diabetes duration, the risk for onset of retinopathy increased by 7%. All the other factors were not significant in univariate analysis.
Table 2.
Significant Univariate Models for the Prediction of Retinopathy
Variable | Coefficient | P | OR (95% CI) |
---|---|---|---|
mfERG Implicit Time | 0.15 | 0.02 | 1.16 (1.02–1.33) |
Duration | 0.07 | 0.04 | 1.07 (1.00–1.15) |
All other factors were not significant.
Next, the potential confounding of mfERG IT by other risk factors was examined. A confounder is a factor that correlates with mfERG IT and with retinopathy development. If a confounder is discovered, it must be included in the model so that it is properly accounted for. Type of diabetes was found to be a significant confounder of mfERG IT because it changed the mfERG IT model coefficient by more then 10%; therefore, it had to be included in the model. The mfERG IT coefficient changed from 0.15 (P = 0.02; odds ratio [OR], 1.16; 95% confidence interval [CI], 1.02–1.33) when type was not accounted for in the model (Table 2) to 0.18 (P = 0.012; OR, 1.20; 95% CI, 1.04–1.53) when it is included. The more general model is log(p/1 − p) = −3.8 + 0.18 (IT Z-score) + 0.42 (Diabetes Type), where p denotes the probability of a given zone developing retinopathy 1 year after the measurements and diabetes type is a binary factor with 0 for type 2 diabetes and 1 for type 1 diabetes. Finally, other factors, including diabetes duration and blood glucose level, were evaluated to determine whether they improved the overall model fit, but none reached significance at a 0.05 level. Thus, the parsimonious model was the ultimate choice.
The coefficient for mfERG IT in the multivariate model yields an odds ratio of 1.20 (95% CI, 1.04–1.53), which can again be interpreted as an approximate relative risk; in other words, for every unit increase in implicit time Z-score, there is a 20% increase in risk for retinopathy within 1 year.
Cross-Validation
Fivefold cross-validation was used to estimate the sensitivity and specificity of the selected model because specificity and sensitivity estimates using the full data set are, by necessity, overly optimistic, justifying the need for cross-validation. This validation yielded the five sets of coefficients (Table 3) whose average was 0.17 for implicit time and 0.41 for diabetes type, similar to that of the coefficients in the final model. Each of these five models yielded an ROC curve that had a range of sensitivities from 82% to 73% and a range of specificities from 80% to 69% (Fig. 2). The average accuracy of these ROC curves indicated that the final model has a validated sensitivity of 80% ± 4% and a specificity of 74% ± 4%.
Table 3.
Fivefold Cross-Validation Coefficients, Odds Ratios, and ROC Curve Parameters
Model | IT Z-Score Coefficient | Type Coefficient | OR IT | OR Type | Sensitivity (%) | Specificity (%) |
---|---|---|---|---|---|---|
1 | 0.18 | 0.39 | 1.20 | 1.48 | 82 | 72 |
2 | 0.18 | 0.41 | 1.19 | 1.50 | 82 | 80 |
3 | 0.17 | 0.31 | 1.18 | 1.36 | 79 | 69 |
4 | 0.18 | 0.48 | 1.20 | 1.62 | 81 | 74 |
5 | 0.15 | 0.44 | 1.16 | 1.55 | 73 | 75 |
Average | 0.17 | 0.41 | 1.19 | 1.50 | 80 | 74 |
All data | 0.18 | 0.42 | 1.20 | 1.38 | 87 | 82 |
Bold data indicate averages of the five curves and give the overall parameters for the model.
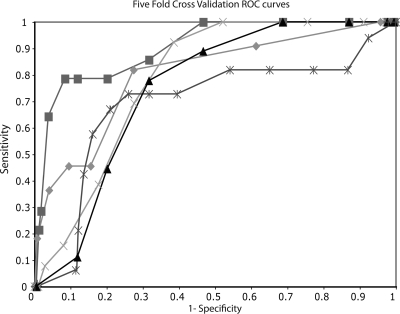
Receiver operating characteristic curves based on the fivefold cross-validation subsets of the data. Each line (symbol) is a curve constructed from one-fifth of the data using coefficients from the other four-fifths. The average of the five curves yields a sensitivity of 80% and a specificity of 74%.
Discussion
In this study, we created a multivariate model for the prediction of retinopathy onset in adult patients with diabetes. The main predictive risk factor in this model is a local retinal neural measure, implicit time of the mfERG. The mfERG IT has been shown in past studies to be delayed in patients with diabetes. Although the exact mechanism causing the delays in diabetes remains unknown, hypoxia, local blood flow changes, or changes in local metabolism may be responsible for the effects observed.22 In our model, a 1-unit Z-score increase in mfERG IT increases the risk by 20% for the onset of retinopathy in a retinal zone in these patients. Furthermore, we found that the power of the mfERG IT for predicting the development of retinopathy is different for adult patients with type 1 and type 2 diabetes; patients with type 1 diabetes display a greater risk for the onset of retinopathy, with smaller comparative delays in mfERG IT than the type 2 group. Consequently, type of diabetes must be adjusted for in these predictions and included in the predictive model.
Previously, our group developed models to locally predict new retinopathy development in patients with and without baseline retinopathy over a 1- to 3-year follow-up period. We found several factors that are predictive of new retinopathy in these patients, including mfERG IT Z-score, diabetes duration, blood glucose, and the presence of DR at baseline. In our previous 1-year model,24 the strongest factor predicting new retinopathy in a retinal zone was previous retinopathy in the eye. (This is perhaps not surprising because it is clinically accepted that an eye with some retinopathy is at a higher risk for more pathology.) Our previous models did not have enough patients without baseline retinopathy who went on to develop retinopathy in the follow-up period to make predictions about the onset of retinopathy in those eyes. Consequently, in this study, we investigated a group of patients with no retinopathy at baseline over a longer period. This model is a critical step for predicting the first funduscopically obvious change in the retina at the typical interval between diabetic eye examinations. The clinical onset of DR (vasculopathy in the retina) signifies an important progression in the microvascular complications in diabetes, which may also be occurring in other organ systems. This progression can be an indicator for the physician and patient to consider more aggressive management, including shorter patient follow-up intervals.
Successful fitting of this model required a sufficient number of patients who developed retinopathy during the testing period and used a much larger sample size than previous predictive modeling studies. This is because the conversion rate to early retinopathy is low and retinopathy is scarce in the retinal tissue. Even with retinopathy developing in half the patients, the local retinopathy development that was observed was relatively low overall (3%). Complicating the analysis and interpretation is that even though it is known that the development of a microaneurysm is a process over time,34 50% of visible microaneurysms are transient.15
A 1-year follow-up interval was used in this study to closely comply with standard clinical care follow-up guidelines of diabetic patients with no DR or mild DR. The cross-validated sensitivity (80%) and specificity (74%) of this model are only slightly less than the 86% and 82% found in the earlier validated 1-year model using patients with baseline retinopathy and more severe retinopathy, even though the retinopathy observed in the present study was more scarce and more difficult to detect.23 Clearly the mfERG IT, when properly adjusted for diabetes type, is a very sensitive test for predicting even the earliest clinical retinal changes over a 1-year window.
Associations between retinopathy development factors (such as duration of diabetes, blood glucose levels, type of diabetes, and blood glucose control) that were significant in previous retinopathy progression models from our group and other studies looking at retinopathy progression10,23,25,35 did not significantly contribute to the multivariate model for predicting retinopathy onset in conjunction with mfERG IT in patients who were retinopathy free. In our previous 3-year model,25 diabetes type was found to be a possible predictive factor; however, the small sample size of type 1 patients led to a large confidence interval for the odds ratio of that prediction. Because of the imprecision surrounding this factor, we did not include it in the previous 3-year model. In the present model, diabetes type was not found to be directly predictive of retinopathy but instead confounded the ability of the mfERG IT to predict the retinopathy. On average, the type 1 patients developed more retinopathy, but they also tended to have faster average implicit times than the type 2 patients. The differences between the two groups are accounted for by including diabetes type in this model, but additional studies on the early differences in neural function between the types of diabetes are needed. Overall differences between the present model and previous models likely stem not only from the difference in the retinopathy itself but also from the fact that this population of patients, who are experiencing the onset of retinopathy, is very different from the population of patients who already have retinopathy. Type 1 adult patients who have yet to develop retinopathy, for example, tended to have longer durations, younger ages, and better blood glucose control (several were using insulin pumps, which have been shown to improve HbA1c percentage levels36) than type 2 patients in this same group. But these differences between type 1 and type 2 would not necessarily be observed in patients who already have retinopathy.
Given that the average age of type 1 patients at the time of diagnosis is younger and more definitive than in type 2 patients, the longer disease durations and younger patient ages we saw in our study of adult patients with type 1 diabetes were to be expected. Most type 1 patients have some retinopathy within 25 years of diagnosis.37,38 Similarly, progression to proliferative diabetic retinopathy was shown to be common (42%) in a study of type 1 patients over a 25-year period.39 On the other hand, perhaps because disease onset is more ambiguous in type 2 diabetes, these patients appear to experience more range in disease progression. Many patients (35%–45%) with type 2 diabetes have retinopathy at the time of their diabetes diagnosis40 and, hence, would not have been eligible for this study. As many as 60% of patients who have type 2 diabetes for more than 20 years have retinopathy, and 58% in the 11- to 20-year duration range also have retinopathy.41 However, these figures include the patients with retinopathy at diagnosis, who had been eliminated from our study. Our study represents a different, and likely healthier, group of type 2 patients than are typically presented in the epidemiologic data, which might in part have accounted for why the type 2 group had a lower risk for first retinopathy in the present study.
In summary, our new model for predicting the onset of retinopathy in eyes with no previous retinopathy reveals that the mfERG IT is a useful tool for predicting retinopathy onset. It is objective, measures retinal function in approximately 8 minutes, and is reproducible.28 The model could be used to identify and enroll patients at higher risk in clinical trials or tests of newer medications aimed at delaying or preventing the earliest retinopathy well before visual acuity is affected. Our results also suggest that the mfERG IT Z-score measurement, when corrected for type of diabetes, could possibly act as a surrogate end point for studies in which the preventive treatment of retinopathy is a primary goal.42,43
Acknowledgments
The authors thank Kevin Bronson-Castain, Brian Wolff, Kavita Dhamdhere, Glen Ozawa, Oscar Davila, and Maria Cardenas for assistance with data collection; Ann Chang for assistance with data analysis; and Andrew Mick, Bernard Dolan, and Vivian Mata for assistance in patient recruitment.
Footnotes
Supported National Institutes of Health Grant EY 02271 (AJA).
Disclosure: W.W. Harrison, None; M.A. Bearse, Jr, None; J.S. Ng, None; N.P. Jewell, None; S. Barez, None; D. Burger, None; M.E. Schneck, None; A.J. Adams, None
References
Articles from Investigative Ophthalmology & Visual Science are provided here courtesy of Association for Research in Vision and Ophthalmology
Full text links
Read article at publisher's site: https://doi.org/10.1167/iovs.10-5931
Read article for free, from open access legal sources, via Unpaywall:
https://europepmc.org/articles/pmc3053106?pdf=render
Free after 12 months at intl.iovs.org
http://intl.iovs.org/cgi/content/full/52/2/772
Free after 12 months at intl.iovs.org
http://intl.iovs.org/cgi/reprint/52/2/772.pdf
Free to read at intl.iovs.org
http://intl.iovs.org/cgi/content/abstract/52/2/772
Citations & impact
Impact metrics
Article citations
Advances in Structural and Functional Retinal Imaging and Biomarkers for Early Detection of Diabetic Retinopathy.
Biomedicines, 12(7):1405, 25 Jun 2024
Cited by: 0 articles | PMID: 39061979 | PMCID: PMC11274328
Review Free full text in Europe PMC
Abnormalities of retinal function in type 2 diabetes mellitus patients without clinical diabetic retinopathy detected by multifocal electroretinogram.
BMC Ophthalmol, 24(1):71, 15 Feb 2024
Cited by: 2 articles | PMID: 38360630 | PMCID: PMC10870650
Evaluation of retinal structure and function in prediabetes.
Diabet Epidemiol Manag, 12:100154, 11 Jun 2023
Cited by: 1 article | PMID: 38037572 | PMCID: PMC10688557
Objective perimetry identifies regional functional progression and recovery in mild Diabetic Macular Oedema.
PLoS One, 18(6):e0287319, 15 Jun 2023
Cited by: 4 articles | PMID: 37319294 | PMCID: PMC10270604
Visual Dysfunction in Diabetes.
Annu Rev Vis Sci, 9:91-109, 10 May 2023
Cited by: 1 article | PMID: 37164027 | PMCID: PMC11262130
Review Free full text in Europe PMC
Go to all (120) article citations
Similar Articles
To arrive at the top five similar articles we use a word-weighted algorithm to compare words from the Title and Abstract of each citation.
Prediction, by retinal location, of the onset of diabetic edema in patients with nonproliferative diabetic retinopathy.
Invest Ophthalmol Vis Sci, 52(9):6825-6831, 29 Aug 2011
Cited by: 20 articles | PMID: 21743017 | PMCID: PMC3176012
Local diabetic retinopathy prediction by multifocal ERG delays over 3 years.
Invest Ophthalmol Vis Sci, 49(4):1622-1628, 01 Apr 2008
Cited by: 93 articles | PMID: 18385083
Formulation and evaluation of a predictive model to identify the sites of future diabetic retinopathy.
Invest Ophthalmol Vis Sci, 45(11):4106-4112, 01 Nov 2004
Cited by: 49 articles | PMID: 15505062
A multifocal electroretinogram model predicting the development of diabetic retinopathy.
Prog Retin Eye Res, 25(5):425-448, 01 Sep 2006
Cited by: 144 articles | PMID: 16949855 | PMCID: PMC2773701
Review Free full text in Europe PMC
Funding
Funders who supported this work.
NEI NIH HHS (2)
Grant ID: EY 02271
Grant ID: R01 EY002271