Abstract
Free full text

The TAGteam motif facilitates binding of 21 sequence-specific transcription factors in the Drosophila embryo
Abstract
Highly overlapping patterns of genome-wide binding of many distinct transcription factors have been observed in worms, insects, and mammals, but the origins and consequences of this overlapping binding remain unclear. While analyzing chromatin immunoprecipitation data sets from 21 sequence-specific transcription factors active in the Drosophila embryo, we found that binding of all factors exhibits a dose-dependent relationship with “TAGteam” sequence motifs bound by the zinc finger protein Vielfaltig, also known as Zelda, a recently discovered activator of the zygotic genome. TAGteam motifs are present and well conserved in highly bound regions, and are associated with transcription factor binding even in the absence of canonical recognition motifs for these factors. Furthermore, levels of binding in promoters and enhancers of zygotically transcribed genes are correlated with RNA polymerase II occupancy and gene expression levels. Our results suggest that Vielfaltig acts as a master regulator of early development by facilitating the genome-wide establishment of overlapping patterns of binding of diverse transcription factors that drive global gene expression.
Genome-wide chromatin immunoprecipitation (ChIP) experiments in diverse metazoans have shown that many transcription factors bind thousands of highly overlapping loci in undifferentiated cells (Boyer et al. 2005; Moorman et al. 2006; Zeitlinger et al. 2007; Chen et al. 2008; Li et al. 2008; Marson et al. 2008; Bradley et al. 2010; Gerstein et al. 2010; Roy et al. 2010; He et al. 2011; Negre et al. 2011). Many known enhancers of gene expression are bound by multiple transcription factors, suggesting that highly overlapping transcription factor binding may imply cis-regulatory potential. Supporting this hypothesis, clusters of transcription factor binding sites have been used to identify novel enhancers (Berman et al. 2002; Markstein et al. 2002), a method that is particularly powerful when combined with sequence conservation (Kazemian et al. 2010), and which has proved useful even in the absence of experimentally determined transcription factor binding specificities (Kazemian et al. 2011). Many, although not all, regions bound in vivo by multiple transcription factors confer enhancer activity and drive gene expression (Chen et al. 2008; Ouyang et al. 2009; Zinzen et al. 2009; Negre et al. 2011). However, the mechanisms underlying these overlapping patterns of binding remain incompletely understood.
We studied the origins and consequences of overlapping binding using Drosophila melanogaster embryonic development as a model system. ChIP data from whole embryos is available for approximately half of the sequence-specific transcription factors responsible for embryonic segmentation, which bind to highly overlapping regions of the genome, as well as for RNA polymerase II (MacArthur et al. 2009). DNase I digestion data, measuring chromatin accessibility, is available as well for identically staged embryos (Li et al. 2011; Thomas et al. 2011). Furthermore, a recent study measured levels of both maternally contributed and zygotically transcribed mRNAs from mitotic cycles 10–14 (Lott et al. 2011) when widespread transcription of the zygotic genome commences, allowing us to connect transcription factor binding to gene expression.
So-called “TAGteam” sequence motifs, which are enriched in the promoters of early expressed genes and known to be important for early embryonic expression (ten Bosch et al. 2006), are bound by the zinc finger protein Vielfaltig, also known as Zelda, a key regulator of the maternal-to-zygotic transition (Liang et al. 2008). While Vielfaltig's mechanism of action is unknown, we and others previously noted an enrichment for TAGteam motifs in regions bound by six anterior–posterior transcription factors (Li et al. 2008; Bradley et al. 2010). Hypothesizing that this relationship between TAGteam motifs and transcription factor binding might be causative, we reanalyzed the ChIP (MacArthur et al. 2009), DNase I (Thomas et al. 2011), and RNA-seq (Lott et al. 2011) data sets described above and found that the presence of TAGteam motifs is associated with high levels of binding of all assayed factors. Levels of binding are globally proportional to RNA polymerase II occupancy of promoters and gene expression levels during the onset of zygotic transcription. Taken together, our results suggest that Vielfaltig facilitates the binding of diverse transcription factors, thereby mediating global activation of the zygotic genome.
Results
Overlapping patterns of transcription factor binding are correlated with TAGteam motifs
Examining ChIP-chip data for 21 sequence-specific transcription factors at select loci reveals high overlap in their binding profiles, as noted previously (Fig. 1; MacArthur et al. 2009). The ChIP data, created from whole embryos, reflects a spatial averaging over distinct nuclei, each with different subsets or concentrations of transcription factors. The high overlap shown in Figure 1 therefore reflects the similar (spatially averaged) genomic binding locations of many transcription factors—even for factors that are not co-expressed—rather than simultaneous binding of all 21 factors in a single nucleus.
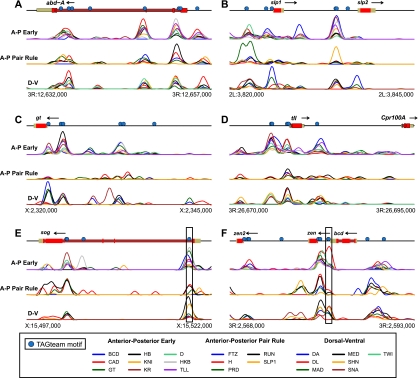
Regions bound by many transcription factors frequently contain TAGteam motifs. ChIP-chip data for 21 transcription factors (MacArthur et al. 2009) is plotted across 25 kb near the genes (A) abdominal A (abd-A), (B) sloppy paired 1 (slp1) and sloppy paired 2 (slp2), (C) giant (gt), (D) tailless (tll), (E) short gastrulation (sog), and (F) bicoid (bcd), zerknullt (zen) and zerknullt-related (zen2). (Blue circles) TAGteam motifs CAGGTAG, tAGGTAG, and CAGGTAa; analyses in all figures use only these three TAGteam motifs and their reverse complements. Boxed TAGteam motifs have been previously demonstrated to be required for early expression (ten Bosch et al. 2006; Liberman and Stathopoulos 2009). ChIP data was smoothed as described in Methods. Vertical axes differ between panels for clarity. Gene models are from FlyBase release 5.12 (Tweedie et al. 2009).
After normalizing the ChIP data for each factor to control for differing antibody affinities and other sources of experimental variation, we assessed the degree of binding overlap using an unbiased principal components analysis (PCA) approach. This analysis treats ChIP data quantitatively, giving more weight to genomic regions with high ChIP signals, in order to identify correlations between the binding patterns of all, rather than pairs of, factors. (Note that DNA-binding affinity, on/off rates, and spatial differences within the embryo all contribute to the measured ChIP signal.) Examining 400-bp windows centered on ChIP peak summits within previously identified bound regions (Li et al. 2008), we confirmed that the primary mode of variation in the binding data was overlapping binding, with binding of all 21 factors accounting for 52% of the total genome-wide variation (Supplemental Fig. 1). Strikingly, all of the highly bound regions illustrated in Figure 1 contain motifs similar to CAGGTAG, the consensus TAGteam motif bound by Vielfaltig, of which the motifs near the transcription start sites (TSSs) of short gastrulation (sog) and zerknullt (zen) (Fig. 1E,F, boxed) are known to be required for early expression (ten Bosch et al. 2006; Liberman and Stathopoulos 2009). This anecdotal relationship between overlapping patterns of binding and TAGteam motifs holds genome-wide. We used the first principal component of our PCA analysis, corresponding to a weighted average over all 21 transcription factors, as a quantitative measurement of levels of overlapping binding. Fewer than 15% of regions with low levels of overlapping binding contain one of the three TAGteam motifs CAGGTAG, tAGGTAG, and CAGGTAa (lowercase indicates mismatches to the consensus CAGGTAG) within 500 bp of ChIP peak summits, whereas 70% of highly bound regions do (Fig. 2A). Furthermore, binding at ChIP peaks is correlated with the number of nearby TAGteam motifs (Fig. 2B), suggesting a dose-dependent relationship between TAGteam motifs and binding of many transcription factors.
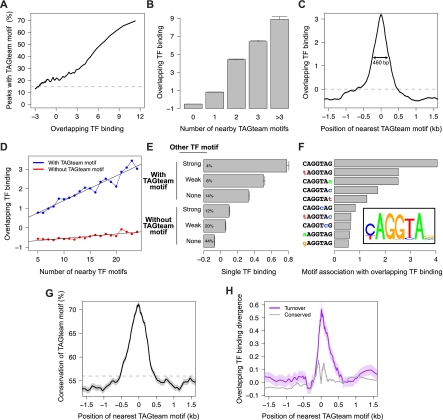
TAGteam motifs are associated with nearby binding of many transcription factors. (A) The fraction of ChIP peaks with nearby TAGteam motifs increases with levels of binding of all factors. “Overlapping TF binding” indicates levels of binding of all factors in arbitrary units (measured as the first principal component of our PCA analysis; see text), where 0 is the mean over ChIP peaks. (Dashed gray line) Genome-wide average frequency. For A,C,D,G, and H, shading around plotted lines indicates standard error of the mean (too small to be visible in some plots). (B) Binding at ChIP peaks increases with the number of nearby TAGteam motifs (within 500 bp). (Error bars) Standard error of the mean. (C) ChIP peaks with nearby TAGteam motifs exhibit high levels of binding. Plot indicates the position of the TAGteam motif closest to each ChIP peak (x-axis) and the corresponding binding level at the ChIP peak (y-axis). (Double-headed arrow) Distance at which the association between TAGteam motifs and binding falls by 50%. (Dashed gray line) Average level of binding (averaged over all ChIP peaks). (D) Number of nearby transcription factor binding sites globally correlates with levels of binding only when TAGteam motifs are present. Restricting to the 14 factors with well-defined binding sites, we computed the number of binding sites as the total number of PWM matches within 500 bp of each ChIP peak summit, and we searched for TAGteam motifs within the same region. (E) TAGteam motifs may facilitate binding of additional transcription factors in concert with cognate recognition motifs for these factors. Matches to transcription factor binding site PWMs within 100 bp of ChIP peak summits were classified as “strong” (P < 10−5), “weak” (10−5 < P < 10−4), or absent. Percentages indicate the fraction of ChIP peaks that fall into each category. “Single TF binding” indicates levels of binding of single transcription factors, averaged over all 14 factors with well-defined binding sites, in arbitrary units, where 0 is the mean over ChIP peaks. (Error bars) Standard error of the mean. (F) CAGGTAG, tAGGTAG, and CAGGTAa are the TAGteam motifs most highly associated with binding of all factors. We ranked each 2-bp polymorphism of the consensus TAGteam motif by its association with overlapping binding, measured as the peak height illustrated in C, but restricted to a single heptamer. (Inset) PWM determined from this ranking of heptamer effectiveness, plotted with seqLogo (http://bioconductor.org/packages/2.6/bioc/html/seqLogo.html). (G) TAGteam motifs near ChIP peaks exhibit increased conservation. Plot indicates the position of the TAGteam motif closest to each ChIP peak (x-axis) and the corresponding motif conservation between D. melanogaster and D. yakuba (y-axis). (Dashed gray line) Genome-wide average conservation of TAGteam motifs. (H) Gain/loss of TAGteam motifs is associated with divergence in nearby binding between D. melanogaster and D. yakuba. Plot indicates the position of the TAGteam motif closest to each ChIP peak in one species (x-axis) and the corresponding divergence in overlapping binding at the ChIP peak in the other species (y-axis), averaged over all ChIP peaks detected in either species.
While only 50% of TAGteam motifs in regions with low levels of overlapping binding are conserved to D. yakuba, a sister species of D. melanogaster, 90% of TAGteam motifs in regions with high levels of overlapping binding are conserved (Supplemental Fig. 2). High conservation of TAGteam motifs is probably due to selective pressure to specifically preserve TAGteam motifs themselves rather than similarities between TAGteam motifs and binding sites for the transcription factors studied. Restricting our analyses to the set of 14 transcription factors with well-defined binding sites that were enriched in the ChIP data (see Methods), we found no strong (P < 10−5) position weight matrix (PWM) match against NNNCAGGTAGNNN for any of the 14 factors, suggesting that high overlap between TAGteam motifs and other transcription factor binding sites is unlikely. Consistent with this, the selective enrichment of conserved TAGteam motifs in regions bound by many transcription factors (Supplemental Fig. 2) is much stronger than we observed previously for conservation of non-TAGteam motifs (Bradley et al. 2010).
TAGteam motifs were associated with high levels of binding of all factors when located up to ~230 bp away from ChIP peak centers, with the association disappearing for motifs further than 500 bp away (Fig. 2C). To confirm this estimate with the higher-resolution ChIP-seq assay, we analyzed the six transcription factors (Bicoid, Caudal, Giant, Hunchback, Knirps, and Kruppel) for which both ChIP-chip (MacArthur et al. 2009) and ChIP-seq (Bradley et al. 2010) data were available, estimating that the two assays have resolutions of ~100 bp (ChIP-chip) and ~20 bp (ChIP-seq) (Supplemental Fig. 3A,B). Computing the first principal component of the ChIP-seq data as a measure of overlapping binding of these six factors, we obtained a very similar estimate of the spatial range of association between TAGteam motifs and factor binding (Supplemental Fig. 3C,D). Furthermore, restricting to the same six transcription factors for which ChIP data was available in D. yakuba (Bradley et al. 2010), we found that TAGteam motifs were associated with high levels of binding of all six factors in this species as well, again with a spatially restricted relationship (Supplemental Fig. 4). This data supports a model where TAGteam motifs proximal, although not necessarily immediately adjacent, to other transcription factor binding sites directly or indirectly facilitate binding of these factors.
Canonical TAGteam motifs have a uniquely strong association with regions bound by many factors
We conducted an ab initio motif search to look for other sequence motifs potentially associated with high levels of overlapping binding by enumerating all possible heptamers and ranking each according to the level of binding of all factors associated with the heptamer's presence (Supplemental Fig. 5). The top 16 heptamers are all TAGteam motif variants, and the consensus TAGteam motif CAGGTAG was associated with approximately threefold higher levels of overlapping binding than was the highest-ranked motif not associated with Vielfaltig (see Discussion).
A recent analysis of the Drosophila even skipped stripe 3+7 enhancer demonstrated that Vielfaltig binds to several noncanonical TAGteam sites (CAGGcAa, gAGGTAt, CcGGTAc, CtGGTtt) (Struffi et al. 2011). However, our heptamer analysis revealed no global enrichment (in the sense of Fig. 2A) for any of these four motifs in highly versus weakly bound regions. While such noncanonical sites probably play important roles in select contexts, they lack the clear genome-wide association with overlapping binding observed for the core TAGteam motifs.
TAGteam motifs demarcate highly bound clusters of transcription factor binding sites
Many studies have noted the difficulty of accurately predicting transcription factor binding from binding site models alone, with few differences in the number or strength of binding sites between regions that are weakly or strongly bound (Kaplan et al. 2011; Pique-Regi et al. 2011). For each of the 14 factors with well-defined binding sites, we computed the total number of recognition motifs for these factors within 500 bp of each ChIP peak summit as a measure of its naïve binding potential. Regions lacking TAGteam motifs exhibited little global correlation between the number of nearby binding sites and levels of binding, which was not surprising given the known difficulty of predicting binding from sequence alone. However, if a TAGteam motif was present, then binding was globally proportional to our binding potential estimate (Fig. 2D). This relationship, where the number of nearby binding sites correlated with binding only if a TAGteam motif was present nearby, persisted in both D. melanogaster and D. yakuba after restricting to the five anterior–posterior factors with well-defined binding sites for which ChIP data was available in both species (Supplemental Fig. 6). These results suggest that the presence of proximal TAGteam sites may be important for efficient interactions between other transcription factors and genomic DNA.
Considering each of the 21 factors separately, we found that nearby TAGteam motifs were associated with high levels of binding of each factor (Supplemental Fig. 7). This effect was primarily due to the association between TAGteam motifs and binding of all, rather than single, factors; after controlling for levels of binding of all factors, regions with strong binding of single factors were no more likely to contain TAGteam motifs than were regions with weak binding (Supplemental Fig. 8).
For each of the 14 factors with well-defined binding sites, we found the highest levels of single-factor binding when both a TAGteam motif and a binding site for the ChIP'ed factor were present. TAGteam motifs proximal to ChIP peaks correlated with strong binding of individual transcription factors even in the absence of canonical binding sites for the ChIP'ed factors (Fig. 2E). While nonspecific interactions between Vielfaltig and the antibodies used for ChIP could also give rise to this correlation, such an explanation seems unlikely given the universality of the effect for all transcription factors studied here.
Vielfaltig, not Grainy head, probably facilitates TAGteam-associated binding
A second CAGGTAG-binding transcription factor, Grainy head, was recently shown to compete effectively with Vielfaltig at equal molarity for binding to TAGteam motifs (Harrison et al. 2010). Overexpression of maternal Grainy head phenocopies Vielfaltig depletion, supporting the hypothesis that Grainy head represses the transcriptional activation mediated by Vielfaltig. In concert with our results, this repression suggests a model where Vielfaltig, not Grainy head, binding at TAGteam sites facilitates binding of additional transcription factors, resulting in zygotic genome activation. The distinct motif preferences of Vielfaltig and Grainy head also support this model. Restricting our ranking of heptamer association with binding of all factors (Supplemental Fig. 5) to all 2-bp polymorphisms of CAGGTAG, we computed a PWM for TAGteam motifs (Fig. 2F). While both Vielfaltig and Grainy head bind CAGGTAG strongly, only Vielfaltig is a high-affinity binder of tAGGTAG, which we identify as the second-ranked heptamer; similarly, the relatively low-ranked heptamer CAGGcAG is strongly bound by Grainy head, but not by Vielfaltig (Harrison et al. 2010). Therefore, our ranking of heptamer association with binding of all factors is concordant with Vielfaltig, but not Grainy head, binding preferences.
Turnover of TAGteam motifs is associated with interspecies binding divergence
The dose-dependent correlation between the number of nearby TAGteam motifs and binding of all factors (Fig. 2B) implies that cis-regulatory potential may be very sensitive to the gain or loss of TAGteam motifs. Transgenic expression experiments support this, with addition of motifs leading to earlier expression (ten Bosch et al. 2006) and loss of motifs reducing or abolishing early expression (ten Bosch et al. 2006; Liberman and Stathopoulos 2009). Notably, TAGteam motifs are most conserved when proximal to ChIP peaks (Fig. 2G).
Using an ab initio motif discovery approach, we previously noted that turnover (gain/loss) of CAGGTAG was associated with changes in binding of six anterior–posterior transcription factors between D. melanogaster and D. yakuba (Bradley et al. 2010). Quantifying this previous observation, we found that turnover of TAGteam motifs proximal to ChIP peaks is associated with changes in nearby binding of all six factors between species (Fig. 2H). This binding divergence is not explained by differences in the number of nearby binding sites for additional transcription factors (data not shown), suggesting that turnover of TAGteam motifs plays a significant role in the observed changes in binding of all six factors.
TAGteam motifs are associated with binding of all factors in different chromatin contexts
Several recent reports noting the widespread overlap in binding of distinct transcription factors in the Drosophila embryo have found that chromatin accessibility plays an important role in determining patterns of transcription factor binding (Kaplan et al. 2011; Li et al. 2011). Using published DNase I digestion data from whole embryos (Thomas et al. 2011) as a proxy for average chromatin accessibility across the many nuclei in an embryo, we noticed that while regions with high levels of binding frequently had very open chromatin, this was not always the case (Fig. 3A–F). For example, the TAGteam motifs near the TSSs of sog and zen both lie in regions with very high levels of overlapping binding, but relatively low chromatin accessibility (Fig. 3E,F, boxed). Quantifying this effect genome-wide, we found that chromatin accessibility correlated well with levels of binding, as expected. Nonetheless, TAGteam motifs were associated with increased binding of all factors even after controlling for chromatin accessibility, although not in the most inaccessible regions (Fig. 3G). This effect was unchanged after controlling for the number of nearby binding sites for other factors (data not shown). Furthermore, genomic regions with very accessible chromatin, but low levels of binding, contain few TAGteam motifs, whereas the majority of regions with moderate to high levels of binding, irrespective of chromatin accessibility, contain TAGteam motifs (Fig. 3H). At a global level, therefore, TAGteam sites are primarily associated with transcription factor binding rather than simply open chromatin, as measured by DNase I accessibility.
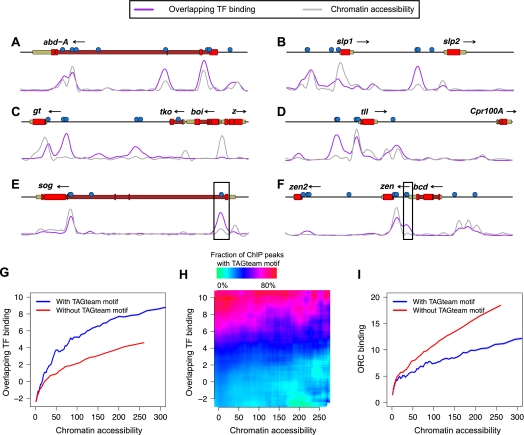
TAGteam motifs are associated with binding in different chromatin contexts. Levels of binding of all factors (purple) and DNase I digestion data (gray), both in arbitrary units, are plotted across 25 kb near the genes (A) abdominal A (abd-A), (B) sloppy paired 1 (slp1) and sloppy paired 2 (slp2), (C) giant (gt), (D) tailless (tll), (E) short gastrulation (sog), and (F) bicoid (bcd), zerknullt (zen), and zerknullt-related (zen2). Vertical axes differ between panels for clarity. Boxed TAGteam motifs have been previously demonstrated to be required for early expression (ten Bosch et al. 2006; Liberman and Stathopoulos 2009). ChIP and DNase I data were smoothed as described in Methods. (G) TAGteam motifs are associated with binding even after controlling for chromatin accessibility. Plot shows average overlapping binding at ChIP peaks with and without nearby (within 500 bp) TAGteam motifs after binning by DNase I signal. (H) TAGteam motifs are primarily associated with binding, not chromatin accessibility. Plot shows fraction of ChIP peaks with nearby TAGteam motifs, binned by both chromatin accessibility and levels of binding. (I) TAGteam motifs are depleted in regions with high ORC binding. Plot shows average ORC binding at ChIP peaks for the 21 transcription factors analyzed here with and without nearby (within 500 bp) TAGteam motifs after binning by DNase I signal.
Binding of the origin recognition complex is anticorrelated with TAGteam motifs
The Drosophila origin recognition complex (ORC) preferentially binds regions with open chromatin (MacAlpine et al. 2010), can interact with multiple transcription factors (Bosco et al. 2001; Beall et al. 2002; Dominguez-Sola et al. 2007), and reportedly localizes to regions with high levels of overlapping transcription factor binding (Roy et al. 2010). Depletion of zygotically transcribed Vielfaltig results in chromosome segregation and DNA replication defects (Staudt et al. 2006), leading us to hypothesize that TAGteam motifs might play a role in ORC positioning. Using published ORC ChIP-seq data from mixed-stage embryos (Roy et al. 2010), we confirmed previous reports (MacAlpine et al. 2010) of a strong correlation between chromatin accessibility and ORC binding. However, to our surprise, the presence of a TAGteam motif near ChIP peaks was associated with a lower level of ORC binding (Fig. 3I). Assessing the simultaneous effects of chromatin accessibility and transcription factor binding on ORC binding, we found that after controlling for chromatin accessibility, binding of all factors was weakly anticorrelated with ORC localization (Supplemental Fig. 9).
Binding of multiple transcription factors is associated with gene expression
Our results, suggesting that Vielfaltig binding to TAGteam sites may facilitate binding of diverse transcription factors, provide a potential explanation of how Vielfaltig could mediate global activation of the zygotic genome. However, this explanation is predicated upon the assumption that regions bound by many transcription factors generally possess enhancer activity. We assessed whether overlapping binding of many factors is globally associated with gene expression using a published RNA-seq time course of single D. melanogaster embryos from mitotic cycles 10–14 (Lott et al. 2011), when widespread zygotic transcription begins. Consistent with previous reports that TAGteam motifs are important for early expression (ten Bosch et al. 2006), we found that TAGteam motifs in gene promoters, defined as 1.5 kb centered on the TSS, were associated with strong increases in the expression level of zygotically transcribed genes from cycles 10–14 (Fig. 4A). Phosphorylated RNA polymerase II (Pol II) occupancy correlated with transcription factor binding in promoters (Fig. 4B), as did downstream gene expression (Fig. 4C), suggesting that binding of many transcription factors drives transcription by facilitating Pol II recruitment. In agreement with this model, Pol II occupancy correlated with gene expression levels (Supplemental Fig. 10A). Our results were unchanged when we measured Pol II occupancy of downstream coding sequences instead of promoters (Supplemental Fig. 10B,C). As with the analyzed transcription factor binding data, the RNA-seq and Pol II ChIP data were obtained from whole embryos, and therefore represent averages over many distinct nuclei.
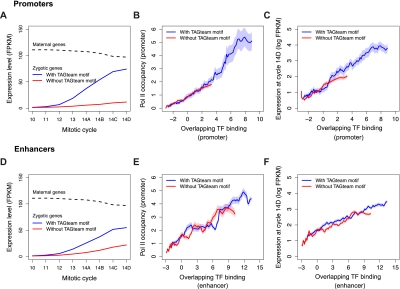
Binding of multiple factors in promoters and enhancers is correlated with Pol II occupancy of promoters and gene expression levels. (A) Zygotically transcribed genes with TAGteam motifs in their promoters (defined as the 1.5 kb centered on the TSS) have increasing expression from mitotic cycles 10–14. Maternally transcribed genes have slowly decreasing expression over the same time course (regardless of presence or absence of TAGteam motifs; data not shown). (FPKM) Fragments per kilobase per million mapped reads, a standard unit of gene expression measured by RNA-seq. (B) Pol II occupancy of promoters is correlated with binding in promoters. (C) Gene expression levels are correlated with binding in promoters. (D) Putative enhancers, defined as ChIP-bound regions 1.5–5 kb upstream of the TSS, with nearby TAGteam motifs (within 500 bp) are associated with increasing expression of downstream genes from cycles 10–14. (E) Pol II occupancy of promoters of downstream genes is correlated with binding in putative enhancers. (F) Gene expression levels are correlated with binding in upstream enhancers.
TAGteam motifs in putative cis-acting enhancers—not just promoters—were associated with high levels of gene expression as well. Defining ChIP peaks 1.5–5 kb upstream of TSSs as putative enhancers, we found that the presence of TAGteam motifs in enhancers was associated with strong increases in gene expression from cycles 10–14 (Fig. 4D). Binding in these cis-acting enhancers was proportional to Pol II occupancy of downstream promoters (Fig 4E) and coding sequences (Supplemental Fig. 10D), as well as downstream gene expression (Fig. 4F). These trends were weaker for enhancers than for promoters, probably due to uncertainty in our assignment of enhancers to their target genes.
We observed relatively small differences in Pol II occupancy (Fig. 4B,E) and gene expression levels (Fig. 4C,F) for regions with and without TAGteam motifs after controlling for levels of binding of all factors. This is compatible with a model in which Vielfaltig binding, whether in promoters or enhancers, primarily facilitates the binding of additional transcriptional regulators rather than directly affecting polymerase recruitment or gene expression.
Previous reports, noting that known Drosophila enhancers tend to have very high levels of transcription factor binding, have suggested that regions with low levels of binding may be nonfunctional and incapable of driving gene expression (Li et al. 2008; MacArthur et al. 2009). However, this model is challenging to reconcile with our recent observation that strongly and weakly bound regions have similar levels of relative binding divergence between D. melanogaster and D. yakuba, potentially due to similar functional constraint (Bradley et al. 2010). The observed association between low levels of overlapping binding and weak, but nonzero, gene expression (Fig. 4C,F) suggests that weakly bound regions may play subtle functional roles, such as driving gene expression in a small subset of nuclei. The similar levels of relative binding divergence between D. melanogaster and D. yakuba observed for strongly and weakly bound regions could therefore be explained by selective pressure to preserve both strong and weak enhancer activity, or alternately by a “ramping up” model of weakly bound regions, wherein they will become strongly bound and drive higher levels of gene expression at later time points.
Discussion
Our results suggest that binding of Vielfaltig to TAGteam sites facilitates or stabilizes the nearby binding of many of the transcriptional regulators active in the early Drosophila embryo, thereby providing a potential mechanism for Vielfaltig's activation of the zygotic genome. Most transcription factor binding site motifs have limited predictive value in the absence of external information such as chromatin accessibility (Kaplan et al. 2011; Li et al. 2011; Pique-Regi et al. 2011), but we observed a clear association between TAGteam motifs and binding of many transcription factors without Vielfaltig ChIP data. Taken together with our finding that the number of nearby transcription factor binding sites globally correlates with levels of binding only if a TAGteam motif is present (Fig. 2D), this suggests that TAGteam motifs may distinguish functional cis-regulatory modules (CRMs) from binding site clusters with little activity in the early embryo.
In order to assess the extent to which Vielfaltig binding near clusters of motifs for other factors may be sufficient to facilitate binding of other transcription factors, we enumerated all promoters of coding genes with clusters of transcription factor binding sites (greater than or equal to eight motifs). The fraction of such promoters with high levels of overlapping binding (≥95th percentile of promoters) exhibited a dose-dependent relationship with the number of nearby TAGteam sites (Supplemental Fig. 11A); 7% of promoters with one nearby TAGteam motif had high levels of overlapping binding, whereas 85% of promoters with more than three nearby TAGteam motifs did. Requiring that promoters have at least one TAGteam site conserved in D. yakuba within 250 bp of the TSS resulted in a still-higher fraction of promoters with high levels of binding (Supplemental Fig. 11B). These results suggest that the presence of nearby TAGteam motifs is frequently, although not always, sufficient to facilitate binding of many transcription factors.
Locally compact chromatin structure may help to explain the existence of regions with TAGteam motifs, but little binding. Promoters with low levels of binding (≤50th percentile of promoters) despite the presence of nearby TAGteam motifs had less accessible chromatin (~10-fold) than did promoters with high levels of binding (≥95th percentile). These results are compatible with recent findings that many Ciona intestinalis and Drosophila CRMs contain sequence signatures, independent of transcription factor binding sites, predicted to promote nucleosome depletion (Khoueiry et al. 2010).
Our ranking of heptamer association with binding (Supplemental Fig. 5) demonstrated the uniqueness of TAGteam sites, but revealed non-TAGteam motifs associated with overlapping patterns of binding as well. Of the 30 highest-ranked heptamers associated with overlapping binding, all of the non-TAGteam motifs contained GA or GAG repeats, suggesting a potential association between binding of many factors and GAGA factor, which is encoded by the gene Trithorax-like and implicated in chromatin remodeling (Granok et al. 1995; Wall et al. 1995; Okada and Hirose 1998). Intriguingly, we observed a 1.5-fold enrichment for GAGA motifs in promoters with high levels of binding when TAGteam motifs were not present (39% of highly bound promoters without TAGteam sites contained GAGA motifs versus 25% with TAGteam sites), suggesting that GAGA factor may help to facilitate binding of transcription factors at some regions not associated with Vielfaltig.
We propose that Vielfaltig facilitates the binding of additional transcription factors to nearby low-affinity sites, although the mechanisms by which it may do so remain unclear. Vielfaltig has six C2H2 zinc fingers, two N-terminal and four C-terminal (Staudt et al. 2006), of which the C-terminal zinc fingers are sufficient to recapitulate its known DNA-binding specificity (Liang et al. 2008). Vielfaltig may help to maintain chromatin in an accessible state, participate in permissive protein–protein interactions, or have particular interaction partners. To investigate this last possibility, we assessed whether nearby binding of any factor could help to explain the relationship between TAGteam motifs and overlapping binding. Intriguingly, after controlling for levels of Medea binding, we observed little difference in binding of all factors between regions with and without TAGteam motifs (Supplemental Fig. 12A). Furthermore, ~80% of regions where Medea is highly bound contain a TAGteam motif—the highest fraction for any of the 21 factors—even in the absence of strong overlapping binding (Supplemental Fig. 12B), suggesting that Medea may frequently interact with Vielfaltig.
Highly conserved sequence homologs of vielfaltig have been identified in other insects, but not in vertebrates (Staudt et al. 2006). However, overlapping patterns of genome-wide binding of diverse transcription factors appears to be a common feature of undifferentiated animal cells. We propose that other animals may possess functional homologs that associate with hotspots of binding of multiple transcription factors, thereby influencing global patterns of gene expression.
Methods
Data location
All data analyzed in this work has been previously published and was downloaded from publicly accessible sites. Wiggle files and the location of 25% false discovery rate (FDR) ChIP peaks for ChIP-chip data sets (21 sequence-specific transcription factors and phosphorylated RNA polymerase II) (MacArthur et al. 2009) were downloaded from the Berkeley Drosophila Transcription Network Project website (http://bdtnp.lbl.gov/Fly-Net/browseChipper.jsp). Wiggle files for DNase I sensitivity measurements were downloaded from the UCSC Genome Browser FTP site (ftp://hgdownload.cse.ucsc.edu/goldenPath); raw sequencing reads are available from the SRA (project SRP002474) (Thomas et al. 2011). Wiggle files for the ORC data set (Roy et al. 2010) were downloaded from the NCBI Sequence Read Archive (SRA) (accession number GSM694124). ChIP-seq binding data for six anterior–posterior transcription factors (Bradley et al. 2010) was obtained from the NCBI Gene Expression Omnibus (GEO) database (accession number GSE20369). When multiple data sets were available (e.g., ChIP data sets for multiple antibodies, or technical replicates), we used the data sets analyzed in the original publications (MacArthur et al. 2009; Bradley et al. 2010; Roy et al. 2010; Li et al. 2011).
Data normalization
We performed a z-score (standard) normalization for each of the ChIP-chip data sets (MacArthur et al. 2009). For each ChIP-chip data set, we computed the maximum ChIP signal for each ChIP peak, and then scaled the ChIP data such that the mean signal over the bound regions was 0, with a standard deviation of 1. We performed a similar normalization for each of the ChIP-seq data sets (Bradley et al. 2010), excepting the DNase I and ORC data sets, which we did not normalize.
We defined each ChIP peak to be the 400 bp centered on the peak summit. Using different peak widths (100–500 bp) did not affect our results.
ChIP binding plots
When creating the plots in Figure 1, linear interpolation was used at base pairs for which ChIP data was unavailable. ChIP data for each factor was smoothed using local polynomial regression performed with the loess function in R (R Development Core Team 2005) with default parameters and a smoothing value of 0.05.
Conservation analysis
We used FSA (Bradley et al. 2009) with the options “–softmasked–refinement -1–mercator cons” to align D. melanogaster and D. yakuba, based on a previously created Mercator map (Dewey 2006; Bradley et al. 2010). For each TAGteam motif instance in D. melanogaster, we assessed whether it was aligned to a TAGteam motif in D. yakuba. The three heptamers CAGGTAG, tAGGTAG, and CAGGTAa, were considered TAGteam motifs, so if, for example, CAGGTAG was aligned to tAGGTAG, then it was considered conserved.
Principal components analysis (PCA)
Taking the union of all ChIP peaks for the 21 factors analyzed, we computed the maximum ChIP signal for each transcription factor in each ChIP peak, and used the prcomp function in R to perform PCA over this data set. We calculated the levels of overlapping binding at ChIP peaks by projecting the binding data onto the first principal component. Divergence in overlapping binding between species represented absolute divergence in overlapping binding, and was calculated as previously (Bradley et al. 2010).
Quantitative plots
All plots with quantitative x-axes used 120 overlapping bins. The degree of overlap differed based on the number of data points in each analysis (80% for Fig. 2A,C,G and Fig. 3G,I, 90% for Fig. 2D and Fig. 4B,C,E,F, and 95% for Fig. 2H). Empirical means for each bin were used to create the plotted lines, and shading around the plotted lines indicates the corresponding estimated standard error of the mean.
Transcription factor binding site motifs
We downloaded transcription factor recognition motifs from the BDTNP website, as well as a separate set of bacterial one-hybrid-derived motifs from the FlyFactorSurvey database (Zhu et al. 2011). For each transcription factor, we assessed the quality of its corresponding downloaded motifs by computing the associated differences in binding between peaks with and without motif matches within 100 bp of ChIP summits, and chose the motif and P-value cutoff (either 10−4 or 10−5) that maximized this difference. For six factors (Knirps, Paired, Tailless, Daughterless, Medea, and Schnurri), we could not find motifs that were either correlated with increases in binding or enriched near ChIP summits (as compared with their average genome-wide occurrence). We excluded these factors as well as Mothers against dpp, whose 5-bp motif was too short to satisfy our P-value cutoffs. We focused on the remaining 14 factors for all transcription factor motif analyses.
The number of transcription factor motifs near each ChIP peak (Fig. 2D) was calculated as the total number of matches to any of these 14 transcription factor motifs within 500 bp of the peak summit. All motif occurrences were identified by running PATSER (Hertz and Stormo 1999) on both the forward and reverse DNA strands using the previously identified P-value cutoffs. For Supplemental Figure 6, we restricted to the five factors with well-defined binding sites for which ChIP-seq data was available in both D. melanogaster and D. yakuba (Bicoid, Hunchback, Caudal, Giant, and Kruppel).
We checked for potential overlap between TAGteam motifs and binding sites for the other factors studied by enumerating all 13-bp variants of the form NNNCAGGTAGNNN and using PATSER to search for matches (P < 10−5) for the 14 factors against these CAGGTAG sites in context. No matches were reported.
Heptamer analysis and Vielfaltig PWM
In order to assess the uniqueness of the relationship between TAGteam motifs and overlapping binding (Supplemental Fig. 5), we enumerated all heptamers. For each heptamer, we computed the increase in overlapping binding at ChIP peaks associated with its presence (as the height of the peak in Fig. 2C, but computed for single heptamers).
To construct a PWM for Vielfaltig (Fig. 2F), we restricted the above analysis to heptamers differing by no more than 2 bp from the consensus CAGGTAG motif. We furthermore required that its fractional occurrence near ChIP peaks exhibit a monotonic dependence (R2 > 0.8) on overlapping transcription factor binding (as in Fig. 2A, but computed for single heptamers), and discarded those that did not. Weighting each heptamer by its association with overlapping binding, we constructed a PWM using the R package seqLogo (http://bioconductor.org/packages/2.6/bioc/html/seqLogo.html).
DNase I and ORC analyses
For Figure 3A–F, interpolated ChIP signals (computed as in Fig. 1) at each base were projected onto the first principal component in order to estimate overlapping transcription factor binding at each base. Per-base estimates of DNase I data were obtained directly from the relevant wiggle files. Both overlapping binding and DNase I data were smoothed (as in Fig. 1).
For DNase I and ChIP quantitative analyses (Fig. 3G,H), we enumerated all ChIP peaks and computed the corresponding DNase I data values as the maximum DNase I signal within the 400 bp centered on ChIP peak summits. ORC analyses (Fig. 3I; Supplemental Fig. 9) were performed similarly. Heat map plots (Fig. 3H; Supplemental Figs. 8,9,12) were created by placing ChIP peaks into a matrix of overlapping bins (120 × 120). Each dimension of a bin was 1/6 of the range of the plot.
Gene expression analyses
A list of genes, their coordinates (including transcription start sites), and their expression levels from mitotic cycles 10–14 was obtained from published data (Data set S1) (Lott et al. 2011). We used this publication's classification of genes as zygotically or maternally transcribed. We defined a gene's promoter as the 1.5 kb centered on its TSS, and putative enhancers as ChIP peaks within 1.5–5 kb upstream of its TSS, screening out all ChIP peaks that lay within any upstream gene in order to minimize incorrect enhancer assignments. Pol II occupancy of a promoter was defined as the highest Pol II ChIP value within the 1.5 kb centered on the gene's TSS.
Acknowledgments
We thank Zeba Wunderlich, Angela DePace, and Lior Pachter for helpful discussions, and Toshio Tsukiyama and Susan Parkhurst for comments on the manuscript. R.K.B. was supported by the Damon Runyon Cancer Research Foundation (DRG 2032-09 and DFS 04-12) and by funding from the Fred Hutchinson Cancer Research Center.
Authors' contributions: R.S. and R.K.B. designed the experiments. R.S. performed the experiments and created the figures. R.K.B. conceived the study and wrote the manuscript.
Footnotes
[Supplemental material is available for this article.]
Article published online before print. Article, supplemental material, and publication date are at http://www.genome.org/cgi/doi/10.1101/gr.130682.111.
References
- Beall EL, Manak JR, Zhou S, Bell M, Lipsick JS, Botchan MR 2002. Role for a Drosophila Myb-containing protein complex in site-specific DNA replication. Nature 420: 833–837 [Abstract] [Google Scholar]
- Berman BP, Nibu Y, Pfeiffer BD, Tomancak P, Celniker SE, Levine M, Rubin GM, Eisen MB 2002. Exploiting transcription factor binding site clustering to identify cis-regulatory modules involved in pattern formation in the Drosophila genome. Proc Natl Acad Sci 99: 757–762 [Europe PMC free article] [Abstract] [Google Scholar]
- Bosco G, Du W, Orr-Weaver TL 2001. DNA replication control through interaction of E2F-RB and the origin recognition complex. Nat Cell Biol 3: 289–295 [Abstract] [Google Scholar]
- Boyer LA, Lee TI, Cole MF, Johnstone SE, Levine SS, Zucker JP, Guenther MG, Kumar RM, Murray HL, Jenner RG, et al. 2005. Core transcriptional regulatory circuitry in human embryonic stem cells. Cell 122: 947–956 [Europe PMC free article] [Abstract] [Google Scholar]
- Bradley RK, Roberts A, Smoot M, Juvekar S, Do J, Dewey C, Holmes I, Pachter L 2009. Fast statistical alignment. PLoS Comput Biol 5: e1000392 10.103l/journal.pcbi.1000392 [Europe PMC free article] [Abstract] [Google Scholar]
- Bradley RK, Li XY, Trapnell C, Davidson S, Pachter L, Chu HC, Tonkin LA, Biggin MD, Eisen MB 2010. Binding site turnover produces pervasive quantitative changes in transcription factor binding between closely related Drosophila species. PLoS Biol 8: e1000343 10.1371/journal.pbio.1000343 [Europe PMC free article] [Abstract] [Google Scholar]
- Chen X, Xu H, Yuan P, Fang F, Huss M, Vega VB, Wong E, Orlov YL, Zhang W, Jiang J, et al. 2008. Integration of external signaling pathways with the core transcriptional network in embryonic stem cells. Cell 133: 1106–1117 [Abstract] [Google Scholar]
- Dewey CN. 2006. “Whole-genome alignments and polytopes for comparative genomics.” PhD thesis, University of California, Berkeley. [Google Scholar]
- Dominguez-Sola D, Ying CY, Grandori C, Ruggiero L, Chen B, Li M, Galloway DA, Gu W, Gautier J, Dalla-Favera R 2007. Non-transcriptional control of DNA replication by c-Myc. Nature 448: 445–451 [Abstract] [Google Scholar]
- Gerstein MB, Lu ZJ, Van Nostrand EL, Cheng C, Arshinoff BI, Liu T, Yip KY, Robilotto R, Rechtsteiner A, Ikegami K, et al. 2010. Integrative analysis of the Caenorhabditis elegans genome by the modENCODE project. Science 330: 1775–1787 [Europe PMC free article] [Abstract] [Google Scholar]
- Granok H, Leibovitch BA, Shaffer CD, Elgin SC 1995. Chromatin. Ga-ga over GAGA factor. Curr Biol 5: 238–241 [Abstract] [Google Scholar]
- Harrison MM, Botchan MR, Cline TW 2010. Grainyhead and Zelda compete for binding to the promoters of the earliest-expressed Drosophila genes. Dev Biol 345: 248–255 [Europe PMC free article] [Abstract] [Google Scholar]
- He Q, Bardet AF, Patton B, Purvis J, Johnston J, Paulson A, Gogol M, Stark A, Zeitlinger J 2011. High conservation of transcription factor binding and evidence for combinatorial regulation across six Drosophila species. Nat Genet 43: 414–420 [Abstract] [Google Scholar]
- Hertz GZ, Stormo GD 1999. Identifying DNA and protein patterns with statistically significant alignments of multiple sequences. Bioinformatics 15: 563–577 [Abstract] [Google Scholar]
- Kaplan T, Li XY, Sabo PJ, Thomas S, Stamatoyannopoulos JA, Biggin MD, Eisen MB 2011. Quantitative models of the mechanisms that control genome-wide patterns of transcription factor binding during early Drosophila development. PLoS Genet 7: e1001290 10.1371/journal.pgen.1001290 [Europe PMC free article] [Abstract] [Google Scholar]
- Kazemian M, Blatti C, Richards A, McCutchan M, Wakabayashi-Ito N, Hammonds AS, Celniker SE, Kumar S, Wolfe SA, Brodsky MH, et al. 2010. Quantitative analysis of the Drosophila segmentation regulatory network using pattern generating potentials. PLoS Biol 8 10.1371/journal.pbio.10004546 [Europe PMC free article] [Abstract] [Google Scholar]
- Kazemian M, Zhu Q, Halfon MS, Sinha S 2011. Improved accuracy of supervised CRM discovery with interpolated Markov models and cross-species comparison. Nucleic Acids Res 39: 9463–9472 [Europe PMC free article] [Abstract] [Google Scholar]
- Khoueiry P, Rothbacher U, Ohtsuka Y, Daian F, Frangulian E, Roure A, Dubchak I, Lemaire P 2010. A cis-regulatory signature in ascidians and flies, independent of transcription factor binding sites. Curr Biol 20: 792–802 [Abstract] [Google Scholar]
- Li XY, MacArthur S, Bourgon R, Nix D, Pollard DA, Iyer VN, Hechmer A, Simirenko L, Stapleton M, Luengo Hendriks CL, et al. 2008. Transcription factors bind thousands of active and inactive regions in the Drosophila blastoderm. PLoS Biol 6: e27 10.1371/journal.pbio.0060027 [Europe PMC free article] [Abstract] [Google Scholar]
- Li XY, Thomas S, Sabo PJ, Eisen MB, Stamatoyannopoulos JA, Biggin MD 2011. The role of chromatin accessibility in directing the widespread, overlapping patterns of Drosophila transcription factor binding. Genome Biol 12: R34 10.1186/gb-2011-12-4-r34 [Europe PMC free article] [Abstract] [Google Scholar]
- Liang HL, Nien CY, Liu HY, Metzstein MM, Kirov N, Rushlow C 2008. The zinc-finger protein Zelda is a key activator of the early zygotic genome in Drosophila. Nature 456: 400–403 [Europe PMC free article] [Abstract] [Google Scholar]
- Liberman LM, Stathopoulos A 2009. Design flexibility in cis-regulatory control of gene expression: synthetic and comparative evidence. Dev Biol 327: 578–589 [Europe PMC free article] [Abstract] [Google Scholar]
- Lott SE, Villalta JE, Schroth GP, Luo S, Tonkin LA, Eisen MB 2011. Noncanonical compensation of zygotic X transcription in early Drosophila melanogaster development revealed through single-embryo RNA-seq. PLoS Biol 9: e1000590 10.1371/journal.pbio.1000590 [Europe PMC free article] [Abstract] [Google Scholar]
- MacAlpine HK, Gordan R, Powell SK, Hartemink AJ, MacAlpine DM 2010. Drosophila ORC localizes to open chromatin and marks sites of cohesin complex loading. Genome Res 20: 201–211 [Europe PMC free article] [Abstract] [Google Scholar]
- MacArthur S, Li XY, Li J, Brown JB, Chu HC, Zeng L, Grondona BP, Hechmer A, Simirenko L, Keranen SV, et al. 2009. Developmental roles of 21 Drosophila transcription factors are determined by quantitative differences in binding to an overlapping set of thousands of genomic regions. Genome Biol 10: R80 10.1186/gb-2009-10-7-r80 [Europe PMC free article] [Abstract] [Google Scholar]
- Markstein M, Markstein P, Markstein V, Levine MS 2002. Genome-wide analysis of clustered Dorsal binding sites identifies putative target genes in the Drosophila embryo. Proc Natl Acad Sci 99: 763–768 [Europe PMC free article] [Abstract] [Google Scholar]
- Marson A, Levine SS, Cole MF, Frampton GM, Brambrink T, Johnstone S, Guenther MG, Johnston WK, Wernig M, Newman J, et al. 2008. Connecting microRNA genes to the core transcriptional regulatory circuitry of embryonic stem cells. Cell 134: 521–533 [Europe PMC free article] [Abstract] [Google Scholar]
- Moorman C, Sun LV, Wang J, de Wit E, Talhout W, Ward LD, Greil F, Lu XJ, White KP, Bussemaker HJ, et al. 2006. Hotspots of transcription factor colocalization in the genome of Drosophila melanogaster. Proc Natl Acad Sci 103: 12027–12032 [Europe PMC free article] [Abstract] [Google Scholar]
- Negre N, Brown CD, Ma L, Bristow CA, Miller SW, Wagner U, Kheradpour P, Eaton ML, Loriaux P, Sealfon R, et al. 2011. A cis-regulatory map of the Drosophila genome. Nature 471: 527–531 [Europe PMC free article] [Abstract] [Google Scholar]
- Okada M, Hirose S 1998. Chromatin remodeling mediated by Drosophila GAGA factor and ISWI activates fushi tarazu gene transcription in vitro. Mol Cell Biol 18: 2455–2461 [Europe PMC free article] [Abstract] [Google Scholar]
- Ouyang Z, Zhou Q, Wong WH 2009. ChIP-Seq of transcription factors predicts absolute and differential gene expression in embryonic stem cells. Proc Natl Acad Sci 106: 21521–21526 [Europe PMC free article] [Abstract] [Google Scholar]
- Pique-Regi R, Degner JF, Pai AA, Gaffney DJ, Gilad Y, Pritchard JK 2011. Accurate inference of transcription factor binding from DNA sequence and chromatin accessibility data. Genome Res 21: 447–455 [Europe PMC free article] [Abstract] [Google Scholar]
- R Development Core Team. 2005 R: A language and environment for statistical computing. R Foundation for Statistical Computing, Vienna. [Google Scholar]
- Roy S, Ernst J, Kharchenko PV, Kheradpour P, Negre N, Eaton ML, Landolin JM, Bristow CA, Ma L, Lin MF, et al. 2010. Identification of functional elements and regulatory circuits by Drosophila modENCODE. Science 330: 1787–1797 [Europe PMC free article] [Abstract] [Google Scholar]
- Staudt N, Fellert S, Chung HR, Jackle H, Vorbruggen G 2006. Mutations of the Drosophila zinc finger-encoding gene vielfältig impair mitotic cell divisions and cause improper chromosome segregation. Mol Biol Cell 17: 2356–2365 [Europe PMC free article] [Abstract] [Google Scholar]
- Struffi P, Corado M, Kaplan L, Yu D, Rushlow C, Small S 2011. Combinatorial activation and concentration-dependent repression of the Drosophila even skipped stripe 3+7 enhancer. Development 138: 4291–4299 [Europe PMC free article] [Abstract] [Google Scholar]
- ten Bosch JR, Benavides JA, Cline TW 2006. The TAGteam DNA motif controls the timing of Drosophila pre-blastoderm transcription. Development 133: 1967–1977 [Abstract] [Google Scholar]
- Thomas S, Li XY, Sabo PJ, Sandstrom R, Thurman RE, Canfield TK, Giste E, Fisher W, Hammonds A, Celniker SE, et al. 2011. Dynamic reprogramming of chromatin accessibility during Drosophila embryo development. Genome Biol 12: R43 10.1186/gb-2011-12-5-r43 [Europe PMC free article] [Abstract] [Google Scholar]
- Tweedie S, Ashburner M, Falls K, Leyland P, McQuilton P, Marygold S, Millburn G, Osumi-Sutherland D, Schroeder A, Seal R, et al. 2009. FlyBase: enhancing Drosophila Gene Ontology annotations. Nucleic Acids Res 37: D555–D559 [Europe PMC free article] [Abstract] [Google Scholar]
- Wall G, Varga-Weisz PD, Sandaltzopoulos R, Becker PB 1995. Chromatin remodeling by GAGA factor and heat shock factor at the hypersensitive Drosophila hsp26 promoter in vitro. EMBO J 14: 1727–1736 [Europe PMC free article] [Abstract] [Google Scholar]
- Zeitlinger J, Zinzen RP, Stark A, Kellis M, Zhang H, Young RA, Levine M 2007. Whole-genome ChIP-chip analysis of Dorsal, Twist, and Snail suggests integration of diverse patterning processes in the Drosophila embryo. Genes Dev 21: 385–390 [Europe PMC free article] [Abstract] [Google Scholar]
- Zhu LJ, Christensen RG, Kazemian M, Hull CJ, Enuameh MS, Basciotta MD, Brasefield JA, Zhu C, Asriyan Y, Lapointe DS, et al. 2011. FlyFactorSurvey: a database of Drosophila transcription factor binding specificities determined using the bacterial one-hybrid system. Nucleic Acids Res 39: D111–D117 [Europe PMC free article] [Abstract] [Google Scholar]
- Zinzen RP, Girardot C, Gagneur J, Braun M, Furlong EE 2009. Combinatorial binding predicts spatio-temporal cis-regulatory activity. Nature 462: 65–70 [Abstract] [Google Scholar]
Articles from Genome Research are provided here courtesy of Cold Spring Harbor Laboratory Press
Full text links
Read article at publisher's site: https://doi.org/10.1101/gr.130682.111
Read article for free, from open access legal sources, via Unpaywall:
http://genome.cshlp.org/content/22/4/656.full.pdf
Citations & impact
Impact metrics
Citations of article over time
Alternative metrics
Smart citations by scite.ai
Explore citation contexts and check if this article has been
supported or disputed.
https://scite.ai/reports/10.1101/gr.130682.111
Article citations
An oncogenic enhancer promotes melanoma progression via regulating ETV4 expression.
J Transl Med, 22(1):547, 07 Jun 2024
Cited by: 0 articles | PMID: 38849954
Setting the stage for development: the maternal-to-zygotic transition in Drosophila.
Genetics, 225(2):iyad142, 01 Oct 2023
Cited by: 1 article | PMID: 37616526 | PMCID: PMC10550319
Review Free full text in Europe PMC
Chromatin accessibility in the Drosophila embryo is determined by transcription factor pioneering and enhancer activation.
Dev Cell, 58(19):1898-1916.e9, 08 Aug 2023
Cited by: 19 articles | PMID: 37557175 | PMCID: PMC10592203
Transcriptional Enhancers in Drosophila.
Genetics, 216(1):1-26, 01 Sep 2020
Cited by: 20 articles | PMID: 32878914 | PMCID: PMC7463283
Review Free full text in Europe PMC
Bicoid-Dependent Activation of the Target Gene hunchback Requires a Two-Motif Sequence Code in a Specific Basal Promoter.
Mol Cell, 75(6):1178-1187.e4, 08 Aug 2019
Cited by: 12 articles | PMID: 31402096 | PMCID: PMC6754290
Go to all (35) article citations
Data
Data behind the article
This data has been text mined from the article, or deposited into data resources.
BioStudies: supplemental material and supporting data
GEO - Gene Expression Omnibus (2)
- (1 citation) GEO - GSE20369
- (1 citation) GEO - GSM694124
Similar Articles
To arrive at the top five similar articles we use a word-weighted algorithm to compare words from the Title and Abstract of each citation.
Zelda and the maternal-to-zygotic transition in cockroaches.
FEBS J, 286(16):3206-3221, 30 Apr 2019
Cited by: 4 articles | PMID: 30993896
Molecular dissection of cis-regulatory modules at the Drosophila bithorax complex reveals critical transcription factor signature motifs.
Dev Biol, 359(2):290-302, 28 Jul 2011
Cited by: 17 articles | PMID: 21821017 | PMCID: PMC3202680
Zelda binding in the early Drosophila melanogaster embryo marks regions subsequently activated at the maternal-to-zygotic transition.
PLoS Genet, 7(10):e1002266, 20 Oct 2011
Cited by: 206 articles | PMID: 22028662 | PMCID: PMC3197655
One thousand and one ways of making functionally similar transcriptional enhancers.
Bioessays, 30(11-12):1052-1057, 01 Nov 2008
Cited by: 7 articles | PMID: 18937349
Review