Abstract
Free full text

Global biogeography of SAR11 marine bacteria
Associated Data
Abstract
The ubiquitous SAR11 bacterial clade is the most abundant type of organism in the world’s oceans, but the reasons for its success are not fully elucidated. We analysed 128 surface marine metagenomes, including 37 new Antarctic metagenomes. The large size of the data set enabled internal transcribed spacer (ITS) regions to be obtained from the Southern polar region, enabling the first global characterization of the distribution of SAR11, from waters spanning temperatures −2 to 30°C. Our data show a stable co-occurrence of phylotypes within both ‘tropical’ (>20°C) and ‘polar’ (<10°C) biomes, highlighting ecological niche differentiation between major SAR11 subgroups. All phylotypes display transitions in abundance that are strongly correlated with temperature and latitude. By assembling SAR11 genomes from Antarctic metagenome data, we identified specific genes, biases in gene functions and signatures of positive selection in the genomes of the polar SAR11—genomic signatures of adaptive radiation. Our data demonstrate the importance of adaptive radiation in the organism’s ability to proliferate throughout the world’s oceans, and describe genomic traits characteristic of different phylotypes in specific marine biomes.
Introduction
An ultimate goal in ecology is to elucidate the distribution of taxa with respect to measureable environmental parameters. However, due to the dynamic nature of marine systems, and the inherent difficulty in examining microbial populations, global biogeographic patterns of bacterioplankton remain largely obscure. The distribution of only one marine bacterial clade, the phototrophic Prochlorococcus, has been defined (Johnson et al, 2006) to a sufficient extent to permit inclusion into large-scale oceanographic models (Follows et al, 2007).
Although only discovered in 1990, and cultured in 2002 (Rappe et al, 2002), it has become clear that the bacterial SAR11 clade is one of the most successful assemblages of closely related organisms on the planet, being ubiquitous in marine environments, comprising about a third of the Bacteria, and dominating the heterotrophic bacterial community composition (Morris et al, 2002). Based on analysis of whole genome sequences, it has been proposed the SAR11 clade comprises sufficient phylogenetic diversity to be considered a distinct family within the Alphaproteobacteria, nominated as Pelagibacteraceae, fam. nov. (Thrash et al, 2011), although the monophyletic and deep phylogenetic placement of this family has been questioned (Rodríguez-Ezpeleta and Embley, 2012).
This abundance of SAR11 might in part be due to efficient genome streamlining (Giovannoni et al, 2005), minimizing the cells’ requirements for nutrients and thus allowing replication under the most limiting nutrient conditions. Another likely mechanism driving this organism’s ecological success is the adaptive divergence of strains into phylotypes specifically suited to either different oceanographic provinces or lifestyles, that is, ‘ecotypes’ (Field et al, 1997; Garcia-Martinez and Rodriguez-Valera, 2000; Brown et al, 2005; Carlson et al, 2009). Across a several year time series in the Sargasso Sea (surface to 300m depth), at least three distinct phylogenetic subgroups have been correlated with seasonal mixing and stratification, raising questions about functional traits associated with ecotype delineations (Carlson et al, 2009). Other work using internal transcribed spacer (ITS) regions of 16S–23S rRNA gene sequences has identified seasonal patterns in the abundance of phylotypes, as defined by distinct ITS clusters (Brown et al, 2005). An association between SAR11 ecotype and functional capacity has also been inferred from whole genome comparisons of isolates (Schwalbach et al, 2010). Recently, the evolutionary loss of the mismatch repair genes mutLS from SAR11 genomes has been hypothesized as the reason why the clade supports such extensive sequence divergence (Viklund et al, 2012).
The various levels of identification (16S rRNA gene, ITS and whole genome sequences) provide different levels of resolution, and genomic differences exist below the resolution of the conserved molecular markers. The first two SAR11 genomes sequenced, HTCC1062 and HTCC1002, contain only one nucleotide difference in their 16S rRNA gene sequences but 10 nucleotide differences in their ITS regions, which have a pairwise similarity of 97.5%. In protein coding regions, the genomes are 97.4% similar in nucleotide sequence (Wilhelm et al, 2007). The two genomes differ in length by 12298 nucleotides with HTCC1002 having a number of gene insertions in one genome relative to the other that are mostly present in the hypervariable region HVR2 (Wilhelm et al, 2007).
Until now insufficient data have been available to examine how phylotypes may be distributed on a global scale or in relation to environmental parameters. Here, we use 16S rRNA gene and ITS sequences to define SAR11 phylotypes and their biogeography, and genomic analysis of representatives of phylotypes to assess functional differences that may explain their biogeography. The link between environmental temperature, latitude and phylotype, and their associated genomic traits, provides strong evidence that SAR11 phylotypes represent environmentally selected ecotypes.
Results
Global distribution of SAR11 phylotypes
A synopsis of the phylogenetic hierarchy used in this paper is provided in Table I. By compiling a database composed of 862 ITS sequences (Supplementary Table S1), originating from ‘polar’ (<10°C), ‘temperate’ (between >10 and <20°C) and ‘tropical’ (>20°C) regions, we identified the abundance of each of nine pre-defined phylotypes relative to the total observable SAR11 community structure in 128 surface marine metagenomes. These included 37 new data sets we generated from the previously underrepresented Southern Ocean and Antarctic marine systems (Supplementary Table S2). Combined, these metagenomic data represent surface marine coastal and open-ocean sites across a global transect from latitude 45°N to 77°S and spanning in-situ temperatures from −2 to 30°C (Supplementary Table S2).
Table 1
Subgroup (16S rRNA gene) | Designation origin | Phylotype (ITS) | Designation origin | Isolate | Reference |
---|---|---|---|---|---|
Percentages relate to the range of pairwise distances, or phylogenetic diversity captured within each group based on full-length 16S rRNA gene sequences in Supplementary Figure S1. | |||||
S1a100–96.52% | Morris et al (2005) | P1a.1100–98.15% | Garcia-Martinez and Rodriguez-Valera (2000) | HTCC1062HTCC1002HTCC8010HTCC8022HTCC8040 | Rappe et al (2002); Stingl et al (2007) |
P1a.2100–98.88% | Brown and Fuhrman (2005) | HTCC8038HTCC8041HTCC8045HTCC8046HTCC8049 | Stingl et al (2007) | ||
P1a.3100–97.73% | Brown and Fuhrman (2005) | HTCC8051HTCC8047HTCC7211HTCC7215HTCC7216HTCC7217 | Stingl et al (2007) | ||
S1b100–97.12% | Morris et al (2005) | P1b99.78–97.12% | This study | N/A | |
NA1 | This study | N/A | |||
NA2 | This Study | N/A | |||
S2100–91.98% | Morris et al (2005) | P2.1100–93.41% | Brown and Fuhrman (2005) | N/A | |
P2.2100–96.49% | Garcia-Martinez and Rodriguez-Valera (2000) | N/A | |||
P2.3100–96.00% | Garcia-Martinez and Rodriguez-Valera (2000) | N/A | |||
S3100–85.44% | Morris et al (2005) | P3.1100–97.55% | This study | HIMB114 | |
P3.2100–97.49% | This study | IMCC9063 | Oh et al (2011) |
Analysis of the 2983 resultant ITS markers indicates that no phylotype is abundant everywhere in surface waters (Figure 1), although our ITS analysis cannot determine if a low abundance phylotype has a consistent level of abundance globally. In tropical biomes, representatives from P1a.3 (HTCC7211), P1b and P2.1 were generally present. Although occurring rarely, P3.1 appeared only in samples from waters warmer than 20.5°C. In cold water samples, P1a.1 (HTCC1002, HTCC1062) dominated. Although in lower total abundances, P2.2 was more abundant than P2.1 in waters <5°C and P3.2 was present only in waters <18.2°C. Although the majority of polar metagenomic data sets originate from the Antarctic, it should be noted that 49.7% of the ITS sequences in our database are from samples taken in the Arctic while only 3% are derived from Antarctic samples (with the rest from temperate and tropical regions—Supplementary Table S1). Thus, by identifying P1a.1 phylotypes in Antarctic waters that have been defined using Arctic samples, the distribution of this phylotype can be considered bipolar. Phylotype P2.2 was defined from Antarctic waters (Garcia-Martinez and Rodriguez-Valera, 2000) but is not represented in Arctic clone libraries. This phylotype is characteristic of Antarctic water metagenome data (Figure 1) and also appears in metagenomes from the English Channel (August 2200, h, 15.8°C), Delaware Bay (GS011, 11°C), Newport Harbor (GS008, 9.4°C) and Nags Head (GS013, 9.3°C). Its distribution in Arctic waters will be able to be determined when metagenome data become available. Temperate waters maintained a more diverse mix of phylotypes, and are habitat to P1a.2 that often dominates samples <20°C, but represents only 0.2% of all detectable SAR11 fragments in surface waters below 2°C. Overall, the data show that transition temperatures are evident where major shifts in phylotype composition occur, indicating that on a global scale there is a strong relationship between the distribution of phylotypes of the SAR11 clade and temperature (Figure 1).

Phylogeny and temperature association with the biogeographic distribution of the SAR11 clade. ARB neighbour-joining trees generated by collapsing significant nodes in trees generated from representative 16S rRNA gene sequences (subgroups), and ITS sequences (phylotypes). Individual clones not included in triangulated clusters maintain the conserved motif of P1a.1 but have several base-pair deletions. These 14 sequences are designated as belonging to phylotype P1a.1 in our database. Expanded trees are shown in Supplementary Figure S1. The four lower graphs show relative contributions of the SAR11 phylotypes (coloured as for the ITS tree) to total SAR11 composition revealing phylotype distribution that closely relates to temperature. The data bars are arranged along the x axis according to increasing temperature of the sampling location for each metagenome (from left to right), with relative phylotype abundance shown on the left y axis and environmental temperature (indicated by black diamonds) shown on the right y axis in °C.
A distance-based linear model (DisTLM) and distance-based redundancy analysis (dbRDA) method was used to analyse and model the relationship between the multivariate SAR11 ITS data set (i.e., the relative abundance of each pre-defined phylotype detected within each metagenomic sample) and the available predictor variables (Supplementary Table S3) including latitude, temperature, chlorophyll concentration, salinity and total depth of the water column (DWC) which was used as a continuous variable proxy for coastal or open ocean. We also analysed data sets against longitude but these results were not significant and were removed from the model. When all phylotypes were used in the analysis, and when each environmental variable was considered in isolation, temperature (57.5%) and latitude (57.3%) explained the greatest proportion of the observed phylotype variability (Supplementary Table S4). The variability described by DWC (9.7%), and chlorophyll (13.7%) were both statistically significant by permutation (P=0.001) while that described by salinity was not (P=0.089). The BEST solution, where all possible combinations of predictor variables were assessed to provide the most parsimonious model, used temperature and latitude as the first two explanatory variables, followed by DWC, salinity and chlorophyll. When all variables were used they accounted for 62.6% of the observed variation. Each variable was also fitted last in a forward selection model to specifically determine the extent to which they contributed to the observed variability once all other factors had been considered. Temperature, latitude and DWC all displayed small (~2%) but statistically significant (P=0.001) contributions. Hence, although temperature and latitude are highly correlated in the complete data set, they each provide some extra explanatory power on their own, as does DWC.
dbRDA analysis of ITS phylotype variation showed that samples originating from polar, temperate and tropical biomes cluster together, with the majority of ITS phylotypes correlating strongly with the dbRDA1 axis (Figure 2A and D). This axis describes 59.6% of the total variation (and 94.8% of the modelled variation) and is defined primarily by temperature and latitude. Analysis of similarity (ANOSIM) confirms these temperature bins as strong (global R for comparison >20°C/10–20°C=0.599, >20°C/<10°C=0.946, 10–20°C/<10°C=0.499) and significant (P=0.0001) descriptive factors.
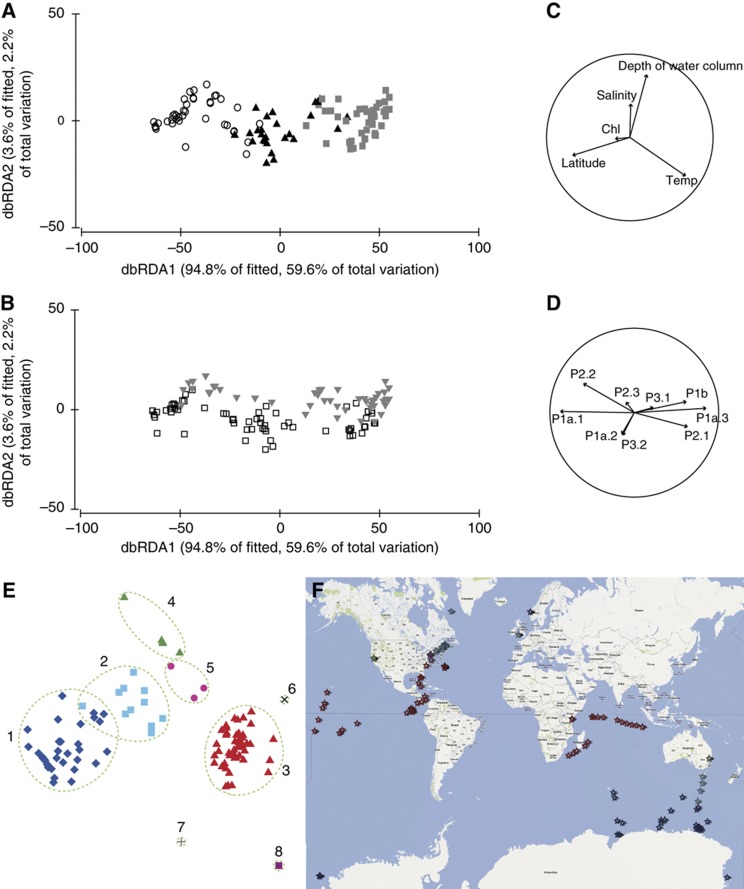
Clustering and geolocation of ocean samples based on SAR11 phylotype composition. (A) dbRDA ordination for the fitted model of SAR11 ITS phylotype composition data (based on Bray-Curtis similarity after square root transformation of abundances) versus environmental variables. Clustering illustrates the strong separation of samples related to temperature bins <10°C (open circles), 10–20°C (black triangles) and >20°C (grey squares). (B) Identical plot to (A), with sample icons changed to reflect the ‘coastal’ (grey triangles) or ‘open ocean’ (open squares) affiliation of each sample. (C) Eigen vectors indicating the strength and direction of correlation for each environmental variable from (A). (D) Eigen vectors indicating the strength and direction of correlation for each SAR11 phylotype from (A). (E) nMDS based on between sample Bray-Curtis similarities calculated using square root transformed SAR11 community composition and abundance data (2D stress: 0.07). Clusters are coloured based on membership to groups identified as having >60% similarity in community composition (marked by green rings). Group number (1) blue diamonds, samples from polar regions; (2) light blue squares, temperate regions; (3) red triangles, tropical regions; (4) green triangles, Western Channel Observatory (UK), Monterey Bay (USA) and Botany Bay (Australia); (5) fuschia dots, Chesapeake Bay, Delaware Bay and nearby Nags Head; (6) black ‘x’, GS037 and S_35139 (tropical Pacific Ocean) and NASB_179_2 and NASB_174_2 (Sargasso Sea) (contain only ‘tropical’ phylotype P1a.3); (7) grey cross, Mont_bay_3 (contains only phylotype P2.1); (8) pink square, NASB-179_1 (Sargasso Sea) (contains only phylotype P1b). (F) Geolocation of the groups shown in (E), depicted by stars (as coloured in E).
dbRDA and ANOSIM analyses also revealed a small (global R=0.154) but statistically significant (P=0.001) separation between coastal and open-ocean sites (Figure 2B and C). Correlation of phylotypes with dbRDA2 axis showed that P3.2 and P1a.2 are the major drivers of this separation (Figure 2D). P3.2 occurred rarely in the data set and 11 of the 13 metagenomes it was identified in were from coastal waters. When considering only the relative abundances of three P1a phylotypes, DistLM again identified latitude and temperature as the greatest explanatory variables (Supplementary Table S4) and ANOSIM results comparing temperature bins (global R>20°C/10–20°C=0.642, >20°C/<10°C=0.957, 10–20°C/<10°C=0.498, all P=0.001) were stronger than comparing coastal versus open ocean (global R=0.176, P=0.001).
To reduce the influence of temperature, the tropical (>20°C), temperate (10–20°C) and polar (<10°C) metagenomes were segregated and each of the three subsets examined. In the tropical subset where P1a.3 (HTCC7211) dominates, there was no significant difference in its relative contribution to the SAR11 community in coastal (n=16) versus open-ocean (n=32) sites (ANOSIM global R=0.072, P=0.1). Similarly, in the polar subset, no significant difference was observed in the distribution of P1a phylotypes, including P1a.1 (HTCC1062) between coastal (n=34) versus open-ocean (n=12) samples (ANOSIM global R=0.113, P=0.113). In both instances, chlorophyll concentration had no significant correlation with distributions (DistLM marginal test tropical P=0.687, polar P=0.242). Therefore, in the tropical and polar subsets, regardless of the coastal or open-ocean environment or chlorophyll levels, there was no significant response observed in the relative abundance of P1a phylotypes. Where a significant difference did occur between coastal (n=24) and open-ocean (n=9) sites was in the temperate subset (ANOSIM global R=0.296, P=0.002), although again no relationship with chlorophyll concentrations was observed using DistLM (P=0.4). Simper analysis of the temperate subset, which determines the relative over or under representation of phylotypes in tested samples, identified P1a.1 (HTCC1062) and P1a.2 as occurring preferentially in coastal samples, with P1a.3 (HTCC7211) overrepresented in open-ocean samples, similar to previous findings (Schwalbach et al, 2010).
As a further test of biogeography, we used non-metric multidimensional scaling (nMDS) analysis (which clusters samples based solely on phylotype composition without taking into account environmental parameters) to visualize clusters of samples containing highly similar SAR11 phylotype compositions (Figure 2E). We identified eight clusters that were defined by >60% similarity (Bray-Curtis) between samples (green rings in Figure 2E). The geographic location of samples within each cluster (Figure 2E) was marked on a global map using the same colour for members of the same cluster (Figure 2F). The three major clusters (87% of the samples) again represent tropical (red triangles), temperate (light blue squares) and polar (blue diamonds) biomes. Minor clusters involved samples from similar types of environments, such as Chesapeake Bay (GS012), Delaware Bay (GS011) and nearby Nags Head (GS013) (Figure 2, fuschia dots). These locations contain a diverse mix of phylotypes including P3.2, P1a.1 (GS012, GS013 only), P1a.2, P1a.3, P2.1 (GS011, GS012 only) and P2.2 (GS011 only). Alternately, minor clusters involved samples from geographically disparate locations, such as Botany Bay (Australia), Western Channel Observatory (UK) and Monterey Bay (USA) (Figure 2, green triangles).
Genomic signatures of adaptive radiation
Ace Lake, Antarctica was formerly a marine inlet and has remnant marine microbiota (Lauro et al, 2011). From metagenome data of Ace Lake, mosaic SAR11 genomes were assembled for the widespread P1a.1 phylotype (ACE_P1a.1), and the largely uncharacterized P3.2 phylotype (ACE_P3.2). ACE_P1a.1 has equivalent genome length and average nucleotide identity (ANI) of 83.27% and percentage conserved DNA (PCD) of 6.16% (Figure 3; Goris et al, 2007) when compared with HTCC1062 (Giovannoni et al, 2005) and ANI of 76.12% and PCD of 0.41% when compared with HTCC7211. The length of the ACE_P3.2 and HIMB114 genomes was also equivalent (Figure 3) with ANI of 69.53% and PCD of 0.257%. The ANI and PCD values for ACE_P1a.1 compared with HTCC1062 are similar to that determined for Shewanella species, all of which had >70% ANI and >94% 16S rRNA gene sequence identity (Goris et al, 2007). The ANI and PCD values for ACE_P1a.1 compared with HTCC7211, and ACE_P3.2 compared with HIMB114 are similar to the values calculated for Pseudomonas species, which had PCD values ranging from 13.1 to 0.0001% (Goris et al, 2007). The Arctic strain, IMCC9063 (Oh et al, 2011) also has equivalent genome length, and has an ANI with ACE_P3.2 of 94.17% and PCD of 74.05% which would be considered similar to a DNA-DNA hybridization of ~70% (Goris et al, 2007). Taken together, these results support a strong correlation between ITS identity and genome similarity and are also consistent with the extensive sequence divergence recently reported for members of the SAR11 clade (Viklund et al, 2012).

Genome synteny and recruitment plots of SAR11 genomes. Whole genome alignments between genomes belonging to different SAR11 phylotypes: HIMB114 (P3.1), ACE_P3.2 (P3.2), HTCC1062 (P1a.1), ACE_P1a.1 (P1a.1) and HTCC7211 (P1a.3). In the synteny plots matches to the +strand (i.e., +/+) are in red, and matches to the −strand (i.e., +/−) in blue. blastn (run with standard parameters and the -m8 flag) matches of at least 30 nucleotides or longer are displayed. Interleaved among the synteny plots are the recruitments, with read-depth shown on a natural logarithmic scale, for each genome against representative metagenomes from Ace Lake (GS232, grey), polar (GS362, purple), temperate (GS368, orange) and tropical (S_35155+S_35163, red) zones. The recruitment appears mainly orange in colour for HIMB114 (P3.1) and HTCC7211 (P1a.3) because these genomes recruit more strongly to the temperate metagenomes, whereas the recruitment for ACE_P3.2 (P3.2) and ACE_P1a.1 (P1a.1) is mainly grey reflecting their origin and high numerical abundance in Ace lake. Black arrows show examples of regions with low recruitment.
Using these Antarctic genomes as polar representatives, and HTCC7211 (P1a.3) and HIMB114 (P3.1) as tropical representatives, a high stringency BLAST search (1e−10; min identity 92%) was performed against global ocean sampling expedition (GS; Rusch et al, 2007) data sets representing the three biomes (polar, temperate and tropical) (Figure 3). The proportion of the normalized reads from the polar metagenomes that recruited to each genome representative was 60% for ACE_P3.2 versus 40% for HIMB114, and 65% for ACE_P1a.1 versus 35% for HTCC7211. The proportion of the normalized reads from the tropical metagenomes that recruited to each genome representative was 44% for ACE_P3.2 versus 56% for HIMB114, and 45% for ACE_P1a.1 versus 55% for HTCC7211. Similar trends were observed for temperate metagenome data (data not shown). All polar versus tropical/temperate differences were statistically significant (two-tailed t-test P<0.005).
The genome synteny comparisons revealed that low synteny coincided with regions of low genome recruitment (Figure 3, examples are shown with black arrows). These regions were flanked by spikes of very high recruitment coverage and may represent sequences functioning as recombination hotspots within the genomes of members of the SAR11 clade.
A comparison of the gene content of the genomes of polar and tropical representatives revealed a set of genes specific to the polar representatives (ACE_P1a.1 versus HTCC7211, 344 genome-specific genes; ACE_P3.2 versus HIMB114, 444 genome-specific genes) which were overrepresented in COG (Cluster of Orthologous Groups of proteins) categories M (Cell wall/membrane/envelope biogenesis) and P (Inorganic ion transport and metabolism) (Figure 4; Supplementary Table S5). In addition, a large number of the polar-specific genes were hypothetical and are indicative of unknown mechanisms of niche adaptation. Many of the genes specific to each phylotype are diagnostic for the distribution of the host strains. A tblastn search for the phylotype-specific proteins in all the metagenomes in CAMERA (Sun et al, 2011) revealed a statistically significant (99% confidence) higher proportion of polar-specific genes within polar metagenomes (>87% of the hits for ACE_P1a.1) and tropical-specific genes within temperate and tropical metagenomes (>60% of the hits for HTCC7211; Supplementary Figure S2).
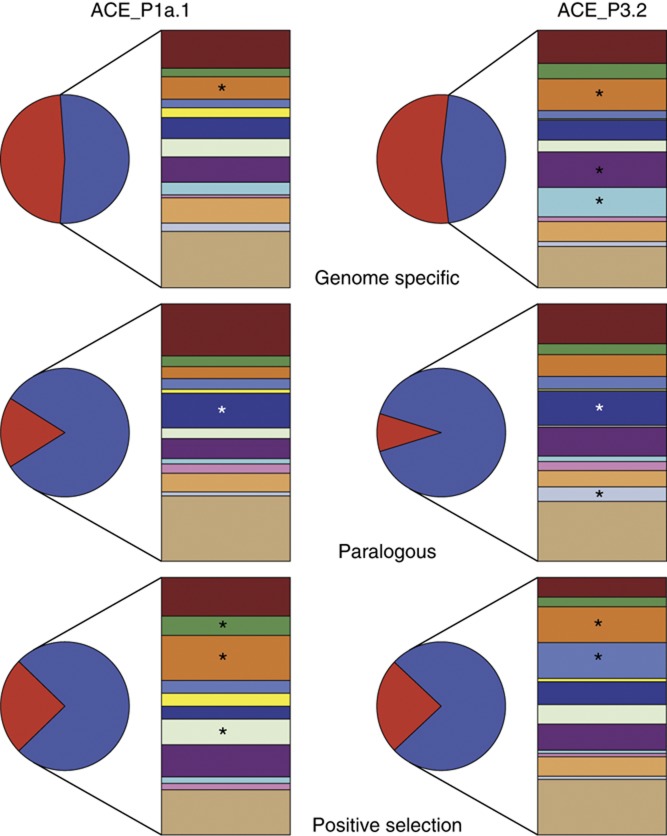
COG composition of genes specific, paralogous and positively selected in the polar SAR11 genomes. The pie chart represents the proportion of genes with (blue) or without (red) matches to the COG database. The matching genes were assigned to COG categories (from top to bottom): E—Amino-acid transport and metabolism (maroon); G—Carbohydrate transport and metabolism (green); M—Cell wall/membrane/envelope biogenesis (orange); H—Coenzyme transport and metabolism (violet); V—Defense mechanism (yellow); C—Energy production and conversion (dark blue); S—Function unknown (light green-grey); R—General function prediction only (dark purple); P—Inorganic ion transport and metabolism (light blue); K—Transcription (pink); J—Translation, ribosomal structure and biogenesis (salmon); Q—Secondary metabolites biosynthesis, transport and catabolism (light blue-grey); Other categories (light brown). An asterisk denotes the categories found to be statistically overrepresented when compared with the genome background.
Both the polar genomes also contained an overrepresentation of paralogous genes in COG category C (Energy production and conversion), which are mainly due to membrane-bound respiratory chain proteins (Figure 4; Supplementary Table S4). Overrepresentation in both the polar genomes is suggestive of adaptive changes in energy generation specific for low temperature environments.
Signatures of positive selection were detected in the two polar genomes compared with their most closely related tropical counterparts. Between ACE_P3.2 and HIMB114 the genes under selection were overrepresented in COG categories G (Carbohydrate transport and metabolism) and M (Cell wall/membrane/envelope biogenesis), and for ACE_P1a.1 versus HTCC7211, COG categories M (Cell wall/membrane/envelope biogenesis) and H (Coenzyme transport and metabolism) were overrepresented (Figure 4; Supplementary Table S4).
A number of other orthologous genes (e.g., cell division proteins ftsZ, lipid A biosynthesis lauroyl/palmitoleoyl acyltransferase) were also under positive selection in the polar representatives (Figure 5A–D). The C-terminal domain of FtsZ had signatures of positive selection. Lauroyl/palmitoleoyl acyltransferase (Lpx) catalyses an essential step in the synthesis of lipid A of lipopolysaccharide (LPS) and is therefore involved in maintaining the function and stability of the outer membrane. In E. coli, two different orthologues of this gene are present. The lpxL (htrB) gene is expressed at high temperature and lpxP at low temperature (Carty et al, 1999), and they function to incorporate laureate or the monounsaturated palmitoleate into lipid A, respectively. The functionally relevant sequence differences between LpxL and LpxP are located in the N-terminal portion, which is the region under selection between the tropical and polar SAR11 phylotypes.
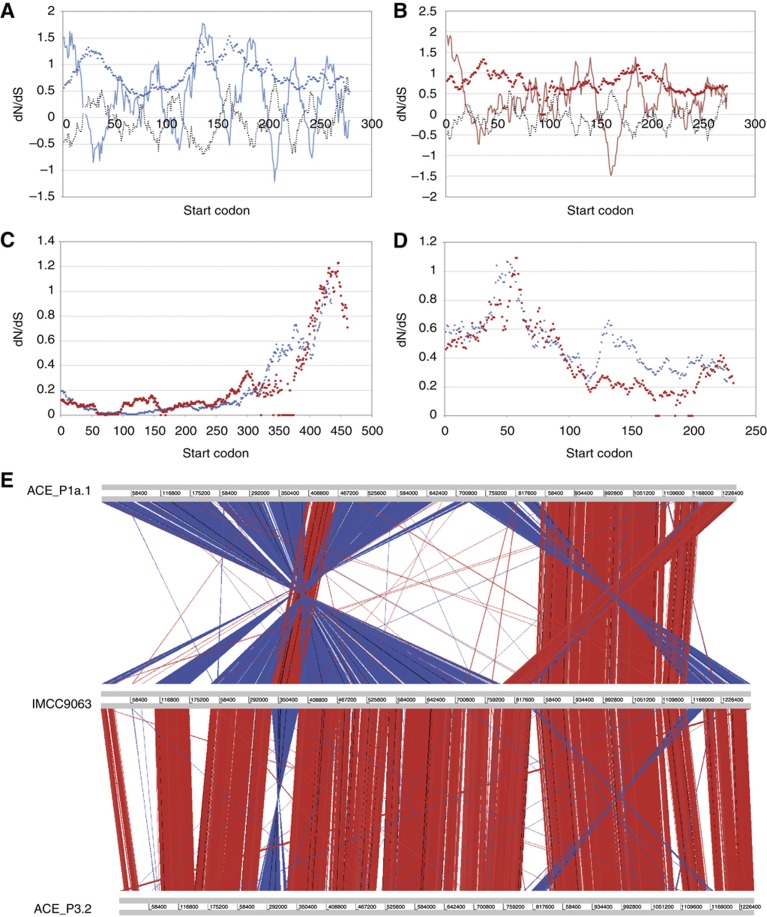
dN/dS ratios over the length of selected protein alignments, and genome synteny between phylotype P3.2 polar strains. (A) Putative porin (ACE_P1a.1 feature: 167717860). () dN/dS ratio over 60 codon sliding window, (
) Hydrophobicity index, (
) Antigenicity index. (B) Putative porin (ACE_P3.2 feature: 167933090). (
) dN/dS ratio over 60 codon sliding window, (
) Hydrophobicity index (
), Antigenicity index. (C) dN/dS ratio over a 60 codon sliding window for the FtsZ of ACE_P1a.1 (blue; feature: 167713888) and ACE_P3.2 (red; feature: 167824744). (D) dN/dS ratio over a 60 codon sliding window for lipid A biosynthesis lauroyl/palmitoleoyl acyltransferase of ACE_P1a.1 (blue; feature: 167719320) and ACE_P3.2 (red; feature: 167824936). (E) Genome synteny between ACE_P1a.1 mosaic genome (as depicted in Figure 3), Arctic IMCC9063, and ACE_P3.2 scaffolds rearranged according to the structure of the IMCC9063 genome (bottom).
Finally, the P3.2 Antarctic and Arctic genomes were strikingly similar to each other (Figure 5E) and the trends observed for ACE_P3.2 in gene content (312 genome-specific genes in IMCC9063), paralagous genes and positive selection were mirrored in IMCC9063 (Supplementary Table S4). Genes of particular note that were under positive selection in both ACE_P3.2 and IMCC9063 included peptidyl-prolyl-cis/trans-isomerase, and proteins involved in LPS biosynthesis, cell division and replication; all functions that could be linked to cold adaptation.
Discussion
Temperature-related phylogenetic and functional characteristics of SAR11 phylotypes
Our data identify that temperature and/or latitude have a highly significant role in shaping the community composition of SAR11 phylotypes over a global scale in both coastal and open-ocean surface waters. These two variables alone provide strong (>60%) and statistically significant explanatory power. The co-occurrence of different phylotypes from subgroups S1 and S2 in tropical (P1a.3 and P2.1), bipolar (P1a.1) and Antarctic (P2.2) waters (Figure 1) indicates that these subgroups have undergone adaptive radiation generating phylotypes that have distinct temperature preferences. At the same time, the co-existence of phylotypes from different subgroups in tropical and in polar waters indicates that these phylotypes compete effectively with each other in the same temperature environment. By being linked to ecological niche specialization (Carlson et al, 2009), these phylotypes effectively describe SAR11 ecotypes. Similarly, P1b and P3.1 also represent ecotypes preferentially adapted to co-existence with other SAR11 members in tropical waters, with P3.2 preferring polar waters. Overall, these phylotype distribution patterns indicate adaptive radiation has played an important role in the successful proliferation of SAR11 throughout the world’s ocean.
In addition to the distribution of ITS sequences being strongly correlated with environmental factors, several other factors are indicative of the phylotypes being ecotypes. A cultured representative of the P1a.3 phylotype, HTCC7211, which we detected primarily in tropical waters, displays a higher optimum growth temperature than representatives of the P1a.1 phylotype (HTCC1062, HTCC1002) (Wilhelm et al, 2007), which we determined dominates polar waters.
Importantly, the distinctiveness of the representative polar genomes to comparative tropical genomes provides genome-scale evidence for a strong latitudinal distribution of specific SAR11 phylotypes. Polar-specific genes may have been acquired or retained during the evolution of the polar strains or lost by the tropical strains. They included glycosyl-transferases and glycosyl synthetases involved in the synthesis of the cell wall; proteins that have previously been linked to cold adaptation in bacteria (Lauro et al, 2008) and archaea (Allen et al, 2009; Williams et al, 2010) due to the roles they have in modifying cell wall structure and integrity. These genes were also under positive selection (Supplementary Table S4). The positive selection in transporters, and representation of transporters in the polar-specific gene set may reflect adaptations to overcome the general inhibitory effect of low temperature on substrate transport systems (Wiebe et al, 1992), including protein structural changes to accommodate alterations in membrane fluidity. For example, a putative outer membrane porin displayed high dN/dS ratios both in transmembrane domains and in the N-terminal domain (Figure 5A and B) which provides ion-selectivity (Benz et al, 1989). The functional differences in Lpx may also relate to polar phylotypes producing unsaturated lipid A for growth at low temperature (Figure 5D). Similarly, for FtsZ (Figure 5C) the protein domain under positive selection has previously been shown to be essential for GTPase activity and ring assembly in E. coli by providing water polarizing residues during the protofilament formation during cell division (Oliva et al, 2004); an activity that is likely to be impaired at low temperature.
The similarity of the genes under positive selection between ACE_P3.2 and IMCC9063 further establish the overall distinctiveness of the genomes of representatives of the polar versus tropical biomes. In this regard, it is noteworthy that many of the geo-physico-chemico-properties of the aquatic systems where the two strains are from are very different: ACE_P3.2, Ace Lake, Antarctica, 68°S, 5000-year-old meromictic system with essentially no exogenous nutrient input, surface water low salinity (2%) with mineral concentrations several fold higher (e.g., copper 30-fold) or lower (e.g., calcium 3-fold) than seawater (Rankin et al, 1999; Lauro et al, 2011); IMCC9063, Dasan Korean Arctic Station, Norway, 79°N, oceanic coastal surface waters (Oh et al, 2011). Given these types of differences for organisms that are poles apart, the extent of genome similarity is striking.
Other effectors of SAR11 biogeography
Beyond temperature and latitude per se, many other factors are likely to influence the abundance of members of the SAR11 clade at a local level. Particularly in temperate and coastal zones the physical interactions of convergent water bodies with different histories, including rivers, may have a role in determining the final SAR11 community composition. Both Chesapeake (GS012) and Delaware (GS011) bays have very complex local hydrographic regimes with relatively extreme local variations in temperature and salinity, as well as large seasonal fluctuations (e.g., Shiah and Ducklow, 1994). Previous work in Chesapeake Bay has highlighted the presence of P1a.1, P1a.2 and P3.2 phylotypes (Kan et al, 2008). We identified these marine phylotypes, as well as P2.2, in GS012, GS011 and GS013.
Several time series analyses have also shown that the SAR11 clade as a whole (Carlson et al, 2009; Eiler et al, 2009; Gilbert et al, 2012), and the composition of subgroups (Brown et al, 2005; Carlson et al, 2009), varies in relative abundance in association with physical parameters (e.g., convective overturning—Morris et al, 2005; Carlson et al, 2009), environmental parameters (Brown et al, 2005; Carlson et al, 2009; Eiler et al, 2009; Gilbert et al, 2012), and with basin scale climatic events (Eiler et al, 2009). Despite methodological differences for studying SAR11 community composition, our data are concordant with many findings from these studies. Of note, at the Bermuda Atlantic Time Series (BATS) in the Sargasso Sea, pronounced vertical and seasonal patterns were observed in the distributions of three SAR11 subgroups: S1a, S1b and S2 (Carlson et al, 2009). S1a became more abundant than S1b in the surface waters (integrated 40m depth) 2 months after convective overturning events, and remained more abundant during the highly oligotrophic and high UV summer period. Our analysis shows P1a.3 is the dominant phylotype within the S1a subgroup in samples taken across a wide range of warm oligotrophic waters (Figure 1) indicating P1a.3 is likely the organism observed by Carlson et al (2009) and other studies (Wilhelm et al, 2007). Of further relevance, during periods of winter mixing at BATS (early January and late February), the S1b subgroup was most abundant (Carlson et al, 2009). In our analysis, S1b was most abundant in Sargasso Sea samples taken in February (Venter et al, 2004), and at an upwelling site (GS031) near Fernandina Island (Rusch et al, 2007). Deep mixing appears to have a significant effect on the relative abundance of S1b in a number of oceanic regions.
However, given the distributions of wind-driven upwelling/downwelling (i.e., Ekman pumping) and of mixed layer depths across the world’s ocean, upwelling or mixing effects alone do not provide the best explanation for the observed SAR11 phylotype distribution and abundance across the 128 samples analysed. The large-scale pattern of wind-driven vertical velocity consists of upward motion in cold high latitude regions and in the warm tropics, and downward motion in the subtropical gyres. If the phylotype distributions were strongly linked to the pattern of Ekman pumping, then we would expect a weak relationship with temperature (as both the warmest and coldest waters upwell, and waters of intermediate temperature downwell). Other factors, like eddies and convergent/divergent surface currents, can drive upwelling and downwelling, but there is no data with which to assess their global distribution. Similarly, the depth of the surface mixed layer and its seasonal variation is not a strong function of latitude or surface temperature. Deepest winter mixed layers are observed on the equatorward side of strong mid-latitude currents (the ‘mode’ waters), while relatively shallow mixed layers are observed in the tropics and polar latitudes. There is also a strong contrast in winter mixed layer depth between the North Pacific and North Atlantic, despite similar surface temperatures (e.g., Tomczak and Godfrey, 1994). Summer mixed layer depths are relatively constant from the tropics to high latitude while summer sea surface temperature is a strong function of latitude. The observed strong association between phylotypes and temperature/latitude argues against a strong dependence on the depth of the surface mixed layer.
It has been suggested that the differences in productivity in coastal versus open-ocean environments may be a primary driver in the distribution of organisms associated with phylotypes P1a.1 and P1a.3 (Schwalbach et al, 2010). This study (Schwalbach et al, 2010) examined the presence and abundance of genes associated with the glycolysis operon (which is present in the genome of HTCC1062, P1a.1 but not in HTCC7211, P1a.3) in 46 surface metagenomes, reporting the overrepresentation of these genes in coastal samples. In our analysis, on a global scale P1a.1 is predominant in polar regions and P1a.3 in tropical regions (Figure 1). Coastal versus open-ocean environments had a small but significant impact in temperate, but not in tropical or polar biomes, and this was caused primarily by the relative abundances of P3.2 and P1a.2. The overabundance of coastal metagenomes from temperate regions is therefore likely to bias analyses of SAR11 representation.
Chlorophyll did not have a strong role in defining the biogeography of SAR11 phylotypes (Supplementary Table S4). Although cold waters tended to contain higher chlorophyll levels than tropical waters, many of the Antarctic samples, typical of High Nutrient Low Chlorophyll regions, had low chlorophyll allowing us to de-convolute these parameters. Irrespective of chlorophyll levels, in cold water the polar phylotypes remained abundant, and in tropical waters the tropical phylotypes remained abundant.
Implications of SAR11 distribution for climate change
Given the global distributions of the SAR11 phylotypes examined here, we suggest they provide molecular markers that may aid in examining how physical oceanographic processes contribute to microbial community assembly, by for example, monitoring the influx of tropical phylotypes into polar regions. To assess the potential for changes in the biogeography of the SAR11 community, we compared the present distribution of sea surface temperature to climate model projections for the end of the century (Supplementary Figure S3). Both the 10 and 20°C isotherms are projected to shift towards the poles by the end of the century. The poleward shift of surface isotherms is larger in the eastern part of the ocean basins, and larger in the northern hemisphere than in the south. The area of the surface ocean with surface temperatures between 10 and 20°C and warmer than 20°C increases by 12.2 and 20.5 million km2, respectively, based on the mean of 15 climate models used in the IPCC 4th Assessment Report (Solomon et al, 2007). Assuming that the relationship between the distribution of SAR11 phylotypes and temperature does not change, we anticipate that the temperate and tropical phylotypes will expand at the expense of polar representatives.
We conclude that the adaptive radiation of SAR11 is linked to environmental parameters selecting for specific phylotypes of SAR11 at different latitudes that differ in gene content. While further studies will be required to tease apart all the selective pressures acting on these putative ecotypes, our data point to temperature playing a strong role in SAR11 radiation and diversification. Furthermore, our findings may now facilitate the development of oceanographic models that predict the effects of ocean temperature on the distribution and function of dominant marine heterotrophic bacteria.
Materials and methods
Antarctic metagenomes
Thirty-seven metagenomic data sets were generated from Southern Ocean and Antarctic marine samples collected during voyages of the Aurora Australis 2006/7, 2007/8 and 2008/9 and from under sea ice in the Ross Sea during 2009 and 2010 (Supplementary Table S2). Surface temperature, salinity and chlorophyll measurements, along with depth of water column were obtained using the underway line aboard the RV Aurora Australis. DNA extraction was performed at the University of New South Wales or at the J. Craig Venter Institute (JCVI), sequencing was performed using titanium 454 at the JCVI, and preprocessing of metagenomic data was carried out as described previously (Rusch et al, 2007; Lauro et al, 2011). The metagenomic data are publically available through the Community Cyber infrastructure for Advanced Microbial Ecology Research and Analysis website ( http://camera.calit2.net/) and the sequence read archive at NCBI ( http://www.ncbi.nlm.nih.gov/). Publically available metagenomic data sets from Antarctic lakes and marine environments were downloaded from the MG-Rast server ( http://metagenomics.nmpdr.org/).
Phylotype definition
The term ‘SAR11 clade’ is used to describe the entire collection of SAR11 type organisms, the term ‘subgroup’ (S) to describe a branch of the 16S rRNA gene tree (Table I; Figure 1; Supplementary Figure S1), and the term ‘phylotype’ (P) to describe the branches within subgroups defined using ITS sequences (Table I; Figure 1; Supplementary Figure S1). Subgroup and phylotype designations are based on previous work: Morris et al (2005) and Carlson et al (2009) for 16S rRNA gene-based SAR11 subgroups, and Garcia-Martinez and Rodriguez-Valera (2000) and Brown and Fuhrman (2005) for ITS-based phylotypes. The 16S rRNA gene tree in Supplementary Figure S1 was generated using the neighbour-joining algorithm in the software package ARB (Ludwig et al, 2004). Near full-length (>1300, bp) 16S rRNA gene sequences representing SAR11 subgroups (Morris et al, 2005) were obtained from Greengenes version 513274 (12 October 2010) alignment (DeSantis et al, 2006) and used to make the scaffold tree, and boostrap values were calculated for 1000 replicate trees using the programs Seqboot, DNAdist, Neighbour and Consense from the PHYLIP package (Felsenstein, 1993). Shorter sequences that also contained ITS regions (that were included in the ITS tree) were manually aligned and added using the maximum parsimony algorithm. The ITS trees in Supplementary Figure S1 were generated using neighbour-joining analysis of a 657 base-pair alignment containing 862 ITS sequences derived from SAR11 16-ITS clones and metagenomic data sets (Supplementary Table S1; Supplementary Figure S1). Individual SAR11 ITS sequences were 379–454bp in length. Bootstrap values were calculated as above. The annotated database and ITS alignment files are available from the authors upon request.
SAR11 subgroup 1a (S1a) consists of a number of phylotypes (Garcia-Martinez and Rodriguez-Valera, 2000; Brown and Fuhrman, 2005) we now define as P1a.1, P1a.2 and P1a.3. The 16S rRNA gene sequences associated with these three phylotypes all branch closely (~98–100% sequence similarity) with the first described species Candidatus Pelagibacter ubique, isolated off the coast of Oregon (Rappe et al, 2002). The separation of P1a.1 sequences from other ITS sequences was first identified using sequences from Antarctic waters (Garcia-Martinez and Rodriguez-Valera, 2000) and named SAR11-A. Ca. P. ubique, along with the isolates HTCC1002 (Rappe et al, 2002), HTCC8022, HTCC8010 and HTCC8048 (Stingl et al, 2007), all isolated from cool waters off the coast of Oregon, belong to P1a.1, as do ITS sequences originating predominantly from surface waters in the Arctic (Supplementary Table S1). A mosaic genome from metagenome data from Ace Lake, Antarctica, ACE_P1a.1 also belongs to this phylotype. P1a.2 was previously defined from ITS sequences originating primarily from the temperate waters at the San Pedro Ocean Time Series, off California and from the Mediterranean Sea. Given the clustering of sequences from different environments, along with moderate bootstrap support (44%) this group of sequences was previously designated as a separate phylotype named S1a (Brown and Fuhrman, 2005). P1a.2 also contains sequences from other Arctic, sub-Arctic and temperate surface waters, along with the strains HTCC8038, HTCC8041, HTCC8045, HTCC8046 and HTCC8049 which were isolated off the coast of Oregon. The phylotypes P1a.1 and P1a.2 are distinguished by the conserved region starting at position 422 in the alignment:
P1a.1 --ATCATTTATATCAATATCTATATCCGAACATT-AAAA-GT-TATTATTTA
P1a.2 TAATAATTAATATCAATATCTATATCCGAACATTAGTAATGT-TATTAGCTA
P1a.3 was originally defined from ITS sequences from temperate and tropical waters including the San Pedro Ocean Time Series, and Sargasso Sea and was previously named S2 (Brown and Fuhrman, 2005). P1a.3 also contains sequences from strains HTCC8051 and HTCC8047 isolated off the coast of Oregon, and HTCC7211, HTCC7215, HTCC7216 and HTCC7217, isolated from warm waters off Bermuda (Stingl et al, 2007). The genome sequence for HTCC7211 is available but unpublished (GenBank Accession: EF616619). SAR11 subgroup S1b contains the 16S rRNA gene sequence from the original SAR11 clone from the Sargasso Sea, and includes a single phylotype P1b. No cultures or genome sequences appear to be available for this phylotype. SAR11 subgroup 2 contains three phylotypes. P2.1 contains surface water sequences from temperate and tropical waters and was originally designated as S3 (Brown and Fuhrman, 2005). P2.2 was initially defined based on ITS sequences from the Antarctic and named SAR11-A21 (Garcia-Martinez and Rodriguez-Valera, 2000). P2.3 was defined based on ITS sequences from the deep waters in the Mediterranean Sea (Garcia-Martinez and Rodriguez-Valera, 2000) and originally named SAR11-D (deep). It contains sequences from waters of 70–3000, m depth (the majority from deep water) from tropical, temperate and polar biomes. No cultures or genome sequences appear to be available for these phylotypes. SAR11 subgroup S3 contains two marine phylotypes along with freshwater SAR11 LD group that are not discussed in our study. P3.1 includes the strain/genome HIMB114, isolated from warm coastal waters of Kanaohe Bay, Hawaii. P3.2 includes the Arctic strain/genome, IMCC9063, isolated from seawater off the coast of Svalbard, Norway (Oh et al, 2011) and the mosaic genome from Ace Lake, Antarctica, ACE_P3.2.
Other potential phylotypes were determined from our ITS phylogeny using our database. Several sequences that branch deeply near the SAR11 subgroup S1b (P1b.a, NA1, NA2 in Figure 1) are defined as SAR11 ITS sequences in GENBANK but generally lack a clear connection to the 16S rRNA gene phylogeny (i.e., a lack of 16S-ITS sequences). Only P1b.a contains a 16S-ITS linked clone (SPOTS_May03_160m_16) which is associated with the S1b subgroup (Supplementary Figure S1, upper panel). Hence the group of sequences within this collapsed node were designated as P1b.a (Figure 1). All sequences in collapsed nodes NA1 and NA2 are derived from deep Arctic waters (3000, m in the Greenland Sea and 500m in the Sub-Arctic North Pacific). The sequences for P1b.a, NA1 and NA2 were included in our BLAST analysis but received no hits from the metagenomes we analysed and were thus excluded from discussion.
Determination of phylotype abundance in metagenomic samples and biogeography
The 862 SAR11 ITS sequences were used to generate an annotated sequence database (each ITS sequence name was annotated with its phylotype designation) against which all metagenomic data sets were compared by blastn (e=0.0001). The phylotype affiliation of the high-scoring pairs for metagenomic sequences that matched the database with >95% sequence identity over at least a continuous 200 base-pair region was recorded, resulting in 2983 records. This multivariate data set (relative abundance of SAR11 phylotypes at each location) was used in further statistical analysis.
DistLM and dbRDA methods were used to analyse and model the relationship between the multivariate SAR11 ITS data set and available predictor variables. Not all variables were available for all samples. To make use of the most available data, missing data for salinity and chlorophyll were estimated using the expectation-maximization algorithm. However, all patterns reported were checked for consistency against the subset of 79 metagenomes for which all variables were present (Supplementary Tables S3 and S4). Variables were normalized (the values for each variable had their mean subtracted and were then divided by their standard deviation) to reduce the effect of different measurement scales. Bray-Curtis similarity matrices were generated from normalized, square root transformed data composed of the relative abundance of each SAR11 phylotype in each sample. MDS, ANOSIM, DistLM, dbRDA and expectation-maximization of variables analysis were performed using the Primer V6+PERMANOVA+ software (Clarke and Gorley, 2006).
Genome assembly and annotation
Initial shotgun metagenomic hybrid assemblies were generated as previously described (Ng et al, 2010; DeMaere et al, 2011; Lauro et al, 2011) from the 0.1μm fraction of the 5
m depth sample from Ace lake, Antarctica (Lauro et al, 2011) using a combination of pyrosequencing and Sanger paired-end reads. Assemblies were manually inspected and validated using AMOS 3.1.0 (Phillippy et al, 2008) and Hawkeye 2.0 (Schatz et al, 2007). The mosaic draft genome of ACE_P1a.1 (~1.26
Mbp) contained 79 contigs (N50=44.3
kbp) that were assembled into 12 scaffolds (N50=150.2
kbp) with 87.8% of the total base-pairs in contigs larger than 10
kbp. The mosaic draft genome of ACE_P3.2 (~1.27
Mbp) contained 129 contigs (N50=15.2
kbp) that were assembled into 8 scaffolds (N50=329.6
kbp) with 73.1% of total base-pairs in contigs larger than 10
kbp. The scaffolds containing a 16S rRNA marker gene were used as phylogenetic anchors for manually binning the resulting scaffolds using a combination of paired-end reads, GC%, tetranucleotide frequencies and phylogenetic congruence using custom perl scripts. This approach yielded 12 scaffolds that could be confidently assigned to ACE_P1a.1 and 8 scaffolds that could be confidently assigned to ACE_P3.2. The scaffolds were annotated with the automatic annotation pipeline SHAP (DeMaere et al, 2011), as previously described (Lauro et al, 2011).
Genome alignment and recruitment
The genomic scaffolds were oriented and joined in alignments to known reference genomes with the 6-frame stop-codon spacer ‘NNNNCACACACTTAATTAATTAAGTGTGTGNNNN’ using the custom perl script scaffolding.pl to create a contiguous pseudomolecule. Whole genome alignments were performed by comparing the pseudomolecule using blastn (run with standard parameters and the -m8 flag and only matches of at least 30 nucleotides or longer used) and ACT (Carver et al, 2005) against reference SAR11 genomes. After removing all N’s from the query sequence, ANI and PCD between representative genomes of the SAR11 clade were computed using 1020 nucleotide fragments (Goris et al, 2007) by employing custom perl scripts. blastn was run using settings as previously described (Goris et al, 2007): parameters were used at the default settings, except X=150 (where X is the drop-off value for gapped alignment), q=21 (where q is the penalty for nucleotide mismatch) and F=F (where F is the filter for repeated sequences). Genome recruitment plots were obtained by comparing marine metagenomes using blastn (e-value <1e−10, sequence identity >90%) and visualized with the custom perl and R script plot_coverage.pl using a sliding window size of 1000, bp and a natural logarithmic scale for recruitment depth. We reported the recruitment of four representative metagenomes from the different temperature biomes (polar, temperate and tropical). The metagenome data sets were chosen because they were the most similar to each other in terms of sampling strategy, sequencing technology and sample size: Ace Lake polar (GS232, 539536 reads), Southern Ocean polar (GS362, 508628 reads), temperate (GS368, 512395 reads) and tropical (S_35155+S_35163, 576392 reads). Recruitment of metagenome data from other sampling sites gave similar results. The recruitment depth averaged over the length of each reference genome was used as a proxy for genome abundance in each data set. For statistical analyses an additional polar metagenome was included (GS358, 491340 reads) so that the polar data could be compared with tropical plus temperate data with equivalent data set sizes, and compared using a two-tailed t-test.
Detection of homology, COG identification and analysis
The orthologous predicted proteins were identified from each pair of SAR11 genomes using the reciprocal smallest distance (RSD) algorithm (Wall and Deluca, 2007) with a blastp e-value threshold of 1e−15 and a sequence divergence for the alignment of 0.5. The protein sequences that were not identified as orthologues during the first RSD run were then subjected to subsequent rounds of reciprocal comparisons and added to the list of paralogues until no more homologues could be identified. The remaining list of protein-encoding genes was considered genome specific. The list of genome-specific proteins was searched against version 2 of the database of all metagenomic 454 reads in CAMERA (Sun et al, 2011) (excluding Ace Lake metagenome data) with tblastn (e<1e−50 and >100 amino acids alignment). The hits were subdivided based on water temperature and statistically significant differences were analysed by 1000 resamplings with replacement of the blast hits at a confidence level of 99%. Briefly, 1000 hits were randomly sampled from all the tblastn hits for ACE_P1a.1 to the metagenomes in CAMERA. This subsample was compared with a similar subsample obtained from the hits of HTCC7211 and the difference in number of hits (binned according to water temperature) was calculated. This process was repeated 1000 times and the median difference in number of hits was calculated for each water temperature bin. To verify that this difference was unlikely to happen by random chance, this process was repeated except that each of the 1000 tblastn hits were sampled from the combined hits of HTCC7211 and ACE_P1a.1. This process was once again repeated 1000 times and the results were ordered from the lowest to the largest difference in hits for each temperature bin. From the combined data sets, the low confidence cutoff for 99% significance was the 10th median difference and the high cutoff was the 991st. If the results for the test comparison fell outside this interval, then the difference was considered statistically significant. This process was also followed for comparisons of ACE_P3.2 to HIMB114. Each protein was assigned to a COG as described previously (Lauro et al, 2011). Overrepresentation of specific COG categories compared with the genome background was verified by a resampling method as previously described (Allen et al, 2009; Lauro et al, 2011) over a confidence interval of 97% using the custom perl script COG_scrambler.pl.
Detection of proteins under positive selection
Each protein on the list of orthologues was aligned with clustalW (Thompson et al, 1994) with protein pairs that differed by >120 amino acids in length discarded from the analysis. The alignments were then back-translated to the corresponding nucleotide coding sequence using RevTans ( http://www.cbs.dtu.dk/services/RevTrans/download.php) and the dN/dS ratios were computed by the method of Nei-Gojobori (Nei and Gojobori, 1986) using yn00 from the PAML package (Yang, 2007) over the whole alignment and over a 60-codon sliding window. The highest dN/dS ratio was recorded. Orthologous protein pairs were considered under positive selection only if dN/dS ratio was >1 over at least one window of 60 amino acids. For the analysis of predicted membrane proteins, the protein topology was inferred by computing antigenicity (Hopp and Woods, 1983) and hydrophobicity (Eisenberg et al, 1984) indexes over a sliding window of 60 amino acids.
Climate models
Fifteen climate models from the Coupled Model Intercomparison Project 3 (CMIP3) (as used in the IPCC 4th Assessment Report; Solomon et al, 2007) were used to predict future shifts in the distribution of sea surface temperature in 1990 (the mean for the period 1980–1999, from the 20th century simulation runs) compared with the distribution projected for 2090 (mean from 2080 to 2099), under the SRES A2 emissions scenario (Nakicenvoic and Swart, 2000). Drift was removed from the climate model output by subtracting a linear trend over 150 years of the control run (pictrl).
Supplementary Material
Acknowledgments
We acknowledge technical support for computing infrastructure and software development from Intersect, and in particular assistance from Joachim Mai, and acknowledge Matthew Lewis from the JCVI for his assistance with DNA sequencing. This work was supported by the Australian Research Council and the Australian Antarctic Division. Funding for sequencing was provided by the Gordon and Betty Moore Foundation to the JCVI.
Author contributions: RC initiated the research program. RC, TT, FML, MVB and JMH designed and performed the field expeditions. AA, JMH, CAP and ML undertook activities leading to the generation of the DNA sequence data. MVB, FML, SSR, LM, DW and RC conceived, designed or performed the data analysis. MVB, RC and FML wrote, and SSR, TT, MZD, MJR, LM and JAF contributed to the manuscript.
Footnotes
The authors declare that they have no conflict of interest.
References
- Allen M, Lauro FM, Williams TJ, Burg D, Siddiqui KS, De Francisci D, Chong KW, Pilak O, Chew HH, De Maere MZ, Ting L, Katrib M, Ng C, Sowers KR, Galperin MY, Anderson IJ, Ivanova N, Dalin E, Martinez M, Lapidus A et al. (2009) The genome sequence of the psychrophilic archaeon, Methanococcoides burtonii: the role of genome evolution in cold-adaptation. ISME J 3: 1012–1035 [Abstract] [Google Scholar]
- Benz R, Schmid A, Van der Ley P, Tommassen J (1989) Molecular basis of porin selectivity: membrane experiments with OmpC-PhoE and OmpF-PhoE hybrid proteins of Escherichia coli K-12. Biochim Biophys Acta 981: 8–14 [Abstract] [Google Scholar]
- Brown MV, Fuhrman JA (2005) Marine bacterial microdiversity as revealed by internal transcribed spacer analysis. Aquat Microb Ecol 41: 15–23 [Google Scholar]
- Brown MV, Schwalbach MS, Hewson I, Fuhrman JA (2005) Coupling 16S-ITS clone libraries and automated ribosomal intergenic spacer analysis to show marine microbial diversity: development and application to a time series. Environ Microbiol 7: 1466–1479 [Abstract] [Google Scholar]
- Carlson CA, Morris R, Parsons R, Treusch AH, Giovannoni SJ, Vergin K (2009) Seasonal dynamics of SAR11 populations in the euphotic and mesopelagic zones of the northwestern Sargasso Sea. ISME J 3: 283–295 [Abstract] [Google Scholar]
- Carty SM, Sreekumar KR, Raetz CRH (1999) Effect of cold shock on lipid A biosynthesis in Escherichia coli: induction at 12°C of an acyltransferase specific for palmitoleoyl-acyl carrier protein. J Biol Chem 274: 9677–9685 [Abstract] [Google Scholar]
- Carver TJ, Rutherford KM, Berriman M, Rajandream MA, Barrell BG, Parkhill J (2005) ACT: the Artemis Comparison Tool. Bioinformatics 21: 3422–3423 [Abstract] [Google Scholar]
- Clarke KR, Gorley RN (2006) PRIMER v6: User Manual/Tutorial Plymouth: PRIMER-E, [Google Scholar]
- DeMaere MZ, Lauro FM, Thomas T, Yau S, Cavicchioli R (2011) Simple high-throughput annotation pipeline (SHAP). Bioinformatics 27: 2431–2432 [Abstract] [Google Scholar]
- DeSantis TZ, Hugenholtz P, Larsen N, Rojas M, Brodie EL, Keller K, Huber T, Dalevi D, Hu P, Andersen GL (2006) Greengenes, a chimera checked 16S rRNA gene database and workbench compatible with ARB. Appl Environ Microbiol 72: 5069–5072 [Europe PMC free article] [Abstract] [Google Scholar]
- Eiler A, Hayakawa H, Church MJ, Karl DM, Rappé MS (2009) Dynamics of the SAR11 bacterioplankton lineage in relation to environmental conditions in the oligotrophic North Pacific subtropical gyre. Environ Microbiol 11: 2291–2300 [Abstract] [Google Scholar]
- Eisenberg D, Weiss RM, Terrwilliger TC (1984) The hydrophobic moment detects periodicity in protein hydrophobicity. Proc Natl Acad Sci USA 81: 140–144 [Europe PMC free article] [Abstract] [Google Scholar]
- Felsenstein J (1993) PHYLIP (Phylogeny Inference Package) Version 3.5c Distributed by the author. Department of Genetics, University of Washington, Seattle [Google Scholar]
- Field KG, Gordon D, Wright T, Rappé M, Urback E, Vergin K, Giovannoni SJ (1997) Diversity and depth-specific distribution of SAR11 cluster rRNA genes from marine planktonic bacteria. Appl Environ Micro 63: 63–70 [Europe PMC free article] [Abstract] [Google Scholar]
- Follows MJ, Dutkiewicz S, Grant S, Chisholm SW (2007) Emergent biogeography of microbial communities in a model ocean. Science 315: 1843–1846 [Abstract] [Google Scholar]
- Garcia-Martinez J, Rodriguez-Valera F (2000) Microdiversity of uncultured marine prokaryotes: the SAR11 cluster and the marine archaea of group I. Mol Ecol 9: 935–948 [Abstract] [Google Scholar]
- Gilbert JA, Steele JA, Caporaso JG, Steinbruck L, Reeder J, Temperton B (2012) Defining seasonal marine microbial community dynamics. ISME J 6: 298–308 [Europe PMC free article] [Abstract] [Google Scholar]
- Giovannoni SJ, Tripp HJ, Givan S, Podar M, Vergin KL, Baptista D, Bibbs L, Eads J, Richardson TH, Noordewier M, Rappé MS, Short JM, Carrington JC, Mathur EJ (2005) Genome streamlining in a cosmopolitan oceanic bacterium. Science 309: 1242–1245 [Abstract] [Google Scholar]
- Goris J, Konstantinidis KT, Klappenbach JA, Coenye T, Vandamme P, Tiedje JM (2007) DNA–DNA hybridization values and their relationship to whole-genome sequence similarities. Int J Syst Evol Microbiol 57: 81–91 [Abstract] [Google Scholar]
- Hopp TP, Woods KR (1983) A computer program for predicting protein antigenic determinants. Mol Immunol 20: 483–489 [Abstract] [Google Scholar]
- Johnson ZI, Zinser ER, Coe A, McNulty NP, Woodward EMS, Chisholm SW (2006) Niche partitioning among Prochlorococcus ecotypes along ocean-scale environmental gradients. Science 311: 1737–1740 [Abstract] [Google Scholar]
- Kan J, Evans SE, Chen F, Suzuki MT (2008) Novel estuarine bacterioplankton in rRNA operon libraries from the Chesapeake Bay. Aquat Microb Ecol 51: 55–66 [Google Scholar]
- Lauro FM, DeMaere MZ, Yau S, Brown MV, Ng C, Wilkins D, Raftery MJ, Gibson JA, Andrews-Pfannkoch C, Lewis M, Hoffman JM, Thomas T, Cavicchioli R (2011) An integrative study of a meromictic lake ecosystem in Antarctica. ISME J 5: 879–895 [Europe PMC free article] [Abstract] [Google Scholar]
- Lauro FM, Tran K, Vezzi A, Vitulo N, Valle G, Bartlett DH (2008) Large-scale transposon mutagenesis of Photobacterium profundum SS9 reveals new genetic loci important for growth at low temperature and high pressure. J Bacteriol 190: 1699–1709 [Europe PMC free article] [Abstract] [Google Scholar]
- Ludwig W, Strunk O, Westram R, Richter L, Meier H, Yadhukumar, Buchner A, Lai T, Steppi S, Jobb G, Förster W, Brettske I, Gerber S, Ginhart AW, Gross O, Grumann S, Hermann S, Jost R, König A, Liss T et al. (2004) ARB: a software environment for sequence data. Nucleic Acids Res 32: 1363–1371 [Europe PMC free article] [Abstract] [Google Scholar]
- Morris RM, Rappé MS, Connon SA, Vergin KL, Siebold WA, Carlson CA (2002) SAR11 clade dominates ocean surface bacterioplankton communities. Nature 420: 806–810 [Abstract] [Google Scholar]
- Morris RM, Vergin KL, Cho JC, Rappé MS, Carlson CA, Giovannoni SJ (2005) Temporal and spatial response of bacterioplankton lineages to annual convective overturn at the Bermuda Atlantic Time-Series Study Site. Limnol Oceanogr 50: 1687–1696 [Google Scholar]
- Nakicenvoic N, Swart R (eds) (2000) Special report on emissions scenarios. A Special Report of Working Group III of the Intergovernmental Panel on Climate Change Cambridge, UK: Cambridge University Press, [Google Scholar]
- Nei M, Gojobori T (1986) Simple methods for estimating the numbers of synonymous and nonsynonymous nucleotide substitutions. Mol Biol Evol 3: 418–426 [Abstract] [Google Scholar]
- Ng C, DeMaere MZ, Williams TJ, Lauro FM, Raferty M, Gibson JAE (2010) Metaproteogenomic analysis of a dominant green sulfur bacterium from Ace Lake, Antarctica. ISME J 4: 1002–1019 [Abstract] [Google Scholar]
- Oh HM, Kang I, Lee K, Jang Y, Lim S, Cho JC (2011) Complete genome sequence of strain IMCC9063, belonging to SAR11 subgroup 3, isolated from the Arctic Ocean. J Bacteriol 193: 3380 [Europe PMC free article] [Abstract] [Google Scholar]
- Oliva MA, Cordell SC, Lowe J (2004) Structural insights into FtsZ protofilament formation. Nat Struct Mol Biol 11: 1243–1250 [Abstract] [Google Scholar]
- Phillippy AM, Schatz MC, Pop M (2008) Genome assembly forensics: finding the elusive mis-assembly. Genome Biol 9: R55. [Europe PMC free article] [Abstract] [Google Scholar]
- Rankin LM, Gibson JAE, Franzmann PD, Burton HR (1999) The chemical stratification and microbial communities of Ace Lake, Antarctica: a review of the characteristics of a marine-derived meromictic lake. Polarforschung 66: 35–52 [Google Scholar]
- Rappe MS, Connon SA, Vergin KL, Giovannoni SJ (2002) Cultivation of the ubiquitous SAR11 marine bacterioplankton clade. Nature 418: 630–633 [Abstract] [Google Scholar]
- Rodríguez-Ezpeleta N, Embley TM (2012) The SAR11 group of Alpha-Proteobacteria is not related to the origin of mitochondria. PLoS ONE 7: e30520. [Europe PMC free article] [Abstract] [Google Scholar]
- Rusch D, Halpern AL, Sutton G, Heidelberg KB, Williamson S, Yooseph S (2007) The Sorcerer II Global Ocean sampling expedition: Northwest Atlantic through Eastern Tropical Pacific. PLos Biol 5: e77. [Europe PMC free article] [Abstract] [Google Scholar]
- Schatz MC, Phillippy AM, Shneiderman B, Salzberg SL (2007) Hawkeye: an interactive visual analytics tool for genome assemblies. Genome Biol 8: R34. [Europe PMC free article] [Abstract] [Google Scholar]
- Schwalbach MS, Tripp HJ, Steindier L, Smith DP, Giovannoni SJ (2010) The presence of the glycolysis operon in SAR11 genomes is positively correlated with ocean productivity. Environ Microbiol 12: 490–500 [Abstract] [Google Scholar]
- Shiah FK, Ducklow HW (1994) Temperature regulation of heterotrophic bacterioplankton abundance, production, and specific growth rate in Chesapeake Bay. Limnol Oceanogr 39: 1243–1258 [Google Scholar]
- Solomon S, Qin D, Manning M, Chen Z, Marquis M, Averyt KB (2007) Climate change 2007: the physical science basis. Contribution of Working Group I to the Fourth Assessment Report of the Intergovernmental Panel on Climate Change Cambridge, UK and New York, NY, USA: Cambridge University Press, [Google Scholar]
- Stingl U, Tripp HJ, Giovannoni SJ (2007) Improvements of high-throughput culturing yielded novel SAR11 strains and other abundant marine bacteria from the Oregon coast and the Bermuda Atlantic Time Series study site. ISME J 1: 361–371 [Abstract] [Google Scholar]
- Sun S, Chen J, Li W, Altintas I, Lin A, Peltier S, Stocks K, Allen EE, Ellisman M, Grethe J, Wooley J (2011) Community cyberinfrastructure for advanced microbial ecology research and analysis: the CAMERA resource. Nucleic Acids Res 39: D546–D551 [Europe PMC free article] [Abstract] [Google Scholar]
- Thompson JD, Higgins HG, Gibson TJ (1994) CLUSTAL W: improving the sensitivity of progressive multiple sequence alignment through sequence weighting, position-specific gap penalties and weight matrix choice. Nucleic Acids Res 22: 4673–4680 [Europe PMC free article] [Abstract] [Google Scholar]
- Thrash JC, Boyd A, Huggett MJ, Grote J, Carini P, Yoder RJ, Robbertse B, Spatafora JW, Rappé MS, Giovannoni SJ (2011) Phylogenomic evidence for a common ancestor of mitochondria and the SAR11 clade. Sci Rep 1: 13. [Europe PMC free article] [Abstract] [Google Scholar]
- Tomczak M, Godfrey JS (1994) Regional Oceanography: An Introduction Oxford: Pergamon, 422pp [Google Scholar]
- Venter JC, Remington K, Heidelberg JF, Halpern AL, Rusch D, Eisen JA (2004) Environmental genome shotgun sequencing of the Sargasso Sea. Science 304: 66–74 [Abstract] [Google Scholar]
- Viklund J, Ettema TJ, Andersson SG (2012) Independent genome reduction and phylogenetic reclassification of the oceanic SAR11 clade. Mol Biol Evol 29: 599–615 [Abstract] [Google Scholar]
- Wall DP, Deluca T (2007) Ortholog detection using the reciprocal smallest distance algorithm. Methods Mol Biol 396: 95–110 [Abstract] [Google Scholar]
- Wiebe WJ, Sheldon WM, Pomeroy LR (1992) Bacterial growth in the cold: evidence for an enhanced substrate requirement. Appl Environ Microbiol 58: 359–364 [Europe PMC free article] [Abstract] [Google Scholar]
- Wilhelm LJ, Tripp HJ, Givan SA, Smith DP, Giovannoni SJ (2007) Natural variation in SAR11 marine bacterioplankton genomes inferred from metagenomic data. Biol Direct 2: 27. [Europe PMC free article] [Abstract] [Google Scholar]
- Williams TJ, Burg DW, Ertan H, Raferty MJ, Poljak A, Guilhaus M, Pilak O, Cavicchioli R (2010) Global proteomic analysis of the insoluble, soluble and supernatant fractions of the psychrophilic archaeon Methanococcoides burtonii Part I: the effect of growth temperature. J Prot Res 9: 640–652 [Abstract] [Google Scholar]
- Yang Z (2007) PAML 4: phylogenetic analysis by maximum likelihood. Mol Biol Evol 24: 1586–1591 [Abstract] [Google Scholar]
Articles from Molecular Systems Biology are provided here courtesy of Nature Publishing Group
Full text links
Read article at publisher's site: https://doi.org/10.1038/msb.2012.28
Read article for free, from open access legal sources, via Unpaywall:
https://onlinelibrary.wiley.com/doi/pdfdirect/10.1038/msb.2012.28
Citations & impact
Impact metrics
Article citations
Endemic, cosmopolitan, and generalist taxa and their habitat affinities within a coastal marine microbiome.
Sci Rep, 14(1):22408, 28 Sep 2024
Cited by: 0 articles | PMID: 39333653 | PMCID: PMC11437011
New insights into the structure and function of microbial communities in Maxwell Bay, Antarctica.
Front Microbiol, 15:1463144, 04 Sep 2024
Cited by: 0 articles | PMID: 39296290 | PMCID: PMC11408308
Ecological associations distribution modelling of marine plankton at a global scale.
Philos Trans R Soc Lond B Biol Sci, 379(1909):20230169, 22 Jul 2024
Cited by: 2 articles | PMID: 39034696 | PMCID: PMC11293856
A marine heatwave drives significant shifts in pelagic microbiology.
Commun Biol, 7(1):125, 24 Jan 2024
Cited by: 1 article | PMID: 38267685 | PMCID: PMC10808424
Structure and dispersion of the conjugative mobilome in surface ocean bacterioplankton.
ISME Commun, 4(1):ycae059, 01 Jan 2024
Cited by: 0 articles | PMID: 38770060 | PMCID: PMC11104534
Go to all (119) article citations
Data
Data behind the article
This data has been text mined from the article, or deposited into data resources.
BioStudies: supplemental material and supporting data
Nucleotide Sequences
- (1 citation) ENA - EF616619
Similar Articles
To arrive at the top five similar articles we use a word-weighted algorithm to compare words from the Title and Abstract of each citation.
d(N)/d(S) does not show positive selection drives separation of polar-tropical SAR11 populations.
Mol Syst Biol, 8:625, 01 Jan 2012
Cited by: 8 articles | PMID: 23169001 | PMCID: PMC3531906
Red Sea SAR11 and Prochlorococcus Single-Cell Genomes Reflect Globally Distributed Pangenomes.
Appl Environ Microbiol, 85(13):e00369-19, 17 Jun 2019
Cited by: 5 articles | PMID: 31028022 | PMCID: PMC6581160
Streamlining and core genome conservation among highly divergent members of the SAR11 clade.
mBio, 3(5):e00252-12, 18 Sep 2012
Cited by: 156 articles | PMID: 22991429 | PMCID: PMC3448164
The unique metabolism of SAR11 aquatic bacteria.
J Microbiol, 51(2):147-153, 27 Apr 2013
Cited by: 23 articles | PMID: 23625213
Review