Abstract
Free full text

Evolutionary conservation of codon optimality reveals hidden signatures of co-translational folding
Abstract
The choice of codons can influence local translation kinetics during protein synthesis. Whether codon preference is linked to co-translational regulation of polypeptide folding remains unclear. Here, we derive a revised translational efficiency scale that incorporates the competition between tRNA supply and demand. Applying this scale to ten closely related yeasts, we uncover the evolutionary conservation of codon optimality in eukaryotes. This analysis reveals universal patterns of conserved optimal and nonoptimal codons, often in clusters, which associate with the secondary structure of the translated polypeptides independent of the levels of expression. Our analysis suggests an evolved function for codon optimality in regulating the rhythm of elongation to facilitate co-translational polypeptide folding, beyond its previously proposed role of adapting to the cost of expression. These findings establish how mRNA sequences are generally under selection to optimize the co-translational folding of corresponding polypeptides.
Introduction
The translational efficiency of individual codons directly modulates the kinetics of protein synthesis1. Optimal codons are thought to be translated both faster and more accurately2, 3. In turn, nonoptimal codons can slow down protein synthesis. Due to the degeneracy of the genetic code, all amino acids except methionine and tryptophan are encoded by both optimal and nonoptimal codons. The evolutionary forces that shape the codon bias, i.e. the unequal usage of synonymous codons, are the focus of intense study. In particular, the pressure to maintain nonoptimal codons is unclear. One attractive hypothesis is that nonoptimal codons slow translation for biologically relevant functions, such as facilitating co-translational folding by allowing the nascent chain more time to develop native-like structure4. Indeed, a link between the mRNA sequences and in vivo folding of the encoded proteins has long been suggested5. For instance, synonymous substitutions reducing translational efficiency have been found to alter folding6, 7, and subsequent function8 of the translated polypeptides. This supports the suggestion that protein synthesis is directly attuned to the co-translational folding9–13. However, no universal correlation across organisms was found between the position of nonoptimal codons and the location of structural units14 or domain boundaries15, or between synonymous codon usage and protein secondary structural elements16. For S. cerevisiae, a codon preference in relation to protein secondary structure could only be found for the amino acids glycine in loops and threonine in helices15. Thus, beyond a few individual examples, a global view linking codon optimality to nascent chain folding in vivo remains elusive.
The classification into optimal and nonoptimal codons reflects the important role of tRNAs on the rate of protein synthesis. During translation, specific tRNAs recognize the codons in the mRNA and deliver the corresponding amino acids to the ribosome17 (Fig. 1a). The abundance of tRNAs varies markedly in the cellular pool and is strongly correlated to their gene copy numbers in the genome18. Since tRNA gene redundancy is a critical factor explaining the correlation between translational selection and codon bias19, a scale of codon-specific translational efficiencies has been devised based on the relative abundance of tRNAs as well as selective constraints on codon-tRNA pairings for the “wobble” non-Watson-Crick interactions19. Codons that are over-represented in highly expressed genes are also recognized by the most abundant tRNAs, and are thus denoted as optimal20.
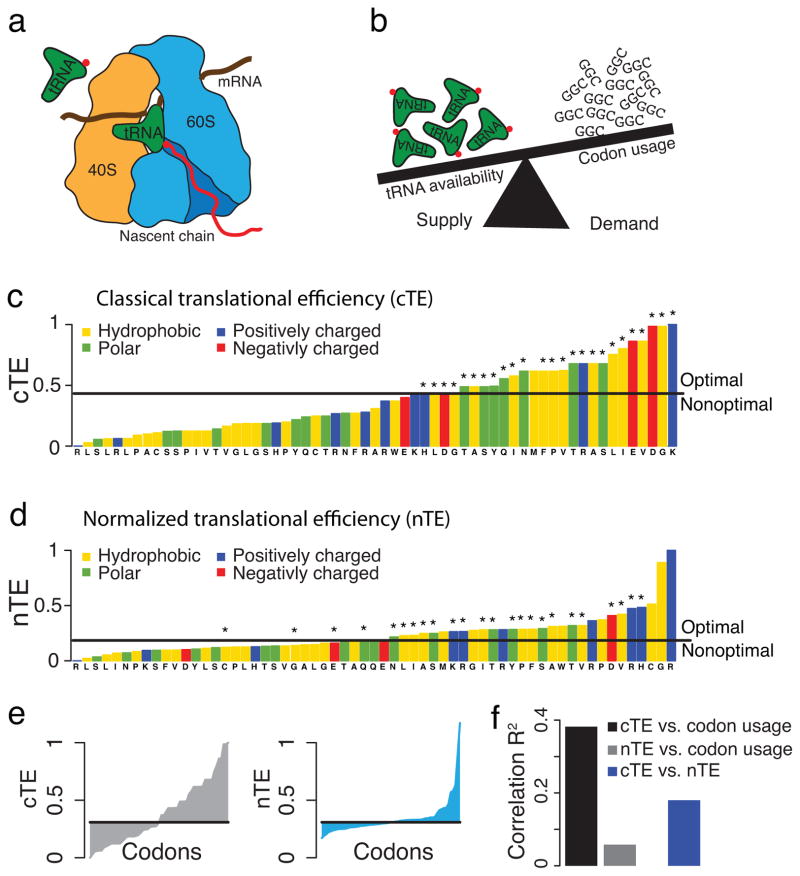
A normalized translational efficiency scale that balances tRNA supply and demand. (a) Charged tRNAs recognize the codons in the mRNA and deliver the corresponding amino acid for elongation of the nascent chain. (b) The competition for tRNAs depends on both their availability in the cellular tRNA pool (supply), and the usage of the corresponding codons in the cell (demand). (c) The classical translational efficiency (cTE) scale is the established tRNA adaptation index. Codons that are over-represented in the most highly expressed genes are marked as optimal, and nonoptimal otherwise. (d) The normalized translational efficiency (nTE) scale is defined by dividing the cTE by the codon usage, incorporating the competition between tRNA supply and demand. Codons are optimal if their relative tRNA availability exceeds the relative codon usage. The set of optimal codons from the classical scale is indicated with *. (e) Comparison of the cTE and nTE scales. (f) Correlations between the cTE and nTE scales and the codon usage.
While this “classical” optimality scale has been very useful to derive fundamental insights into the role of the prevalent codon bias, some of its simplifying assumptions may reduce its power. Because the classical view of codon optimality is based on a subset of the most highly expressed genes and not the full genome, it is biased towards optimal codons, as only optimal codons are explicitly defined20. In addition, the “classical” scale relies solely on tRNA abundances, without taking into consideration the competition for tRNAs among all translating ribosomes1, 21. However, translation rates depend on the balance between supply and demand for charged tRNAs, and a highly used codon may effectively deplete its cognate tRNAs by increasing demand. Indeed, kinetic modeling of translation elongation highlights the key role of this competition in determining translation rate of the corresponding codon21. Furthermore, the classical definition of translational efficiency incorporates species-specific tRNA pools and genomic sequences19, 22, but does not directly include mRNA expression levels, thereby overlooking the effect of divergent gene expression observed even between closely related species23. Since mRNA expression levels will affect the demand for tRNAs, its explicit incorporation into a translational efficiency scale may allow for a better comparison between organisms.
To circumvent the above limitations of the classical scale, we developed a new translational efficiency scale incorporating the balance of tRNA supply and demand. The resulting scale reflects the competition for tRNAs in the cell. Importantly, by also including the expression profile of the genome as major characteristic of organism divergence23, the new scale allows for a better evolutionary comparison of patterns of codon optimality across organisms. We find that codon optimality is evolutionarily conserved across ten closely related yeasts in a site-specific manner that is independent of levels of expression. Importantly, such evolutionary conservation of codon optimality reveals a direct and uniform link between codon optimality in the mRNAs and the secondary structures and folding elements of the encoded translated polypeptides.
Results
A normalized translational efficiency scale
The classical view of translational efficiency (cTE), which is the known tRNA adaptation index19 (tAI), does not incorporate the cellular dynamics of tRNAs, driven by a trade-off between tRNA supply and demand1, 21 (Fig. 1b). We hypothesized that a translational efficiency scale that reflects this competition for the cellular pool of tRNAs may better capture the biological forces shaping the codon bias. We thus normalized the cellular tRNA abundances and selective constraints on codon-tRNA interactions as defined in tAI19 by the codon usage (see Methods, Supplementary Fig. 1a). How often a codon is translated in the cell depends on the codon frequencies in the mRNAs, the abundances of the mRNAs that are attached to ribosomes, and the densities of ribosomes on the specific mRNAs24. We verified that mRNA abundances alone serve as a sufficient and readily available proxy for the calculation of the codon usage (Supplementary Fig. 1b–d). In this normalized translational efficiency (nTE) scale, codons are considered optimal if the relative availability of cognate tRNAs exceeds their relative usage.
Comparing the nTE to the cTE scale (Fig. 1c–e, Supplementary Fig. 2a, b), the nTE scale has a more shallow plateau-like middle region with two distinct tails of high and low efficiency codons. This stands in contrast to the cTE that scale increases almost linearly (Fig. 1e). The tail of low efficiency codons and plateau-like middle region are unique to the nTE scale, i.e. the ratio of corresponding tRNA availability and codon usage (Supplementary Fig. 2c). Importantly, this analysis suggests that tRNA supply and demand are closely matched for most codons at steady-state expression, likely supporting cost-effective proteome maintenance. Also interesting, the nTE scale contains more optimal codons that encode hydrophobic amino acids, which resonates with observations that optimal codons are associated with structurally sensitive, buried sites20. The most efficient new optimal codons in the normalized scale encode glycine, the smallest thus most neutral amino acid, and arginine, cysteine, and proline, which are important gatekeeper residues that can prevent aberrant protein aggregation25. The higher fraction of optimal codons encoding gatekeeper and hydrophobic residues revealed by the nTE scale likely reflects the need for increased fidelity during translation of sensitive polypeptide segments critical to correct folding and avoidance of aggregation20, 26. In addition, the codons encoding the most abundant amino acid glutamine are the most balanced between supply and demand in the nTE scale. The only non-degenerate amino acids methionine and tryptophan, which are nonoptimal in the cTE scale, are optimal in the nTE scale. Importantly, the nTE scale does not, unlike the cTE scale, correlate with the codon bias; the nTE scale is also not directly correlated with the cTE scale (Fig. 1f). Thus, while the sets of optimal codons before and after normalization largely overlap, the nTE scale provides a new and independent metric of translational efficiency that reflects the cellular competition for tRNAs1, 21.
A conserved translational efficiency “dip” at the start of mRNAs
Evolutionary conservation is a strong indication of functional importance. Thus, any uniform link between translational efficiency patterns in the mRNA sequences and the folding of the encoded polypeptides would expectedly be evolutionarily conserved. We undertook a systematic analysis of the conservation of codon optimality across ten closely related yeasts (see Methods). For this, we computed the nTE scale for all ten yeasts; in all cases we observed the characteristic shape of the nTE scales as observed for S. cerevisiae. Previous work using the cTE scale revealed an evolutionarily conserved region of low translational efficiency at the beginning of coding sequences, termed the “ramp”, spanning the first ca. 35–50 codons18. We thus next tested if the new nTE scale can also detect the “ramp”. The average nTE profile of the S. cerevisiae genome indeed validated the initial region of low translational efficiency (Fig. 2a), but showed that it is only ca. 10 codons long, i.e. much shorter than found using the cTE scale (Fig. 2b). Importantly, this short “dip” was evolutionarily conserved in all other analyzed yeasts (Supplementary Fig. 3). While the longer “ramp” is not observed in translational efficiency profiles of individual genes using either the cTE or nTE scales (Fig. 2c–e), this characteristic “dip” can be observed in almost all individual translational efficiency profiles, both in highly expressed genes, e.g. hydroxylase LIA1 or AAA ATPase CDC48 (Fig. 2c, d), and in lowly expressed RNA polymerase II transcription factor gene TFB3 (Fig. 2e). Interestingly, the length of the evolutionarily conserved “dip” corresponds to roughly the distance from the peptidyltransferase center to a constriction site within the ribosome10, 11 (Fig. 2f). The constriction site consists of ribosomal proteins L4 and L17, which are thought to sense nascent chain conformations to signal to the outside of the ribosome27, 28. The finding of only a short dip of low translational efficiency also stands in good agreement with the experimental determination of ribosome densities along mRNAs in yeast, which is dominated by systematic ribosome pausing at the very start of the coding sequences24. Importantly, this analysis indicates that the combination of the nTE scale and evolutionary analysis can reveal sequence signatures important for translation.
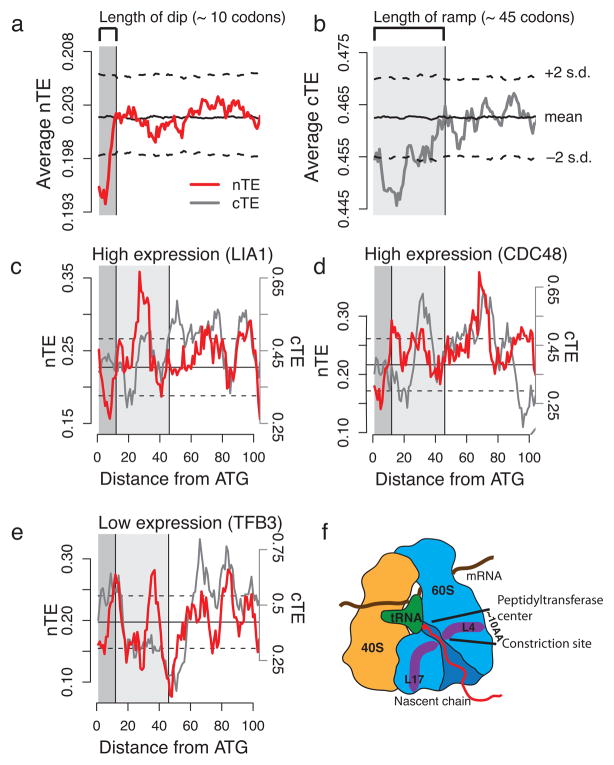
A conserved short “dip” of low translational efficiency at the beginning of mRNAs. (a) The average nTE profile of the S. cerevisiae genome exhibits a very short “dip” of low translational efficiency at the beginning of the coding regions that spans approx. across the first 10 codons. (b) The average cTE profile shows an initial region of low translational efficiency that spans approx. across 35–50 codons, previously reported as the “ramp”18. (c–e) The nTE scale reveals short “dips” in individual genes such as the highly expressed hydroxylase LIA1 (c) and cell division control protein CDC48 (d), and the lowly expressed RNA polymerase II transcription factor TFB3 (e). In contrast, the cTE scale does neither reveal the “ramp” nor the “dip” in individual profiles. (f) The length of the “dip” matches approx. the distance from the peptidyltransferase center of the ribosome to a constriction site within the exit tunnel, where ribosomal proteins L4 and L17 sense nascent chains.
Site-specific evolutionary conservation of codon optimality
A functional link between the positioning of optimal and nonoptimal codons along the mRNA and co-translational folding would predict that codon optimality is conserved in a site-specific manner, that relates to how nascent chains fold. To test for site-specific conservation of codon optimality we constructed sequence alignments across orthologs of ten closely related yeasts, and for each gene computed a conservation score of codon optimality for each position (see Methods, Fig. 3a, Supplementary Fig 4a, b). Randomizing the alignments yielded the distributions of random conservation scores, which allowed us to determine the alignment-specific minimal conservation scores found by less than 5% chance (i.e. significance thresholds with p < 0.05). Sites in the biological alignment that have higher conservation scores than these significance thresholds are thus considered significantly conserved. If the number of significantly conserved sites in an observed alignment exceeds the corresponding number in the randomized alignment, than these positions in the sequence alignment must be under direct selective pressure for site-specific conservation of codon optimality (Fig. 3b). Because expression alone can explain the fraction of optimal codons in mRNA sequences29, we devised a randomization procedure that maintains the overall codon composition in each sequence. To avoid a persistent expression bias, all analyses were performed for two curated sets of 404 alignments of high and 302 alignments of low mRNA expression levels respectively (see Methods, Supplementary Fig. 4c). Importantly, we also employed independent randomization procedures that take into account the distribution of optimal and nonoptimal codons for each amino acid in the genetic code. These latter additional analyses demonstrated that the conservation of codon optimality is completely independent of amino acid biases (Supplementary Fig. 5a–g). Furthermore, these results are independent of the 5′ coding regions (Supplementary Fig. 5h). Together, these independent analyses clearly indicate that there is site-specific evolutionary conservation of codon optimality regardless of amino acid bias or expression level.
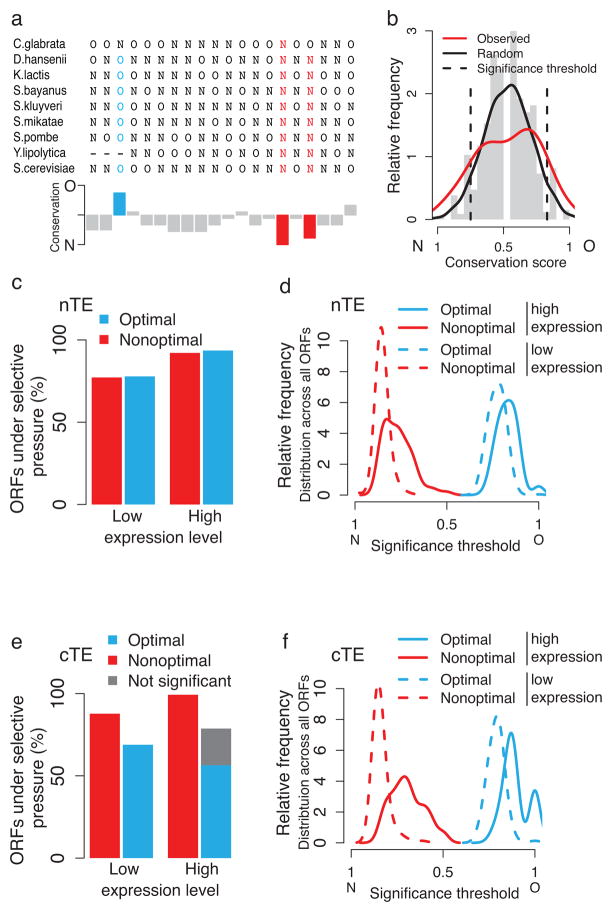
Site-specific evolutionary conservation of codon optimality. (a) Optimal (O) and nonoptimal (N) codons are projected onto an exemplary sequence alignment of the S. cerevisiae gene RIB5, and shown together with positional conservation scores. Significantly conserved optimal codons are indicated in blue, and nonoptimal codons in red. (b) Comparison of the distributions of random (grey histogram and black line) and observed (red line) conservation scores. Sites with higher conservation scores than expected by chance, i.e. outside the alignment-specific significance thresholds (dotted lines), are considered significantly conserved sites. (c) Fraction of analyzed ORFs that show a higher number of significantly conserved optimal and nonoptimal sites than would be expected by chance, thus can be assumed under selective pressure. (d) Distributions of significance thresholds for conserved optimal and nonoptimal codons for highly and lowly expressed genes, obtained using the nTE scale. (e) Fraction of ORFs under selective pressure computed with classical codon optimality. If perfect site-specific conservation of optimal codons can already be observed by chance, selection for the site-specific conservation of optimal codons cannot be assumed, and these cases are thus indicated as ‘not significant’. (f) Distributions of significance thresholds for conserved optimal and nonoptimal codons for highly and lowly expressed genes, obtained using the cTE scale.
We found that codon optimality is under selection in almost 80% of the low expression, and over 90% of the high expression genes (Fig. 3c). This is consistent with the observation that highly expressed proteins are generally more conserved30. The significance thresholds for optimal and nonoptimal codons in high and low expression genes distribute homogeneously for the nTE scale, and reflect the higher content of optimal codons in highly expressed genes as expected (Fig. 3d). Strikingly, both optimal and nonoptimal codons are found conserved in equal measure in both highly and lowly expressed genes. This challenges the view that mostly optimal codons are selected for in the context of translation. Instead, our findings indicate that codon optimality is not only tuned to expression but rather fulfills an evolutionarily selected function in protein biogenesis6–8. Of note, the same analysis using cTE to define codon optimality produces similar results for conservation of optimal codons (Fig. 3e, f). However, the cTE scale reduces the significance of conserved nonoptimal codons (Fig. 3e, f). While optimal codons are important for translational fidelity, nonoptimal codons play a key role in modulating the speed of elongation. For this reason, the nTE scale is better suited to assess the possible link between codon optimality and co-translational folding.
Conserved hidden signatures of co-translational folding
Having determined that optimal and nonoptimal codons are generally conserved raises the question regarding their role in co-translational folding events. We thus mapped the codon conservation profiles from the alignments described above onto the corresponding S. cerevisiae protein sequences and structures (e.g. Fig. 4a). Both conserved optimal and nonoptimal codons are generally distributed throughout the mRNA sequences, and often appear in clusters (Fig. 4a). We next tested for statistical associations between conserved optimal and nonoptimal codons and secondary structure elements of the encoded nascent chains (Fig. 4b, c). A control analysis using randomized synonymous codons confirmed the independence of our results from amino acid biases (Supplementary Fig. 6a, b).
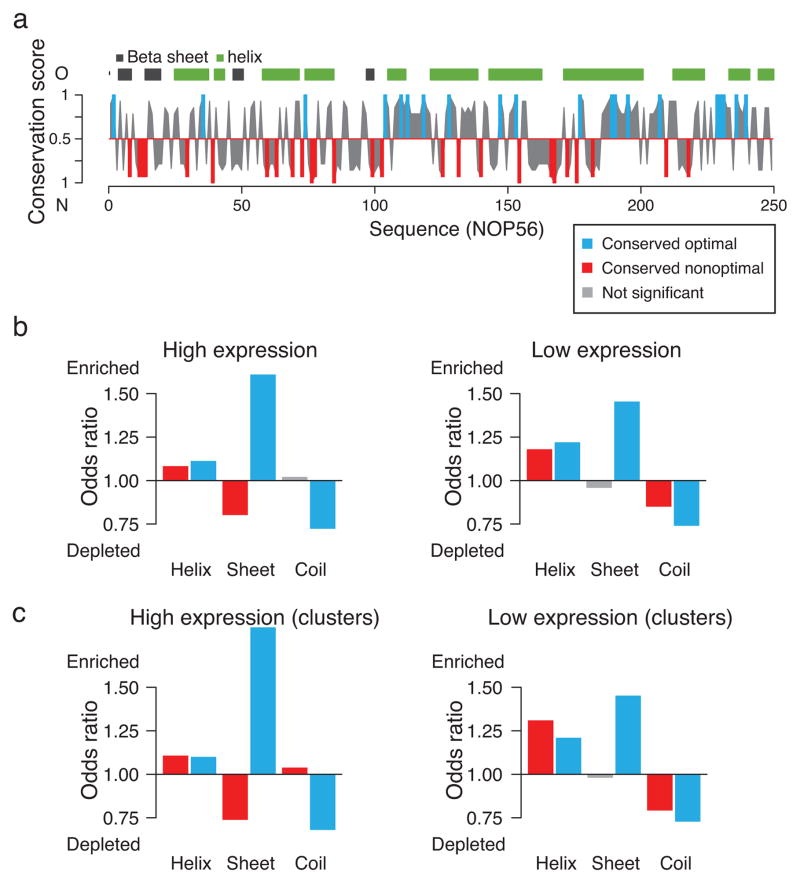
Conserved codon optimality associates with signatures of co-translational folding. (a) Exemplary conservation profile of codon optimality for the gene NOP56, calculated using the nTE scale, together with the predicted protein secondary structure. Conserved optimal codons are shown in blue, conserved nonoptimal codons in red, and not significantly conserved sites in grey. (b) Associations between conserved codon optimality and predicted secondary structure for highly (left) and lowly (right) expressed genes. (c) As in (b), but for conserved optimal and nonoptimal codons that appear in clusters.
We found distinct patterns of codon optimality conservation depending on the secondary structure of the encoded polypeptides, for both high and low expression proteins. Predicted α-helices are enriched in both conserved optimal and conserved nonoptimal codons, independent of expression (Fig 4b). In contrast, β-sheets are enriched in conserved optimal but depleted in conserved nonoptimal codons, in both highly and lowly expressed genes (Fig 4b). Of note, coil regions are always depleted of conserved optimal codons. Conserved nonoptimal codons are weakly enriched in highly expressed genes, and depleted in lowly expressed genes (Fig. 4b). Interestingly, α-helices can already form co-translationally even within the ribosomal tunnel10, 11, while coil regions, comprising loops that fold near the exit of the ribosomal tunnel, have been shown to play key roles in co-translational protein folding7. In contrast, β-sheet containing domains are topologically discontinuous and must await synthesis to begin folding. Furthermore, β-sheets are characterized by their high content of hydrophobic residues, the presence of gatekeepers, and a high aggregation propensity, thus, the general strong enrichment of conserved optimal as well as depletion of conserved nonoptimal codons could primarily serve to reduce the risk of phenotypic missense mutations leading to aggregation.
Since hydrophobicity is linked to both protein folding and aggregation, we also tested for associations between conserved codon optimality and hydrophobicity. As expected, conserved optimal codons are enriched in hydrophobic regions, and conserved nonoptimal codons are depleted. This observation is stronger for highly expressed genes (Supplementary Fig. 6c), likely owing to a greater need for translational fidelity in these abundant proteins3, 20, 26.
We next considered only sites that appear in clusters. This analysis provided a more stringent test for our results that validated and increased the significance of all the above associations between codon optimality and secondary structure propensity (Fig. 4c). In particular, the enrichment of conserved nonoptimal codons appearing in clusters is much stronger in α-helices at both high and low expression levels, and in coil regions of highly expressed genes.
To validate these observations with confirmed secondary structures, we analyzed 357 experimentally determined protein structures from the protein data bank (PDB). Importantly, PDB structures allow to distinguish between more defined structural elements such as hydrogen-bonded turns that often connect the more regular elements of secondary structure, α-helix and β-strand, and are important for folding into the final structure. This analysis indicated that conserved nonoptimal codons are strongly enriched in turns (Fig. 5a, Supplementary Fig. 6d, e). The enrichment of conserved nonoptimal codons only in α-helices and in turns is remarkable, since these secondary structure elements can form co-translationally10, 11. These findings are not detected when using the classical definition of codon optimality (Fig. 5b). All the above findings for conserved optimal codons are weaker using cTE and all significant associations to conserved nonoptimal codons are lost (Fig. 5b). This may explain why the link between codon optimality and co-translational folding has been hard to detect. Individual examples support our findings. For instance, the all-helical Myosin light chain 1 shows alternating patterns of conserved optimal and nonoptimal codons in all its helices (Fig. 5c). The 20S proteasome subunit G is characterized by a clear conservation of optimal codons in its structural core of β-sheets, complemented by conservation of both optimal and nonoptimal codons in its helices and turns (Fig. 5c).
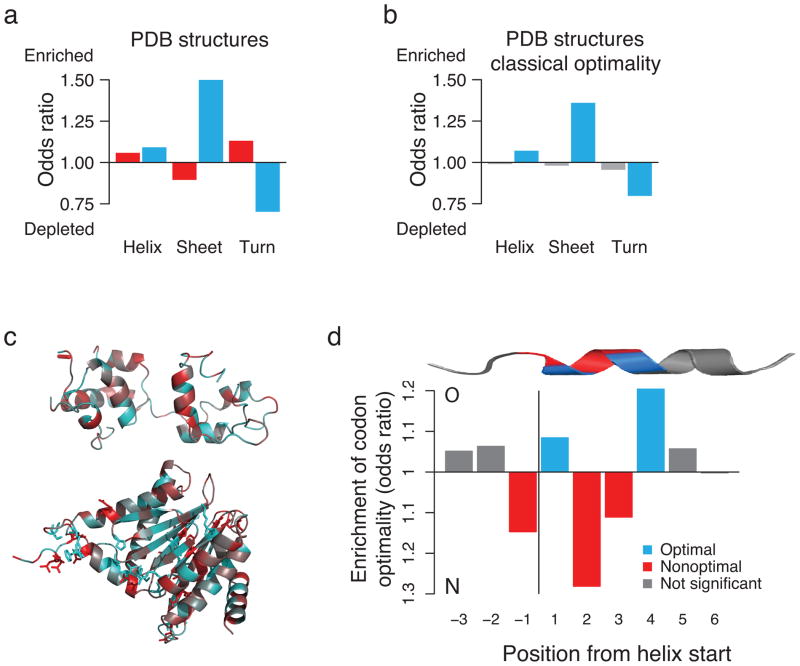
Conserved codon optimality maps onto known protein structures. (a) Associations between clusters of conserved codon optimality and secondary structures in experimentally determined protein structures. (b) Associations between codon optimality as defined in cTE, and secondary structures in experimental PDB structures. All associations for nonoptimal codons are lost. (c) Secondary structure representations, colored according to the conservation of nonoptimal (red) and optimal (blue) codons, of the exemplary Myosin light chain 1 (top, PDB structure: 1M45_A) and 20S proteasome subunit (bottom, 1RYP_G). (d) A distinct and positional pattern of optimal and nonoptimal codons characterizes α-helices in S. cerevisiae. Positions 1 and 4 are strongly enriched in optimal codons, while the transition into the helix as well as positions 2 and 3 show a clear preference for nonoptimal codons
What stands out from this analysis is the enrichment of both conserved optimal and nonoptimal codons in α-helices. The formation of α-helices is one of the elementary steps in protein folding, characterized by complex kinetics due low cooperativity that depend on an initial nucleation step31. Furthermore, this is the main folding event that has been found to occur inside the ribosome exit tunnel11, 32, 33; indeed helix formation has been experimentally observed to occur very early in the ribosomal tunnel, between the peptidyltransferase center and the constriction site28. Strikingly, for the α-helices in the experimental protein structures of S. cerevisiae, we found a distinct alternating pattern of codon optimality. Specifically, we observed a preference for a nonoptimal codon at the transition into the helix, followed by an enrichment of optimal codons at position 1 and 4, interspersed with a strong preference for nonoptimal codons at positions 2 and 3 (Fig. 5d). Moreover, the significance of this profile only extends across the first full helix turn, independent of helix length (Fig. 5d). This suggests that codon optimality may be evolutionarily selected to tune the translation and folding rates of helices early in their entry into the ribosomal tunnel. The ribosomal tunnel has been found to possess distinct folding zones that may facilitate helix formation deep inside the ribosome33, with the strongest compaction into secondary structure observed proximal to the peptidyltransferase center33. Helix formation inside the ribosomal tunnel appears strongly sequence dependent, but cannot be explained by sequence hydrophobicity or helical propensity alone33. The specialized environment within the tunnel is proposed to create a rugged solvation landscape that may slow down folding34. We speculate that the evolutionarily conserved patterns of codon optimality in helices could facilitate specific interactions with the exit tunnel wall, and may even assist helix nucleation inside the exit tunnel.
Discussion
We propose a new translational efficiency scale that incorporates the cellular competition for tRNAs into the definition of codon optimality. Codon specific translational efficiencies have long been found to correspond to a distinct codon bias, and have been suggested to play a role in the co-translational folding of the encoded polypeptides. However, a coherent and uniform link has so far remained elusive, and may be difficult to detect, in part likely due to generally weak selection on synonymous substitutions and complex but robust polypeptide folding patterns. Our analysis provides conceptual advances on two fundamental aspects of this problem. First, our normalized translational efficiency scale (nTE) incorporates the biologically relevant competition for tRNAs among all ribosomes, which is known to influence the kinetics of translation elongation1, 21. As a result, nTE allows for a better comparison between organisms. This is important, as a general and systematic link between codon optimality and co-translational folding, would have to be evolutionarily conserved. Second, we uncover a uniform relationship between the evolutionary conservation of codon optimality and the folding patterns of the nascent polypeptides, further confirming our overall approach.
Our new nTE scale reveals an evolutionarily conserved relationship between preferences in optimal and nonoptimal codons in the mRNA sequences and the secondary structures of the corresponding translated polypeptides. In light of the established correlation between the levels of expression and the fraction of optimal codons, it is even more remarkable that the site-specific evolutionary conservation of codon optimality is observed independent of expression levels. This suggests a functional role in setting a rhythm of translation elongation that correlates with the folding elements in the nascent polypeptides. Strikingly, we found that conserved nonoptimal codons are only enriched in α-helices and hydrogen-bonded turns. Helices comprise the structural elements that have been observed to fold already deep inside the ribosomal exit tunnel11, 28, 32, 33. The sensing of helical conformations at the constriction site near the peptidyltransferase center has been shown to critically influence ribosome conformation and signaling28, and further physiological roles and detailed mechanisms await to be uncovered. Since hydrogen-bonded turns and loops connect more defined folding elements within the emerging polypeptide, their enrichment in nonoptimal codons may reflect their role in coordinating co-translational folding outside the ribosome. For instance, exemplary experimental work has demonstrated the importance of nonoptimal codons in loops for the successful co-translational folding of protein domains7.
Our results point to a complex trade-off in the selection of optimal and nonoptimal codons to balance the need to allow time for successful protein folding while avoiding aberrant aggregation (Fig. 6). We find optimal codons predominantly at sites where translational fidelity is important to prevent aggregation, namely in β-sheets and for gatekeeper residues. This complements the found preference for optimal codons at structurally sensitive and aggregation-prone sites20, 26. Nonoptimal codons, often in clusters, can slow translation elongation and thus coordinate co-translational folding.
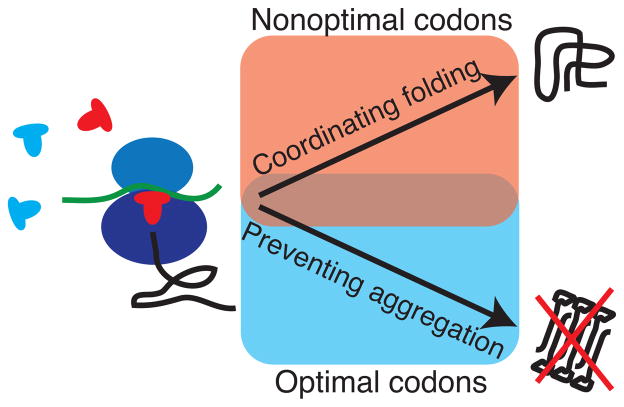
Optimal and nonoptimal codons in protein synthesis and co-translational folding. Optimal codons are translated faster and more accurately, while nonoptimal codons can induce translational pausing. Evolutionarily conserved optimal codons are found predominantly at sites where translational accuracy is important to prevent aggregation. Evolutionarily conserved nonoptimal codons are found preferentially in secondary structure elements that can fold co-translationally, even already inside the ribosomal exit tunnel.
While mRNA secondary structure may add an additional layer of translational regulation35, we found that its evolutionary conservation is much lower (Supplementary Fig. 6f–h). This suggests a weaker role in orchestrating the timing of elongation, consistent with the fact that the ribosome itself acts as helicase, unraveling the translated mRNAs36.
One interesting aspect of the new nTE scale is that tRNA supply and demand are very balanced for most codons at steady state. Remarkably, almost all amino acids are encoded by equal numbers of optimal and non-optimal codons in nTE. As a result, the strong and consistent link between conserved codon optimality and protein secondary structure we observe is independent of amino acid biases and must thus be the direct result of site-specific selection on codon optimality. Evolutionary selection appears to exploit and amplify very subtle effects, as highlighted by the remarkable fact that we consistently find clusters of conserved optimal and nonoptimal codons. Our definition of nTE assumes steady state conditions, and no limitation in amino acid supply. It is interesting to speculate that tRNA recycling, dynamics and modifications37, 38 may further influence the rhythm of translation elongation, both at steady state and in response to cellular stresses such as amino acid starvation39.
In summary, we found uniform and evolutionarily conserved signatures in the mRNA sequences that link to folding patterns of the encoded polypeptides. The ribosome emerges in this analysis as a very active folding environment and the choice of the coding sequence emerges as finely tuned to the action of the ribosome. Our findings present a promising avenue to increase our understanding of in vivo protein folding, still a fundamental and poorly understood problem in biology.
Online Methods
Data sources
Genomic sequences and ortholog assignments for S. cerevisiae, C. glabrata, D. hansenii, K. lactis, S. bayanus, S. kluyveri, S. mikatae, S. paradoxus, S. pombe, and Y. lipolytica were retrieved from the Broad Institute (http://www.broadinstitute.org/regev/orthogroups)40, and alignments of the genetic sequences between orthologs were computed with ClustalW41 via the corresponding amino acid sequences. tRNA counts were retrieved from the tRNA database (http://www.gtrnadb.ucsc.edu) if available, otherwise predicted with tRNAscan-SE from the genomic sequences42. mRNA expression levels for S. cerevisiae were obtained from43, for S. pombe from44, and for all other yeasts from45. 1574 alignments contained more than 7 sequences, our requirement to obtain meaningful conservation scores. To remove any intrinsic expression bias, we normalized the expression levels across the yeasts by quantile normalization, and selected 500 alignments each of high and low expression and with the lowest inner expression divergence measured by the standard deviation across orthologs. Removing alignments with more than 30% gaps yielded a set of 404 alignments of highly, and 302 alignments of lowly expressed genes. The mapping of PDB structures to S. cerevisiae genes was obtained from46, yielding a curated set of 357 alignments.
Protein secondary structures were predicted with PSIPRED47. RNA secondary structures were predicted with the Vienna package48. For alignments with assigned PDB structure, secondary structure and relative accessible surface area were extracted with the DSSP program49. Sequence hydrophobicity was computed using the Kyte&Doolittle scale.
Translational efficiency and codon optimality
The classical translational effiency cTEi for each codon is the published tRNA adaptation index (tAI) as computed with the codonR program19. It estimates the tRNA availability for each codon i from a weighted sum of the gene copy numbers tGCNij of the matching tRNA isoacceptors j under a selective constraint sij on the efficiency of the codon–anticodon coupling, incorporating Crick’s wobble rules19: ni
The selective constraint on codon–anticodon interactions sij is 0 for cognate tRNAs, and small for wobble interactions19. The overall efficiency of a codon is thus given by the sum of the contributions of the recognizing individual tRNAs under consideration of specific selective constraints based on the codon–anticodon interaction19. The division of the individual translational efficiencies Wi by the maximum translational efficiency Wmax linearly rescales all translational efficiencies so that the maximum value is 1.
The codon usage cui was defined as relative estimate of how often each codon is translated. It is derived from the number of occurrences of each codon in an ORF, weighted by the corresponding transcript abundance, and summed up over all ORFs (Supplementary Fig. 1a). For codon i this is the sum of the counts cij of the codon i in gene j, weighted by the transcript abundance aj of gene j, considering all genes in the genome g. For comparability, the codon usage is also rescale to have a maximum value of 1.
In this work, the normalized translational efficiency nTEi is subsequently defined as the ratio of tRNA availability cTEi (supply), which is based on cellular tRNA abundance and selective constraints for wobble interactions, and codon usage cui (demand), linearly rescaled to have a maximum value of 1.
Codons i with cTEi ≥ cui are considered optimal, and nonoptimal otherwise. For comparison, we used the set of classical optimal codons reported in20. They are those found significantly enriched in the highest expressed genes by a Chi-square test20. Average translational efficiency profiles were computed as described in reference 18.
Randomization procedure & significant sites
We tested for evolutionary conservation of codon optimality in 10 closely related yeasts. The conservation score S of optimal or nonoptimal codons at any given position i is defined as Si = ni/ Ni, where ni is the number of optimal or nonoptimal codons at position i, and Ni the total number of aligned codons at position i respectively. We only considered alignments with at least 7 orthologous sequences, and a minimum of 5 codons has to be aligned for a conservation score to be computed at that position. Each alignment of orthologs was randomized 1000 times by individually shuffling each sequence to keep its original composition and maintain the individual codon bias. We employed additional randomization schemes to verify independence of amino acid biases (Supplementary Fig. 5,6). From the distribution of the conservation scores in the randomized alignments, we extracted alignment-specific the minimal conservation scores that are observed at less than 5% chance as significance thresholds. We considered sites with higher conservation scores than the significance thresholds as significantly conserved. If more optimal and nonoptimal codons respectively are significantly conserved in the biological alignment than in the randomized alignment, the site-specific evolutionary conservation of optimal and nonoptimal codons respectively is the result of selective pressure.
Individual and average translational efficiency profiles
Individual translational efficiency profiles were computed with the nTE and cTE scales, and smoothed with a sliding window of size 15, the size of the immediate ribosome footprint18. Average translational efficiency profiles were computed by aligning all genes at the start codon, and subsequently calculating the average translational efficiency for each position as described in18. Thus, the average profiles only reflect those fluctuations of the individual profiles that are present in all sequences. We randomly reshuffled all sequences to calculate the mean and standard deviation for each position18.
Statistical testing
All statistical testing of associations was performed using the Cochran–Mantel–Haenszel test in the statistical computing environment R (http://www.r-project.org), and as described in reference 20. The enrichment of optimal or nonoptimal codons at specific helix positions in S. cerevisiae sequences was tested with Fisher’s exact test and corrected for multiple-testing with the Benjamini & Hochberg (1995) method. All test statistics and definitions of optimal and nonoptimal codons are listed in the Supplementary Information (Supplementary Tables 1–8).
Data availability
All datasets of this study are available at http://www.stanford.edu/group/frydman/codons.
Acknowledgments
We thank the Frydman Lab for helpful discussions. We gratefully acknowledge support from an EMBO Long-Term Fellowship (ALTF 1334-2010) to S.P., and NIH grants GM56433 and AI91575 to J.F.
Footnotes
Author Contributions
S.P. performed all analyses; S.P. and J.F. designed research, interpreted the data and wrote the manuscript.References
Full text links
Read article at publisher's site: https://doi.org/10.1038/nsmb.2466
Read article for free, from open access legal sources, via Unpaywall:
https://www.ncbi.nlm.nih.gov/pmc/articles/PMC3565066
Citations & impact
Impact metrics
Citations of article over time
Alternative metrics
Smart citations by scite.ai
Explore citation contexts and check if this article has been
supported or disputed.
https://scite.ai/reports/10.1038/nsmb.2466
Article citations
Pulling Forces Differentially Affect Refolding Pathways Due to Entangled Misfolded States in SARS-CoV-1 and SARS-CoV-2 Receptor Binding Domain.
Biomolecules, 14(10):1327, 18 Oct 2024
Cited by: 0 articles | PMID: 39456260 | PMCID: PMC11505858
A machine learning approach uncovers principles and determinants of eukaryotic ribosome pausing.
Sci Adv, 10(42):eado0738, 18 Oct 2024
Cited by: 0 articles | PMID: 39423268 | PMCID: PMC11488575
Codon usage and expression-based features significantly improve prediction of CRISPR efficiency.
NPJ Syst Biol Appl, 10(1):100, 03 Sep 2024
Cited by: 0 articles | PMID: 39227603 | PMCID: PMC11372048
Massively parallel identification of sequence motifs triggering ribosome-associated mRNA quality control.
Nucleic Acids Res, 52(12):7171-7187, 01 Jul 2024
Cited by: 2 articles | PMID: 38647082 | PMCID: PMC11229359
The Effects of Codon Usage on Protein Structure and Folding.
Annu Rev Biophys, 53(1):87-108, 28 Jun 2024
Cited by: 3 articles | PMID: 38134335
Review
Go to all (291) article citations
Data
Data behind the article
This data has been text mined from the article, or deposited into data resources.
BioStudies: supplemental material and supporting data
Protein structures in PDBe (2)
-
(1 citation)
PDBe - 1M45View structure
-
(1 citation)
PDBe - 1RYPView structure
Similar Articles
To arrive at the top five similar articles we use a word-weighted algorithm to compare words from the Title and Abstract of each citation.
Understanding the influence of codon translation rates on cotranslational protein folding.
Acc Chem Res, 47(5):1536-1544, 01 May 2014
Cited by: 61 articles | PMID: 24784899
Review
Silent substitutions predictably alter translation elongation rates and protein folding efficiencies.
J Mol Biol, 422(3):328-335, 12 Jun 2012
Cited by: 135 articles | PMID: 22705285 | PMCID: PMC3576719
The Effects of Codon Usage on Protein Structure and Folding.
Annu Rev Biophys, 53(1):87-108, 28 Jun 2024
Cited by: 3 articles | PMID: 38134335
Review
Evidence of multifaceted functions of codon usage in translation within the model beetle Tribolium castaneum.
DNA Res, 26(6):473-484, 01 Dec 2019
Cited by: 2 articles | PMID: 31922535 | PMCID: PMC6993815
Funding
Funders who supported this work.
NIAID NIH HHS (1)
Grant ID: P01 AI091575
NIGMS NIH HHS (3)
Grant ID: R01 GM056433
Grant ID: GM56433
Grant ID: R37 GM056433