Abstract
Free full text

The tumor microenvironment shapes lineage, transcriptional, and functional diversity of infiltrating myeloid cells
Abstract
Myeloid cells play important regulatory roles within the tumor environment by directly promoting tumor progression and modulating the function of tumor-infiltrating lymphocytes, and as such, they represent a potential therapeutic target for the treatment of cancer. Although distinct subsets of tumor-associated myeloid cells have been identified, a broader analysis of the complete myeloid cell landscape within individual tumors and also across different tumor types has been lacking. By establishing the developmental and transcriptomic signatures of infiltrating myeloid cells from multiple primary tumors we found that tumor-associated macrophages (TAMs) and tumor-associated neutrophils (TANs), while present within all tumors analyzed, exhibited strikingly different frequencies, gene expression profiles, and functions across cancer types. We also evaluated the impact of anatomic location and circulating factors on the myeloid cell composition of tumors. The makeup of the myeloid compartment was determined by the tumor microenvironment rather than the anatomic location of tumor development or tumor-derived circulating factors. Pro-tumorigenic and hypoxia-associated genes were enriched in TAMs and TANs compared with splenic myeloid-derived suppressor cells. While all TANs had an altered expression pattern of secretory effector molecules, in each tumor type they exhibited a unique cytokine, chemokine and associated receptor expression profile. One such molecule, haptoglobin, was uniquely expressed by 4T1 TANs and identified as a possible diagnostic biomarker for tumors characterized by the accumulation of myeloid cells. Thus, we have identified considerable cancer-specific diversity in the lineage, gene expression, and function of tumor-infiltrating myeloid cells.
INTRODUCTION
The tumor microenvironment contains a multiplicity of stromal cells of hematopoietic and non-hematopoietic developmental origin, such as T cells, B cells, NK cells, myeloid cells, fibroblasts, pericytes, adipocytes, and endothelial cells, which collectively shape the disease course [1–4]. Although specific roles have been identified for discrete stromal subsets, factors controlling their recruitment, expansion, and function in different tumors remain enigmatic. Therefore, a more complete characterization of these subsets and a better understanding of how they are recruited to and expand within growing tumors and metastases are of utmost importance to developing novel therapies and improving existing ones against cancer.
Tumor growth is associated with the accumulation of a variety of myeloid cell types [2]. Common myeloid cell progenitors in the bone marrow can give rise to myeloid cells with immunosuppressive potential, oft referred to as myeloid-derived suppressor cells (MDSCs). Monocyte-like CD11b+Gr1low and granulocyte/neutrophil-like CD11b+Gr1hi subsets of MDSCs have been reported to accumulate in the spleen, liver, blood, and bone marrow during tumor progression. Within tumors, myeloid cells with similar phenotypes are referred to as tumor-associated macrophages (TAMs) or neutrophils (TANs), possibly reflecting a more differentiated identity. In vitro studies have shown that differentiation of bone marrow progenitors into MDSCs requires a combination of cytokines, particularly IL-6 and G-CSF or GM-CSF, and the transcriptional regulator CCAAT/enhancer-binding protein (C/EBP) [5]. Although splenic MDSCs are considered a reservoir for tumor-infiltrating myeloid cells [6], the exact relationship between these cells remains elusive. Accumulating evidence indicates that MDSCs, whether in the spleen or in the tumor, have direct suppressive effects on cytotoxic leukocytes. In addition to MDSCs, conventional and plasmacytoid dendritic cells (DCs) may exert immunoregulatory effects in tumors [2] using a variety of mediators such as indoleamine 2,3-dioxygenase (IDO), inducible nitric oxide synthase (iNOS), and arginase I to suppress T-cell proliferation, cytotoxicity and effector cytokine production.
Given that myeloid cells with protumorigenic and immunomodulatory functions have been observed in multiple animal tumor models and in cancer patients, they represent important targets for immunotherapy. Efforts are underway to identify myeloid-focused strategies. Approved chemotherapy agents, such as Gemcitabine [7], 5-fluorouracil [8], and Sunitinib [9], can eliminate or prevent the accumulation of MDSCs, especially in lymphoid organs, and retard tumor progression. Likewise, agents that block myeloid recruitment to tumors, such as CSF1R inhibitors [10], hold clinical promise. However, to improve current strategies and identify new universal targets for therapeutic intervention, it is essential to understand how each myeloid cell subset from one tumor relates to the same population in other tumor types.
In this study, we analyzed myeloid subsets in multiple murine tumors to study how phenotype, frequency, and transcriptional profiles relate within different tumors, using triple-negative 4T1 breast cancer, Her2+ breast cancer, and B16 melanoma as models. Strikingly, each tumor type contained a distinct myeloid cell landscape, with TAMs, TANs and DCs represented in all tumors but at markedly different ratios, while systemic MDSC accumulation was exquisitely tumor-specific. Our data suggest that tumor type, rather than anatomic location, dictates myeloid composition in the tumor lesion. Furthermore, although each subset exhibits similar transcriptional signatures associated with its identity in different tumors, our study demonstrates that functional differences exist across myeloid subsets from different tumors. In particular, our data suggest that haptoglobin may represent a biomarker for tumors characterized by systemic accumulation of myeloid cells. Thus, our study provides important insights into the identity and functional characteristics of tumor-associated myeloid subsets. Perhaps more importantly, our data support that in-depth transcriptomic analysis of tumor-infiltrating myeloid cells may reveal novel therapeutically attractive targets for cancer.
MATERIALS AND METHODS
Ethics statement
All animal work has been carried out in accordance with US National Institutes of Health guidelines. This study is reviewed and approved by the Dana-Farber Cancer Institute Animal Care and Use Committee (ACUC) (protocol IDs: 04-025 and 07-038).
Mice
Six-week old, sex-matched C57BL/6, Balb/c, or CD45.1+ (B6.SJL-PtprcaPepcb/BoyJ and CBy.SJL(B6)-Ptprca/J) mice were purchased from Jackson Laboratories. F1 (Balb/cxC57BL/6) mice were bred in-house. RIP1-Tag2 mice were obtained from Mouse Model of Human Cancer Consortium (NCI). Mice with spontaneous invasive pancreatic ductal adenocarcinoma (PDA, Pdx1-Cre LSL-KrasG12D PtenL/+) were kindly provided by Dr. DePinho (while at the Dana-Farber Cancer Institute; currently at the MD Anderson Cancer Center) [11].
Cell lines and tumor models
B16, EL4, 4T1, A20, and CT26 cells obtained from ATCC. Pan02 cells were obtained from the NCI DTP repository. The 4T07 cells were obtained from Dr. J. Lieberman (Harvard University, MA); they were expanded, aliquoted, were banked in liquid nitrogen. No additional authentication was performed on these cells. The Her2 cell line was derived from a spontaneous breast tumor in a Balb/c Her-2/neu-transgenic mouse that was found to lose Her-2/neu expression in vitro. Transgenic rat Her-2/neu sequence was inserted into a pMFG retroviral vector to obtain a stably over-expressing cell line. Cell lines were maintained in DMEM (B16 and EL4) or RPMI (4T1, Her2, A20, Pan02, CT26, and 4T07) supplemented with 10% FBS and 1x penicillin/streptomycin.
For subcutaneous (s.c.) tumors, 1–2.5×105 cells were injected into the flanks of naive mice (4T1, Her2, CT26 and 4T07 into Balb/c; B16 and Pan02 into C57BL/6; A20 into Balb/cxCD45.1, EL4 into C57BL/6xCD45.1), and tumor growth was monitored using calipers until tumor size reached 0.5–1 cm diameter. For transgenic models, tumors were monitored and analyzed at different time points based on tumor growth (RIP-Tag2 at 11–12 weeks, and PDA and Her2 transgenic at 11–24 weeks).
Cell preparation and flow cytometry
Spleens and tumors were processed by gentle mechanical disruption with forceps followed by enzymatic digestion using 0.2 mg/mL collagenase P (Roche), 0.8 mg/mL dispase (Invitrogen), and 0.1 mg/ML DNase I (Sigma). Tissues were incubated in digestion medium at 37°C for 30 min; released cells were collected, filtered and the remaining tissue was further processed. Blood was collected into tubes containing 2 mM EDTA, and bone marrow cells were isolated by flushing media through tibias and femurs. Red blood cells were lysed using ACK solution. Cells were resuspended in FACS buffer containing 1% FBS and 2 mM EDTA.
Cells were stained in FACS buffer containing FcR-blocking antibody (2.4G2) with combinations of fluorochrome-conjugated or biotinylated antibodies against CD11b (M1/70), CD11c (N418), CD45 (30.F11), Gr1 (RB6-8C5), MHC class II (M5/114.15.2), CD80 (16-10A1), CD86 (GL-1), CD40 (HM40-3), CD26 (H194-112), CD103 (2E7), cKit (2B8), Flt3 (A2F10) or isotype controls purchased from BioLegend and BD Biosciences. Cells were analyzed using BD FACS Aria II or BD FACSCalibur (BD Biosciences), and FlowJo Software (Tree Star, Inc). For cell sorting from spleens, myeloid cells were enriched by depletion of CD3+, CD19+ and CD49b+ cells using biotinylated antibodies and anti-biotin beads (MACS, Miltenyi). In tumor samples, CD45+ cells were enriched by positive selection using a biotinylated antibody and anti-biotin beads (EasySep, StemCell Technologies). After enrichment, cells were stained with fluorochrome-conjugated antibodies and propidium iodide (Sigma) to exclude dead cells. Cells were sorted using a BD FACS Aria II equipped with a 100 μm nozzle running at 20 psi. After an initial sort to verify purity, a second sort was performed to collect myeloid cell populations of 95–100% purity directly into TRIzol reagent (Invitrogen). Sample size for each population was as follows: CD11b−/intGr1−CD11c+MHCII+ (N=4), CD11b+Gr1lowCD11c+MHCII+ (N=3), CD11b+Gr1lowCD11c−MHCII− (N=3), and CD11b+Gr1hiCD11c−MHCII− (N=2) from B16 tumors; CD11b+Gr1lowCD11c+MHCII+ (N=3), CD11b+Gr1lowCD11c−MHCII− (N=3), and CD11b+Gr1hiCD11c−MHCII− (N=2) from 4T1 tumors; CD11b+Gr1lowCD11c+MHCII+ (N=3) and CD11b+Gr1hiCD11c−MHCII− (N=3) from Her2 tumors; CD11b+Gr1lowCD11c−MHCII− (N=2) and CD11b+Gr1hiCD11c−MHCII− (N=3) from spleens of 4T1 tumor bearing mice.
RNA isolation and microarray analysis
Total RNA was prepared from TRIzol using chloroform extraction according to the manufacturer’s protocol, and 100 ng of RNA from each sample was used for amplification, labeling, and hybridization by Expression Analysis, Inc. (Durham, NC). Mouse Gene ST 1.0 chips (Affymetrix) were used for microarray analysis. All data are MIAME-compliant and the raw data generated as part of the Immunological Genome Project (ImmGen) have been deposited in a MIAME-compliant database [NCBI Gene Expression Omnibus (GEO) data repository; record no: GSE15907 and GSE37448]. Various modules included in GenePattern platform (Broad Institute) were used for data analysis. Additional myeloid cell populations from healthy tissues analyzed within the same microchip batch of ImmGen were used as a comparison [F4/80 Macrophages from peritoneal cavity (PC, n=2), Ly6C+ DCs from bone marrow (BM, n=2), CD24−Sirpa+ DC from BM (n=3), CD24+Sirpa+ DC from BM (n=3), CD4 DC from spleen (n=1), CD11c−CD103+ DC from small intestine (SI, n=1), neutrophil from BM (C57BL/6, n=2), and neutrophil from BM (Balb.c, n=2)]. Raw data were normalized with ExpressionFileCreator module using the RMA method. The MultiPlot module was used for dataset comparisons including fold-change analysis and statistical filtering. In each analysis, we preprocessed the dataset for populations included in specific analyses. In fold-change analyses, an arbitrary cutoff value of 2 was used, in combination with coefficient of variation (CV)<0.5 for replicates. T-test P values <0.05 were considered statistically significant for each probe. After filtering, we selected all probes with a mean expression above 100 in at least one of the populations analyzed, which indicates actual expression with 95% confidence. For hierarchical clustering, Pearson’s correlation was used with datasets following log2 transformation, row centering, and row normalization in HierarchicalClustering module. Heatmaps were constructed using HeatmapViewer module. Pathway enrichments were analyzed by Ingenuity Pathway Analysis. DCs- and macrophages-associated genes were determined previously [12,13], and a similar analysis was performed to identify neutrophil-associated genes (unpublished data). Principal component analyses were conducted using the PopulationDistances module created by ImmGen (probes with mean expression value >120 selected, dataset log2 transformed, probes with top 15% variability selected).
Cytokine arrays
Cell culture supernatants from 4T1, Her2 and B16 cells were collected in two separate batches when cells were ~80% confluent and analyzed with Mouse Cytokine Antibody Array 3 (RayBiotech, Inc.) according to the manufacturer’s protocol. Spot densities were measured using ImageJ (NIH), and relative amounts were calculated based on positive and negative controls. Only cytokines and chemokines with a positive signal are shown.
ELISA for Haptoglobin (HP)
Serum was obtained from naive or tumor-bearing mice. Human sera were obtained from patients with metastatic breast carcinoma upon enrollment into IRB approved vaccine trials at the Dana-Farber Cancer Institute. Informed consent was obtained for each participant regarding usage of collected samples for research purposes. Samples were collected by routine procedures prior to beginning study treatment. HP levels were quantified using Mouse or Human Haptoglobin ELISA (ICL, Inc.) according to the manufacturer’s protocol. Concentrations were calculated using a four-parameter logistics curve.
Statistical analysis for non-microarray data
Data are expressed as mean±SD and were analyzed using one-tailed, unpaired Student’s T-test. ELISAs were analyzed using ANOVA and Tukey’s test. P values <0.05 were considered statistically significant.
RESULTS and DISCUSSION
Identification and enumeration of tumor-associated myeloid cells reveal tumor-specific diversity
Thus far, it remains uncertain how tumor origin, localization, and history influence the myeloid cell landscape of different solid malignancies. To address these questions, we initially sought to compare the composition of myeloid cell infiltrates among 4T1 and Her2, two distinct murine tumors sharing the same mammary tissue origin. Tumors were established s.c. in the flanks of Balb/c mice and analyzed when they reached different tumor sizes. We characterized multiple myeloid cell populations isolated from each tumor type on the basis of myeloid-specific surface markers CD11b, Gr1, and CD11c by cytofluorimetry. In both types of tumors and throughout tumor history, the CD45+ hematopoietic compartment was dominated by CD11b+ cells (>75%) (Fig. 1A, Supplementary Fig. S1A). The intratumoral CD11b+ cells comprised three sub-populations based on Gr1 and CD11c expression (Fig. 1A). In 4T1 tumors, the hematopoietic compartment contained ~20% CD11b+Gr1hiCD11c− cells and >45% CD11b+Gr1low; with >60% of the latter expressing CD11c. Compared to 4T1, Her2 tumors were infiltrated by a relatively small population of CD11b+Gr1hiCD11c− cells (3%); while the majority (>95%) of myeloid cells were CD11b+Gr1lowCD11c+. We also found a Gr1−CD11c+ population among CD11b− cells, which comprised <1% of all hematopoietic cells in both 4T1 and Her2 tumors. Further analysis showed that CD11b+Gr1lowCD11c+ and CD11b−Gr1−CD11c+ cells in the tumor were largely MHCII+, whereas CD11b+Gr1lowCD11c− and CD11b+Gr1hiCD11c− cells were MHCII− (Fig. 1B). A marked accumulation of splenic CD11b+Gr1low and CD11b+Gr1hi cells (Fig. 1C) was observed in 4T1-bearing mice whereas the splenic numbers in mice with Her2 tumors were similar to naive mice. These data suggest that each tumor type may contain a distinct myeloid cell infiltrate.
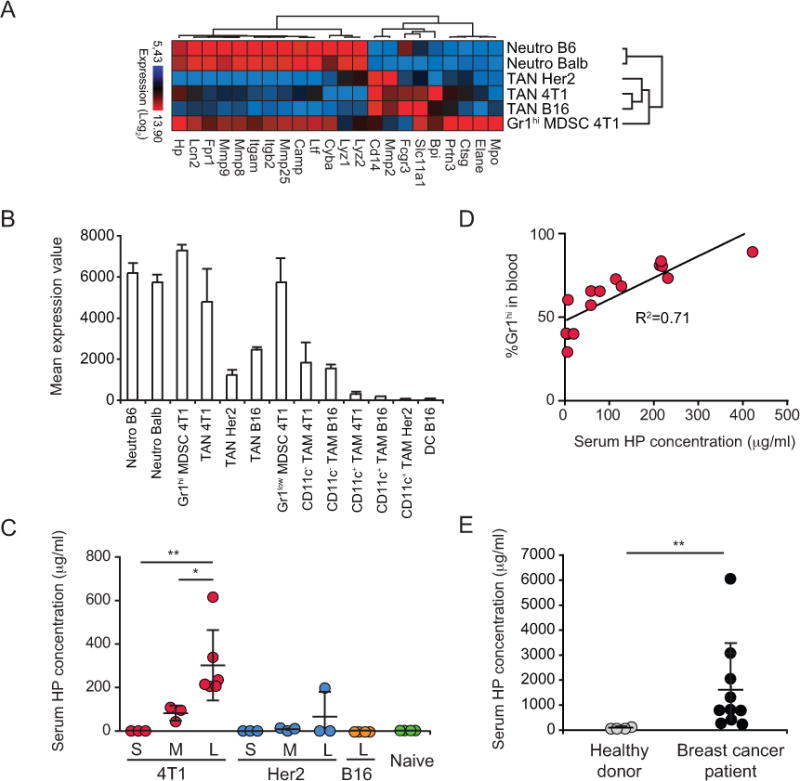
A, Analysis of myeloid cells subsets within subcutaneous 4T1 and Her2 tumors by flow cytometry. Hematopoietic cells were analyzed for the expression of CD11b and Gr1, and gated populations in the dot-plots were further analyzed for CD11c expression. B, MHCII expression by indicated subsets. C, Accumulation of CD11b+Gr1+ cells in the spleens of 4T1 tumor-bearing mice (top) and Gr1 expression (bottom). Numbers indicate percentage of cells for each gate or region. D, Dendrogram analysis based on hierarchical clustering of tumor-associated and steady-state myeloid cells (SI, small intestine, PC peritoneal cavity; Spl, spleen; BM, bone marrow; Tmr, tumor). Sample sizes are indicated in Materials and Methods. E, Hierarchical clustering of the same populations based on a list of 139 genes associated with DCs, macrophages or neutrophils.
Lineage determination of tumor-infiltrating myeloid cell subsets based on transcriptional profiling
We next sought to determine the lineage of each tumor-infiltrating myeloid cell population using transcriptomic analysis. To this end, myeloid subsets from 4T1 and Her2 breast carcinomas, and B16 melanoma were sorted to high purity, according to the ImmGen standard operating procedure. Transcriptional profiling data were generated on Affymetrix ST1.0 microarrays adhering to profiling and quality control pipelines of ImmGen [14]. We utilized hierarchical clustering of ~13.5K probes, after excluding those with coefficient of variation values <0.5 and mean expression values <120 to compare populations of tumor-infiltrating myeloid cells with representative myeloid cell subsets (DCs, macrophages, and neutrophils) from lymphoid and non-lymphoid tissues of healthy animals. Dendogram analysis based on gene clustering depicted similarities between neutrophils and tumor-derived Gr1hi cells, macropahges and Gr1low cells (regardless of CD11c expression), and conventional DCs and CD11b−Gr1−CD11c+ cells (Fig. 1D). Hierarchical clustering of 139 probes corresponding to signature genes of DCs, macrophages [12,13], and neutrophils (unpublished data) demonstrated that tumor-infiltrating myeloid cell populations are indeed CD11c+ and CD11c− TAMs, TANs, and DCs (Fig. 1E and Supplementary Table S1).
Striking heterogeneity in myeloid cell infiltrates across different tumor types
Among the three tumor types analyzed, Her2 tumors were uniquely characterized by the presence of a large CD11c+ TAM population whereas 4T1 tumors contained a relatively large TAN population (Fig. 2A). In B16 tumors, myeloid cells comprised only 40% of tumor-infiltrating leukocytes compared to >75% in 4T1 and Her2 tumors (Fig. 2A). The aforementioned differences in myeloid abundance also reflected variation in the abundance of other leukocytes infiltrating tumors including T, B, NK, and NKT cells, and pDCs. For example, B16 tumors were enriched in CD3+ T cells compared to 4T1 and Her2 tumors, consistent with a more immunogenic environment in B16 melanoma (Fig. 2B). To further ascertain the degree of myeloid heterogeneity, we extended our analysis to five additional transplanted tumors including 4T07 breast cancer, CT26 colon carcinoma, EL4 T lymphoma, A20 B lymphoma, and Pan02 pancreatic ductal adenocarcinoma (PDA), and three autochthonous mouse models including RIP-Tag2 (insulinoma), Her-2/neu (mammary carcinoma), and Pdx1-Cre LSL-KrasG12D PtenL/L (PDA) mice. The same four major populations were present within each tumor model but at significantly different frequencies across the tumors analyzed (Fig. 2A–C).
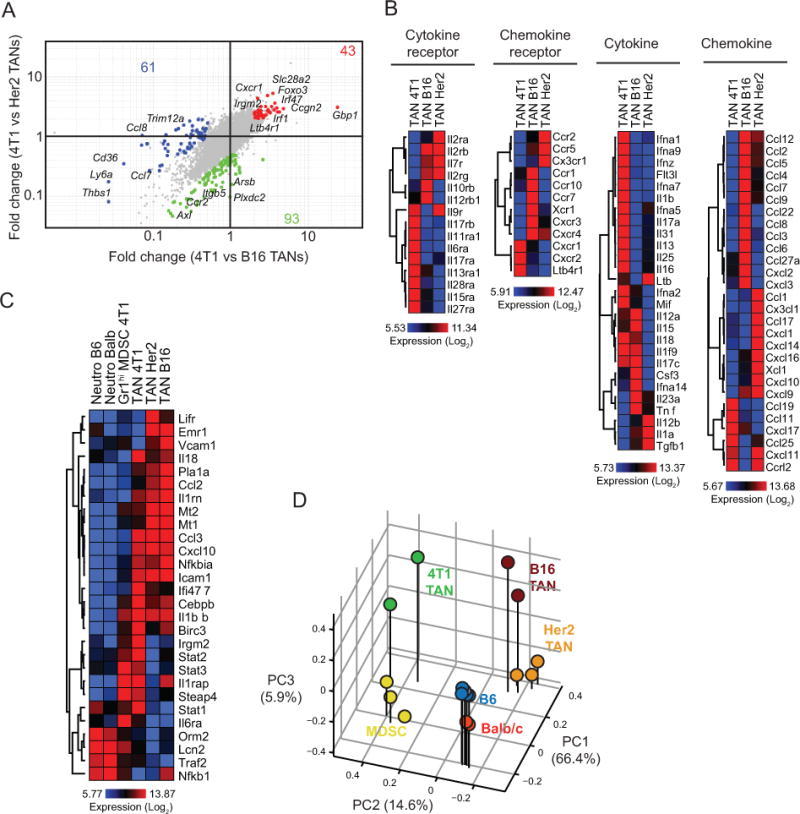
A, Whisker box-plots summarizing the relative frequency of myeloid subsets among hematopoietic cells within 4T1, Her2 and B16 tumors. B, Frequency of CD3+ T cells within hematopoietic cells in 4T1, Her2 and B16 tumors. C, Frequency of myeloid cells in 4T07, Pan02, EL4, CT26, PDA, RIP-Tag2 and A20 tumors (n=3–7). * P<0.05, ** P<001, *** P<0.001.
In all tumors, DCs were present at very low frequencies, with the highest levels in B16, EL4 and RIP-Tag2 tumors (4–10%) (Fig. 2). Given that DCs account for only a small percentage of hematopoietic cells within the tumors, and that the major subset expressing CD11c in tumors is TAMs, our study also suggests that CD11c alone is an unreliable marker for DCs in neoplastic lesions. Further analysis comparing DCs in B16 tumors to TAMs indicated that while TAMs expressed a variety of endocytic and regulatory receptors including Pilrb, Sirpa, Lilrb4, and Clec5a (Supplementary Fig. S1B), tumor DCs expressed signature genes such as Dpp4, Flt3, and Kit, as well as CD8/CD103 DC-associated genes [13] including Itgae, Batf3, Tlr3, ifi205, and Xcr1 identifying them as CD11b−CD103+ tissue-resident DCs. Flow cytometric analysis confirmed that DCs were the myeloid cells expressing high levels of CD26 (Dpp4), Flt3, cKit, and CD103 (Itgae) protein in these tumors (Supplementary Fig. S1C, and data not shown). Based on these distinctions, DCs may be considered as a reference population in future studies to identify unique targets on TAMs and TANs to modulate their tumor-promoting functions. For example, DCs lack the surface receptor Clec5a (MDL-1), whereas expression in TAMs, TANs, and neutrophils is relatively high (>18-fold higher than in DCs), suggesting that it may represent an attractive target for antibody-mediated depletion of CD11b+Gr1+ cells in tumors [15].
Differences in Gr1low and Gr1hi cells across several diverse tumors were not restricted to the tumor lesion itself. For example, a substantial increase in MDSCs was observed in the spleen and blood of 4T1 tumor-bearing mice throughout tumor progression but not in mice bearing other tumor types (Supplementary Fig. S1D and S1E).
Differences in myeloid cell composition arise from specificity of the tumor microenvironment
The myeloid composition of different tumors is likely shaped by a combination of tumor-specified growth factors. Indeed, 4T1, Her2, and B16 cell lines produce unique combinations of cytokines and chemokines, suggesting that each tumor may create a distinctive microenvironment (Supplementary Fig. S2A). These differences may also explain why MDSCs preferentially accumulate in certain tumors. For example, high levels of G-CSF and LIX (CXCL5) expression by 4T1 cells may contribute to systemic accumulation of MDSCs in vivo [16,17]. To assess whether specific tumor microenvironments directly influence the myeloid cell content, we analyzed myeloid cells within the same tumor type growing at different anatomical locations. Comparison of myeloid cell composition in subcutaneous Her2 tumors and the parental spontaneous tumor in mammary glands revealed that both lesions contained high frequencies of CD11c+ TAMs (50–72%) and relatively low frequencies of TANs (<5%) among hematopoietic cells (Fig. 3A and Supplementary Fig. S2B). A strong similarity was observed between the myeloid cell composition of subcutaneous 4T1 tumors and spontaneous metastatic lesions in the peritoneal cavity, occurring within 2–3 weeks after tumor challenge. Similar to primary 4T1 tumors, these metastases are enriched with TAMs (~50%, of which >60% are CD11c+) and TANs (15–21%) among hematopoietic cells (Fig. 3A and Supplementary Fig. S2C). Furthermore, these results were corroborated in a B16 metastatic model. When B16 cells are injected intravenously, tumor nodules grow in lungs and kidneys within 2–3 weeks and contain a similar myeloid landscape to subcutaneous tumors: ~30% TAMs (~45% expressing CD11c) and 4–9% TANs among hematopoietic cells (Fig. 3A and Supplementary Fig. S2D). Altogether, these data provide evidence that tumor type rather than its anatomic location shapes the myeloid infiltrate.
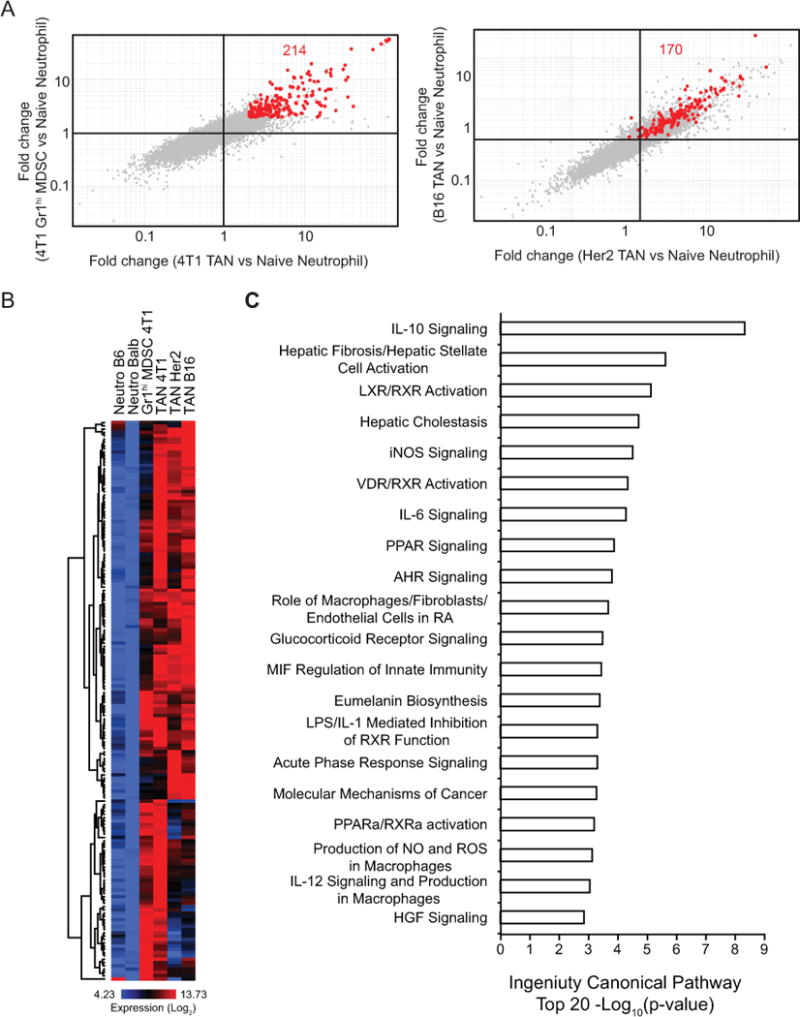
A, Myeloid cells within s.c. and mammary gland of transgenic Her2 tumors (n=4–7); within primary s.c. and spontaneous peritoneal metastatic 4T1 tumors (n=3); and within s.c. B16 tumors or lung and kidney metastases (n=3–4). B, Left, Analysis of myeloid subsets within tumors from Balb/c mice with single (Her2 or 4T1, n=5) or double (Her2 and 4T1 on opposite flanks, n=8) tumors. Right, Frequency of myeloid cells in the spleens of tumor-bearing mice. C, Left, Analysis of myeloid cell subsets within tumors from F1 (Balb/c × C57BL/6) mice with single (Her2 or B16, n=3) or double (Her2 and B16 on opposite flanks, n=3) tumors. Right, Frequency of myeloid cells in the spleens of tumor-bearing mice. Numbers indicate percentage of cells for each gate or region. N.S., not significant, * P<0.05, *** P<0.001.
Next, we tested the possible dominant effect of the microenvironment in dictating myeloid cell composition by analyzing myeloid cells in mice with two tumors growing simultaneously. For this purpose, we compared Her2 and 4T1 or Her2 and B16 tumors, as each of these tumors has distinct myeloid infiltrates (Figs. 1 and and2).2). Balb/c mice were inoculated with Her2 tumors under one flank and simultaneously with 4T1 tumors under the contralateral flank (Fig. 3B and Supplementary Fig. 2E). Similarly, we established Her2 tumors in the presence of B16 tumors on the opposite flank in F1 (Balb/c × C57BL/6) mice (Figure 3C and Supplementary Fig. 2F). In both models, we did not observe a difference in growth rate of either tumor when compared with tumors grown alone (data not shown). Likewise, the myeloid content of each tumor, either alone or in the presence of a second tumor in the same host was similar (Figs. 3B–C, left and Supplementary Figs. S2E–F), even though there was pronounced systemic accumulation of MDSCs associated with 4T1 tumor growth (Figs. 3B–C, right). Overall, these results suggest that the signals attracting specific myeloid cells into tumors are driven by cancer cells in the primary lesion regardless of anatomic location or presence of distant signals from other microenvironments.
Tumor-infiltrating myeloid cells exhibit a hypoxia-associated signature compared to their splenic counterparts
As shown in Fig. 1, MDSCs accumulate in the spleens of 4T1 tumor-bearing mice at high frequencies. As splenic MDSCs may serve as reservoirs for tumor-infiltrating cells [6], it is important to understand how they relate to their counterparts within tumors. Thus, we compared the gene expression profiles of CD11b+Gr1+ cells from the spleens and tumors of 4T1 tumor-bearing mice. As shown in Fig. 4A, 263 probes were upregulated in TAMs (both CD11c− and CD11c+ subsets) within 4T1 tumors compared to Gr1low MDSCs from spleens, whereas 152 probes were enriched in splenic cells. In addition, 201 probes were enriched in TANs compared to splenic Gr1hi MDSCs, while 647 probes were enriched in Gr1hi MDSCs (Fig. 4B). Next, we compared expression of probes upregulated in 4T1 tumors to that of myeloid cells originated in other tumor models. Hierarchical clustering indicated that TAMs, TANs, and splenic MDSC populations clustered separately, confirming differences between tumor and splenic myeloid cells. Notably, similar profiles were obtained from equivalent populations originated from different tumor types (Fig. 4C), supporting that transcriptomic analysis may illuminate universal features of tumor myeloid cells. Indeed, this analysis identified several genes that highlight the pro-tumorigenic roles of tumor-infiltrating myeloid cells. For example, genes involved in extracellular matrix remodeling (Mmp12, Mmp13, Mmp14, Adam8, Tgm2), immunomodulation (Arg1, Nos2, Cd274, Ptgs2, Spp1) and hypoxia regulation (Vegfa, Hif1a, Slc2a1) were markedly upregulated in TAMs and TANs compared with splenic MDSCs. Interestingly, expression of these genes was similar between CD11b−CD103+ tumor DCs, and DCs from tumor-free mice. This result suggests that hypoxic responses are not uniform within the same microenvironment and may be tightly regulated among tumor-infiltrating leukocytes. Immunosuppression and tissue remodeling are critical functions for promoting tumor progression, and hypoxia, through hypoxia inducible factor 1α (Hif-1α) [18], can promote transcriptional changes that facilitate the selection of aggressive tumor cells. Expression analysis of Hif-1α-regulated hypoxia-associated genes [19]revealed that these genes are indeed enriched in TAMs and TANs compared to splenic MDSCs and steady-state populations, indicating reprogramming in the tumor environment (Fig. 4D and Supplementary Table S2). Hypoxia and Hif-1α have been implicated in prolonged survival and reduced oxidative burst in neutrophils [20,21], and as such may also contribute to the accumulation of these short-lived cells.
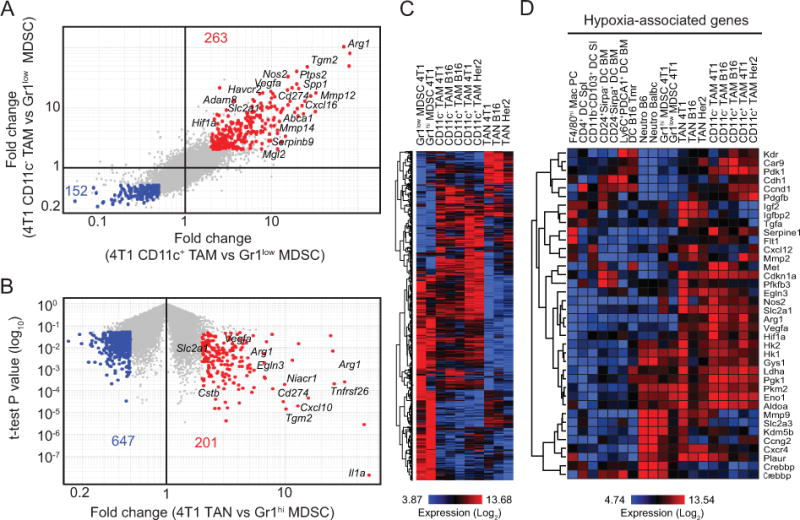
A, Comparison of gene expression profiles of CD11c+ and CD11c− TAMs to Gr1low MDSCs from 4T1 tumor-bearing mice based on 2-fold change (fold-change vs fold-change plot). B, Comparison of gene expression profiles of TANs and Gr1hi MDSCs from 4T1 tumor-bearing mice (fold-change vs t-test P value plot). C, Heatmap generated by hierarchical clustering using probes identified in A and B for all tumor myeloid subsets. D, Heatmap showing the expression of hypoxia-related genes by tumor-associated and steady-state myeloid cells. Selected genes associated with the populations are indicated on the plots.
Neutrophils in the tumor environment are pro-tumorigenic and exhibit an altered functional profile
Our finding that the myeloid cell landscape of each tumor type is distinctive raises the possibility that different tumor microenvironments impart unique functional attributes in the myeloid cells therein. While TAMs in different tumors have been extensively characterized by functional and transcriptional profiling approaches [22–26], our understanding of TANs is limited. Recently, Fridlender et al. compared steady-state neutrophils to splenic MDSCs and TANs in a mesothelioma model by transcriptional profiling and identified striking differences [27]. However, this study focused only on a single tumor and it is not known if such characteristics of TANs are common across different tumors. By comparing TANs from 4T1, B16, and Her2 tumors to Gr1hi MDSCs from 4T1 tumor-bearing mice and neutrophils from the bone marrow of naive mice (Fig. 4), we identified a conserved expression pattern of immunomodulatory and hypoxia-related genes across these tumors. We also compared genes highly expressed in both Gr1hi MDSCs and TANs from 4T1 tumors to their expression in steady-state neutrophils (Fig. 5A, left) and found 214 probes selectively enriched in 4T1-associated neutrophils. Interestingly, many of these genes were similarly enriched in TANs from B16 and Her2 tumors compared to steady-state neutrophils (170 out of 214 passed CV<0.5 filter) (Fig. 5A, right). Heatmap analysis also indicated that these probes were highly expressed by all TANs and Gr1hi MDSCs compared to steady-state neutrophils (Fig. 5B and Supplementary Table S3). Importantly, these genes are involved in pathways critical for tumor growth and immune responses, such as iNOS, IL-10, IL-6 and peroxisome proliferator-activated receptor (PPAR) signaling (Fig. 5C). Collectively, our data support that tumor-associated myeloid cells exhibit a pro-tumorigenic transcriptional program.
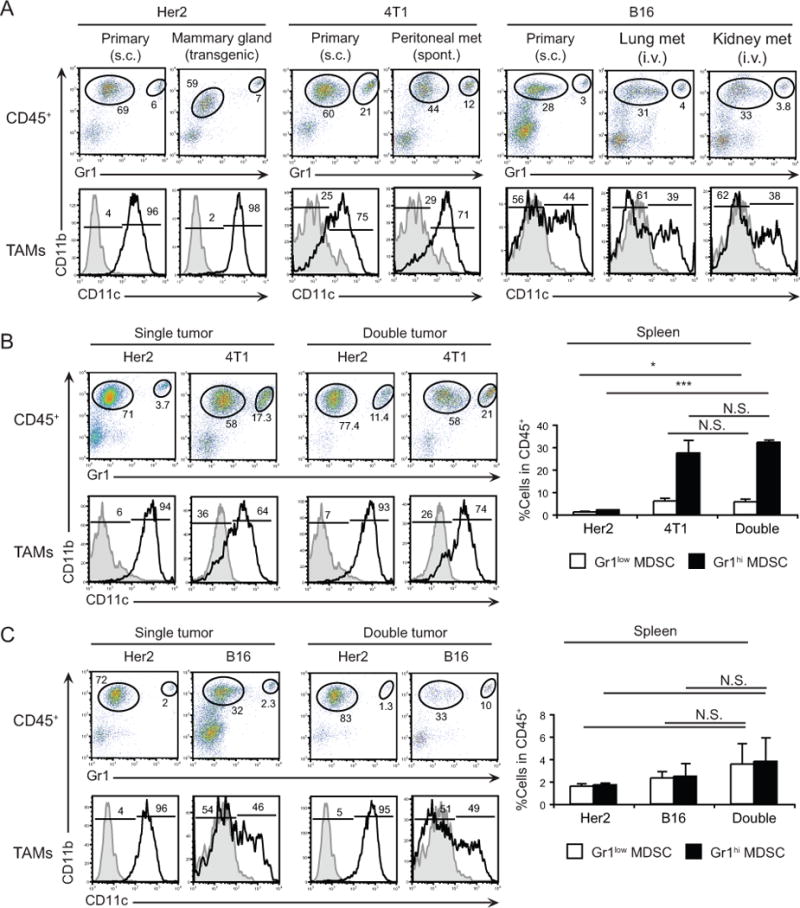
A, Comparison of gene expression profiles of TANs and Gr1hi MDSCs from 4T1 tumor-bearing mice to naive neutrophils (left, fold-change vs fold-change, 2-fold change). Expression of these probes was further analyzed in fold-change vs fold-change plots comparing TANs from B16 and Her2 tumors to naive neutrophils (right). 170 of the 214 probes passed the CV<0.5 filter. B, Heatmap showing the expression of the 170 probes identified in A across neutrophils. C, Top 20 canonical pathways enriched for TANs and Gr1hi MDSCs compared to naive neutrophils analyzed by Ingenuity Pathway Analysis.
To further interrogate molecular programs in TANs across different tumors, a side-by-side comparison of TANs from 4T1, Her2 and B16 tumors was performed. We identified 43 probes enriched in 4T1 TANs, 61 in Her2 TANs, and 93 in B16 TANs (Fig. 6A). Genes involved in cell trafficking or differentiation, such as Cxcr1, Foxo3, Slc28a2, were enriched 2–5 fold in 4T1 TANs and may regulate the marked accumulation of TANs in this cancer type [28]. Given that our data point to a direct, tumor cell-specific involvement in the recruitment and expansion of TANs, we analyzed the expression levels of cytokines, cytokine receptors, chemokines, and chemokine receptors by TANs, as these may account for the tumor-myeloid cell cross-talk regulating cell infiltration (Fig. 6B and Supplementary Table S4). Strikingly, we identified different expression profiles for each TAN population, which may reflect the differences observed in the frequencies of these populations in each tumor. In particular, TANs from 4T1 tumors expressed significantly greater levels of Ltb4r1 (BLT1), a leukotriene B4 chemotactic factor for neutrophils [29,30]. Interestingly, we also found enrichment of genes in the anti-inflammatory PPARα signaling pathway (Fig. 5D), which is also a target for leukotriene B4. We further analyzed the expression of PPARα target genes associated with inflammation [31] in neutrophil subsets (Fig. 6C and Supplementary Table S5). While many of these genes are expressed at higher levels in TANs compared to Gr1hi MDSCs and steady-state neutrophils, neutrophils from 4T1-bearing mice expressed higher levels of Stat1, Stat2, and Stat3, among other genes. Stat3 is a mediator of CXCR2 expression in neutrophils upon G-CSF stimulation [32]. In agreement with this, G-CSF is highly expressed by 4T1 cells (Fig. S2A) and Cxcr2 is expressed at higher levels by 4T1 TANs (Fig. 6B). Overall, these signaling pathways may incite the marked accumulation of TANs and Gr1hi MDSCs in the 4T1 model. The relationship between neutrophil populations from different tumors was also confirmed by the principal component analysis to highlight functional differences. Neutrophils from naive mice clustered separately and more closely to MDSCs on PC2 (Fig. 6D). On the other hand, while TANs clustered separately from neutrophils and MDSCs, variability on PC1 reflected tumor-to-tumor differences among TANs.
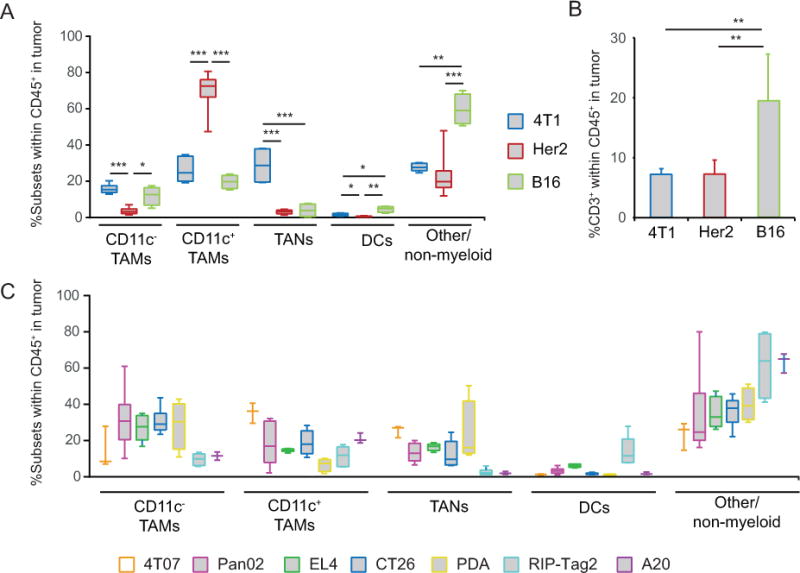
A, Fold-change vs fold-change plot comparing 4T1 TANs to TANs in Her2 and B16, based on 2-fold change. Selected genes associated with the populations are indicated on the plots. B, Chemokine, chemokine receptor, cytokine, and cytokine receptor expression profiles of TANs. C, PPARα-associated gene expression profiles of neutrophils. D, Principal component analysis of neutrophil populations.
Haptoglobin levels are associated with myeloid cell accumulation
During acute inflammatory responses, neutrophils exocytose effector molecules stored in specific granules [33]. Recently, Fridlender et al showed that some of these molecules are actually down-regulated in TANs compared to those in steady-state neutrophils and Gr1hi MDSCs [27]. To understand how this critical function is influenced by different tumors, we compared the expression of these effector molecules in steady-state neutrophils, in splenic Gr1hi MDSCs from 4T1 tumor-bearing mice, and in TANs from 4T1, Her2, and B16 tumors. Heatmap analysis and hierarchical clustering indicated that all TANs shared an altered expression profile compared to naive granulocytes (Fig. 7A and Supplementary Table S6). Interestingly, Gr1hi MDSCs expressed the majority of the effector molecules. Among the molecules associated with neutrophil granules is the acute phase responder Haptoglobin (HP) [34]. HP is known to be produced in the liver, however recent studies indicated that it is also expressed by neutrophils and packaged into secretory granules [35]. Analysis of Hp expression by tumor-associated myeloid cells revealed that Hp is not expressed by DCs or CD11c+ TAMs in tumors, and its level is moderate in CD11c− TAMs and in TANs from B16 and Her2 tumors (Fig. 7B). However, Hp expression is high in naive granulocytes, Gr1hi MDSCs, and TANs from 4T1 tumors. Accordingly, serum HP levels were elevated in mice bearing advanced 4T1 tumors but not in Her2 or B16 tumor-bearing hosts (Fig. 7C). Indeed, serum HP levels correlated with the frequency of Gr1hi MDSCs in the blood of 4T1 tumor-bearing mice (Fig. 7D). This select pattern suggests that HP expression may be associated with the considerable myelopoiesis observed in the 4T1 setting (Supplementary Fig. S1E). Although further studies are required to confirm a direct relationship between neutrophils and HP levels, we detected elevated serum HP in breast cancer patients (Fig. 7E), consistent with reports for glioblastoma and ovarian cancer patients [36,37]. Thus, HP may serve as a diagnostic marker indicative of cancers with myeloid cell accumulation.
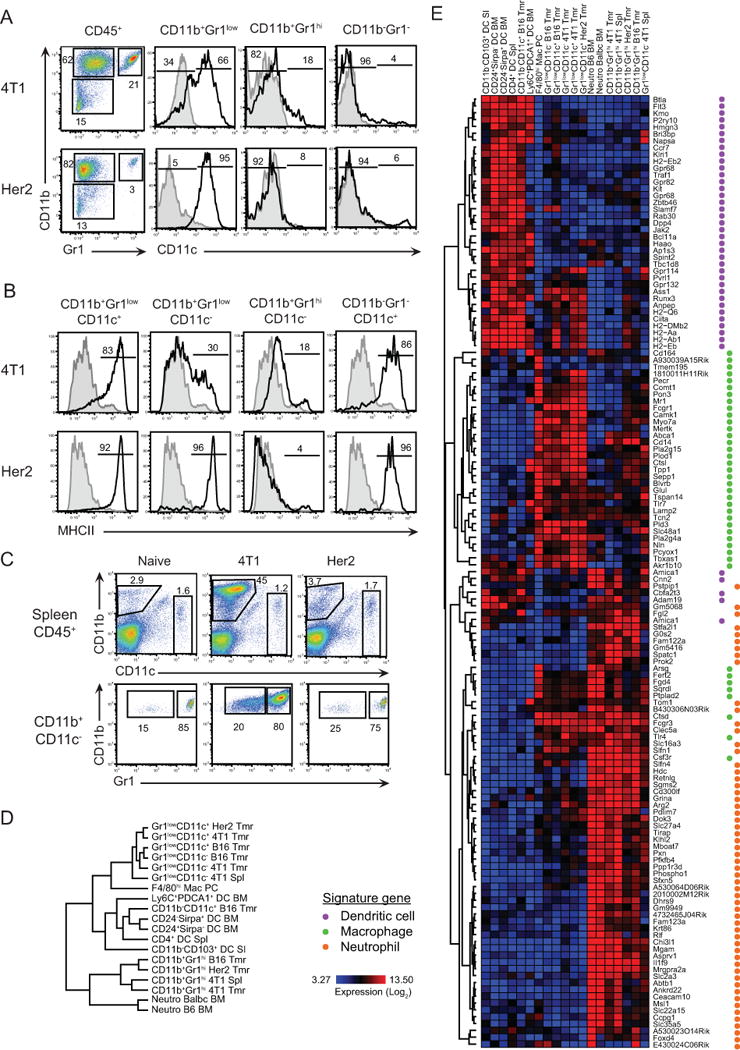
A, Expression of granular effector molecules by neutrophils. B, Mean expression value of haptoglobin (Hp) across neutrophils and tumor-associated myeloid cells. C, Serum HP levels in mice with small (S), medium (M), large (L) tumors and naive mice. D, Correlation between the frequency of Gr1hi cells in blood and the serum HP concentration. E, HP levels in serum from healthy donors (n=4) and breast cancer patients (n=10). * P<0.05; ** P<0.01.
Tumors require a sustained influx of myeloid cells to support angiogenesis, remodel peritumoral stroma, and suppress antitumor immunity. Therefore, tumor-associated myeloid cells represent potential targets to improve immunotherapy as well as chemotherapy against cancer. Although there are limited strategies available, identifying novel targets for elimination or functional differentiation of these cells requires a deeper understanding of their development, trafficking, and phenotypic and functional characteristics. Moreover, to ascertain whether a broader therapeutic application is feasible, it is critical to determine if key molecular attributes are conserved among specific myeloid populations from tumors of different origins. A better understanding of the functional differences among discrete myeloid subsets in tumor lesions as well as secondary lymphoid organs may lay the groundwork for development of novel therapeutics. In this regard, our study provides fundamental insights into functional differences of tumor-infiltrating myeloid cells, and identifies potential molecular pathways that could be investigated for therapies aimed at reducing infiltration and/or reprogramming function of tumor myeloid cells.
Supplementary Material
1
2
3
4
5
6
7
8
9
Acknowledgments
We thank the Immunological Genome Project team for technical help and discussions; Drs. Dobrin Draganov and Glenn Dranoff for the Her2 cell line; and Dr. Ronald DePinho for PDA mice. This study was supported by research funding from NIH T32 grant to KGE, NIH T32 CA 070083-15 and Postdoctoral Fellowship from the Cancer Research Institute to VC, NIH grants (AG028082, AG033049, AI098108, AI101918) and an Established Investigator Award (0940006N) from American Heart Association to DRG, NIH grants (ROI DK074500, PO1 AI045757, R21 CA182598), American Cancer Society Research Scholar Grant, and Claudia Adams Barr Award for Innovative Cancer Research to SJT.
Abbreviations and acronyms
TAM | tumor associated-macrophage |
TAN | tumor associated-neutrophil |
MDSC | myeloid derived suppressive cell |
DC | dendritic cell |
HP | haptoglobin |
References
Full text links
Read article at publisher's site: https://doi.org/10.1158/2326-6066.cir-13-0209
Read article for free, from open access legal sources, via Unpaywall:
https://aacrjournals.org/cancerimmunolres/article-pdf/2/7/655/2347410/655.pdf
Citations & impact
Impact metrics
Citations of article over time
Alternative metrics
Smart citations by scite.ai
Explore citation contexts and check if this article has been
supported or disputed.
https://scite.ai/reports/10.1158/2326-6066.cir-13-0209
Article citations
BatchFLEX: feature-level equalization of X-batch.
Bioinformatics, 40(10):btae587, 01 Oct 2024
Cited by: 0 articles | PMID: 39360977 | PMCID: PMC11486499
Construction of a 12-Gene Prognostic Risk Model and Tumor Immune Microenvironment Analysis Based on the Clear Cell Renal Cell Carcinoma Model.
Cancer Control, 31:10732748241272713, 01 Jan 2024
Cited by: 0 articles | PMID: 39115042 | PMCID: PMC11311166
Human serous cavity macrophages and dendritic cells possess counterparts in the mouse with a distinct distribution between species.
Nat Immunol, 25(1):155-165, 15 Dec 2023
Cited by: 6 articles | PMID: 38102487
Chronic psychological stress promotes breast cancer pre-metastatic niche formation by mobilizing splenic MDSCs via TAM/CXCL1 signaling.
J Exp Clin Cancer Res, 42(1):129, 20 May 2023
Cited by: 10 articles | PMID: 37210553 | PMCID: PMC10199643
Activated fibroblasts in cancer: Perspectives and challenges.
Cancer Cell, 41(3):434-449, 01 Mar 2023
Cited by: 73 articles | PMID: 36917949
Review
Go to all (54) article citations
Data
Data behind the article
This data has been text mined from the article, or deposited into data resources.
BioStudies: supplemental material and supporting data
GEO - Gene Expression Omnibus (2)
- (1 citation) GEO - GSE15907
- (1 citation) GEO - GSE37448
Similar Articles
To arrive at the top five similar articles we use a word-weighted algorithm to compare words from the Title and Abstract of each citation.
Modulating the expression of IFN regulatory factor 8 alters the protumorigenic behavior of CD11b+Gr-1+ myeloid cells.
J Immunol, 183(1):117-128, 01 Jul 2009
Cited by: 31 articles | PMID: 19542426 | PMCID: PMC2744444
TFEB is a master regulator of tumor-associated macrophages in breast cancer.
J Immunother Cancer, 8(1):e000543, 01 Jun 2020
Cited by: 38 articles | PMID: 32487570 | PMCID: PMC7269543
The Lineage-Defining Transcription Factors SOX2 and NKX2-1 Determine Lung Cancer Cell Fate and Shape the Tumor Immune Microenvironment.
Immunity, 49(4):764-779.e9, 01 Oct 2018
Cited by: 112 articles | PMID: 30332632 | PMCID: PMC6197489
Tuning the Tumor Myeloid Microenvironment to Fight Cancer.
Front Immunol, 10:1611, 25 Jul 2019
Cited by: 70 articles | PMID: 31402908 | PMCID: PMC6673698
Review Free full text in Europe PMC
Funding
Funders who supported this work.
NCI NIH HHS (3)
Grant ID: T32 CA070083
Grant ID: R21 CA182598
Grant ID: T32 CA 070083-15
NIA NIH HHS (4)
Grant ID: AG028082
Grant ID: K02 AG033049
Grant ID: AG033049
Grant ID: R01 AG028082
NIAID NIH HHS (5)
Grant ID: AI101918
Grant ID: R21 AI098108
Grant ID: R21 AI101918
Grant ID: P01 AI045757
Grant ID: AI098108
NIDDK NIH HHS (2)
Grant ID: R0I DK074500
Grant ID: R01 DK074500