Abstract
Free full text

High-density genotyping of immune-related loci identifies new SLE risk variants in individuals with Asian ancestry
Associated Data
Abstract
Systemic lupus erythematosus (SLE) has a strong but incompletely understood genetic architecture. We conducted an association study with replication in 4,492 SLE cases and 12,675 controls from six East-Asian cohorts, to identify novel and better localize known SLE susceptibility loci. We identified 10 novel loci as well as 20 known loci with genome-wide significance. Among the novel loci, the most significant was GTF2IRD1-GTF2I at 7q11.23 (rs73366469, Pmeta=3.75×10−117, OR=2.38), followed by DEF6, IL12B, TCF7, TERT, CD226, PCNXL3, RASGRP1, SYNGR1 and SIGLEC6. We localized the most likely functional variants for each locus by analyzing epigenetic marks and gene regulation data. Ten putative variants are known to alter cis- or trans-gene expression. Enrichment analysis highlights the importance of these loci in B- and T-cell biology. Together with previously known loci, the explained heritability of SLE increases to 24%. Novel loci share functional and ontological characteristics with previously reported loci, and are possible drug targets for SLE therapeutics.
SLE is a debilitating autoimmune disease (AID) characterized by pathogenic autoantibody production that can affect virtually any organ. Asians have higher SLE incidence, more severe disease manifestations, and greater risk of organ damage (e.g., lupus nephritis)1,2 compared to European-derived populations. SLE has a strong genetic component, e.g. a sibling risk ratio3 (λs) of ~30, with ~40 susceptibility loci reported through candidate gene and genome-wide association studies (GWAS)4–6. However, only 8–15% of disease heritability7,8 is accounted for, leaving many contributing loci unidentified. Since multiple susceptibility loci are shared among AIDs, and studying high-risk populations can facilitate novel risk locus identification, we performed high-density association analysis in East-Asians.
Our study was conducted in three stages (Fig. 1, Online Methods). First, we used ImmunoChip9-based association analysis in 2485 cases and 3947 controls from Koreans (KR), Han Chinese (HC) and Malaysian Chinese (MC), and identified 578 associated regions (P<5×10−3) (Supplementary Fig. 1, Supplementary Tables 1–3). To increase statistical power, we included 3669 out-of-study KR controls (Supplementary Table 1, Supplementary Fig. 2), and conducted imputation-based association analysis (Online Methods). Second, we followed up 16 novel loci with PDiscovery-meta<5×10−5 in three replication cohorts: one Japanese (JAP), and two independent Han Chinese from Beijing (BHC) and Shanghai (SHC). We identified 10 novel loci with genome-wide significance (GWS; Pmeta< 5×10−8, Table 1, Figs. 2,,3,3, Supplementary Fig. 3), and 6 novel suggestive loci (Supplementary Table 4). Third, we used a series of bioinformatic analyses including two recently developed Bayesian-based tests10,11 (Online Methods) to identify the most likely functional variants at each locus. Since the lead SNPs might not be functional, we examined SNPs in high linkage disequilibrium (LD; r2>0.8). Variants were annotated using Encyclopedia of DNA Elements (ENCODE)12 and Blood eQTL data13. We estimated the proportion of the heritability and sibling risk (λs) explained by novel and known SLE loci.
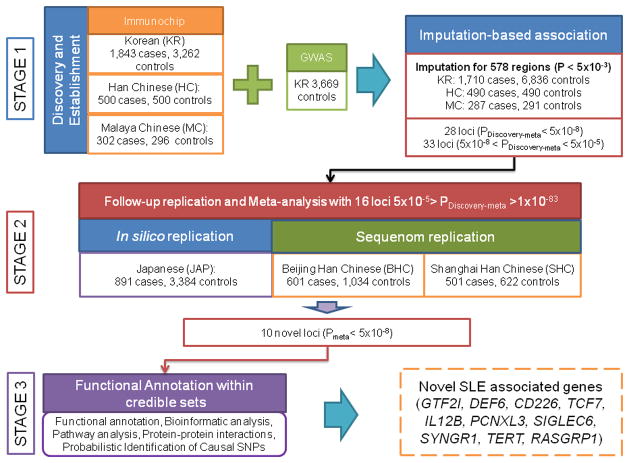
Flowchart of our experimental design. This study followed three stages: in Stage 1, we genotyped three Asian cohorts of SLE patients and controls and identified 578 regions with P<5×10−3. Next we performed imputation-based fine-mapping, association tests and conditional analysis on the quality controlled data. We identified 16 statistically independent loci (P<5×10−5) for replication. In Stage 2, we performed an in silico replication of these 16 loci in an independent Japanese (JAP) cohort, and two independent Chinese cohorts from Shanghai (SHC) and Beijing (BHC). We identified novel regions represented by 10 replicated SNPs that passed the genome-wide significance threshold (P < 5×10−8). In Stage 3, we performed integrated functional and interaction analyses of SLE loci.
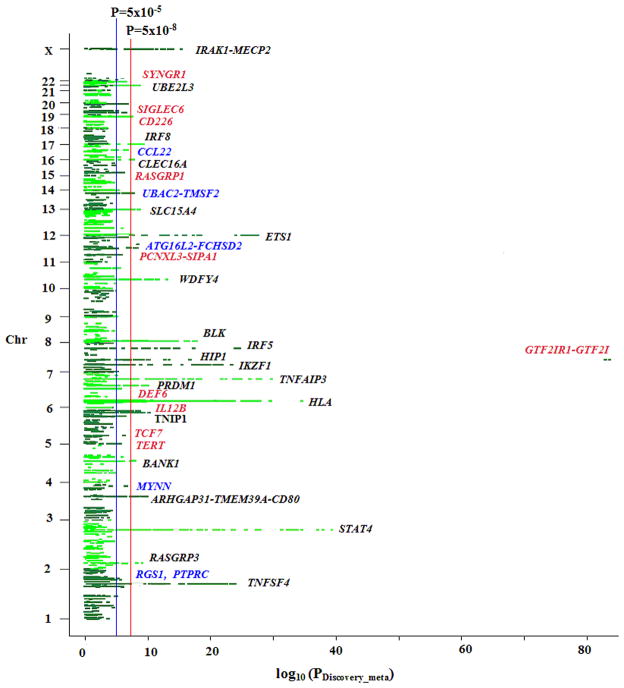
Manhattan plot of the meta-analysis results using discovery sets. Novel significant loci are highlighted in red, “suggestive” loci are in blue and previously known SLE loci are in black.
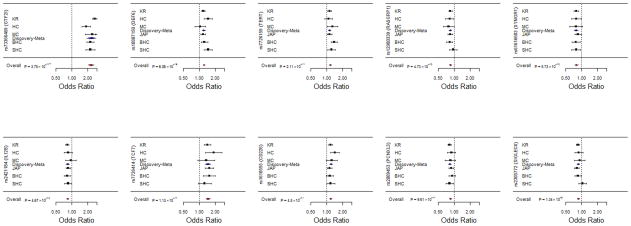
Meta-analysis of lead SNPs from 10 novel genes. We identified 10 novel loci in KR, HC and MC cohorts that were replicated in at least 2 independent cohorts. A partial Discovery-meta-analysis is presented in the middle of the plot, and the overall Meta-analysis is presented below the replication cohorts. KR: Korean; HC: Han Chinese; MC: Malaysian Chinese; JAP: Japanese; BHC: Beijing Han Chinese; SHC: Shanghai Han Chinese.
Table 1
Meta-analysis results for novel and suggestive loci associated with SLE in Asian cohorts.
SNP (Alleles) | Gene | DISCOVERY COHORTS | REPLICATION COHORTS | OVERALL META ANALYSIS | ||||||||||||
---|---|---|---|---|---|---|---|---|---|---|---|---|---|---|---|---|
KR 1710 cases, 6836 controls | HC 490 cases, 493 controls | MC 287 cases, 291 controls | JAP 891 cases, 3384 controls | SHC 501 cases, 622 controls | BHC 601 cases, 1034 controls | 4480 cases, 12660 controls | ||||||||||
MAF | P value | MAF | P value | MAF | P value | MAF | P value | MAF | P value | MAF | P value | Pmeta | OR | CI | ||
NOVEL SIGNALS | ||||||||||||||||
rs73366469 (C/T) | GTF2I | 0.29/0.13* | 1.47×10−69 | 0.36/0.23 | 1.71×10−9 | 0.25/0.12 | 1.53×10−7 | NA | NA | 0.30/0.16 | 1.17×10−16 | 0.33/0.18 | 3.57×10−21 | 3.75×10−117 | 2.38 | 2.22–2.56 |
rs10807150 (C/T) | DEF6 | 0.36/0.32 | 9.21×10−6 | 0.41/0.32 | 2.49×10−4 | 0.37/0.36 | 5.81×10−1 | 0.39/0.35 | 3.34×10−4 | 0.41/0.32 | 2.90×10−6 | 0.38/0.33 | 1.36×10−3 | 6.06×10−16 | 1.25 | 1.19–1.32 |
rs2421184 (G/A) | IL12B | 0.49/0.54 | 4.03×10−8 | 0.49/0.53 | 1.54×10−1 | 0.46/0.47 | 8.93×10−1 | 0.47/0.51 | 1.35×10−3 | 0.49/0.53 | 4.69×10−2 | 0.53/0.58 | 6.53×10−2 | 4.67×10−12 | 0.84 | 0.80–0.88 |
rs7726414 (T/C) | TCF7 | 0.07/0.05 | 1.20×10−4 | 0.09/0.05 | 4.77×10−3 | 0.13/0.10 | 8.17×10−2 | 0.09/0.06 | 3.66×10−4 | 0.10/0.08 | 4.21×10−2 | 0.09/0.06 | 1.19×10−2 | 1.13×10−11 | 1.40 | 1.27–1.54 |
rs7726159 (A/C) | TERT | 0.40/0.37 | 2.10×10−4 | 0.44/0.42 | 1.61×10−1 | 0.48/0.42 | 3.70×10−2 | 0.38/0.35 | 7.60×10−3 | 0.46/0.41 | 1.21×10−2 | 0.49/0.41 | 6.92×10−6 | 2.11×10−11 | 1.21 | 1.14–1.28 |
rs1610555 (T/G) | CD226 | 0.43/0.39 | 2.19×10−5 | 0.38/0.30 | 1.10×10−3 | 0.37/0.32 | 7.16×10−2 | 0.41/0.38 | 7.70×10−3 | 0.38/0.34 | 4.77×10−2 | 0.34/0.31 | 3.86×10−2 | 4.50×10−11 | 1.19 | 1.13–1.26 |
rs2009453 (T/C) | PCNXL3 | 0.40/0.45 | 6.13×10−7 | 0.42/0.45 | 2.08×10−1 | 0.43/0.47 | 1.52×10−1 | 0.37/0.40 | 2.56×10−3 | 0.43/0.48 | 2.43×10−2 | 0.41/0.43 | 3.25×10−1 | 9.61×10−11 | 0.84 | 0.8–0.89 |
rs12900339 (G/A) | RASGRP1 | 0.40/0.44 | 5.85×10−5 | 0.46/0.51 | 1.22×10−1 | 0.46/0.52 | 3.32×10−2 | 0.36/0.40 | 3.52×10−4 | 0.51/0.52 | 8.94×10−1 | 0.45/0.50 | 9.00×10−3 | 4.73×10−10 | 1.18 | 1.12–1.24 |
rs61616683 (C/T) | SYNGR1 | 0.13/0.16* | 4.50×10−4 | 0.20/0.24 | 2.00×10−2 | 0.19/0.23 | 7.00×10−2 | 0.14/0.16 | 2.00×10−2 | 0.20/0.24 | 1.30×10−2 | 0.18/0.22 | 4.00×10−3 | 5.73×10−10 | 0.79 | 0.73–0.85 |
rs2305772 (A/G) | SIGLEC6 | 0.42/0.46 | 2.33×10−6 | 0.41/0.44 | 2.22×10−1 | 0.39/0.41 | 5.17×10−1 | 0.40/0.45 | 3.90×10−4 | 0.42/0.41 | 6.97×10−1 | 0.42/0.46 | 3.40×10−2 | 1.34×10−9 | 0.86 | 0.81–0.9 |
SUGGESTIVE SIGNALS | ||||||||||||||||
rs11235604 (T/C) | ATG16L2-FCHSD2 | 0.07/0.10 | 2.69 ×10−9 | 0.09/0.1 | 5.19 ×10−1 | 0.09/0.12 | 1.71 ×10−1 | 0.07/0.09 | 2.45 ×10−2 | 0.08/0.09 | 6.98 ×10−1 | 0.09/0.10 | 5.87 ×10−1 | 1.90 ×10−9 | 0.76 | 0.69–0.84 |
rs10936599 (G/A) | MYNN-LRRC34 | 0.44/0.39 | 5.39 ×10−7 | 0.49/0.46 | 1.15 ×10−1 | 0.46/0.48 | 3.16 ×10−1 | 0.39/0.36 | 1.49 ×10−2 | 0.49/0.46 | 9.19 ×10−2 | 0.48/0.46 | 1.27 ×10−1 | 4.93 ×10−9 | 0.87 | 0.82–0.91 |
rs223881 (C/T) | CCL22 | 0.46/0.49 | 9.31 ×10−5 | 0.46/0.53 | 1.29 ×10−3 | 0.51/0.59 | 7.23 ×10−3 | 0.36/0.40 | 2.60×10−3 | 0.54/0.53 | 9.08 ×10−1 | 0.50/0.51 | 5.94 ×10−1 | 2.06 ×10−8 | 0.87 | 0.84–0.93 |
rs1885889 (A/G) | UBAC2 | 0.41/0.36 | 6.34 ×10−7 | 0.40/0.37 | 1.38 ×10−1 | 0.43/0.36 | 1.43 ×10−2 | 0.43/0.41 | 2.25 ×10−1 | 0.37/0.38 | 8.53 ×10−1 | 0.38/0.37 | 4.47 ×10−1 | 3.17 ×10−7 | 1.14 | 1.08–1.2 |
rs7556469 (G/A) | PTPRC | 0.12/0.15 | 2.33 ×10−6 | 0.11/0.14 | 1.45 ×10−1 | 0.11/0.10 | 4.94 ×10−1 | 0.17/0.17 | 3.86 ×10−1 | 0.12/0.14 | 1.59 ×10−1 | 0.13/0.15 | 1.85 ×10−1 | 1.95 ×10−6 | 0.84 | 0.78–0.90 |
rs12022418 (C/A) | RGS1 | 0.32/0.36 | 1.53 ×10−4 | 0.29/0.32 | 1.90 ×10−1 | 0.31/0.38 | 1.26 ×10−2 | 0.35/0.37 | 1.95 ×10−1 | 0.32/0.35 | 9.56 ×10−2 | 0.33/0.32 | 6.82 ×10−1 | 1.12 ×10−5 | 0.88 | 0.83–0.93 |
KR = Korean; HC = Han Chinese; MC = Malaysian Chinese; JAP = Japanese; BHC = Beijing Han Chinese; SHC = Shanghai Han Chinese; Alleles = Minor/major allele; MAF = minor allele frequency for cases/controls; OR = odds ratio; CI = confidence interval.
The strongest novel signal [Pmeta=3.75×10−117, ORmeta(95% CI)=2.38 (2.22–2.56)] is at rs73366469 between two “general transcription factor” genes14 GTF2I and GTF2IRD1 (Supplementary Table 5). Surprisingly this signal is much stronger than human leukocyte antigen (HLA). Notably, rs117026326 within GTF2I (92kb from rs73366469) was recently identified as a major risk locus for primary Sjögren’s syndrome (SS), another AID, in HC15 and Southern Chinese16. Two recent SS GWAS15,17 showed substantial overlap with SLE18, emphasizing the validity and immune significance of this region. To confirm the veracity of this extraordinary association signal, we genotyped 2–6 SNPs (including rs73366469) in ~40% of our discovery samples, and in two replication cohorts (Supplementary Table 6). Associations were consistently replicated; rs117026326 showed the strongest association, but is linked to rs73366469 (r2KR=0.76; r2BHC=0.65; r2SHC=0.64 in controls), making it difficult to separate their effects (Supplementary Table 6). Interestingly, conditional analysis on four SNPs showed that rs80346167 (GTF2IRD1) was independent in KR, supporting involvement of both genes. However, due to the strong correlation structure between variants, genotyping and fine-mapping at larger scale are required to further delineate this signal. ENCODE data indicate that high-LD SNPs rs7800325 (r2 = 0.99) and in/del rs587608058 (r2=0.81), ~1000bp from rs73366469, lie within conserved enhancers, active chromatin and transcription factor binding sites (TFBSs) in CD4+ T-cells and GM12878 lymphoblastoid cells (Supplementary Fig. 4a). Chromatin interaction analysis by paired-end tag sequencing (ChIA-PET) and chromosome conformation capture (Hi-C) showed that this region overlaps transcription start sites for GTF2I and VGF (Supplementary Tables 7,8, Supplementary Fig. 5).
The second strongest signal is at intronic rs10807150 (DEF6, Pmeta=6.06×10−16) and correlated rs8205 (ZNF76 promoter, r2=1), a cis-eQTL altering expression of ZNF76 and DEF6 (Supplementary Tables 5,9). Nearby SNP rs4711414 (r2=0.91) alters a highly conserved promoter/TFBS cluster (Supplementary Fig. 4b). The third strongest signal is near interleukin-12β (IL12B, rs2421184, Pmeta=4.67×10−12), in a highly conserved enhancer (Supplementary Tables 5,7, Supplementary Fig. 4c).
Among the other novel signals, TCF7 rs7726414 (Pmeta=1.13×10−11) in the distal promoter is highly linked to rs6874758 (r2=0.99), in a conserved enhancer (Supplementary Tables 5,7, Supplementary Fig. 4d). Nearby rs201806887 (r2=0.79) alters a strong enhancer/TFBS cluster. The 5p15.33 signal is an oncogene19 (TERT, intronic rs7726159, Pmeta=2.11×10−11) tightly bound by RNA-binding proteins PABPC1 and SLBP (Supplementary Fig. 4e); high-LD rs7705526 (r2=0.94) has been linked to chronic lymphocytic leukemia20. The CD226 signal was explained by intronic rs1610555 (Pmeta=4.50×10−11), linked (r2= 0.74) to non-synonymous rs763361 (Supplementary Fig. 4f), associated with multiple AIDs. rs763361 is a cis-eQTL for CD226 and also a trans-eQTL for ACRBP and MAP3K7CL (Supplementary Table 9). The signal at PCNXL3 (rs2009453, Pmeta=9.61×10−11) was in strong LD (r2=0.95) with rs931127 (Supplementary Fig. 4g), a cis-eQTL for PCNXL3, SIPA1 and RELA (Supplementary Table 9). The signal at RASGRP1 (rs12900339, Pmeta=4.73×10−10) is connected with multiple chromatin interactions (Supplementary Table 8) as well as correlated (r2=0.77) with rs12324579, a cis-eQTL for C15orf53 (Supplementary Table 9). Intronic rs61616683 (SYNGR1, Pmeta=5.73×10−10), is in active chromatin (Supplementary Fig. 4i), and is a cis-eQTL of SYNGR1 (Supplementary Table 9). Correlated SNP (r2=0.86) rs909685 is associated with rheumatoid arthritis (RA) in Koreans21. Intronic rs2305772 (SIGLEC6, Pmeta=1.34×10−9) is a cis-eQTL for SIGLEC6/SIGLEC12 (Supplementary Table 9) and disrupts a conserved SIGLEC6 splice junction (Supplementary Fig. 4j).
We also confirmed association (P<0.005) with 36 previously reported SLE loci (Supplementary Table 10, Supplementary Fig. 6). Conditional analysis (Online Methods) at each locus identified secondary associations in 3 novel and 10 reported loci (Supplementary Table 11).
As expected, HLA association was replicated in all cohorts (Supplementary Table 10, Supplementary Fig. 7a). The strongest signal was at HLA Class II (rs113164910, PDiscovery-meta=2.48×10−37, OR=1.65), 14 kb 3′ of HLA-DRA. In order to further delineate the HLA signal, we imputed SNPs, classical HLA alleles and HLA amino acid residues in all three cohorts (Online Methods). The most significant association was identified at HLA-DRB1 amino-acid position 13 (P =9.5×10−45) and its linked position 11 ( P = 7.37×10−39), as shown in a recent HLA-fine-mapping study using a subset (~ 60%) of KR22 (Supplementary Table 12). Our results also confirmed the reported associations of the two linked classical alleles, HLA-DRB1*15:01 (P=4.19×10−29) and HLA-DQB1*06:02 (P=6.46×10−26) (Supplementary Table 12; Supplementary Figure 7b). To investigate the secondary effect within and out of HLA-DRB1, we performed a conditional analysis. Consistent with the recent study22, the associations of HLA-DRB1 were almost explained by residues at amino-acid position 13 (and 11) with a primary effect and position 26 (P=4.09×10−17) as a secondary effect. After accounting for the effect of the HLA-DRB1 locus (Online Methods), no new signals were detected. Thus the DRB1 locus explained most of the MHC associations (Supplementary Table 12; Supplementary Figure 7c). Comparing SNP versus classical allele associations, we find that both association results co-locate the strongest effects towards the HLA-DRB1 region (Supplementary Fig. 7b), as evidenced by HLA-DRB1*15:01 and nearby rs113164910.
Additionally, we identified six novel “suggestive” loci (1.9×10−9<Pmeta<1.12×10−5) with three missense variants (Supplementary Tables 4, 13). Although three of these loci (ATG16L2-FCHSD2, MYNN-LRRC34 and CCL22) passed GWS, further replications are needed to confirm their association.
We replicated most of the previously reported genes with the same published or highly correlated SNPs (Supplementary Table 14). We also found four genes with novel uncorrelated SNPs shifting the association peaks in Asians (Supplemental Table 15). Of them, ARHGAP31-TMEM39A-CD80 was of special interest: previously reported association signals from TMEM39A (rs1132200)23 and CD80 (rs6804441)24 were now explained by a novel synonymous SNP in ARHGAP31 (rs2305249, Pmeta=1.64×10−9), a cis-eQTL of B4GALT4 and POGLUT1 (a NOTCH1 signaling regulator25).
To identify the most likely functional variants within a locus, we used Bayesian-based analyses10,11, eQTLs and epigenetic analyses (Online Methods, Supplementary Tables 7–9, 16). We found that lead SNPs in GTF2I, IL12B, PCNXL3, SYNGR1, RASGRP1 and SIGLEC6 had a high probability of being functional (Supplementary Table 17).
To explore biological functions and pathways related to SLE loci (novel and replicated), we performed gene set enrichment analysis (GSEA) (Online Methods). We identified pathways and gene ontology categories (including immunity, inflammation and cytotoxicity) (Supplementary Fig. 8) in common between novel and published loci. Moreover, GSEA with a drug target database26 identified a set of 56 significantly enriched drugs (adjusted P-value <0.05, Supplementary Table 18), including SLE therapeutics27 (cyclosporine, zinc acetate, hydrocortisone, methotrexate), that affected expression of the target loci. Of note was GTF2I, significantly enriched in drugs used for the treatment of leukemia (imatinib, Padj=1.82×10−10) and lymphoma (cisplatin, Padj=2.68×10−4). Immune system involvement was confirmed by enrichment analysis of SLE loci on mouse immune phenotypes, with significant enrichment in abnormal lymphocyte/leukocyte/immune cell physiology and abnormal cell-mediated/adaptive immunity (Supplementary Table 19).
To understand the relationship between our novel loci and known SLE loci, and to identify possible molecular mechanisms involved in SLE pathogenesis, we performed network interaction analsyis28,29 (Online Methods). We found that SLE novel and replicated loci are connected directly and indirectly to each other through gene regulation, protein and biochemical interactions (Supplementary Fig. 9,10). Text-mining methods30 confirmed that many of these loci have strong associations with one another in the literature, and show how the novel loci are related to the replicated loci (Supplementary Fig. 11). Within these relationships, we further identified sub-networks of molecules interacting with our novel loci in the context of known SLE genes (i.e., TERT, IL12B, GTF2I, RELA, SRC and NFKB2 (Supplementary Fig. 12).
We identified only one non-synonymous variant (rs2305772, Pro246Ser/splice junction, SIGLEC6) in LD (r2≥0.8) with the novel SNPs (Supplementary Table 20), suggesting other variants likely contribute to SLE pathogenesis through epigenetic regulation, rather than protein structure/function alterations. Joint analysis of lead and correlated (r2>0.8) SNPs indicated 13-fold enrichment in strong enhancers in K562 and up to 22-fold enrichment in DNase hypersensitivity in MCF-7 cells (Supplementary Table 21).
In six of the ten novel genes (GTF2I, DEF6, CD226, PCNXL3, RASGRP1 and SIGLEC6), highly conserved, ancestral alleles were risk alleles. Except for SIGLEC6, all derived, protective alleles are major alleles in Asians and European-derived populations (CEU); in SIGLEC6, the derived, protective allele is major in only Europeans. Notably, derived risk alleles for SYNGR1 occur at >80% in Asians (CHB+JPT), compared to CEU (~20%), suggesting that SYNGR1 is undergoing selection in Asian populations, as indicated by FST, iHS and XP-EHH analyses (Supplementary Table 22).
We assessed whether regions associated with these SNPs (novel and replicated) harbored genes expressed in distinct immune cell types31 (Online Methods). We identified significant (1×10−9<P<4×10−4) cell type-specific expression of our loci in human B-cells, T-cells, natural killer cells and dendritic cells (Fig. 4, Supplementary Fig. 13a). This result was further strengthened by replication of homologous mouse genes in mouse cell lines, with significant enrichment in CD19+ B-cells (P=1.0×10−5) and transitional B-cells (P=1.0×10−5) (Supplementary Fig. 13b). Thus, our results point to a strong (and conserved) effect of gene expression in B- and T-cells in SLE pathogenesis.
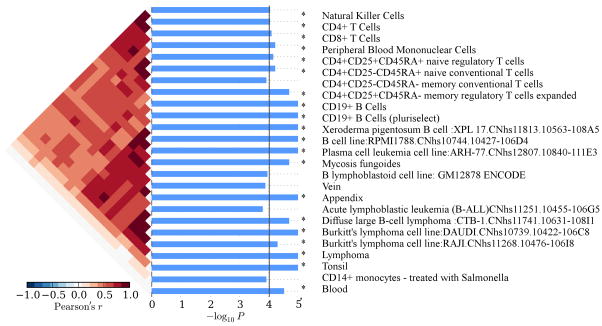
Cell-specific gene expression analysis of the novel and SLE loci. We estimated enrichment of our gene-set in a set of human (FANTOM5) cell lines. Overrepresented cell types have a high correlation (Pearson’s correlation coefficient) of SLE-loci expression (dark red). P-values (blue bars) that passed the multiple testing threshold (black line) show significant enrichment in SLE loci.
Six of the ten novel genes are also associated with other AIDs including celiac disease (CD), RA, T1D, and multiple sclerosis (MS) (Supplementary Table 23), suggesting pleiotropic effects. This pattern extended to suggestive ATG16L2, PTPRC, UBAC2 and RGS1, which are reportedly associated with other AIDs.
Collectively, these novel and known SLE susceptibility variants (47 SNPs) explain 24% of total heritability of SLE in Asians (Supplementary Table 24). Among them, HLA explains 2%, and the 10 novel loci account for 6%. These loci also explain 24% of λs (Supplementary Table 25); novel loci explain 7%. To quantify the predictive effect of these variants, we estimated genetic risk through the weighted genetic risk score (wGRS). Novel risk alleles significantly (P =6.58×10−39) increased the wGRS area under the curve [95% CI] from 0.82 to 0.85 [0.85–0.86] (Supplementary Fig. 14a,b).
In summary, our results further define the genetic architecture and heritability of SLE risk (especially in Asians) and provide insights into disease pathogenesis. Through comprehensive analysis of multiple Asian populations, we identified 10 novel SLE-predisposing loci, and validated association in 36 reported loci (often refining intervals). We pinpointed and annotated independently associated variants at each locus. Further analysis in additional populations and experimental validation in cultured and patient cell types (as previously performed32–34) will confirm which SNPs are causal, and elucidate biochemical pathways through which genetic changes contribute to SLE. This study highlights the success of targeting high-risk populations for genetic analysis, followed by systematic bioinformatics analysis to set up future experimental validation.
Online Materials and Methods
Study overview
This study was conducted in three stages (Fig. 1). In the first stage, we genotyped three Asian cohorts: Koreans (KR), Han Chinese (HC) and Malaysian Chinese (MC). This step was followed by quality control (QC) and preliminary association analysis to identify 578 regions with P<5×10−3. Then we increased the KR sample size with out-of-study controls and performed imputation-based meta-analysis to discover 16 novel regions with P Discovery-meta<5×10−5. In the second stage, we followed up with these 16 novel regions, doing in silico replication on a Japanese (JAP) GWAS59 data set and two independent replications on separate Beijing Han Chinese (BHC) and Shanghai Han Chinese (SHC) to identify 10 novel loci with Pmeta<5×10−8. In the third stage, we used bioinformatic databases to annotate the identified variants, and carried out comprehensive analyses to uncover potential disease predisposing variants involved in SLE pathogenesis (Supplementary Table 1, Supplementary Note).
Imputation-based association, meta-analysis and conditional analysis
For the first stage of our study (Fig. 1), we performed single-SNP case-control association analysis based on QC’d ImmunoChip genotype data in each population. We calculated association P-values, standard error (SE), odds ratio (ORs) and 95% confidence intervals (95% CIs) using PLINK60. This identified 578 regions with P<5×10−3 in at least one Asian cohort for imputation (Supplementary Fig. 1, Supplementary Table 3). In order to perform the imputation more intensively and accurately, we wrote a script based on a recursive algorithm to define imputation regions. Imputation regions were defined if they contained a peak SNP with P<5×10−3. Region size was defined by the length of the linkage disequilibrium (LD) region (r2>0.2) with respect to the peak SNP. To avoid edge effects we extended a further 100 kilobases (kb) on each side for each region. The recursive algorithm to define imputed regions used the following steps:
Find the peak SNP with minimal P value ≤5×10−3 in a region a(x,y) (the region starting with the whole chromosome (x=start position, y=end position)). If such a peak SNP exists, continue; otherwise, stop.
Define imputation region d(u,v) = LD region (r2>0.2 with peak SNP) ±100kb.
If (x,u) exists, go to a(x,u) recursively (step a); if (v,y) exists, go to a(v,y) recursively (step a). Otherwise, stop.
Collect all regions d(u,v) for final imputation.
For the second stage of our study (Fig. 1), we integrated additional GWAS data from KR out-of-study controls to increase both SNP density and statistical power. Since KR ImmunoChip and KR GWAS data sets are genotyped in two different platforms and their overlapping SNP number is less than the original SNP number from either KR ImmunoChip or KR GWAS data set, we imputed each set separately on its original number of real genotyping SNPs using MACH-Admix61. HC and MC ImmunoChip data sets were imputed separately as well following the KR IC protocol. We took 504 Asians (104 Japanese in Tokyo-JPT + 200 Han Chinese in Beijing-CHB + 200 Southern Han Chinese-CHS) from 1000 Genomes Project data (2013-05-02 1000G Phase 3 Integrated Release Version 5 Haplotypes) as the reference panel for imputations. All SNP names and strands for the three ImmunoChip and one out-of-study control datasets were aligned with the Asian reference panel (n=504) before those four datasets were imputed separately. This imputation strategy has been used by many earlier studies62,63, and has also been recommended as a best practice by the eMERGE network64.
After imputation, we performed strict QC on post-imputed SNPs. In addition to the QC steps described above (PHWE>0.0001 in controls, MAF>0.5%), post-imputed SNPs were also required to have high imputation quality (Rsq>0.7 for MAF≥3% and Rsq>0.9 for MAF<3%) to be included for further analysis. In order to take into account imputation uncertainty, we used mach2dat65,66 for single-SNP post-imputation-based association tests and for conditional logistic regression analysis, with adjustment for population stratification. We used the first three principal components as covariates to correct for population stratification and potential batch effects. Additionally, as a complementary analysis, we used a newly developed genotype-conditional association test (GCAT)67 to confirm our PCA-corrected associations, the results of which were very consistent (data not shown). We used METAL68 to perform the meta-analysis based on post-imputation associations for three ImmunoChip cohorts (KR, HC and MC), as well as for the combined KR dataset (the merged dosage data set of KR ImmunoChip and KR GWAS controls), HC ImmunoChip and MC ImmunoChip. To include the highest-quality SNPs in the follow-up association analysis, we used imputed SNPs with high imputation quality (Rsq>0.7) in each of the separately imputed data sets (ImmunoChip and GWAS). We then merged the two imputation sets according to the stringent quality controls described above.
Finally we analyzed SLE-association in 152,918 post-imputation QC’d SNPs and identified 20,213 associated SNPs (PDiscovery-meta<0.005), from which we successfully replicated 36 SLE loci with PDiscovery-meta<0.005 (Supplementary Table 10) and identified 16 novel suggestive regions with PDiscovery-meta<5×10−5 for follow-up replication.
In order to test if any systematic bias was introduced by this imputation procedure, we also performed an association analysis of the lead SNPs between the controls (IC versus GWAS). We found no evidence of systematic bias introduced by the imputation and thus consider the imputation results sound (Supplementary Table 26).
We performed conditional analysis for 20 known SLE loci with genome-wide significance (GWS) and 10 novel regions with GWS after replication in the largest cohort (KR). Conditional analysis was iterative, starting with the top SNP with the lowest P-value as the first SNP to be conditioned upon; all subsequent significant SNPs after conditioning were added to the regression model as covariates until no SNP with P<5×10−5 remained. To ensure that SNPs were truly independent, SNPs in high LD (r2>0.3 with the SNP being conditioned upon) were filtered out before the next iteration, and only the associated SNPs with P<5×10−5 entered conditional analysis.
Functional annotation of novel loci
In order to localize candidate causal variants, we annotated each lead SNP along with its surrounding correlated SNPs (r2>0.7 in Asian samples from the 1000 Genomes Project), as implemented in Haploreg69 on data obtained from 1000 Genomes Phase 1, and ENSEMBL70. We surveyed allele-dependent gene expression regulation (i.e. expression quantitative trait loci, eQTLs) by querying the Blood eQTL13 database (which houses the experimental meta-analysis from gene expression experiments performed on non-transformed peripheral blood samples of 5311 individuals of European descent and later replicated on 2775 individuals) for cis- and trans-eQTLs (Supplementary Table 9). The functional significance of independent SNPs from novel regions is shown in Supplementary Table 7, and we report eQTL results in Supplementary Table 9.
We annotated epigenetic regulatory features for all independent lead SNPs (and their correlated variants r2>0.8) in our novel regions using the Haploreg69, GWAS3D71, and rSNPBase72 online tools. Haploreg69 provides functional annotations for binding motifs and epigenetic marks. GWAS3D71 aggregates epigenetic data from 16 cell types from multiple databases, including the ENCODE Project, and identifies multiple regulatory SNPs in high LD with the queried SNPs. Among the regulatory elements queried were enhancer marks (P300, H3K4me1, H3K27Ac), promoter regions, CTCF insulator marks and DNase hypersensitive sites (DHS). ChromHMM was used to predict histone states and chromatin interactions. In order to understand distal regulatory relationships among the novel loci, chromatin interactions between candidate loci were gathered from ChIA-PET and Hi-C data on 8 cell lines (K562, NB4, GM12878, CD4+ T-cells, H1-hESC, IMR90, RWPE1, MCF-7), available through ENCODE. We reported data for lead SNPs with at least three ChIA-PET or Hi-C hits (Supplementary Table 8, Supplementary Fig. 5). Additionally, rSNPBase72 provided putative functional SNPs with experimentally validated regulatory elements controlling transcriptional and post-transcriptional events.
Functional fine-mapping
In order to identify the set of variants most likely to house a functional variant, we used two Bayesian methods. The first one was based on a Bayesian regression to estimate each SNP’s Bayes Factor, and thereafter its posterior probability of association within the region10. Second, we used the Probabilistic Identification of Causal SNPs (PICS) algorithm11, which incorporates the underlying epigenetic information for those variants, to further narrow down the available SNPs within the Bayesian credible set.
Bayesian logistic regressions for each of the SNPs at the novel imputation regions was implemented in the Bayes Factor (BF)73 library in R. Henceforth, we estimated the posterior probability for each SNP, as well as the proportion of the total BF explained by each variant. We formed the 95%–99% credible sets as the cumulative proportion of the BF 10. In order to assess how much of the effects could be explained by the credible sets, we annotated each candidate SNP with dbSNP functions (intron, missense, UTR, synonymous, intergenic), as well as epigenetic annotations (promoter, enhancer, DNase hypersensitivity, bound proteins, motifs, drivers disrupted, rSNP, LD-proxy of rSNP (r2>0.8), proximal regulation, distal regulation, miRNA regulation, RNA-binding protein mediated regulation, eQTL).
We implemented the PICS method11 to identify the set of variants with probable functional effects. This method uses the epigenetic information at each locus and estimates the posterior probability of a SNP to be causal, given the strength of association, its linkage neighbourhood, as well as regulatory element annotations.
Gene-gene interaction
In order to identify gene-gene interaction, we performed logistic regression with an interaction term between all pairs of lead SNPs (Table 1) using PLINK. Both BOOST74 and joint effects75 methods were used to screen for SNP-SNP interactions. We used a significance threshold of 1×10−4.
Network interactions
In order to investigate how our novel loci interact with other genes, we used curated network interactions using the Disease Association Protein-Protein Link Evaluator database (DAPPLE V2.0)28. We used a seed of all our novel loci (both left and right flanking genes were also used for intergenic signals) and 20,000 within-degree-node permutations. We chose to simplify our networks given the number of potential interactions (Supplementary Fig. 12). The network represents all significant interactions between proteins that form a network.
Additionally, we confirmed network interactions using the aggregated database ConsensusPathDB29. ConsensusPathDB scores the confidence level of protein interactions on a scale between 0 and 1, and aggregates 11 pathway databases for gene set enrichment analysis (GSEA). We chose interactions with high confidence score (Intscore >0.9). Additionally, we plotted all possible high-confidence interactions for all novel loci (Supplementary Figs. 9,10).
To investigate how our updated set of novel SLE loci were related to each other and to previously established loci, we used a literature mining-based approach, implemented in IRIDESCENT30 (Supplementary Fig. 11). This approach identifies genes mentioned together in the same MEDLINE titles/articles (over 24 million currently) and weights their relevance based on relative frequencies of gene mention and gene-gene co-mention.
Gene set enrichment analysis (GSEA)
In order to identify if there were significant enrichments of our SLE (novel and replicated) loci as compared to reported SLE loci in human and mouse ontology, we performed gene set enrichment analysis using GREAT76 (Supplementary Table 19). In order to compare interacting pathways and ontological properties of novel versus published SLE genes, we used ConsensusPathDB29. Additionally, in order to identify and compare drug perturbation signatures between novel and reported loci, we used the gene enrichment analysis software Enrichr26 (Supplementary Table 18).
In order to test if there was bias in enrichment due to the choice of Immunochip as a genotyping platform, we conducted 100 over-representation analysis tests using sets of 58 genes taken at random from the ImmunoChip gene set in ConsensusPathDB29. We computed the number of times any pathway or ontology category was observed in the 100 random sets (Supplementary Table 29).
Cell type-specific enrichment analysis
In order to identify enrichment in cell type-specific expression of novel and replicated SLE loci (57 SNPs), we used a previously reported approach31,77 described as follows. We used normalized expression data from 79 human cell types from GeneAtlas78 (curated by the Genomic Institute of the Novartis Research Foundation), as well as from 249 mouse cell types sorted by FACS and assayed at least three times from the Immunological Genome Project (ImmGen)79. Additionally, we used cell-specific expression of the collection of 573 human cell samples from the FANTOM580 Project.
In this analysis, we extracted genes from the regions where SNPs correlated with the lead SNPs (Table 1; r2>0.5), spanning between recombination hotspots. We used normalized cell-specific expression profiles of the extracted genes to identify which cell types significantly express SLE candidate genes. Specificity P-values were estimated based on the permutation of ranked expression levels for each locus (1010 permutations) using SNPSEA77 (Fig. 4, Supplementary Fig. 13). P-values (blue bars) that passed the multiple testing threshold (black line) show significant enrichment in SLE loci. Threshold lines are dependent on the number of categories present in each database, i.e., for the 1751 GO categories possible, the significance threshold would be at 2×10−5.
Explained heritability
We assessed the variance in liability (Vg) explained for each of our GWS SNPs using the liability threshold method7. We estimated Vg for novel, reported and HLA loci separately. We used the weighted risk allele frequency and meta-analysis OR for each variant to calculate the liability threshold for each genotype (Supplementary Table 24). We present values estimated using a prevalence estimate (K) of 0.0030653 following So and Sham7. In order to check the consistency of this heritability estimate, we also used the allele frequencies from each cohort, as well as the allele frequencies for HapMap/1000 Genome populations CHB and JPT.
Sibling relative risk
We estimated the contribution of SLE susceptibility loci to the familiar relative risk (Supplementary Table 25), especially for the sibling relative risk (λs) under the multiplicative model81:
sibling relative risk
where λ0 is the overall sibling relative risk, assumed here to be ~30 (ref. 82), with the relative sibling risk from each locus (λ) given by
where p is the frequency of the risk allele (q=1−p) and r is the per-allele risk ratio83.
Weighted cumulative genomic risk score
In order to assess the effect of accumulation of risk variants between cases and controls, we estimated the weighted cumulative genomic risk score (wGRS) for all individuals with high imputation quality (Rsq >0.7). We weighted the number of risk variants by the natural logarithm of the meta-analysis OR84 for all 10 novel loci, 2 HLA loci and 35 replicated loci from a total of 2476 cases and 8426 controls. Significant differences in wGRS were estimated using a logistic regression model including gender and the top three principal components as covariates (Supplementary Fig. 14). Differences between mean wGRS in cases and controls were estimated through a linear model.
Area under the curve (AUC)
We estimated the predictive power of variants’ wGRS, as well as the marginal contribution of the novel variants, by comparing AUC for the baseline model (including reported loci) versus the expanded model (including reported + novel loci) (Supplementary Fig. 14). AUC corrected for gender was estimated in R using the pROC library85. Confidence intervals for AUC were estimated using the nonparametric DeLong method86.
Evidence for natural selection
To assess evidence for natural selection, we used HapMap2 and Human Genome Diversity Project (HGDP) population data through Haplotter and the HGDP Selection Browser. For each of the 10 novel genes we looked for evidence of positive natural selection in the 1 Mb region around each gene. Haplotter uses three statistics: iHS (Integrated Haplotype Score), FST (fixation index of population differentiation) and the empirical P-value for the distribution of Tajima’s D and Fay’s H87, while the HGDP Selection Brower uses XP-EHH88 (Cross Population Extended Haplotype Homozygosity) to identify positive natural selection in addition to iHS. Evidence of natural selection was considered positive if the empirical P-value<0.05 for the distribution of both Tajima’s D and Fay’s H, and −log(Q) >3 for Fst, D, iHS, or XP-EHH, where Q is the empirical P-values rank ordering the summary statistic value (a given region divided by total number of regions) (Supplementary Table 22).
Graphical display of the epigenetic landscape of the loci
For Supplementary Fig. 4, plots were assembled similarly to ref 33. Most data were downloaded from the UCSC Genome Browser and displayed using custom MATLAB code. ATAC-Seq tracks for CD4+ cells and GM12878 cells were downloaded from NCBI Gene Expression Omnibus accession number (GSE47753)89. DNase hypersensitivity, ENCODE sequence classification, histone marks and binding data for transcription factors (to the DNA) and RNA-binding proteins (to the RNA) were all downloaded from UCSC. ENCODE regulatory elements are color-coded according to their standard; other signals are shown in grey-scale, with dark representing higher signal. All tested SNPs are shown as bars of −log10 (P-value) height at the top. In the zoomed images, SNPs of interest are labeled.
Supplementary Material
1
2
3
Acknowledgments
We are grateful to the affected and unaffected individuals who participated in this study. We thank the research assistants, coordinators, and physicians who helped in the recruitment of subjects, including the individuals in the coordinating projects. A part of the Korean control data was provided from Korean Biobank Project supported by the Korea Center for Disease Control and Prevention at the Korea National Institute of Health. Genomic DNA from ~100 Korean SLE patients were obtained from the Korean National Biobank at Wonkwang University Hospital that is supported by the Ministry of Health & Welfare, Republic of Korea.
This work was supported by grants from the US National Institutes of Health (AR060366, MD007909, AI103399, AI024717, AI083194, HG006828), the Department of Defense (PR094002), the U.S. Department of Veterans Affairs, the Research Fund of Beijing Municipal Science and Technology for the Outstanding PhD Program (20121000110), the National Natural Science Foundation of China (No. 81200524) and the High Impact Research MoE Grant UM.C/625/1/HIR/MoE/E000044-20001, Malaysia. This study was also supported by a grant from the Korea Healthcare Technology R&D Project (HI12C1834, HI13C2124), Ministry for Health & Welfare, Republic of Korea.
Footnotes
URLs
GCTA v1.24: http://www.complextraitgenomics.com/software/gcta/
EIGENSTRAT: http://genepath.med.harvard.edu/~reich/EIGENSTRAT.htm
PLINK version 1.07: http://pngu.mgh.harvard.edu/~purcell/plink/
MACH-Admix: http://www.unc.edu/~yunmli/MaCH-Admix/
1000 Genomes Project: http://www.1000genomes.org/
Mach2dat: http://genome.sph.umich.edu/wiki/Mach2dat:_Association_with_MACH_output
METAL: http://www.sph.umich.edu/csg/abecasis/metal
HaploReg: http://www.broadinstitute.org/mammals/haploreg/haploreg.php
ENSEMBL: http://www.ensembl.org/
Blood eQTL: http://genenetwork.nl/bloodeqtlbrowser/
GWAS3D: http://jjwanglab.org/gwas3d
rSNPBase: http://rsnp.psych.ac.cn/
ENCODE Project: http://www.genome.gov/10005107
ChromHMM: http://compbio.mit.edu/ChromHMM/
DAPPLE v2.0: http://www.broadinstitute.org/mpg/dapple/dappleTMP.php
ConsensuspathDB: http://cpdb.molgen.mpg.de/
GREAT v2.0.2: http://bejerano.stanford.edu/great/public/html/
Enrichr: http://amp.pharm.mssm.edu/Enrichr/
GeneAtlas: http://biogps.gnf.org
ImmGen, http://www.immgen.org/
FANTOM5: http://fantom.gsc.riken.jp/5/
SNPSEA: http://www.broadinstitute.org/mpg/snpsea/
R version 3.0.0: http://www.r-project.org/
pROC: http://web.expasy.org/pROC/
Haplotter: http://haplotter.uchicago.edu/
HGDP Selection Browser: http://hgdp.uchicago.edu/cgibin/gbrowse/HGDP/
UCSC Genome Browser, http://genome.ucsc.edu;
AUTHORS CONTRIBUTIONS
S.K.N. and S-C.B. conceived and initiated the study. S.K.N. designed, coordinated and supervised the overall study. C.S., X-J.Z., P.M., K.B., A.A., and X.K-H prepared samples, performed genotyping, cleaned the data, combined various data sets and maintained the database. C.S., J.E.M., K.K. and Y. O. performed data imputation, association analysis, and various statistical analyses on the data. L.L.L., J.E.M., M.D. and J.D.W. performed the bioinformatic analysis. S-C.B., H.Z., K-H.C., X-J.Z., K.K., S-Y.B., H-S.L., T-H.K., Y.M.K., C-H.S., W.T.C., Y-B.P., J-Y.C., S.C.S., S-S.L., Y.J.K., B-G. H., ., Y. K., A. S., M. K., T.S., K.Y.,J. M., Y-Y. Q., K.M.K., J.B.H, and N, S. recruited and characterized SLE patients and controls, supplied the demographic and clinical data. C.S., J.E.M., X.K-H., K.K., S-C.B., L.L.L and S.K.N. drafted the manuscript. All authors approved the study, reviewed the manuscript, commented and helped revising the manuscript.
COMPETING FINANCIAL INTERESTS
The authors declare no competing financial interests.
References
Full text links
Read article at publisher's site: https://doi.org/10.1038/ng.3496
Read article for free, from open access legal sources, via Unpaywall:
https://europepmc.org/articles/pmc4767573?pdf=render
Citations & impact
Impact metrics
Article citations
Lymphocytes Change Their Phenotype and Function in Systemic Lupus Erythematosus and Lupus Nephritis.
Int J Mol Sci, 25(20):10905, 10 Oct 2024
Cited by: 0 articles | PMID: 39456692 | PMCID: PMC11508046
Review Free full text in Europe PMC
Epstein-Barr virus as a potentiator of autoimmune diseases.
Nat Rev Rheumatol, 20(11):729-740, 10 Oct 2024
Cited by: 0 articles | PMID: 39390260
Review
Early human migration determines the risk of being attacked by wolves: ethnic gene diversity on the development of systemic lupus erythematosus.
J Rheum Dis, 31(4):200-211, 23 Jul 2024
Cited by: 0 articles | PMID: 39355544 | PMCID: PMC11439634
Review Free full text in Europe PMC
KLF2 enhancer variant rs4808485 increases lupus risk by modulating inflammasome machinery and cellular homoeostasis.
Ann Rheum Dis, 83(7):879-888, 12 Jun 2024
Cited by: 0 articles | PMID: 38373841
Targeting T-bet expressing B cells for therapeutic interventions in autoimmunity.
Clin Exp Immunol, 217(2):159-166, 01 Jul 2024
Cited by: 0 articles | PMID: 38647337
Review
Go to all (152) article citations
Data
Data behind the article
This data has been text mined from the article, or deposited into data resources.
BioStudies: supplemental material and supporting data
GEO - Gene Expression Omnibus
- (1 citation) GEO - GSE47753
IGSR: The International Genome Sample Resource
- (1 citation) IGSR/1000 Genomes - GM12878
SNPs (Showing 23 of 23)
- (4 citations) dbSNP - rs73366469
- (2 citations) dbSNP - rs2305772
- (1 citation) dbSNP - rs12900339
- (1 citation) dbSNP - rs10807150
- (1 citation) dbSNP - rs12022418
- (1 citation) dbSNP - rs4711414
- (1 citation) dbSNP - rs2009453
- (1 citation) dbSNP - rs7726159
- (1 citation) dbSNP - rs7726414
- (1 citation) dbSNP - rs61616683
- (1 citation) dbSNP - rs113164910
- (1 citation) dbSNP - rs223881
- (1 citation) dbSNP - rs1885889
- (1 citation) dbSNP - rs7556469
- (1 citation) dbSNP - rs587608058
- (1 citation) dbSNP - rs2421184
- (1 citation) dbSNP - rs80346167
- (1 citation) dbSNP - rs2305249
- (1 citation) dbSNP - rs7800325
- (1 citation) dbSNP - rs909685
- (1 citation) dbSNP - rs11235604
- (1 citation) dbSNP - rs1610555
- (1 citation) dbSNP - rs10936599
Show less
Similar Articles
To arrive at the top five similar articles we use a word-weighted algorithm to compare words from the Title and Abstract of each citation.
Confirmation of five novel susceptibility loci for systemic lupus erythematosus (SLE) and integrated network analysis of 82 SLE susceptibility loci.
Hum Mol Genet, 26(6):1205-1216, 01 Mar 2017
Cited by: 35 articles | PMID: 28108556 | PMCID: PMC5731438
Meta-analysis of 208370 East Asians identifies 113 susceptibility loci for systemic lupus erythematosus.
Ann Rheum Dis, 80(5):632-640, 03 Dec 2020
Cited by: 75 articles | PMID: 33272962 | PMCID: PMC8053352
Identification of a New Susceptibility Locus for Systemic Lupus Erythematosus on Chromosome 12 in Individuals of European Ancestry.
Arthritis Rheumatol, 68(1):174-183, 01 Jan 2016
Cited by: 15 articles | PMID: 26316170 | PMCID: PMC4747422
Recent advances in systemic lupus erythematosus genetics in an Asian population.
Int J Rheum Dis, 18(2):192-199, 19 Dec 2014
Cited by: 5 articles | PMID: 25522798
Review
Funding
Funders who supported this work.
Howard Hughes Medical Institute
NCATS NIH HHS (3)
Grant ID: UL1 TR001417
Grant ID: UL1 TR001425
Grant ID: TR001425
NHGRI NIH HHS (4)
Grant ID: HG006828
Grant ID: HG008666
Grant ID: U01 HG006828
Grant ID: U01 HG008666
NIAID NIH HHS (9)
Grant ID: AI103399
Grant ID: R21 AI107176
Grant ID: AI083194
Grant ID: AI024717
Grant ID: R37 AI024717
Grant ID: AI107176
Grant ID: R21 AI103399
Grant ID: P01 AI083194
Grant ID: R01 AI024717
NIAMS NIH HHS (2)
Grant ID: AR060366
Grant ID: R01 AR060366
NIMHD NIH HHS (2)
Grant ID: MD007909
Grant ID: R01 MD007909