Abstract
Objective
Systemic sclerosis (SSc) is a fibrotic disease attributed to both genetic susceptibility and environmental factors. This study was undertaken to investigate the associations between SSc-associated genetic variants and the expression of extracellular matrix (ECM) genes in human fibroblasts stimulated with silica particles in time-course and dose-response experiments.Methods
A total of 200 fibroblast strains were examined for ECM gene expression after stimulation with silica particles. The fibroblasts were genetically profiled using Immunochip assays and then subjected to whole-genome genotype imputation. Associations of genotypes and gene expression were first analyzed in a Caucasian cohort and then validated in a meta-analysis combining the results from Caucasian, African American, and Hispanic subjects. A linear mixed model for longitudinal data analysis was used to identify genetic variants associated with the expression of ECM genes, and the associations were validated by using a haplotype-based longitudinal association test on regions that included the loci identified.Results
The single-nucleotide polymorphism rs58905141 in TNFAIP3 was consistently associated with time-course and/or dose-response expression of MMP3 and MMP1 in the fibroblasts stimulated with silica particles in both the analysis of Caucasian subjects only and the meta-analysis. Results of the haplotype-based analysis validated the association signals.Conclusion
Our findings indicate that a genetic variant of TNFAIP3 is strongly associated with the silica-induced profibrotic response of fibroblasts. In silico functional analysis based on the ENCODE database revealed that rs58905141 might affect the binding activities of the transcription factors for TNFAIP3. This is the first genome-wide study of interactions between genetic and environmental factors in a complex SSc fibroblast model.Free full text

Integrative studies of scleroderma-associated genetic and environmental factors with fibroblasts identify polymorphisms of TNFAIP3 in association with MMP expression
Abstract
Objectives
Systemic Sclerosis (SSc) is a fibrotic disease attributed to both genetic susceptibility and environmental factors. Our studies tried to demonstrate how human fibroblasts with SSc associated genetic variants respond to time-course and dose-response expression of the extracellular matrix (ECM) genes with silica particle stimulation.
Methods
A total of 200 fibroblast strains were examined for ECM gene expression after stimulation by silica particles. The fibroblasts were genetically profiled with Immunochip assays, and followed by whole-genome genotype imputation. Associations of genotypes and gene expressions were first analyzed in a Caucasian cohort, and then validated by a meta-analysis which combines the results from Caucasian, Blacks and Hispanics. We applied the linear mixed model for longitudinal data analysis to identify genetic variants associated with ECM genes’ expressions; we implemented haplotype-based longitudinal association test on identified loci region as a validation approach.
Results
SNP rs58905141 of TNFAIP3 was consistently associated with time-course and/or dose-response expressions of MMP3 gene and MMP1 gene of the fibroblasts stimulated with silica particles in both Caucasian only and meta-analysis. The haplotype-based analysis validated the association signals.
Conclusions
A genetic variant of TNFAIP3 is strongly associated with the silica-induced profibrotic response of the fibroblasts. In silico functional analysis based on ENCODE revealed that rs58905141 might affect binding activities of the transcription factors for TNFAIP3. This is the first genome-wide study of interaction between genetic and environmental factors in a complex SSc fibroblast model.
Introduction
Systemic Sclerosis (SSc) is a multi-system disorder of connective tissue characterized by extensive cutaneous and visceral fibrosis. Although the etiopathogenesis of SSc is still unclear, both genetic susceptibility and environmental triggers are widely believed as two major contributors. Genetic association studies have reported a number of SSc-associated genes including HLA genes (e.g. HLA-DQB1, -DPB1 and -DRB1), STAT4, IRF5, CD247, TBX21, BANK1, C8orf13, PTPN22, TNFSF4, FAS, TNFAIP3, CD226, IRAK1, MECP2, MIF, ITGAM, PLD4, TLR-2, CAV1, IL2RA, NLRP1, OPN, PXK, JAZF, KIAA0319, IL-6, IL-21, CXCL8, CSK, PSD3, NFKB1, XRCC1, XRCC4, CCR6, IRF8, GRB10, SOX5, NOTCH4, TNIP1, PSOR1C1, RHOB, DNASEIL3, SCHIP1/IL12A and ATG5 (1–27). On the other hand, studies of environmental hazards contributing to SSc were mainly suggested by occupations with high incidence of SSc. Silica particles have been implicated as an environmental trigger of SSc in several epidemiological studies. Particularly, stonemasons and gold miners who were often exposed to silica particles were more likely to develop SSc (28, 29).
Fibrosis in connective tissues is the most prominent feature of SSc. A major component of connective tissues is fibroblasts. Fibroblasts synthesize, secrete, and maintain all major extracellular matrix (ECM) components that are significantly increased in fibrotic tissues, which directly contribute to fibrosis. Perturbed expression of some major ECM genes have been observed in fibroblasts of SSc patients (30, 31). In particular, COL1A2 and COL3A1 are two major genes encoding structure proteins collagen type I and III, respectively. These collagens are frequently over-expressed in fibroblasts, and are accumulated in fibrotic tissue of SSc patients. Connective tissue growth factor (CTGF) is a profibrotic cytokine that is also over-expressed in SSc patients, and it induces ECM production. Matrix metalloproteinases (MMP) are antifibrotic molecules that induce the degradation of the collagens and other ECM components. Unbalanced expressions of MMP and tissue inhibitor of metalloproteinases (TIMP) are involved in fibrotic process in SSc (30, 31). Our hypothesis is that inherited genetic variations modulate cellular responses to the environment, and in some situations in a pathologic manner. The purpose of this paper is to use a systems-based approach in a fibroblast model that integrates genome-wide genetic variants and the expression of some fibrosis-associated ECM genes to investigate their interactive responses to environmental perturbation.
Methods
Fibroblasts
We examined 200 fibroblast strains obtained from skin biopsies (upper arm of non-lesional skin) of 96 SSc cases and 104 controls. The primary cultures were maintained in Dulbecco’s Modified Essential Media (DMEM) with 10% FBS and supplemented with antibiotic and antimycotic as described previously (32). The 5th passage of fibroblast strains were plated at a density of 2.5 × 105 cells in a 35 mm dish and grown until 80% confluence. The culture medium was replaced with DMEM without FBS before stimulation assays.
All patients fulfilled American College of Rheumatology criteria for SSc (33). Among them, there were 37 limited and 53 diffused form of SSc (six patients were not reported in skin types). Autoantibodies positivity showed twenty patients with anti-topoisomerase I, twelve with anti-centromere, seventy with anti-RNA polymerase III. For disease duration, forty-nine patients were less than 5 years, eighteen were between 5 and 10 years, seventeen were 11 to 20 years, twelve were unknown. All normal controls were individuals with no history of autoimmune diseases. The study was approved by the Committee for the Protection of Human Subjects at University of Texas Health Science Center at Houston.
Silica stimulation on fibroblasts
Culture media then were replaced with FCS-free DMEM containing different doses (1, 5, 10, 25 and 50 µg) of silica particles obtained from Sigma-Aldrich. The cultures also were grouped into different time-courses including 24-, 48-, 72-, 96-, 120-hours stimulation with 10 µg silica. After stimulation, the fibroblasts were harvested for extraction of RNA. The RNAs were examined with RT-PCR for gene expression of COL1A2, COL3A1, CTGF, MMP1, MMP3 and TIMP3. We used the “AQchange” (absolute quantification change) as the phenotype variable in the genetic association analysis, defined as
Genotyping of fibroblasts from each subject
Samples were genotyped using the Immunochip, an Illumina Infinium platform designed to densely genotype 186 immune-mediated disease loci identified in prior GWAS of autoimmune diseases (26), according to the manufacturer's recommendations. Bead intensity data was processed and normalized for each sample in Genome Studio; data of successfully genotyped samples was extracted and genotypes were called within collections using optiCall (34). We obtained 196,517 SNPs before quality control (QC) as described below. NCBI build 36 (hg18) mapping was used in factory assembly file (Illumina manifest file Immuno_BeadChip_11419691_B.bpm); we then re-mapped all the SNPs to NCBI build 37 (hg19) locations.
Measurement of the ECM gene expression
Quantitative real time RT-PCR was performed using an ABI 7900 sequence detector (Applied Biosystems) for gene expression of human COL1A2, COL3A1, CTGF, MMP1, MMP3 and TIMP3 using standard protocol described previously (32, 35). The specific primers and probes for each gene were purchased through Assays-on-Demand from Applied Biosystems. Synthetic DNA oligos of the target gene were used as standards (sDNA). The sDNA standards were quantified by absorbance at 260 nm. Standard curves were run from 2 to 200 pg sDNA on each plate. The number of molecules per sDNA mass was then calculated from the length of the sDNA for each assay. Each set of standards yielded a linear curve (r2 > 0.992), and efficiencies calculated from the slope of each standard curve were >95%. Each gene expression was were analyzed using SDS software (life technologies, Foster City, CA).
Linear mixed model to detect time-course and dose-response loci
We used the linear mixed model (LMM) to test genome-wide associations between SNPs and time-course/elevated-dosage responded mRNA levels, as measured by the quantitative real time RT-PCR, for each of the six ECM genes. The LMM takes into account the correlations between repeated measurements within the same subject by introducing population-level fixed effects and subject-specific random effects (random intercept and slope), and enjoys the parsimonious modeling of correlated outcomes and the ease of accommodating missing values (36). To correct for multiple hypotheses testing, we used the Bonferroni procedure with a genome-wide significance cut-off of 5.72×10−8 adjusting for a total of 874,949 genotyped and 1000 Genomes-imputed SNPs with non-missing p-values from the LMM. After we performed the race-specific association analyses by the LMM, we performed fixed-effect meta-analysis as well as random-effect meta-analysis if determined necessary, combining results from the Caucasians, Hispanics and African Africans. Details on the statistical methods are provided in the Supplemental Methods, including QC procedures, 1000 Genomes-based imputation, LMM model specification, meta-analysis, haplotype-based association analysis, functional annotation and power calculation.
Results
Initial analysis
After stringent quality control measures, 183 subjects including 85 cases and 98 controls were included in the final association analyses; see Supplemental Methods for details. Demographic information of the study subjects is shown Table 1. We first analyzed all Caucasians among the subjects (50 SSc cases and 65 controls) as a discovery cohort (Table S1). Using LMM model assuming an additive genetic model, we found several genetic loci with SNPs significantly associated with expression of the ECM genes in this cohort (Table 2). Among them, three previously reported SSc loci including multiple SNPs of TNFAIP3 (6q23) (10, 37), IL2RA (10p15-p14) (15) and ITGAM (16p11.2) (12, 38) showed strong associations with the expression of MMP1 and/or MMP3 of the fibroblasts in responses to dosage-dependent and/or time-course stimulations of silica particles (Table 2). SNPs of CASC9 (8q21.11), intergenic region between LINC00284 and SMIM2-AS1 (13q14.11), FGFR1OP (6q27) and THADA (2p21) also showed associations with the expression of specific ECM genes of the fibroblasts (Table 2), but these genes have not been reported as SSc-associated.
Table 1
Distribution of demographics among SSc cases and controls
Variable | SSc (n = 85) n (%) | Controls (n = 98) n (%) | P-value (χ2) |
---|---|---|---|
Age group | |||
≤30 | 7 (8.24) | 25 (25.51) | |
31–40 | 14 (16.5) | 23 (23.47) | |
41–50 | 24 (28.24) | 22 (22.45) | |
>50 | 40 (47.06) | 28 (28.57) | 0.0034 |
Racea | |||
Caucasians | 50 (58.82) | 65 (66.33) | |
Hispanics | 22 (25.88) | 10 (10.20) | |
African Americans | 13 (15.29) | 23 (23.47) | 0.015 |
Sex | |||
Female | 67 (78.82) | 60 (61.22) | |
Male | 18 (21.18) | 38 (38.78) | 0.016 |
Table 2
Associations between expression of the ECM genes and genetic backgrounds in Caucasians and mixed populations by meta-analysis.
Peak SNP | Chr | Positiona | Type | Gene(s)b | RA | Pf | P-FE | P-RE | HetISq (%) | HetP | Directionc | Estimates(W|B|H)e | StdErr(W|AA|H)e | MAF(W|AA|H)e | responseType |
---|---|---|---|---|---|---|---|---|---|---|---|---|---|---|---|
rs58417815* | 8 | 76159689 | ncRNA_intronic | CASC9 | C | 5.10E-08 | -g | - | - | - | +?? | 1.77|NA|NA | 0.29|NA|NA | 0.074|NA|NA | COL3A1_dose |
rs7823944 | 8 | 76182788 | ncRNA_intronic | CASC9 | T | 3.47E-08 | 2.00E-07 | 7.10E-02 | 51.20 | 0.13 | +−+ | 1.54|−0.03|1.00 | 0.25|0.73|1.05 | 0.052|0.033|0.021 | COL3A1_dose |
rs79411652 | 6 | 138138945 | intergenic | OLIG3|TNFAIP3d | C | 3.87E-11 | - | 5.69E-01 | 88.80 | 0.00 | +−? | 2.29|−0.80|NA | 0.32|0.97|NA | 0.039|0.033|NA | MMP1_dose |
rs58905141 | 6 | 138132123 | intergenic | OLIG3|TNFAIP3** | G | 3.97E-11 | 3.46E-11 | 0.402918 | 43.1 | 0.17 | ++− | 2.29|62.41|−2.18 | 0.32|32.09|65.44 | 0.039|0.014|0.016 | MMP1_dose |
rs41290329 | 10 | 6054083 | UTR3 | IL2RA | C | 1.51E-08 | - | - | - | - | +?? | 3.43|NA|NA | 0.57|NA|NA | 0.013|NA|NA | MMP1_dose |
rs79365263 | 13 | 44618508 | intergenic | LINC00284|SMIM2-AS1 | C | 3.13E-09 | - | - | - | - | +?? | 2.22|NA|NA | 0.35|NA|NA | 0.024|NA|NA | MMP1_dose |
rs79411652 | 6 | 138138945 | intergenic | OLIG3|TNFAIP3 | C | 5.55E-18 | - | 5.12E-01 | 93.30 | 0.00 | +−? | 3.69|−0.91|NA | 0.41|0.99|NA | 0.039|0.033|NA | MMP3_dose |
rs58905141 | 6 | 138132123 | intergenic | OLIG3|TNFAIP3** | G | 6.43E-18 | 6.00E-18 | 1.32E-17 | 0 | 0.81 | +++ | 3.69|28.75|22.87 | 0.41|34.61|128.32 | 0.039|0.014|0.016 | MMP3_dose |
rs41290329 | 10 | 6054083 | UTR3 | IL2RA | C | 1.02E-11 | - | - | - | - | +?? | 5.29|NA|NA | 0.74|NA|NA | 0.013|NA|NA | MMP3_dose |
rs79365263 | 13 | 44618508 | intergenic | LINC00284|SMIM2-AS1 | C | 5.33E-08 | - | - | - | - | +?? | 2.80|NA|NA | 0.49|NA|NA | 0.024|NA|NA | MMP3_dose |
rs12926702 | 16 | 31240971 | intergenic | TRIM72|ITGAM | C | 1.33E-10 | - | 3.42E-01 | 87.90 | 0.00 | +−? | 5.09|−0.35|NA | 0.76|1.52|NA | 0.014|0.016|NA | MMP3_dose |
rs78409037 | 2 | 43740411 | intronic | THADA | C | 4.51E-05 | - | 9.14E-02 | 94.10 | 0.00 | +?+ | 0.97|NA|3.63 | 0.23|NA|0.55 | 0.069|NA|0.041 | MMP3_timecourse |
rs77533229 | 2 | 43479638 | intronic | THADA** | G | 2.68E-08 | - | - | - | - | +?? | 3.35|NA|NA | 0.60|NA|NA | 0.010|NA|NA | MMP3_timecourse |
rs79411652 | 6 | 138138945 | intergenic | OLIG3|TNFAIP3 | C | 3.52E-10 | - | 7.42E-01 | 93.80 | 0.00 | +−? | 1.86|−1.02|NA | 0.29|0.64|NA | 0.039|0.033|NA | MMP3_timecourse |
rs58905141 | 6 | 138132123 | intergenic | OLIG3|TNFAIP3** | G | 3.73E-10 | 3.23E-10 | 0.446757 | 29.1 | 0.24 | ++− | 1.85|38.02|−11.59 | 0.29|21.66|53.94 | 0.039|0.014|0.016 | MMP3_timecourse |
rs75701002 | 6 | 167443918 | intronic | FGFR1OP | C | 2.18E-08 | 5.68E-08 | 5.17E-02 | 47.50 | 0.17 | +?+ | 2.39|NA|0.32 | 0.42|NA|1.32 | 0.026|NA|0.021 | MMP3_timecourse |
rs41290329 | 10 | 6054083 | UTR3 | IL2RA | C | 1.03E-10 | - | - | - | - | +?? | 3.25|NA|NA | 0.47|NA|NA | 0.013|NA|NA | MMP3_timecourse |
RA: risk allele; P: P-value in Caucasians; P-FE: P-value by fixed-effect meta-analysis; P-RE: P-value in random-effect meta-analysis; HetISq: heterogeneity measure I2; HetP: Cochran's heterogeneity test p-valie; Estimates/StdErr/MAF: per risk allele effect estimates/standard error/minor allele frequency in different ethnic groups; W|AA|H: Caucasians|African Americans|Hispanics; responseType: the ECM gene expression in response to silica stimulation in elevated-dosage or time-course.
Rs79411652 in the upstream of TNFAIP3 was associated with MMP1 dose-response, MMP3 dose-response and time-course response. The minor allele frequency (MAF) of rs79411652 was 3.9% and 3.3%, respectively, in the Caucasian and African American samples. However, the effect size of this SNP was quite large as shown in Figure 4A, B and C, with 3.6 to 12.7 fold change of gene expression between heterozygous alleles and homozygous major allele. Thus, we had high enough power (over 80%) to detect this association. In addition, regional plots, as shown in Figure 3A, B and C, indicate that there was a cluster of SNPs in high LD with rs79411652 showing association signals, which strengthen the findings about the potential pleiotropic effects of TNFAIP3 on MMP1 and MMP3.
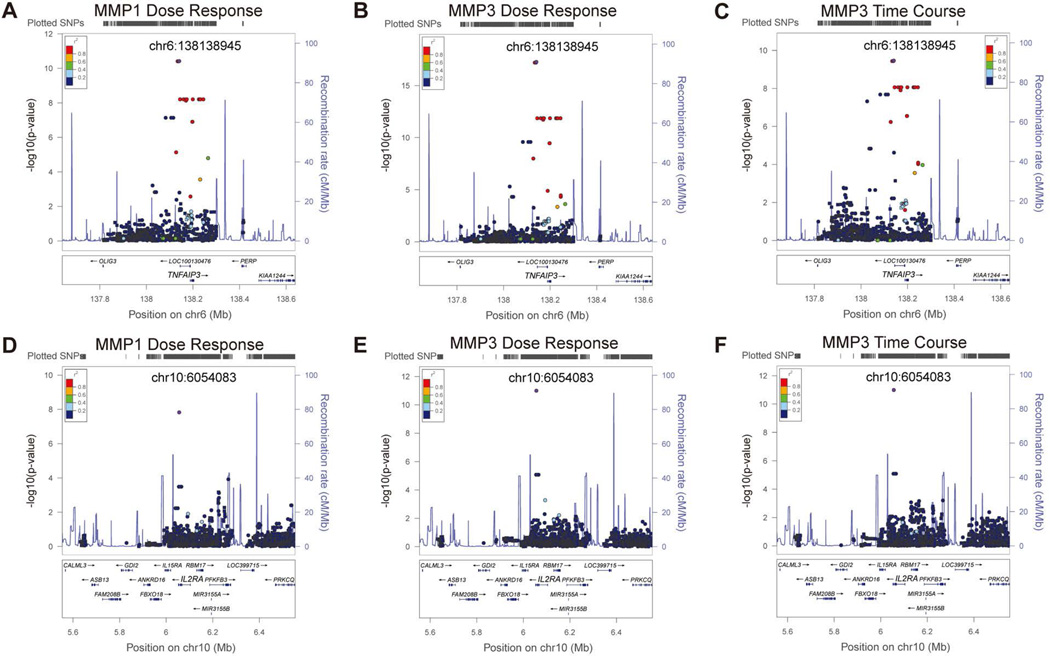
500kb upstream and downstream of the peak SNPs are plotted. Panels A to C: genetic locus at 6q23.3 containing SNPs of TNFAIP3 in association with expression of (A) MMP1 in dose-response assays, (B) MMP3 in dose-response assays, and (C) MMP3 in time-course assay. Panels D to F: genetic locus at 10p15.1 containing SNPs of IL2RA in association with expression of (D) MMP1 in dose-response assays, (E) MMP3 in dose-response assays, and (F) MMP3 in time-course assays. Round dots represent genotyped SNPs while square dots represent imputed SNPs. Purple dots correspond to the peak SNPs. Linkage disequilibrium (r2) with the peak SNP (rs79411652 of TNFAIP3 and rs41290329 of IL2RA, respectively) is shown by different colors.
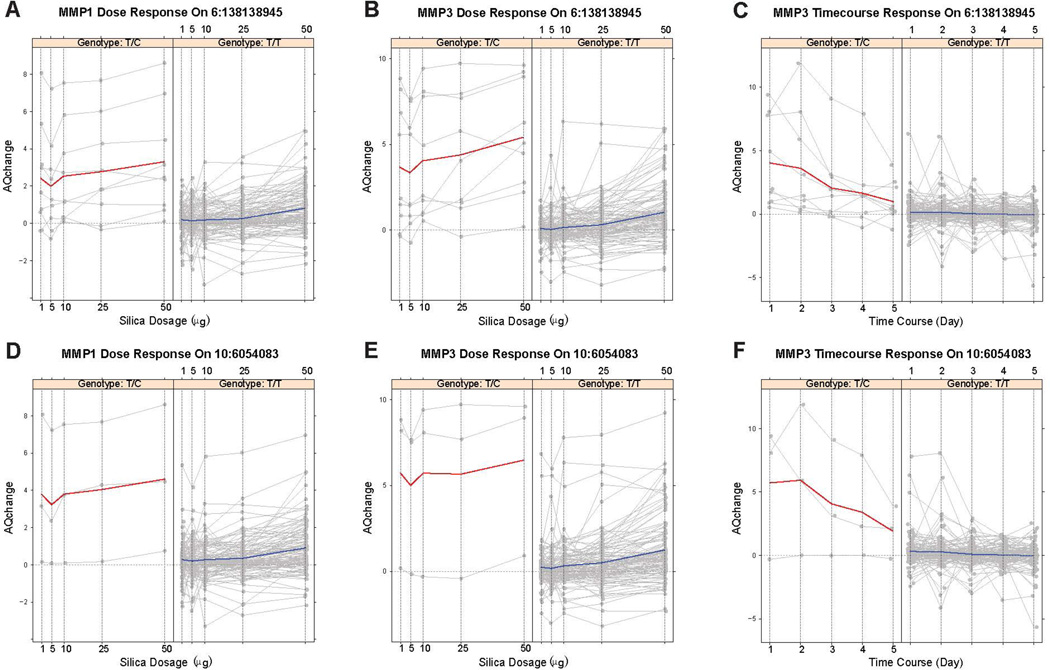
Panels A-F correspond to panels A-F in Figure 3. Red solid line: sample average trajectory for the heterozygous alleles; blue solid line: sample average trajectory for the homozygous major alleles; gray solid lines: individual trajectories; dashed horizontal grey line at 0 of the y-axis: the reference line representing constant gene expression across time/doses. Y-axis corresponds to the absolute quantity change (AQchange); x-axis corresponds to silica dosage (in µg) or time course (in days).
Figures 3, ,44 and S3 also show regional plots and genotype-specific expression trajectories for other previously identified SSc loci. SNP rs41290329 located in the 3’ untranslated region (UTR) of IL2RA was significantly associated with MMP1 dose-response, MMP3 dose-response and time-course expression profiles (Figure 3D, E and F) and with 9.5 to 39 fold change of gene expression of MMP1/MMP3 between heterozygous alleles and homozygous major allele (Figure 4D, E and F). Of note, rs41290329 is a low frequency SNP with an MAF of 1.3% in Caucasians and monomorphic in African Americans and Hispanics in our samples as well as in the 1000 Genomes Project. In addition, SNP rs12926702 was significantly associated with MMP3 dose-response expression profile (Figure S3 H) with 34 fold change of gene expression of MMP3 between heterozygous alleles and homozygous major alleles. Rs12926702 is located at the intergenic region of TRIM72 and ITGAM. It is also a low frequency SNP with a MAF of 1.4% and 1.6% in Caucasians and African Americans, respectively, and monomorphic in our Hispanics samples. Given the low MAF of the peak SNPs and the current moderate sample size, we caution that future studies with larger sample size are warranted to confirm the signals identified here and obtain more reliable effect size estimates.
To evaluate whether the association signals of TNFAIP3 and IL2RA could be better explained by multiple associated SNPs in LD or single peak SNPs, we performed haplotype-based analysis of the chromosomal regions defined by 200 SNPs upstream and downstream of the peak SNPs, rs79411652 of TNFAIP3 and rs41290329 of IL2RA. We defined haplotypes based on 3, 5 or 7 consecutive SNPs in sliding windows. The peak signals of haplotype-based analyses agreed well with those of single SNP-based association analyses, although the former yielded no as strong signals as the latter (Figure S2).
In addition to the signals within or near SSc-associated genes, we also identified several genetic loci that have not been reported to be associated with SSc (Table 2), including rs7823944 of CASC9 associated with COL3A1 dose-response expression (Figure S3 A), rs79365263 in the intergenic region of LINC00284 and SMIM2-AS1 associated with MMP1 and MMP3 dose-response expression (Figure S3 D and G), rs78409037 of THADA and rs75701002 of FGFR1OP associated with MMP3 time-course expression (Figure S3 I and K). In particular, rs7823944 of CASC9 was supported by a cluster of imputed SNPs in high LD (r2 around 0.80) (Table 2 and Figure S3 A), while rs79365263 of LINC00284|SMIM2-AS1 was clustered with a number of genotyped SNPs in high LD (Figure S3 D and G).
HLA class II genes are well-documented in association with SSc. The nominal association signals (5 × 10−8 < P < 5 × 10−2) of HLA class II genes were observed in the Caucasian cohort. Other SSc-loci did not show significant association with gene expression of the fibroblasts.
As elaborated in Supplemental Methods, the standard normal distribution-based p-values for the SNP effect in the LMM can be too liberal, i.e., not controlling the Type I error at the nominal level. We therefore calculated the more accurate t-distribution-based p-values using the computationally demanding Satterthwaite approximation for the peak SNPs in Table 2. As shown in Table S2, although all of the p-values became larger as expected, the peak SNPs in the SSc-associated loci of TNFAIP3, IL2RA and ITGAM, remained significant.
Meta-analysis of Caucasian, African American and Hispanic cohorts
We conducted meta-analysis of the Caucasian (50 SSc patients and 65 controls), African American (13 SSc patients and 23 controls) and Hispanics (22 SSc patients and 10 controls) cohorts via the fixed-effect inverse-variance weighting method as well as random-effect meta-analysis if determined necessary; see the Supplemental Methods for details. Of note, in the presence of potential heterogeneous genetic effects across the three populations, fixed-effect meta-analysis may not be appropriate. To investigate this, we calculated the heterogeneity measure I2 and Cochran’s Q test p-value (Table 2), which indicated that most of genome-wide significant SNPs in the Caucasian cohort, might have heterogeneous genetic effects across different populations (I2 > 56%) (39). We, therefore, also performed random-effect meta-analysis for these SNPs. Noticeably, SNP rs58905141 of TNFAIP3 remained genome-wide significantly associated with MMP3 dose-response expression by both fixed-effect and random-effect meta-analysis, even though I2 and Cochran’s test results suggested that the random-effect model was not necessary for this SNP. Other SNPs did not show significance with random-effect meta-analysis. The Manhattan plots for fixed-effect and random-effect meta-analysis are, respectively, shown in Figure 1 and Figure S1. Meta-analysis results for all the genome-wide significant SNPs in the discovery analysis of the Caucasian samples are shown in Table S3. The non-significant random-effect meta-analysis results for most of the peak SNPs in Caucasians may be attributed to the low MAF of the SNPs, smaller sample sizes in the African American and Hispanic cohorts and disparate LD patterns across populations, leading to diminished statistical power.
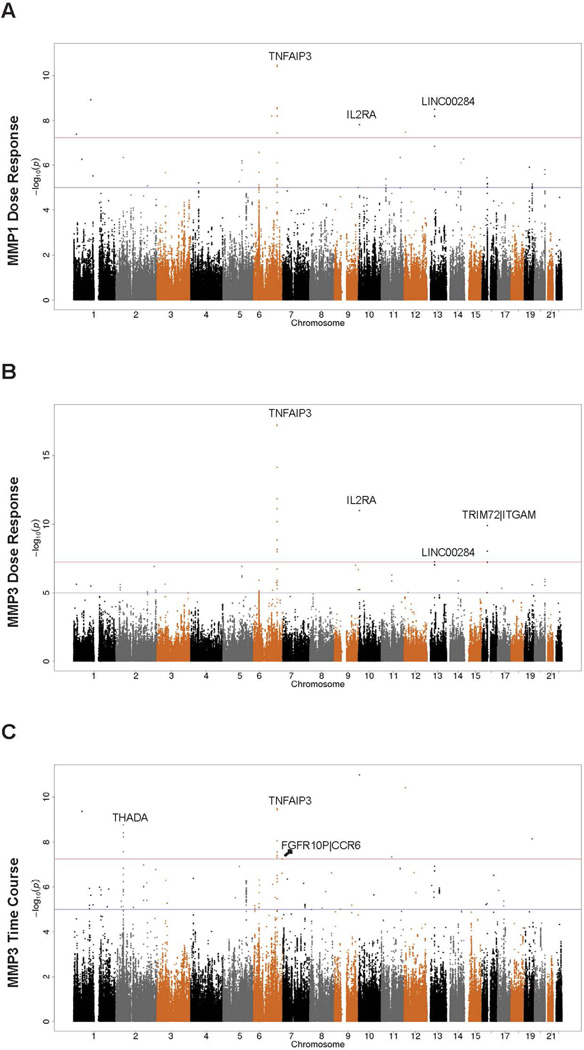
A. MMP1 gene expression in silica dose response showed significant signals at 6q23.3 (TNFAIP3), 10p15.1 (IL2RA) and 13q14.11 (LINC00284). B. MMP3 gene expression in silica dose response showed significant signals at 6q23.3 (TNFAIP3), 10p15.1 (IL2RA), 13q14.11 (LINC00284) and 16p11.2 (TRIM72|ITGAM). C. MMP3 gene expression in silica time course assays showed significant signals at 2p21(THADA), 6q23.3 (TNFAIP3) and 6q27(FGFR10P|CCR6). Red line corresponds to the p-value cutoff for genome-wide significance (5 × 10−8); blue line corresponds to the suggestive significance cutoff (1 × 10−5). P-values from Caucasian-only analysis are displayed if fixed-effect meta-analysis was not performed due to missing association results in African Americans and Hispanics.
Subgroup analysis
To evaluate whether there might be heterogeneity across patient subgroups regarding the findings here we performed subgroup analysis. The main findings regarding TNFAIP3 remained the same and did not appear to be specific to certain SSc disease subgroups. We first evaluated whether the time-course and dose-response expression of the ECM genes was different between the limited and diffused subtypes, and found that all the ECM gene expression was homogenous across the two subgroups (p-values for the disease subtype covariate were all around 0.5). We therefore pooled the two subtypes together to ensure the feasibility of genetic association analysis, but included the disease subtype as a covariate in all LMM analyses to control for its potential subtle confounding effect. The two significant SNPs rs79411652 and rs58905141 in TNFAIP3 (Table 2) gave identical p-values in the subgroup analysis as their minor allele carriers were exactly the same in these patients. Both SNPs remained genome-wide or nominally significant: the p-value was 4.52×10−5, 2.10×10−5, and 0.002 for MMP1 dose-response, MMP3 dose-response and MMP3 time-course expression, respectively.
Discussion
SSc is a fibrotic disease with complex genetic traits, to which environmental factors may trigger a pathological process toward systemic fibrosis. Functional association studies of genetic polymorphisms in connection with environmental triggers are challenge. It is unknown whether the SSc susceptibility loci contribute to specific fibrotic process in the presence of an environmental hazard. In comparison with conventional gene by environment (GxE) analysis based on observational studies (40, 41), the studies herein are novel in that we examined profibrotic effects of silica particles in a complex cell model of human fibroblasts that were genetically profiled with Immuno-chip SNPs.
A previously reported SSc locus at 6q23.3 with SNPs of TNFAIP3 (10, 37) appeared to be persistently in association with expression of MMP1 and/or MMP3 genes of the fibroblasts in responses to dosage-dependent and time-course stimulations of silica particles in both Caucasian only analysis and meta-analysis of diverse populations (Table 2). TNFAIP3 stands for tumor necrosis factor-α-induced-protein 3. It encodes A20, an ubiquitin-modifying enzyme that inhibits NF-kB activity and is a key regulator of TNF-mediated immune responses and inflammation (42, 43). Inflammation has been reported as a major consequence induced by silica particles in in vivo and in vitro studies (35, 44). Our previous studies indicated that silica might activate fibroblasts to overexpress the ECM genes through proinflammatory process (32, 35). Therefore, the results suggest that an interaction between genetic factors of TNFAIP3 and environmental factor of silica stimulation may control fibroblasts to express MMP1/MMP3 genes, which may be associated with development of SSc through an inflammatory and immune-control mechanism. Of note, intronic SNP rs5029939 in TNFAIP3 was reported to be associated with SSc in a previous case-control study (10). LD analysis by the SNAP tool (45) indicated that this SNP was in perfect LD (r2 = 1 and D’=1) with our top hit SNP rs79411652 in the discovery analysis of Caucasian samples, and in high LD with our meta-analysis top hit SNP rs58905141 (r2 = 0.80 and D’=1) associated with MMP3 dose-response and time-course expression as well as MMP1 dose-response expression. Regional plots (Figure 3A, C and E) also suggest that these SNPs were likely to tag the same functional locus/loci. We annotated the top SNPs of TNFAIP3 with RegulomeDB (46) according to the ENCODE database (47). While rs79411652 was annotated to category 7, i.e., no known biological function, rs58905141 was annotated to the second highest functional category “2b”, which is likely to affect binding of transcription factors FOXA1 and SMARCA4 by ChIP-seq experiments, binding of STAT1 by motif analysis, and is in DNase footprint by DNase-seq and in DNase peak by ChIP-seq experiments. This suggests that rs58905141 may play a functional role in regulating TNFAIP3 expression and directly or indirectly regulate MMP1/MMP3 expression in fibroblasts. Further replication and functional studies of the signals in TNFAIP3 are warranted to confirm this hypothesis.
Two other reported SSc-associated genetic loci with SNPs of IL2RA (10p15-p14) and ITGAM (16p11.2) genes were significantly associated with the expression of MMP1 and/or MMP3 in silica response assays in Caucasians (Table 2). IL2RA stands for interleukin 2 receptor α. IL2RA is involved in various pathways that help control the differentiation of effector cells, T-cell proliferation, and immune tolerance (48). A meta-analysis study with a large Caucasian European cohort revealed an association between rs2104286 of IL2RA and ACA positive SSc patients (15). LD analysis using the SNAP software showed that MMP1/MMP3 expression associated rs41290329 was in complete LD with rs2104286 (D’ = 1), but in low LD as measured by r2 (=0.06), due to the disparate MAFs of the two SNPs (0.013 and 0.225 in the 1000 Genomes CEU population, respectively). Functional analysis by RegulomeDB indicated that biological function for rs41290329 was unknown. As for ITGAM that encodes the α subunit of the αMβ2-integrin, it has been recently identified as an autoimmune disease risk gene (49). It is expressed on the surface of leukocytes, and it regulates adhesion of neutrophils and monocytes, cell activation, which is important for innate immunity (50). Rs1143679 of ITGAM was associated with SSc in European cohorts (12) and a large-scale meta-analysis (38). LD analysis suggested that MMP3 dose-response expression associated rs12926702 was in complete LD with rs1143679 (D’ = 1), but in low LD as measured by r2 (=0.003), due to the low MAFs of both SNPs (0.033 and 0.083 in the 1000 Genomes CEU population, respectively). Considering the low MAFs of rs41290329 and rs12926702, in addition to our moderate sample size, especially for non-Caucasian samples, we caution that the findings on IL2RA and ITGAM need to be confirmed in future studies with larger sample size.
In addition, several other genetic loci and genes unrelated to SSc susceptibility were also strongly associated with expression of the ECM genes of silica-stimulated fibroblasts in Caucasian-only analysis and/or meta-analysis (Table 2). Whether these associations represent a general impact of specific genetic loci on immune-mediated diseases is worth further investigation.
It is worth noting that many of those known SSc-loci did not show significant impact on gene expression of the fibroblasts in this study. For instance, the HLA class II genes that confer the strongest susceptibility to SSc (1) showed only nominal association signals (see Figure 2), which suggest that potential genetic impact of these genes to profibrotic responses of silica-stimulated fibroblasts may be less significant in this study model. It is likely that genetic impact of specific SSc-loci may be determined by interactions of specific types of cells and environmental triggers, as well as specific responding genes.
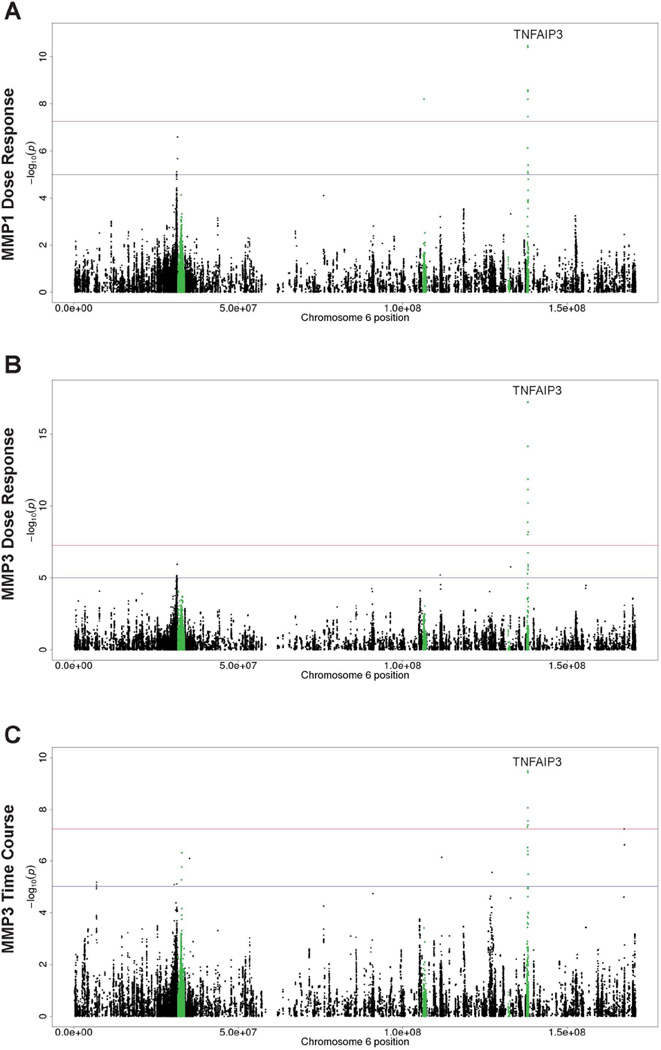
Panels A, B and C correspond to Figure 1 A, B and C with a zoom in for chromosome 6 only. Green dots show loci on or near known SSc risk genes. Red line corresponds to the p-value cutoff for genome-wide significance (5 × 10−8); blue line corresponds to the suggestive significance cutoff (1 × 10−5). P-values from Caucasian-only analysis are displayed if fixed-effect meta-analysis was not performed due to missing association results in African Americans and Hispanics.
The current study employed the cost-effective Immunochip targeted at 186 gene regions which were identified in previous association studies of autoimmune diseases. Although we successfully performed 1000 Genomes-based imputation to boost the 130,000 directly genotyped SNPs to around 800,000 total SNPs, the coverage was likely to be very low beyond the 186 gene regions. As a result, we cannot exclude the possibility that other genetic variants that were not investigated in the current study might be associated with the ECM gene expression in the fibroblasts. In addition, heterogeneity of patients’ clinical status is another concern. This warrants further investigation in the future.
In summary, this is the first attempt to study interactions between genome-wide genetic variants and environmental factors of SSc in a complex fibroblast model. The results indicated that a previously identified SSc locus of TNFAIP3 was strongly and persistently associated with silica-induced profibrotic responses of the fibroblasts in both Caucasians and meta-analysis of mixed populations. Similar associations were observed in two other reported SSc loci of IL2RA and ITGAM in Caucasians. In addition, some non-SSc loci may also impact a general response of fibroblasts to silica particles. SSc subtype analysis of the associations was limited by small sample size. Therefore, this notion may be further verified in other cohorts with larger sample size and in functional studies beyond the ECM genes.
Acknowledgments
This work was supported by the grants from the Department of the Army, Medical Research Acquisition Activity [PR064803 to XD.Z]; Scleroderma Foundation [2012 to XD.Z]; and the National Institutes of Health [NIAID UO1, 1U01AI09090 to XD.Z]. P.W. was partially supported by NIH grants R01CA169122 and R01HL116720. The authors acknowledge the Texas Advanced Computing Center (TACC) at The University of Texas at Austin for providing HPC resources that have contributed to the research results reported within this paper.
References
Full text links
Read article at publisher's site: https://doi.org/10.1002/art.39476
Read article for free, from open access legal sources, via Unpaywall:
https://europepmc.org/articles/pmc4767670?pdf=render
Citations & impact
Impact metrics
Citations of article over time
Alternative metrics
Article citations
Immunogenetics of Systemic Sclerosis.
Genes (Basel), 15(5):586, 05 May 2024
Cited by: 0 articles | PMID: 38790215 | PMCID: PMC11121022
Review Free full text in Europe PMC
The Pathophysiological Roles of Regulatory T Cells in the Early Phase of Systemic Sclerosis.
Front Immunol, 13:900638, 24 May 2022
Cited by: 6 articles | PMID: 35686127 | PMCID: PMC9172592
Review Free full text in Europe PMC
Screening and identification of potential biomarkers and therapeutic targets for systemic sclerosis-associated interstitial lung disease.
Arch Rheumatol, 36(4):548-559, 24 Jun 2021
Cited by: 0 articles | PMID: 35382367 | PMCID: PMC8957772
Single nucleotide polymorphism rs5029937 in TNFAIP3 gene is correlated with risk of rheumatoid arthritis.
Med J Islam Repub Iran, 35:42, 31 Mar 2021
Cited by: 2 articles | PMID: 34268230 | PMCID: PMC8271223
The Ubiquitin Proteasome System and Skin Fibrosis.
Mol Diagn Ther, 25(1):29-40, 12 Jan 2021
Cited by: 11 articles | PMID: 33433895
Review
Go to all (16) article citations
Data
Data behind the article
This data has been text mined from the article, or deposited into data resources.
BioStudies: supplemental material and supporting data
SNPs (Showing 11 of 11)
- (9 citations) dbSNP - rs79411652
- (7 citations) dbSNP - rs58905141
- (6 citations) dbSNP - rs41290329
- (4 citations) dbSNP - rs79365263
- (2 citations) dbSNP - rs78409037
- (2 citations) dbSNP - rs12926702
- (2 citations) dbSNP - rs7823944
- (2 citations) dbSNP - rs75701002
- (1 citation) dbSNP - rs77533229
- (1 citation) dbSNP - rs5029939
- (1 citation) dbSNP - rs58417815
Show less
Similar Articles
To arrive at the top five similar articles we use a word-weighted algorithm to compare words from the Title and Abstract of each citation.
Brief report: candidate gene study in systemic sclerosis identifies a rare and functional variant of the TNFAIP3 locus as a risk factor for polyautoimmunity.
Arthritis Rheum, 64(8):2746-2752, 01 Aug 2012
Cited by: 46 articles | PMID: 22488580
Association of the TNFAIP3 rs5029939 variant with systemic sclerosis in the European Caucasian population.
Ann Rheum Dis, 69(11):1958-1964, 28 May 2010
Cited by: 87 articles | PMID: 20511617
Matrix metalloproteinase gene polymorphisms and susceptibility to systemic sclerosis.
Genet Mol Res, 15(4), 19 Dec 2016
Cited by: 6 articles | PMID: 28002595
PLD4 as a novel susceptibility gene for systemic sclerosis in a Japanese population.
Arthritis Rheum, 65(2):472-480, 01 Feb 2013
Cited by: 48 articles | PMID: 23124809
Funding
Funders who supported this work.
Department of the Army, Medical Research Acquisition Activity (1)
Grant ID: PR064803
NCATS NIH HHS (1)
Grant ID: UL1 TR000371
NCI NIH HHS (1)
Grant ID: R01 CA169122
NHLBI NIH HHS (1)
Grant ID: R01 HL116720
NIAID NIH HHS (2)
Grant ID: U01 AI090909
Grant ID: 1U01 AI090909
NIH (4)
Grant ID: R01-CA-169122
Grant ID: U01
Grant ID: R01-HL-116720
Grant ID: 1U01-AI-09090
Scleroderma Foundation (1)
Grant ID: 2012