Abstract
Objective
Although glucagon-secreting α-cells and insulin-secreting β-cells have opposing functions in regulating plasma glucose levels, the two cell types share a common developmental origin and exhibit overlapping transcriptomes and epigenomes. Notably, destruction of β-cells can stimulate repopulation via transdifferentiation of α-cells, at least in mice, suggesting plasticity between these cell fates. Furthermore, dysfunction of both α- and β-cells contributes to the pathophysiology of type 1 and type 2 diabetes, and β-cell de-differentiation has been proposed to contribute to type 2 diabetes. Our objective was to delineate the molecular properties that maintain islet cell type specification yet allow for cellular plasticity. We hypothesized that correlating cell type-specific transcriptomes with an atlas of open chromatin will identify novel genes and transcriptional regulatory elements such as enhancers involved in α- and β-cell specification and plasticity.Methods
We sorted human α- and β-cells and performed the "Assay for Transposase-Accessible Chromatin with high throughput sequencing" (ATAC-seq) and mRNA-seq, followed by integrative analysis to identify cell type-selective gene regulatory regions.Results
We identified numerous transcripts with either α-cell- or β-cell-selective expression and discovered the cell type-selective open chromatin regions that correlate with these gene activation patterns. We confirmed cell type-selective expression on the protein level for two of the top hits from our screen. The "group specific protein" (GC; or vitamin D binding protein) was restricted to α-cells, while CHODL (chondrolectin) immunoreactivity was only present in β-cells. Furthermore, α-cell- and β-cell-selective ATAC-seq peaks were identified to overlap with known binding sites for islet transcription factors, as well as with single nucleotide polymorphisms (SNPs) previously identified as risk loci for type 2 diabetes.Conclusions
We have determined the genetic landscape of human α- and β-cells based on chromatin accessibility and transcript levels, which allowed for detection of novel α- and β-cell signature genes not previously known to be expressed in islets. Using fine-mapping of open chromatin, we have identified thousands of potential cis-regulatory elements that operate in an endocrine cell type-specific fashion.Free full text

Integration of ATAC-seq and RNA-seq identifies human alpha cell and beta cell signature genes
Abstract
Objective
Although glucagon-secreting α-cells and insulin-secreting β-cells have opposing functions in regulating plasma glucose levels, the two cell types share a common developmental origin and exhibit overlapping transcriptomes and epigenomes. Notably, destruction of β-cells can stimulate repopulation via transdifferentiation of α-cells, at least in mice, suggesting plasticity between these cell fates. Furthermore, dysfunction of both α- and β-cells contributes to the pathophysiology of type 1 and type 2 diabetes, and β-cell de-differentiation has been proposed to contribute to type 2 diabetes. Our objective was to delineate the molecular properties that maintain islet cell type specification yet allow for cellular plasticity. We hypothesized that correlating cell type-specific transcriptomes with an atlas of open chromatin will identify novel genes and transcriptional regulatory elements such as enhancers involved in α- and β-cell specification and plasticity.
Methods
We sorted human α- and β-cells and performed the “Assay for Transposase-Accessible Chromatin with high throughput sequencing” (ATAC-seq) and mRNA-seq, followed by integrative analysis to identify cell type-selective gene regulatory regions.
Results
We identified numerous transcripts with either α-cell- or β-cell-selective expression and discovered the cell type-selective open chromatin regions that correlate with these gene activation patterns. We confirmed cell type-selective expression on the protein level for two of the top hits from our screen. The “group specific protein” (GC; or vitamin D binding protein) was restricted to α-cells, while CHODL (chondrolectin) immunoreactivity was only present in β-cells. Furthermore, α-cell- and β-cell-selective ATAC-seq peaks were identified to overlap with known binding sites for islet transcription factors, as well as with single nucleotide polymorphisms (SNPs) previously identified as risk loci for type 2 diabetes.
Conclusions
We have determined the genetic landscape of human α- and β-cells based on chromatin accessibility and transcript levels, which allowed for detection of novel α- and β-cell signature genes not previously known to be expressed in islets. Using fine-mapping of open chromatin, we have identified thousands of potential cis-regulatory elements that operate in an endocrine cell type-specific fashion.
1. Introduction
Glucose homeostasis is regulated closely by pancreatic α- and β-cells, which secrete glucagon to raise and insulin to decrease plasma glucose levels, respectively. Despite these distinct functions, the two cell types share a common developmental origin [1] and similar epigenetic regulation of gene expression [2]. Dysfunction of α- and β-cells contributes to the phenotypes of both type 1 and type 2 diabetes [3], [4]; however, the molecular pathophysiological mechanisms by which this occurs are not well understood. Most prior studies investigating the roles of transcriptional regulatory networks under normal and disease conditions have used whole islets, making it difficult to determine which regulatory elements such as promoters or enhancers are specifically active in β-cells versus other islet cell types. This is particularly problematic in studies of human islets, where β-cells make up on average only 54% of all endocrine cells, and can range as low as 28% [5]. Our laboratory previously reported that although the transcriptomes of sorted human α- and β-cells are fairly distinct, their histone methylation marks are more similar than expected [2]. These findings help to explain how various experimental models result in transdifferentiation of α-cells into β-cells, or vice-versa [6], [7], [8], [9], [10], [11], [12], [13]. However, it is unclear how human islet cells maintain cell type specification under normal conditions, yet allow for plasticity under conditions of metabolic stress [10]. We hypothesized that correlating cell type-specific transcriptomes with an atlas of open chromatin could identify novel cis-regulatory elements involved in these processes.
In this study, we utilized the “Assay for Transposase-Accessible Chromatin with high throughput sequencing” (ATAC-seq) [14], [15] to detect open chromatin regions in highly enriched human α- and β-cells. We then correlated these maps of accessible chromatin with mRNA-seq data from sorted human α- and β-cells to identify the cis-regulatory elements that may be responsible for the regulation of cell type-specific signature genes (Figure 1A). Notably, we identified many novel α-cell- and β-cell-selective genes.
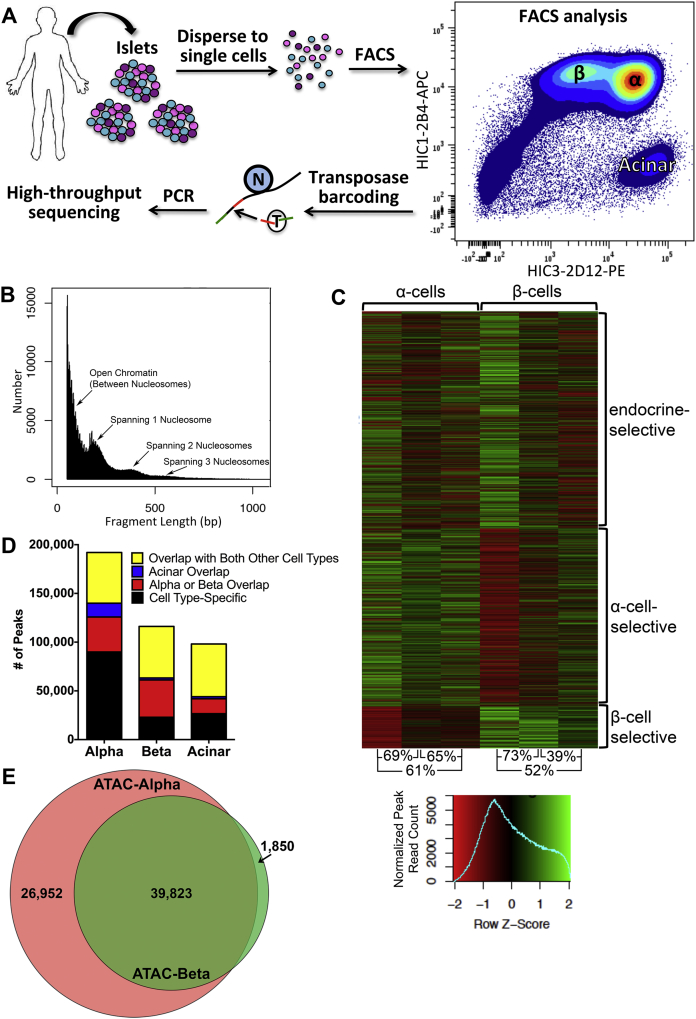
ATAC-seq results in sorted human α-, β-, and acinar cells. (A) Experimental design. Islets from deceased organ donors were dispersed and FACS sorted into α-, β-, and acinar cell fractions, and processed for ATAC-seq analysis. N: nucleosome; T: transposase. Red and green bars represent PCR/sequencing barcodes. (B) Fragment lengths within a representative ATAC-seq library. The small fragments represent sequence reads in open chromatin, while the peak at ~150 bp results from sequence reads that span one nucleosome, and larger peaks represent progressively more compact chromatin. (C) Heatmap of ATAC-seq peak data showing clustering of endocrine-selective peaks (present in α- and β-, but not acinar cells), α-cell-selective peaks, and β-cell-selective peaks. Inter-sample correlation is noted at the bottom. (D) Number of peaks identified by ATAC-seq in each cell type that are specific to that cell type versus also found in either of the other two cell types investigated. (E) Venn diagram of overlap of α-cell-selective and β-cell-selective ATAC-seq peaks, after removal of peaks also found in acinar cells.
2. Material and methods
2.1. Human islets
Human islets from deceased organ donors (Supplemental Table 1) were provided by the Islet Cell Resource Center of the University of Pennsylvania and the Integrated Islet Distribution Program (iidp.coh.org). After shipment, islets were cultured either in CMRL1066 with 0.5% human albumin, 2 mM l-glutamine, 1% heparin, and 1% penicillin/streptomycin or in Prodo PIM(S) media supplemented with 5% PIM(ABS) and 1% PIM(G) at 37 °C with 5% CO2 prior to fluorescence-activated cell sorting (FACS). FACS was performed as described in [2] using antibodies against cell surface markers as reported in [16].
2.2. ATAC-seq
ATAC-seq was performed as described [15] on three α-cell, three β-cell, and two acinar FACS sorted cell samples (Supplemental Table 1). Reagent volumes were adjusted according to starting cell number. Libraries were generated using the Ad1_noMX and Ad2.1–2.4 barcoded primers from [14] and were amplified for 7–9 total cycles. Libraries were purified with AMPure beads (Agencourt) to remove contaminating primer dimers. Library quality was assessed using the Agilent Bioanalyzer High-Sensitivity DNA kit. Libraries were quantitated using Qubit.
All libraries were sequenced on the Illumina HiSeq 2500 with 50 bp paired-end reads, then on the Illumina NextSeq 500 with 40 bp paired-end reads. Illumina adapters were trimmed by Trimmomatic [17], and the 50 bp reads were shortened by 10 bp with fastx_trimmer (http://hannonlab.cshl.edu/fastx_toolkit/). All reads for each sample were combined and aligned to hg18 with bowtie2 [18] using default settings. Reads were aligned to hg18 so that data could be compared to relevant published ChIP-seq (Chromatin Immunoprecipitation followed by high throughput sequencing) and FAIRE-seq (Formaldehyde-Assisted Isolation of Regulatory Elements followed by high throughput sequencing) data [2], [19]. Greater than 100 million reads were obtained for each library, and reads mapping to mitochondrial DNA were excluded from the analysis together with low quality reads (MAPQ < 10, duplicates and reads in Encode blacklist regions). Between 30 million and 233 million high-quality reads per sample that mapped to genomic DNA were retained. All mapped reads were offset by +4 bp for the +strand and −5 bp for the −strand [14]. Peaks were called for each sample using MACS2 [20] with parameters “-q 0.05 --nomodel --shift 37 --extsize 73”, and differential peaks were identified using the MACS2 bdgdiff module comparing different cell types from the same donor. Then peaks were merged for the same cell types using Bedtools [21]. Individual peaks separated by <100 bp were joined together. Peak annotation was performed using HOMER [22]. Motif analysis on peak regions was performed by HOMER function findMotifsGenome.pl. All sequencing tracks were viewed using the Integrated Genomic Viewer (IGV 2.3.61). All genomics datasets were deposited in GEO under accession number GSE76268.
2.3. mRNA-seq
mRNA-seq was performed on seven α-cell and eight β-cell sorted samples from ten different islet donors (Supplemental Table 1). Results from three of these samples were previously published [2]. For the results presented here, all mRNA-seq libraries were generated using the Illumina TruSeq Stranded Total RNA LT Sample Prep Kit (Cat. #RS-122-2301). Sequencing and computational analysis were performed as described in [2].
2.4. Protein expression
Whole islets or trypsin-dispersed islet cells were fixed in 4% paraformaldehyde, then washed with phosphate-buffered saline (PBS). Dispersed islet cells were attached to positively-charged slides using a CytoSpin. Islets were incubated in blocking solution (PBS with 0.3% Triton and 10% fetal bovine serum [FBS]), and slides in CAS-Block (ThermoFisher), prior to and concurrent with antibodies. Primary antibodies (rabbit anti-GC [Abcam ab65636], rabbit anti-CHODL [Abcam ab134924], mouse anti-glucagon [Abcam ab82270], guinea pig anti-insulin [Invitrogen 180067], rabbit anti-somatostatin [Santa Cruz sc-13099], goat anti-pancreatic polypeptide [PP; Abcam ab77192], and goat anti-ghrelin [Santa Cruz sc-10368]; all 1:100) were incubated overnight at 4 °C, and secondary antibodies (anti-rabbit Cy2 or Cy3, anti-mouse Cy3 or Cy5, anti-goat Cy3, and anti-guinea pig Cy5 [Jackson ImmunoResearch]; all 1:200) were incubated at room temperature for 3 h. DAPI (4′,6-diamidino-2-phenylindole) was added with the mounting media to counterstain DNA. Whole islets were imaged on a Leica TCS SP8 confocal microscope, and dispersed cells were imaged on a Nikon Eclipse 80i widefield microscope.
3. Results
3.1. Mapping α- and β-cell open chromatin with ATAC-seq
To correlate fine-mapped open chromatin regions with gene expression profiles, we followed the experimental paradigm outlined in Figure 1A. Human islets were dispersed into single cell suspension with trypsin, labeled with antibodies, and subjected to FACS as published previously [2]. Sorted cell fractions were subjected to “transposase barcoding”, in which the Tn5 transposase integrates sequencing adapters only into open chromatin regions, but is prevented from doing so in genomic regions tightly packed into nucleosomal arrays [15]. All ATAC-seq libraries yielded the expected distribution of fragment lengths, with the majority of fragments being small, representing internucleosome open chromatin, and progressively fewer fragments of larger size which are spanning nucleosomes (Figure 1B). As predicted by prior expression profiling data, the open chromatin structure of the two endocrine cell types were more similar to each other than either was to that of acinar cells (Figure 1C,D). Approximately 78% of peaks identified by ATAC-seq in β-cells were also present in α-cells, and 47% also occurred in acinar cells (Figure 1D). Similarly, 46% of peaks detected in α-cells were also observed in β-cells, while only 34% were also seen in acinar cells. Endocrine-specific peaks were categorized as those found in α- and β- but not acinar cells, and nearly 40,000 such regions were identified (Figure 1E). Interestingly, nearly 27,000 ATAC-seq peak regions were classified as α-cell-specific, whereas only 1,850 β-cell-specific regions were found.
Overall, our ATAC-seq data for sorted α- and β-cells correlated well (60%) with published FAIRE-seq results using whole islets [19] (Figure 2A). However, our ATAC-seq analysis identified many more open chromatin regions and associated genes in sorted α- and β-cells compared to whole islet FAIRE-seq (Figure 2B), as expected given the much higher sensitivity and resolution of the ATAC-seq methodology. This is easily seen when comparing the ATAC-seq and FAIRE-seq profiles of specific genes, such as the ARX locus (Figure 2C). There are strong ATAC-seq peaks in α-cells at the ARX promoter and at known enhancers within the third intron and within an intron of a neighboring gene [23] that are not present in β- or acinar cells, while the previously published whole islet FAIRE-seq signals [19] are very broad and do not detect these α-cell-specific open chromatin regions. Furthermore, ATAC-seq identified an α-cell-specific peak approximately 5 kb upstream of the ARX promoter that overlapped with α-cell-specific H3K4me3 and whole islet H2A.Z, indicating that this region may function as an enhancer; again, this region was not recognized by whole islet FAIRE-seq [19].
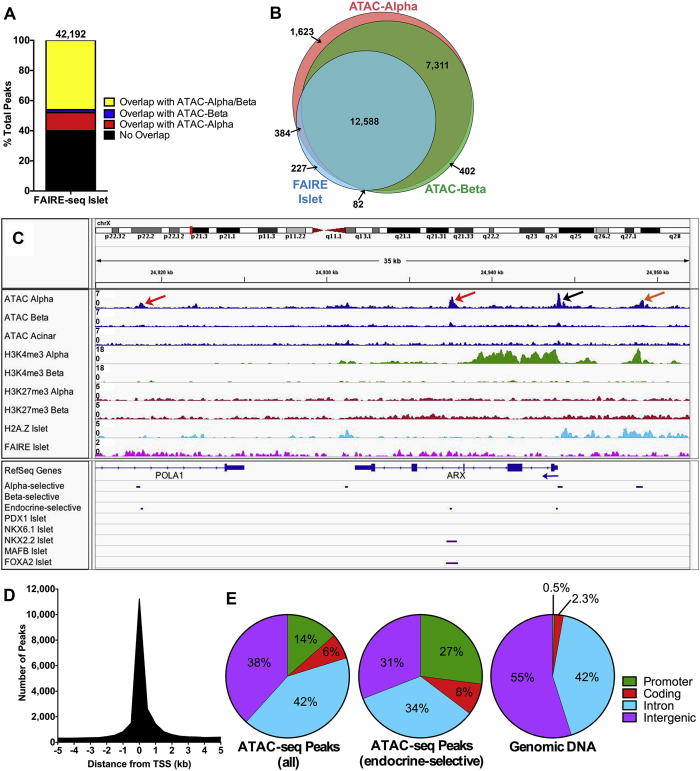
Integration of ATAC-seq data with other genomics datasets. (A) Bar graph of % of overlapping open chromatin regions identified by FAIRE-seq [32] in whole islets versus by ATAC-seq in α- and β-cells (including peaks also found in acinar cells). Total number of FAIRE-seq peaks is noted at top. (B) Venn diagram of distinct genes with open chromatin regions in α- and β-cells identified by ATAC-seq (including peaks also found in acinar cells) versus in whole islets identified by FAIRE-seq. (C) Sequencing tracks for the ARX locus shows distinct α-cell-specific ATAC-seq peaks at the promoter (black arrow), at known intronic and distal enhancers (red arrows), and at a putative 5′ enhancer (orange arrow), none of which were identified by FAIRE-seq. (D) Histogram of distance from the nearest transcriptional start site (TSS) for all ATAC-seq peaks within 5 kb of the nearest TSS that were identified in α- and β-cells. Not shown are peaks 5–280 kb from the nearest TSS. (E) Proportions of the ATAC-seq peak regions identified in α- and β-cells that represent the various genome annotations, compared to the representation of a given sequence element in the human genome [47].
Most ATAC-seq peaks from the α-, β-, and acinar cell samples mapped to within 250 bp of transcriptional start sites (TSS; Figure 2D), marking the accessible chromatin of promoters. In fact, the ATAC-seq dataset was significantly enriched (~28-fold) for promoter regions compared to the overall abundance of promoters in the genome (Figure 2E). Notably, there was even greater enrichment (~54-fold) for open promoter regions in the peaks that were specifically identified in α- and β-cells. In addition, many open chromatin regions identified in our analysis were located in introns and intergenic regions, suggestive of enhancers (Figure 2E).
3.2. Integration of ATAC-seq and mRNA-seq results
To determine whether cell type-selective open chromatin regions from the ATAC-seq analysis correlated with cell type-selective gene expression, we integrated our α- and β-cell ATAC-seq data with α- and β-cell mRNA-seq data. Overall, 785 genes that were expressed at significantly higher levels in α- versus β-cells (defined as ≥2-fold difference, with a false discovery rate [FDR] <0.1) had at least one associated α-cell-specific open chromatin region that was not identified in β- or acinar cells (Figure 3A), which accounted for 78% of differentially-expressed α-cell genes. In contrast, only 41% of differentially expressed β-cell genes were similarly identified as having β-cell-specific open chromatin regions. These results suggest that open chromatin may be a better predictor of gene activation in α-cells than in β-cells, perhaps due to inherent differences in gene regulation in these two different cell populations, or possibly due to a higher degree of cellular heterogeneity within the β-cell versus α-cell population.
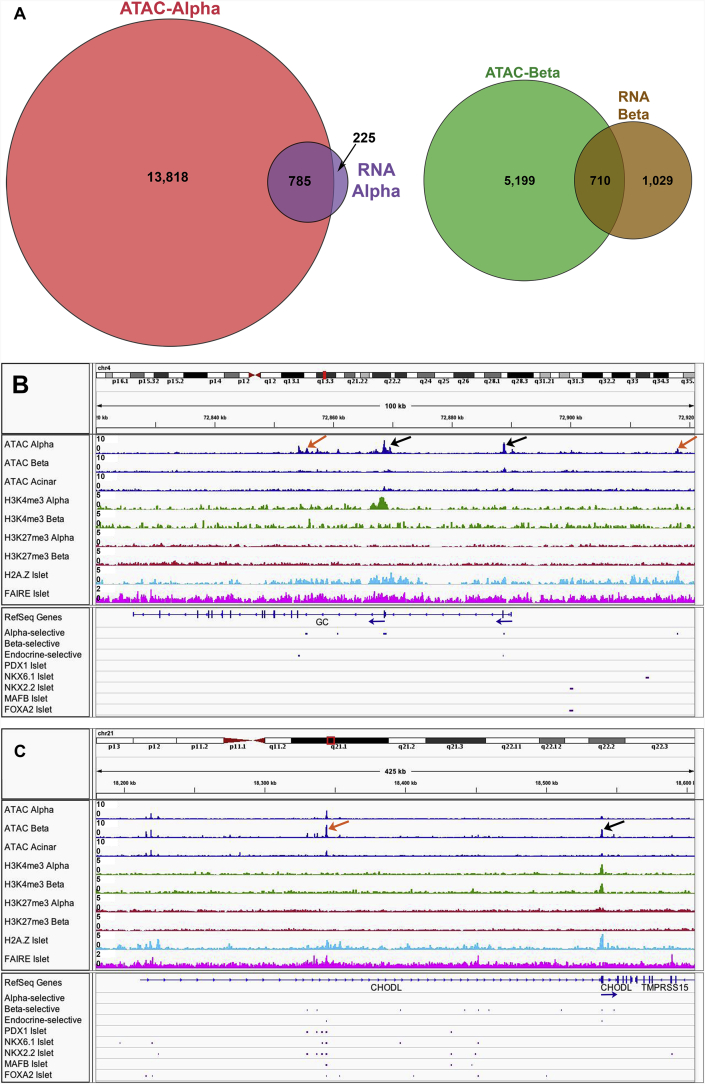
Integration of ATAC-seq data with mRNA-seq, histone marks, FAIRE-seq, and transcription factor binding data for novel α- and β-cell genes. (A) Venn diagrams indicating the proportion of genes that are differentially expressed in α- or β-cells (based on mRNA-seq) that have cell type-selective ATAC-seq peaks (identified only in α- or β-cells, including peaks also identified in acinar cells). (B) Pooled sequencing tracks for the GC locus from α-cell, β-cell, and acinar cell ATAC-seq data (blue); α-cell and β-cell H3K4me3 (green) and H3K4me27 (red) ChIP-seq data; whole islet H2A.Z ChIP-seq data (light blue); and whole islet FAIRE-seq data (pink). Cell type-selective peaks are identified in blue below the row labeled “RefSeq Genes”, followed by islet transcription factor ChIP-seq peaks in purple. Promoter regions are indicated with black arrows, and putative enhancer regions are labeled with orange arrows. (C) Pooled sequencing tracks for the CHODL gene. Annotation as in (B).
Furthermore, there were many more genes in both α- and β-cells with cell type-selective ATAC-seq peaks that were not differentially expressed in a cell type-selective manner. Only 5% of the α-cell-specific and 12% of the β-cell-specific ATAC-seq peaks mapped to differentially expressed genes (Figure 3A). These results concur with the growing understanding that gene activation depends on multiple regulatory regions, many of which are located far from the gene locus itself. In fact, further peak annotation analysis revealed no enrichment for open promoter regions compared to intronic, intergenic, or coding regions in differentially expressed genes in either cell population (Supplemental Figure 1A–H).
Narrowing the integrated ATAC-seq and mRNA-seq results to the genes that were the most highly and significantly expressed in each cell type (defined as ≥10-fold expression difference between α- and β-cells, with FDR <0.05), we identified 33 α-cell-selective and 35 β-cell-selective transcripts (Table 1). Several of the α-cell-enriched transcripts were expected from the literature (including ARX, GCG, DPP4, and IRX2), and we found that all of these loci had α-cell-selective open chromatin regions. Furthermore, α-cell-selective open promoter regions were specifically noted for ARX (Figure 2C), GCG, and DPP4 (Supplemental Figure 2A). Interestingly, 28 of the α-cell-enriched transcripts had not previously been described to be expressed in islets (Table 1). Of these novel α-cell genes, 24 were marked by α-cell-selective ATAC-seq peaks, with 12 of these having open chromatin identified in promoter regions.
Table 1
α- and β-cell-selective transcripts based on mRNA-seq.
α-Cell signature genes | β-Cell signature genes | ||||||
---|---|---|---|---|---|---|---|
Gene symbol | Any ATAC-seq peak | Peak at promoter | α-cell-specific peak | Gene symbol | Any ATAC-seq peak | Peak at promoter | β-cell-specific peak |
APOH | ACPP | ||||||
ARX | X | X | X | ADCYAP1 | X | X | |
BVES | X | X | X | ANXA8 | |||
CARTPT | X | X | ASB9 | ||||
CRH | X | X | ASCL1 | X | |||
CRYBA2 | BMP5 | ||||||
DPP4 | X | X | X | CAPN13 | |||
F10 | X | X | X | CHODL | X | X | X |
FAM83B | X | X | X | CST2 | |||
FAP | X | X | CXCL5 | ||||
GC | X | X | X | DLK1 | |||
GCG | X | X | X | ESR1 | |||
GJA3 | X | X | X | GPM6A | X | ||
IGFBP2 | X | X | GRIN2A | ||||
IRX2 | X | X | IGF2 | ||||
LOXL4 | X | X | IGSF11 | ||||
LPAR1 | X | X | INS | ||||
MBOAT4 | X | X | X | KRTAP4-7 | |||
MUC13 | X | X | LAPTM5 | ||||
MYO10 | X | X | LRFN2 | ||||
NPNT | LRRTM3 | X | |||||
POPDC3 | X | X | X | MAFA | |||
PTPRT | X | X | P2RY1 | X | |||
SERPINA1 | X | X | X | PCDH7 | X | ||
SERTM1 | X | X | X | PLCH2 | |||
SPOCK3 | X | X | PPAPDC1A | ||||
STK32B | X | X | X | PTGS2 | |||
SYNDIG | X | X | RGS16 | ||||
SYTL5 | SIX3 | ||||||
TM4SF4 | X | X | X | SLC27A6 | X | X | |
TMEM236 | STEAP3 | X | X | ||||
TMEM45B | X | X | SULF2 | ||||
TTR | X | X | X | TFCP2L1 | X | X | |
TGFBR3 | X | X | |||||
UNC5D | X |
Similarly, the β-cell-selective transcripts included those of MAFA, INS, and IGF2 as expected, but also 22 transcripts that had not previously been identified in islets. Of these novel β-cell genes, 5 had β-cell-specific ATAC-seq peaks, 2 of which had peaks in promoter regions. In contrast to what was observed in α-cells, most of the genes selectively expressed in β-cells have open chromatin regions that were identified in both α- and β- but not acinar cells (i.e. endocrine-specific, Supplemental Figure 2B). Together, these findings suggest that many genes with preferential expression in β-cells nevertheless are in an at least partially open chromatin state in α-cells, which would favor α-to β-cell transdifferentiation over the reverse process. These results thus extend and support the notion of “epigenomic plasticity” in human α-cells made previously based on the mapping of active and repressive histone marks [2].
3.3. Integration of ATAC-seq results with additional epigenetic marks
To distinguish which open chromatin regions in the α- and β-cell-selective genes may represent gene regulatory regions, we integrated our ATAC-seq data with previously published α- and β-cell ChIP-seq data for H3K4me3 (an activating histone mark) and H3K27me3 (a repressive histone mark) [2], as well as with whole islet H2A.Z ChIP-seq and FAIRE-seq data [19]. We first verified that known promoter and enhancer regions for well-established cell type-specific genes were identified within our dataset including those at the ARX [23], DPP4, and MAFA loci (Figure 2C, Supplemental Figure 2A,B), as well as for well-known pan-endocrine expressed genes such as NEUROD1 (Supplemental Figure 2C). Importantly, the genes expressed exclusively in α-cells exhibited α-cell-selective open promoter regions associated with α-cell-specific H3K4me3 marks. In contrast, as mentioned above, the genes expressed exclusively in β-cells exhibited open promoter regions in both α- and β-cells, and were associated with bivalent H3K4me3 (a mark of active promoters) and H3K27me3 (a mark of a repressed chromatin state) in α-cells. Thus, as has been suggested previously [2], our data indicate that many β-cell signature genes are bivalently marked and thus poised for activation in α-cells, which correlates with the propensity of α-cells to transdifferentiate into β-like cells under conditions of extreme metabolic stress, such as complete induced β-cell ablation [6].
Next, we identified cell type-selective open chromatin regions at the promoters of the novel α-cell signature gene GC (Figure 3B) and of the novel β-cell signature gene CHODL (Figure 3C), as examples. In addition, α-cell-selective ATAC-seq peaks were also present within an intron of GC, as well as ~28 kb upstream of the GC promoter, associated with H2A.Z, suggesting that this region may function as an α-cell-specific enhancer (Figure 3B). Similarly, multiple β-cell-selective ATAC-seq peaks are present 5′ upstream of and within an intron of CHODL, many of which overlap with β-cell-specific (PDX1, NKX6.1) and islet (NKX2.2, MAFB, FOXA2) transcription factor binding sites (Figure 3C).
3.4. Confirmation of cell type-specific protein expression of novel signature genes
Next, we wanted to determine the cell type-selective expression of our newly identified α- and β-cell signature genes extended to the protein level. For most of these genes no antibodies are commercially available at present; however, we were able to test protein expression of two genes by immunolabeling. We stained both whole human islets and dispersed islet cells with an antibody against the GC protein, and detected strong GC immunoreactivity in α-cells (Figure 4A,B), validating our RNA-seq analysis. GC protein was also present in a small subset of pancreatic polypeptide (PP)-expressing cells, but not in insulin, somatostatin, or ghrelin expressing cells (Figure 4C–E). GC, or “group-specific component”, is more commonly known as vitamin D binding protein (DBP). Its primary role is to bind and transport vitamin D to its receptor in the nucleus, which then transcriptionally activates target genes. There is currently no known role for the vitamin D receptor in α-cells, but vitamin D deficiency and metabolism have been associated with type 1 and type 2 diabetes [24]. Furthermore, common GC non-coding variants have been associated with increased risk of gestational diabetes mellitus [25], as well as altered fasting insulin levels in normoglycemic individuals, although this was attributed primarily to changes in insulin sensitivity [26]. Thus, GC activity in α-cells may influence β-cell function via indirect intercellular or paracrine interactions.
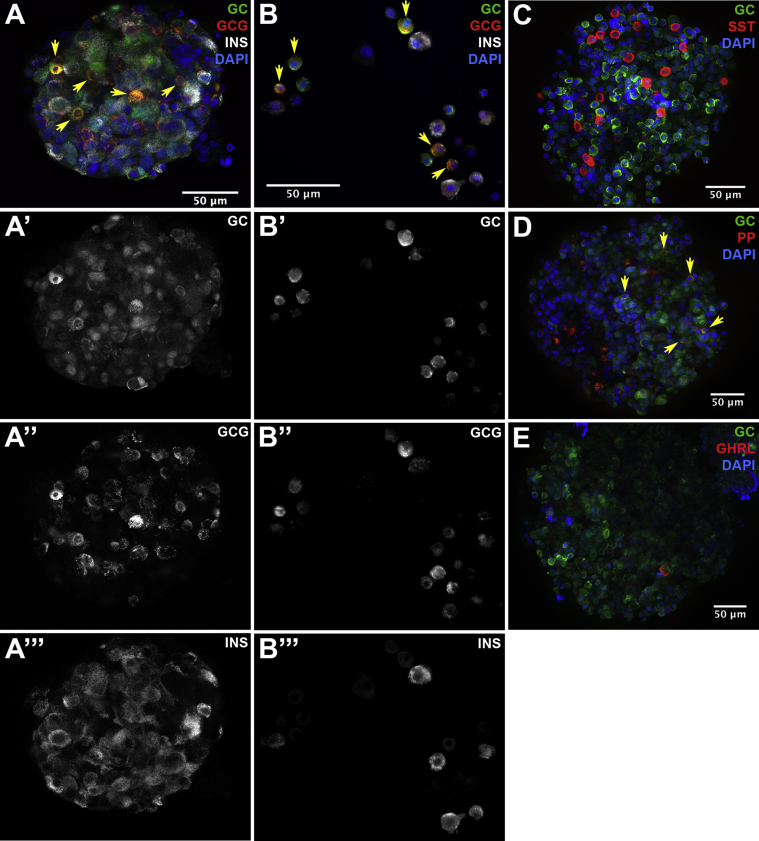
Immunofluorescent labeling for the novel α-cell-selective protein GC. Whole mount immunofluorescent labeling of human islets (A, C-E) and dispersed human islet cells (B) for GC and the islet hormones as indicated in the figure labels. Yellow arrowheads indicate cells expressing GC and islet hormones. GC: group-specific component; GCG: glucagon; INS: insulin; SST: somatostatin; PP: pancreatic polypeptide; GHRL: ghrelin; DAPI: 4′,6-diamidino-2-phenylindole.
β-cell-specific expression of the CHODL (chondrolectin) protein was also confirmed in whole human islets (Figure 5). CHODL currently has no recognized role in β-cells, but it is a member of the C-type lectin superfamily of proteins, which contain a single transmembrane domain and a calcium-dependent carbohydrate binding domain [27]. Thus, it can bind to carbohydrate moieties on glycosylated proteins. CHODL and other C-type lectin family members have been implicated in intercellular adhesion, extracellular matrix interactions, and intracellular protein transport.
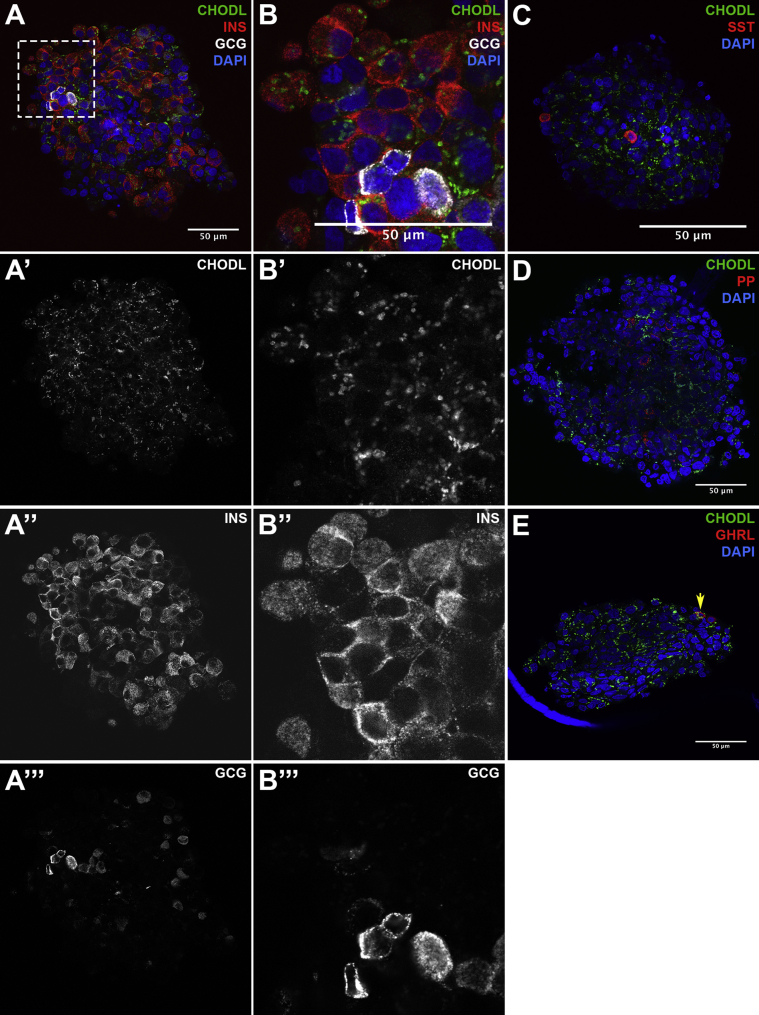
Immunofluorescent labeling for the novel β-cell-selective protein CHODL. (A–E) Whole mount immunofluorescent labeling of human islets for CHODL and the islet hormones as indicated in the figure labels. The boxed region in (A) is magnified in (B). CHODL: chondrolectin; GCG: glucagon; INS: insulin; SST: somatostatin; PP: pancreatic polypeptide; GHRL: ghrelin; DAPI: 4′,6-diamidino-2-phenylindole.
3.5. Transcription factor binding motifs in α- and β-cell-selective open chromatin regions
Because many of the genes with annotated α- or β-cell-selective ATAC-seq peaks were not differentially expressed (Figure 3A), we sought to determine whether the cell type-selective peak regions enriched for particular transcription factor binding sites. Not surprisingly, α-cell open chromatin regions were enriched for binding sites of transcription factors known to play important roles in α-cells, including the FOX factors, ISL1, and MAFB (Table 2). Other enriched binding sites included those for FRA1, TFAP4, RFX5, CTCF, ATF2, STAT1, and GATA3. Expression of all of these transcription factors was confirmed in α-cells by mRNA-seq (data not shown).
Table 2
Transcription factor binding motifs enriched in α- or β-cell-specific open chromatin regions.
Factor | Binding Motif | % Regions | P-value |
---|---|---|---|
α-Cells | |||
FRA1/FOSL1 | ![]() | 21.4% | 10−1145 |
FOX | ![]() | 19.7% | 10−432 |
RFX5 | ![]() | 5% | 10−386 |
CTCF | ![]() | 3.9% | 10−349 |
AP4/TFAP4 | ![]() | 41.2% | 10−235 |
RFX4 | ![]() | 42.5% | 10−181 |
ATF2 | ![]() | 5.4% | 10−139 |
ISL1 | ![]() | 26% | 10−128 |
STAT1 | ![]() | 40.1% | 10−121 |
GATA3 | ![]() | 12.1% | 10−111 |
E2F6 | ![]() | 32.6% | 10−97 |
IRF4 | ![]() | 25.8% | 10−86 |
MAFK | ![]() | 6.9% | 10−84 |
MAFB | ![]() | 76.9% | 10−62 |
HAND1 | ![]() | 13.6% | 10−44 |
β-Cells | |||
FRA1/FOSL1 | ![]() | 47.2% | 10−264 |
LHX2 | ![]() | 51.4% | 10−92 |
AP4/TFAP4 | ![]() | 23% | 10−32 |
CUX1 | ![]() | 22.4% | 10−30 |
FOX | ![]() | 18.6% | 10−29 |
PIT1/POU1F1 | ![]() | 6.3% | 10−19 |
JUN-FOS | ![]() | 4.2% | 10−18 |
SMAD2 | ![]() | 7% | 10−14 |
MEF2C | ![]() | 3.2% | 10−12 |
Binding site motifs for FRA1, TFAP4, and the FOX factors were also enriched in β-cell open chromatin regions, as was the motif for SMAD2, which is known to mediate TGF-β signaling and affect pancreatic endocrine cell development as well as mature β-cell function [28], [29] (Table 2). Binding sites for other transcription factors that do not have known roles in β-cells were also enriched. Again, expression of these transcription factors in human β-cells was confirmed by mRNA-seq (data not shown).
3.6. Diabetes risk loci within open chromatin regions identified by ATAC-seq
We also sought to determine whether α- or β-cell-selective open chromatin regions in our ATAC-seq dataset included single nucleotide polymorphism (SNP) loci previously found in genome-wide association studies (GWAS) to be associated with increased risk of type 1 or type 2 diabetes mellitus [30], [31]. Notably, two risk loci for type 2 diabetes were identified in endocrine-specific open chromatin by ATAC-seq: rs7732130 and rs7903146, which are located within introns of TCF7L2 and ZBED3-AS1, respectively (Table 3). Eleven additional type 2 diabetes risk loci were located near cell type-specific open chromatin regions, of which 6 were specific to α-cells. These findings suggest that multiple type 2 diabetes risk loci might be associated with α-cell dysfunction.
Table 3
Diabetes risk-associated SNPs near α- or β-cell-specific ATAC-seq peaks.
SNP | Associated genes | Location in gene | ATAC-seq peak cell type-specificity | Preferential mRNA expression | TF binding sites [19] |
---|---|---|---|---|---|
Type 2 diabetes mellitus | |||||
rs7732130a | TCF7L2 | Intron | Endocrine cells | Same | NKX6.1, NKX2.2, FOXA2, MAFB |
rs7732130a | ZBED3-AS1 | Intron | Endocrine cells | β-cells | NKX2.2 |
rs1169288 | HNF1A | 5’ UTR | Endocrine cells | Same | NKX2.2, FOXA2 |
rs1800574 | HNF1A | 5′ UTR | Endocrine cells | Same | NKX2.2, FOXA2 |
rs5215 | KCNJ11 | 5′ UTR | Endocrine cells | β-cells | FOXA2 |
rs17066842 | MC4R | n/a (5′) | Endocrine cells | Same | NKX2.2 |
rs13266634 | SLC30A8 | Intron | Endocrine cells | Same | NKX2.2, FOXA2 |
rs11708067a | ADCY5 | Intron | α-cells | Same | none |
rs10811660a | CDKN2A-CDKN2B | n/a (5′) | α-cells | Same | MAFB, FOXA2 |
rs10757283 | CDKN2A-CDKN2B | n/a (5′) | α-cells | Same | MAFB, FOXA2 |
rs2237895 | KCNQ1 | Intron | α-cells | Same | NKX2.2, FOXA2 |
rs74046911 | KCNQ1 | Intron | α-cells | Same | NKX2.2, FOXA2 |
rs458069 | KCNQ1 | Intron | α-cells | Same | NKX2.2, FOXA2 |
Type 1 diabetes mellitus | |||||
rs11755527 | BACH2 | Intron | Endocrine cells | Same | None |
rs10517086 | none | n/a | Endocrine cells | n/a | None |
TF: transcription factor; UTR: untranslated region; n/a: not applicable.
4. Discussion
In this study, we utilized the sensitive ATAC-seq technology to determine regions of open chromatin in highly enriched populations of human α-, β-, and acinar cells. This is the first evaluation of open chromatin in distinct islet cell subtypes. Previous studies have performed FAIRE-seq on whole human islets [19], [32], but because of the multiple cell types included in such analyses and the variability of islet composition (28–70% β-cells, 10–60% α-cells, 1–20% δ-cells, as well as up to 25% each of contaminating duct and acinar cells [5], [33]), these prior studies were not designed to detect cell type-specific chromatin states. ATAC-seq also provides several advantages over the older techniques such as FAIRE-seq or DNase-seq for the identification of open chromatin regions. First, ATAC-seq requires many fewer cells than the two older techniques, making subtype analysis possible, and second, ATAC-seq results have a much higher signal-to-noise ratio than FAIRE-seq, as documented above, and map open chromatin regions much more precisely [34].
Using ATAC-seq for purified islet cell subtypes, we identified cell type-specific open chromatin regions that were not apparent from the whole islet FAIRE-seq data. By integrating ATAC-seq data with mRNA-seq data from sorted human α- and β-cells, we were also able to locate open chromatin regions in genes that previously were not known to be expressed in these cell types. Importantly, we defined novel sets of both α- and β-cell ‘signature genes’, i.e. genes with high expression in one cell type or the other, but not both. These data will provide useful guideposts for the efforts in the field to derive β-cells from human embryonic stem cells or induced pluripotent stem cells, as they allow for an easy distinction between intermediate endocrine cells and mature β-cells.
A limitation of this study is reliance on obtaining cells for analysis from human deceased organ donors, among which there is unknown but likely significant inherent genetic, epigenetic, and physiological variation. Furthermore, there is significant variability among the samples due to the fact that each islet sample must be sorted and undergo the transposase reaction individually. In an attempt to limit the effects of such variation, we performed differential peak calling of α- versus β-cells separately for each donor, then pooled the results and discarded any peaks that were differentially represented in the other cell type from another donor, in order to arrive at cell type-selective peaks. However, it is possible that such criteria resulted in inclusion of peaks that were only significant in one sample and thus perhaps not truly reflective of canonical α- or β-cell-specific open chromatin regions. This could contribute to the fact that a large number of cell type-selective ATAC-seq peaks were identified in genes that were not differentially expressed in these cell types. Furthermore, because different donor samples were used for ATAC-seq and mRNA-seq, it is not surprising that there is incomplete overlap of these two datasets. However, as previously mentioned, that result could also reflect the fact that small regions of open chromatin alone are not sufficient for gene activation.
Similar to our previous findings in murine liver [35], this study indicates that nucleosome structure in mammalian cell types is remarkably consistent. In that study, nucleosome positions were mapped genome-wide through micrococcal nuclease digestion followed by sequencing of the remaining, nucleosome-protected DNA. If nucleosome position were highly variable from cell to cell, then we would have expected to find few if any nucleosome peaks within a specific cell population, but instead we found strong, well-spaced peaks in the nucleosome maps of hepatocytes [35], indicating that these positions are similar between at least the majority of hepatocytes. Likewise, in the current study, the fact that ATAC-seq mapping of open chromatin regions in human α- and β-cells produced clearly defined peaks indicates that the flanking nucleosomes are fixed in place, or nearly so.
The current and previous [2] results indicate that many genes in α-cells are “poised” to be activated, and that many of these “poised” genes are β-cell signature genes. This is reflected in the ATAC-seq dataset by the finding of many more open chromatin regions in α-cells versus β-cells. We also performed de novo transcription factor binding site motif analysis using α- and β-cell-selective open chromatin regions. In addition to the expected enrichment for binding sites of transcription factors already known to play important roles in α- and β-cells, we also identified several additional transcription factors whose binding site motifs were enriched in our dataset. This exploration uncovered novel putative transcriptional regulators within human α- and β-cells.
For example, we found the CTCF consensus motif significantly enriched in open chromatin regions of α-cells (Supplemental Figure 2D). CTCF has been shown to repress Pax6 expression in murine α-cells, and global constitutive over-expression of CTCF in a transgenic mouse model significantly impaired α-cell differentiation and glucagon expression [36], similar to what was observed in Pax6 deletion mouse models [37], [38], [39], [40]. However, only α-cells were affected in the CTCF overexpression model, while in the Pax6 deletion models, both α- and β-cell populations were affected, although β-cells to a lesser degree. These results suggest that CTCF regulates PAX6 expression only in α-cells but not β-cells, despite PAX6 being expressed to similar levels in both cell types, and despite α- and β-cells having similar open chromatin maps at the PAX6 locus (Supplemental Figure 2D). Because CTCF functions as a general transcriptional insulator [41], it may be responsible for binding to open chromatin regions in α-cells and preventing or limiting gene activation, which may be one of the mechanisms by which α-cells are able to maintain their cell-specific gene expression pattern despite displaying an open chromatin at many β-cell signature loci.
STAT1, another transcription factor whose binding site motif was enriched in α-cell-selective open chromatin regions, has been implicated in antiviral intracellular responses in α-cells [42], but its specific targets are still unknown. Furthermore, FRA1 and TFAP4 binding sites were highly enriched in both α- and β-cells, suggesting that these factors may function in both cell types. FRA1 has been implicated in mediating TGF-β signaling with SMAD2 in other cell types [43]. TFAP4 has been shown to regulate proliferation in other cell types by directly repressing transcription of the cell cycle inhibitors p16 and p21 [44], both of which are important regulators of β-cell replication [45], [46]. Thus, further study of these transcription factors in pancreatic islets is warranted.
In summary, we provide here a novel resource for identifying open chromatin regions in human α-, β-, and acinar cells. This dataset builds upon the growing body of genome-wide epigenetic studies performed in purified human α- and β-cells. We have shown that integrating ATAC-seq data with other epigenetic information enhances interpretation of computational results, and we anticipate that this ATAC-seq dataset will be useful for integration with future genomic analyses of human islets. Such studies are important for understanding the tightly regulated gene expression networks that make and sustain functioning α- and β-cells, determining how these networks become dysregulated in disease states such as diabetes, and identifying ways in which these networks can be manipulated for diabetes therapy.
5. Conclusions
We present the first analysis of open chromatin in purified human α- and β-cells using highly sensitive ATAC-seq. This technique allowed for precise mapping of potential α- and β-cell-specific gene regulatory regions. Integration with human α- and β-cell transcriptomes led to the identification of novel signature genes for these two cell types. Further mining of the open chromatin regions defined by ATAC-seq revealed overlap with several diabetes risk SNPs, as well as enrichment for novel transcription factors that may play important roles in α- and β-cells. This study provides an important dataset and multiple new avenues of investigation for the future.
Acknowledgments
We thank the following core facilities at the University of Pennsylvania for their assistance in obtaining the data presented here: Bioinformatics Core, Cell and Developmental Microscopy Core, Flow Cytometry and Cell Sorting Resource Laboratory, and the Functional Genomics and Islet Cell Biology Cores of the Penn Diabetes Research Center (P30-DK19525). We also thank the Islet Cell Resource Center of the University of Pennsylvania and the Integrated Islet Distribution Program for providing human islets, as well as the donors and their families. We thank Drs. Nuria Bramswig, Vasumathi Kameswaran, and Logan Everett for their contributions to the RNA-seq datasets. This work was supported by the Juvenile Diabetes Research Foundation grant 3-PDF-2014-186-A-N to AMA and by NIDDK grant UC4DK104119 to KHK.
Footnotes
Appendix ASupplementary data related to this article can be found at http://dx.doi.org/10.1016/j.molmet.2016.01.002.
Appendix A. Supplementary data
The following are the supplementary data related to this article:
Supplemental Figure 1
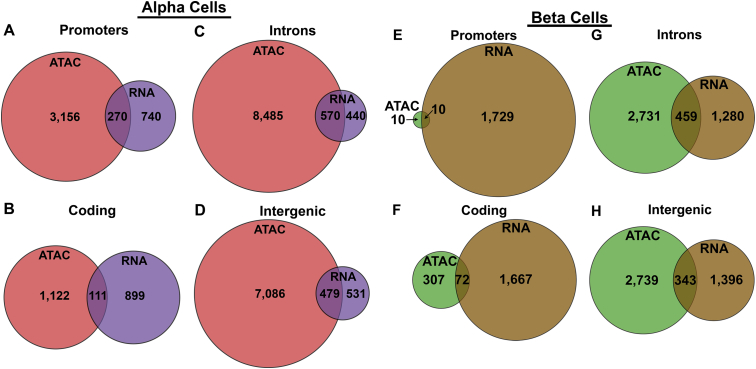
Integration of ATAC-seq data with mRNA-seq data. Venn diagrams showing overlap of ATAC-seq peaks and gene expression based on annotation for (A-D) α-cell-selective genes and (E-H) β-cell-selective genes.
Supplemental Figure 2
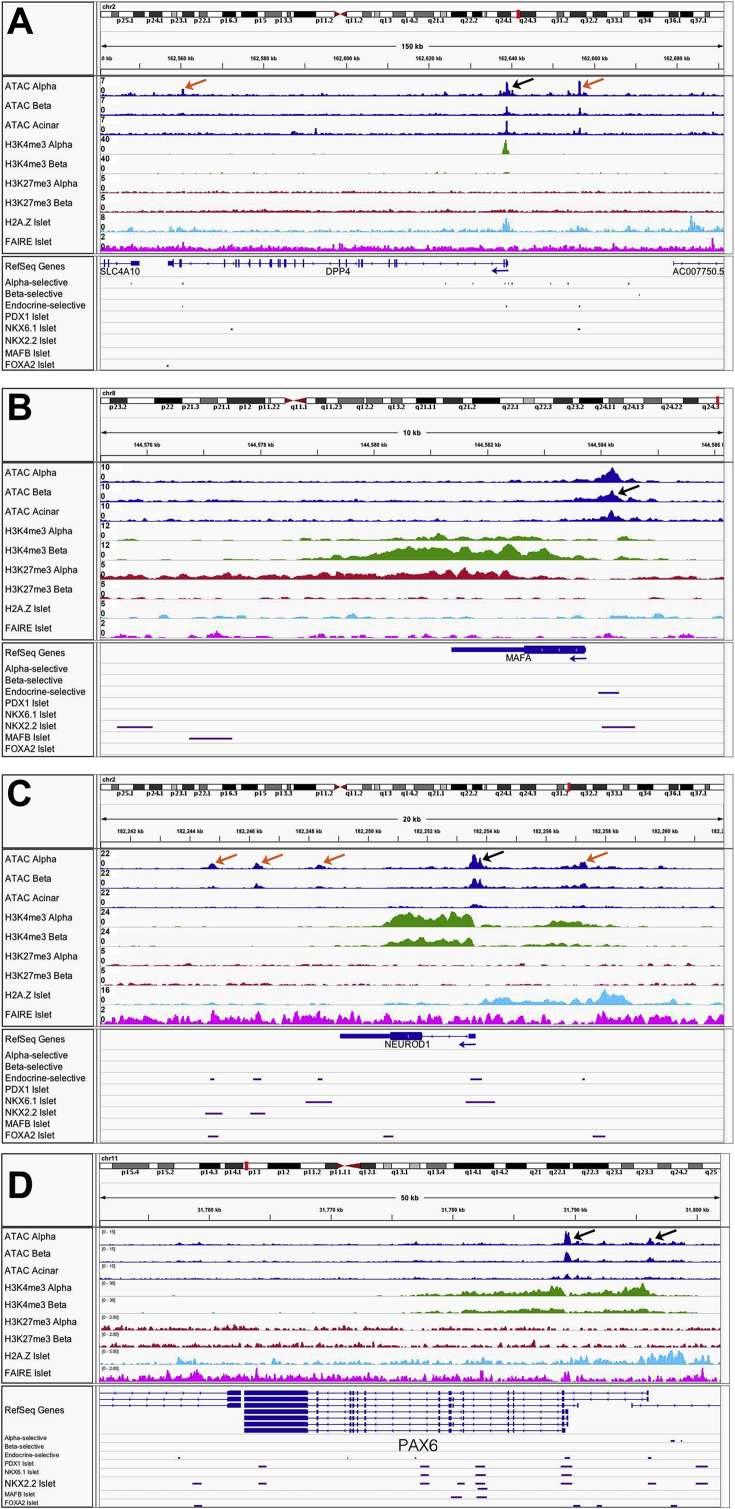
Integration of ATAC-seq data with histone epigenetic ChIP-seq, and FAIRE-seq data for known α- and β-cell genes. Pooled sequencing tracks for the (A) DPP4, (B) MAFA, (C) NEUROD1 and PAX6 (D) loci from α-cell, β-cell, and acinar cell ATAC-seq data (blue); α-cell and β-cell H3K4me3 (green) and H3K4me27 (red) ChIP-seq data; whole islet H2A.Z ChIP-seq data (light blue); and whole islet FAIRE-seq data (pink). Cell type-selective peaks are identified in blue below RefSeq Genes, followed by islet transcription factor ChIP-seq peaks in purple. Known promoter regions are indicated with black arrows, and putative enhancer regions are indicated with orange arrows.
References
Articles from Molecular Metabolism are provided here courtesy of Elsevier
Citations & impact
Impact metrics
Citations of article over time
Alternative metrics
Article citations
ATAC-seq for Characterizing Host and Pathogen Genome Accessibility During Virus Infection.
Methods Mol Biol, 2866:111-122, 01 Jan 2025
Cited by: 0 articles | PMID: 39546200
LncRNA <i>Snhg3</i> aggravates hepatic steatosis via PPARγ signaling.
Elife, 13:RP96988, 22 Oct 2024
Cited by: 0 articles | PMID: 39436790 | PMCID: PMC11495842
RUNX1 interacts with lncRNA SMANTIS to regulate monocytic cell functions.
Commun Biol, 7(1):1131, 13 Sep 2024
Cited by: 0 articles | PMID: 39271940 | PMCID: PMC11399395
Ca2+ signaling and metabolic stress-induced pancreatic β-cell failure.
Front Endocrinol (Lausanne), 15:1412411, 02 Jul 2024
Cited by: 0 articles | PMID: 39015185 | PMCID: PMC11250477
Review Free full text in Europe PMC
The chromatin accessibility and transcriptomic landscape of the aging mice cochlea and the identification of potential functional super-enhancers in age-related hearing loss.
Clin Epigenetics, 16(1):86, 04 Jul 2024
Cited by: 0 articles | PMID: 38965562 | PMCID: PMC11225416
Go to all (161) article citations
Data
Data behind the article
This data has been text mined from the article, or deposited into data resources.
BioStudies: supplemental material and supporting data
GEO - Gene Expression Omnibus
- (1 citation) GEO - GSE76268
SNPs (Showing 15 of 15)
- (3 citations) dbSNP - rs7732130
- (1 citation) dbSNP - rs1169288
- (1 citation) dbSNP - rs1800574
- (1 citation) dbSNP - rs7903146
- (1 citation) dbSNP - rs11708067
- (1 citation) dbSNP - rs10811660
- (1 citation) dbSNP - rs10517086
- (1 citation) dbSNP - rs17066842
- (1 citation) dbSNP - rs10757283
- (1 citation) dbSNP - rs2237895
- (1 citation) dbSNP - rs5215
- (1 citation) dbSNP - rs13266634
- (1 citation) dbSNP - rs458069
- (1 citation) dbSNP - rs11755527
- (1 citation) dbSNP - rs74046911
Show less
Similar Articles
To arrive at the top five similar articles we use a word-weighted algorithm to compare words from the Title and Abstract of each citation.
ATAC-seq reveals alterations in open chromatin in pancreatic islets from subjects with type 2 diabetes.
Sci Rep, 9(1):7785, 23 May 2019
Cited by: 34 articles | PMID: 31123324 | PMCID: PMC6533306
Chromatin accessibility differences between alpha, beta, and delta cells identifies common and cell type-specific enhancers.
BMC Genomics, 24(1):202, 17 Apr 2023
Cited by: 5 articles | PMID: 37069576 | PMCID: PMC10108528
Genome-wide profiling of histone H3K27 acetylation featured fatty acid signalling in pancreatic beta cells in diet-induced obesity in mice.
Diabetologia, 61(12):2608-2620, 03 Oct 2018
Cited by: 18 articles | PMID: 30284014
[Advances in assay for transposase-accessible chromatin with high-throughput sequencing].
Yi Chuan, 42(4):333-346, 01 Apr 2020
Cited by: 2 articles | PMID: 32312702
Review
Funding
Funders who supported this work.
Juvenile Diabetes Research Foundation (1)
Grant ID: 3-PDF-2014-186-A-N
NIDDK (1)
Grant ID: UC4DK104119
NIDDK NIH HHS (2)
Grant ID: UC4 DK104119
Grant ID: P30 DK019525