Abstract
Free full text

Distinct neural mechanisms for spatially lateralized and spatially global visual working memory representations
Abstract
Visual working memory (VWM) allows humans to actively maintain a limited amount of information. Whereas previous electrophysiological studies have found that lateralized event-related potentials (ERPs) track the maintenance of information in VWM, recent imaging experiments have shown that spatially global representations can be read out using the activity across the visual cortex. The goal of the present study was to determine whether both lateralized and spatially global electrophysiological signatures coexist. We first show that it is possible to simultaneously measure lateralized ERPs that track the number of items held in VWM from one visual hemfield and parietooccipital α (8–12 Hz) power over both hemispheres indexing spatially global VWM representations. Next, we replicated our findings and went on to show that this bilateral parietooccipital α power as well as the contralaterally biased ERP correlate of VWM carries a signal that can be used to decode the identity of the representations stored in VWM. Our findings not only unify observations across electrophysiology and imaging techniques but also suggest that ERPs and α-band oscillations index different neural mechanisms that map on to lateralized and spatially global representations, respectively.
NEW & NOTEWORTHY
Our work shows that there exist lateralized and spatially global visual working memory (VWM) representations concurrently in mind and that VWM representations are supported by dissociable electrophysiological correlates measured by human scalp electroencephalograms. Our work not only bridges the gap between recent functional magnetic resonance imaging studies and more traditional electrophysiological event-related potential studies of VWM but also provides novel insight into the organization of VWM representations.
visual working memory (VWM) allows us to store a limited amount of information that we use to reason, solve problems, and have a coherent experience across interruptions in visual input (Fukuda et al. 2010; Unsworth et al. 2014a). Previous studies have shown that storing information in VWM results in lateralized electrophysiological activity. In contrast, functional magnetic resonance imaging (fMRI) studies have shown that nonlateralized VWM activity dominates during the retention interval of short-term memory tasks. That is, objects presented in one visual hemifield (e.g., left of fixation) elicit a sustained pattern of activity across multiple areas in the visual cortex (Ester et al. 2009; Harrison and Tong 2009; Pratte and Tong 2014; Serences et al. 2009). Our goal here was to determine if we could find an electrophysiological counterpart of this spatially global signal coexisting with the already established lateralized event-related potentials (ERPs).
By recording subjects' ERPs, Vogel and colleagues (2004) demonstrated the existence of an electrophysiological correlate of VWM maintenance called the contralateral delay activity (CDA). In their experiments, subjects were first directed by a central arrow cue to remember objects presented in either the left or right hemifield. Next, a bilateral array of colored squares was presented. Subjects remembered as many colored squares as possible from the cued side. After a 1-s retention interval, subjects reported whether or not the colors in the test array matched those from the initial memory array. During the retention interval, parietooccipital channels contralateral to the task-relevant hemifield showed a sustained negativity compared with ipsilateral channels. This CDA was further linked to VWM storage because it increased in amplitude up to individual subject's VWM capacity, plateauing across set sizes beyond one's capacity.
In contrast to these electrophysiological experiments that focused on lateralized activity, recent fMRI studies have indicated that VWM may maintain spatially global representations (Ester et al. 2009; Harrison and Tong 2009; Pratte and Tong 2014). Ester and colleagues (2009) used multivoxel patterns (MVPs) of BOLD responses to decode the content of the visual cortex (i.e., areas V1-V4) during the retention interval of a VWM task. The representations in VWM were reliably decoded from the contralateral visual cortex. Surprisingly, Ester and colleagues (2009) also reliably decoded the contents of VWM from ipsilateral MVPs. This indicates that ipsilateral brain areas participate in representing information in VWM, thus suggesting the existence of spatially global VWM representations in the brain.
Is it the case that electrophysiological and imaging techniques yield truly incompatible results regarding the nature of VWM representations? Or is it possible to simultaneously measure electrophysiological activity indexing spatially specific (i.e., lateralized) and spatially global representations in VWM? In experiments 1 and 2, we tested the hypothesis that the CDA of the subjects' ERPs provides a metric of spatially specific VWM, while the simultaneously measured bilateral α activity of the electroencephalogram (EEG) provides a metric of spatially global VWM. In addition, we show in experiment 3 that both lateralized ERPs and spatially global oscillations can be used to decode the content of VWM, which verified their roles as neural correlates of VWM.
MATERIALS AND METHODS
Subjects
After obtaining informed written consent for procedures approved by the Vanderbilt University Institutional Review Board, we ran subjects with normal or corrected-to-normal vision in experiments 1 (n = 20), 2 (n = 20), and 3 (n = 24; Fig. 1). They were compensated $10/h for their participation. In experiment 1 (12 men and 8 women), 3 additional subjects' data were excluded from analyses due to an excessive number of trials contaminated by ocular artifacts (>30% of trials in any condition). Similarly, in experiment 2 (10 men and 10 women), 3 additional subjects' data were excluded. In experiment 3 (13 men and 11 women), 1 additional subject's data were excluded.
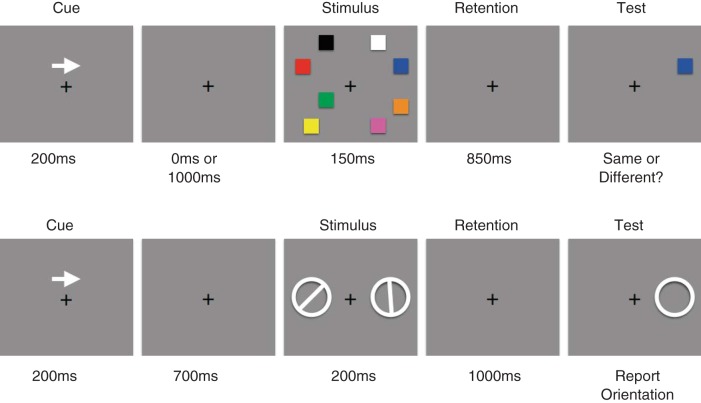
Procedures of the bilateral visual working memory (VWM) tasks used in the present study. The top image shows the color change-detection task used in experiment 1 (and in experiment 2, with the number of objects in the distractor array manipulated). The bottom image shows the orientation recall task used in experiment 3.
Procedures
Experiment 1.
Subjects performed a bilateral change-detection task based on that used in Vogel and Machizawa (2004). Subjects were instructed to maintain fixation on a white central dot (0.2° in visual angle, x = 0.293, y = 0.323, 38.5 cd/m2) presented on a gray background (x = 0.294, y = 0.322, 15.7 cd/m2) throughout each trial of the experiment. After subjects initiated each trial with a button press, a central arrow cue was presented for 200 ms. After the arrow cue offset, a cue-to-stimulus interval followed, during which the screen was blank other than the fixation point. This stimulus-onset asynchrony (SOA) was either 200 ms (short SOA) or 1,000 ms (long SOA), randomly chosen with equal probability. We used these different SOAs to disentangle the activity related to selecting the task-relevant hemifield (i.e., shifts of spatial attention) from that related to actually maintaining information in VWM, as we will discuss below in results. The next event on each trial was the presentation of a bilateral stimulus array consisting of one, two, four, or eight colored squares in each hemifield for 150 ms. Each set size was presented with equal probability, and the different set sizes were randomly interleaved across trials. Each square subtended 0.7 × 0.7° of visual angle, and the color was chosen from a set of nine highly discriminable colors [red (x = 0.592, y = 0.367, 9.60 cd/m2), green (x = 0.299, y = 0.579, 27.6 cd/m2), blue (x = 0.15, y = 0.08, 4.35 cd/m2), yellow (x = 0.396, y = 0.509, 35.5 cd/m2), magenta (x = 0.295, y = 0.171, 13.3 cd/m2), cyan (x = 0.219, y = 0.315, 31.2 cd/m2), orange (x = 0.483, y = 0.447, 18.6 cd/m2), black (x = 0.393, y = 0.423, 0.31 cd/m2), and white (x = 0.293, y = 0.323, 38.5 cd/m2)] without repetition. The selected squares were distributed in a left and right rectangular area subtending 4.8 (horizontal) × 10.4 (vertical)° of visual angle whose center was 4.5° away from the central fixation. After a retention interval of 850 ms, during which the screen was blank other than the fixation point, a single test square was presented on the cued side. The subject indicated by a button press if the test square was the same color as the stimulus presented at the same location a moment ago. Subjects completed 200 trials at each set size and SOA combination.
Experiment 2.
Subjects performed the same basic bilateral change-detection task used in experiment 1 with the following modifications. First, the cue-to-stimulus SOA was fixed at 1,000 ms. Second, the set size in the task-relevant hemifield was one, four, or eight objects. These set sizes allowed us to more efficiently sample VWM loads both below and above the subjects' capacity. Finally, there were three different types of distractor conditions crossed with these three set sizes. In one condition, the number of distractors matched with the number of targets (termed the matched distractor condition because this is the typical procedure for measuring the CDA). In the next condition, there was only one distractor regardless of the number of targets (termed the one-distractor condition). In the last condition, there were always eight distractors regardless of the number of targets (termed the eight-distractor condition). We used these different distractor conditions to determine whether the electrophysiological measures were related to maintaining the different number of target items or ignoring different numbers of distractors. Each distractor condition was presented in a separate block, with the order randomized across subjects. Each subject completed 200 trials in each combination of set size and distractor condition.
Experiment 3.
Subjects in experiment 3 performed a different VWM recall task. Instead of color being the critical feature with the set size varying across trials, subjects had to remember the orientation of one bar presented in the left or right visual field. This change of task allowed us to test the hypothesis that the scalp distribution of bilateral α-band activity as well as that of the CDA could be used to decode the orientation of the bar that subjects were holding in VWM.
After subjects initiated each trial with a button press, a central arrow cue was presented for 200 ms to indicate the task-relevant hemifield for that trial (i.e., left or right). Then, 900 ms after the offset of the arrow cue, one white oriented bar surrounded by a ring (ring radius: 1.6°, bar width: 0.5°) was presented in each hemifield (3.1° horizontal to the central fixation spot) for 200 ms. For each trial, the orientation of the bar was randomly chosen from eight equally spaced seed angles (0, 22.5, 45, 67.5, 90, 112.5, 135, and 157.5°) and presented with a random jitter (range: ±11.25°). After a retention interval of 1,000 ms, one ring was presented on the cued side, and subjects reported the orientation of the bar by clicking where the bar met the ring using a computer mouse. Subjects completed 12 blocks of 128 trials.
EEG Acquisition and Preprocessing
The EEG was recorded using a right-mastoid reference, rereferenced offline to the average of the left and right mastoids. Signals were amplified with a gain of 20,000, a bandpass of 0.01–100 Hz, and digitized at 250 Hz. We used 10–20 electrode sites (Fz, Cz, Pz, F3, F4, C3, C4, P3, P4, PO3, PO4, O1, O2, T3, T4, T5, and T6) and a pair of custom sites, OL (halfway between O1 and OL) and OR (halfway between O2 and OR). Eye movements were monitored using electrodes placed 1 cm lateral to the external canthi for horizontal eye movements [i.e., the horizontal electrooculogram (HEOG)] and an electrode placed beneath the right eye for blinks and vertical eye movements [i.e., the vertical electrooculogram (VEOG)].
For each experiment, the continuous EEG data were first segmented into trial epochs. For experiment 1, the trial epoch was defined as −400 to 1,200 ms after the cue onset for the short SOA condition and −400 to 2,200 ms after the cue onset for the long SOA condition. For experiment 2, the trial epoch was defined as −400 to 2,200 ms after the cue onset. For experiment 3, the trial epoch was defined as −400 to 2,200 ms after the cue onset. Trials accompanied by horizontal eye movements (>30 μV mean threshold across observers) or eye blinks (>75 μV mean threshold across observers) were rejected before further analyses. Subjects' data with >30% of trials rejected for ocular or motor artifacts in any given condition were excluded.
ERP Analyses
To measure ERPs time locked to the event of interest, we averaged EEG responses across trials for each condition. ERPs were baseline corrected using the potential measured from −400-0 ms relative to the time-locking event. In other words, the mean amplitude in the baseline window was subtracted from the entire trial epoch. Based on the previous literature (Fukuda and Vogel 2009; Vogel and Machizawa 2004), we created grand-average contralateral and ipsilateral waveforms by averaging across parietooccipital channels from P3/4, PO3/4, O1/2, OL/R, and T5/6 relative to the task-relevant hemifield. We then created a difference wave by subtracting the ipsilateral average from the contralateral average across the five pairs of posterior, lateralized channels. The mean amplitude from 300–1,000 ms after the memory array onset defined the CDA that we measured on each trial.
For experiment 1, two-way ANOVA with factors of set size (1, 2, 4, and 8) and SOA (short vs. long) was run on the CDA data. For experiment 2, separate one-way ANOVAs with a factor of set size (1, 4, and 8) were run for each distractor conditions. This was done because the matched distractor condition had varying number of distractors for each set size, whereas other conditions did not, thus making the distractor condition factor nonorthogonal to the other factor. To better examine the effect of distractors on the CDA, we ran an additional two-way ANOVA (with the factors of set size and distractor condition) excluding matched distractor conditions.
EEG Analyses
To examine the oscillatory responses, the EEG from each trial was subjected to spectral decomposition with a fixed window size of 400 ms and a window overlap of 380 ms with a MATLAB function (spectrogram.m). Next, we measured the contralateral α power suppression using the following analysis steps. First, the baseline power spectrum was defined as the mean power spectrum observed in the precue time window (−400 to 0 ms relative to the cue onset). This baseline spectrum was subtracted from the entire epoch, and the resultant spectral difference was divided by the baseline spectrum and then multiplied by 100. This allowed us to calculate the percent change in power at each frequency.
We created the contralateral and ipsilateral averaged α power (8–12 Hz) by averaging the same set of the parietooccipital channels as the CDA analysis (i.e., P3/4, PO3/4, O1/2, OL/R, and T5/6). We then created a difference measure by subtracting the ipsilateral average from the contralateral average. This allowed us examine if there is a set size-dependent contralateral bias to the α power suppression.
To test the hypothesis that α power suppression is involved in representing information in VWM in a spatially global manner, we also examined contralateral and ipsilateral α power separately. Because we were interested in the oscillatory responses to the onset of the stimulus, we used the prestimulus time window (−400 to 0 ms relative to the stimulus onset) as the baseline to calculate α power responses for each channel. The same set of parietooccipital channels was then averaged to create contralateral and ipsilateral α power measures separately.
To test the bilateral α power suppression for experiment 1, three-way ANOVA with factors of set size (1, 2, 4, and 8), SOA (short vs. long), and laterality (contralateral vs. ipsilateral) was run. For experiment 2, separate two-way ANOVAs with a factor of set size (1, 4, and 8) and laterality (contralateral vs. ipsilateral) were run for each distractor condition. This was done because the matched distractor condition had varying number of distractors for each set size, whereas other conditions did not, thus making the distractor factor nonorthogonal to the set size factor. To better examine the effect of distractors on the bilateral α power suppression, we ran an additional thee-way ANOVA (with the factors of set size, distractor, and laterality) excluding matched distractor conditions.
Decoding the Contents of VWM
In experiment 3, we determined if it was possible to reliably decode the content of VWM from the scalp distribution of both lateralized ERPs and EEG oscillations. First, we divided the entire experimental session into six temporally defined epochs. Specifically, each epoch consisted of two consecutive experimental blocks with the first epoch being the blocks 1 and 2, the second epoch being blocks 3 and 4, and so forth. For the oscillatory signals, the power spectrum for each seed angle (0, 22.5, 45, 67.5, 90, 112.5, 135, and 157.5°) was averaged (average number of trials for each seed angle: 14) for each epoch. Using these averaged power spectra across channels as inputs, we trained separate linear classifiers (linear discriminant analysis) for contralateral and ipsilateral channels. We did this for each frequency across each time window from −200 to 1,000 ms relative to the stimulus onset1 with a hold-one-out procedure. More precisely, the classifier was fed a set of power responses for a given frequency across contralateral (or ipsilateral) parietooccipital channels (i.e., P3/4 PO3/4, O1/2, OL/R, and T5/6) observed in a given time window for each seed angle. The classifier was then trained using the data from five averaged epochs before determining whether it could then classify the averaged data from the remaining epoch. This routine was repeated so that each epoch served as the test data. Once completed, we then moved on to a different frequency to cover the entire frequency range of interest (i.e., 2–30 Hz). We then moved to a different time window and repeated the whole procedure. This analysis sequence provided a time course of classification accuracy for contralateral and ipsilateral power at each frequency for each subject. As a control analysis, we also applied the same procedure to decode the content of the distractor orientation.
For lateralized ERPs, we first calculated average amplitudes with the same sliding time windows as EEG data (i.e., a 400-ms window with an overlap of 380 ms) for each parietooccipital channel (i.e., P3/4, PO3/4, O1/2, OL/R, and T5/6). This was done to equate the temporal resolution of ERP-based and oscillation-based classifications for the purpose of direct comparison. We then created difference channels for each parietooccipital channel pair by subtracting the amplitude of the ipsilateral channel from its contralateral counterpart. The resultant five difference channels were subjected to the same analytic sequence as the oscillatory signals.
To address the potential alternative explanation that our decoding results were contaminated by small but systematic eye movements, we applied the same decoding analysis to the EOG channels (i.e., HEOG and VEOG channels). We first calculated average amplitudes for the same sliding time windows as EEG data (i.e., 400-ms window with an overlap of 380 ms) for each EOG channel. For each time window, we then trained the classifier using the set of EOG channels from five epochs and tested its accuracy on the remaining epoch. This procedure was repeated so that each epoch served as the test data.
Verifying the Spatially Global Nature of Posterior α Power Suppression
If the decoding ability of the scalp distribution of contralateral and ipsilateral α power in fact reflects the existence of spatially global VWM representations, then we should expect that contralateral and ipsilateral decoding performances are temporally synchronized. We tested by examining whether both contralateral and ipsilateral α power decoders output a correct response on the same trials. We compared against the assumption that contralateral and ipsilateral decoders predict the response based on independent evidence.
Regression Analysis for Decoding Performance
To understand the relationship between lateralized and spatially global VWM representations, we examined the correlational structure among decoding accuracies based on different neural correlates of VWM. These correlates included the scalp distributions of contralateral and ipsilateral parietooccipital α (8–12 Hz) and θ (4–7 Hz) power responses, scalp distribution of the lateralized (i.e., contralateral − ipsilateral) visual ERPs (e.g., the N1), and CDA distributions. In doing so, we found a univariate outlier based on θ-based decoding measures (shown as the open circle in Fig. 9), and, thus, the statistical results are reported excluding this data point.
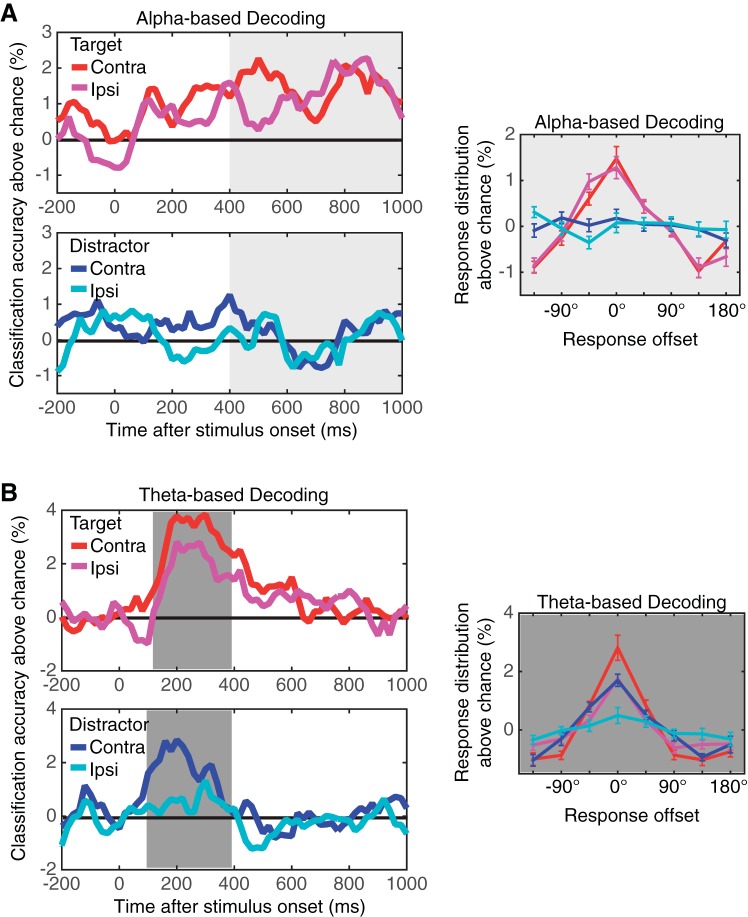
Oscillation-based classification results from experiment 3. A: results of the classification analyses based on the scalp distribution of α (8–12 Hz) power. The top left panel shows the classification accuracy for target item using the scalp distribution of the contralateral (red) and ipsilateral (magenta) α power. The bottom left panel shows the classification accuracy for distractor item based on contralateral (blue) and ipsilateral (cyan) α power distribution. Note that the laterality was defined with respect to the item of interest. The solid black line indicates the chance level of classification. The line graph on the right shows the distribution of response offsets produced by the α-power-based decoders. The red (contralateral) and magenta (ipsilateral) lines show the result for target decoding, and the blue (contralateral) and cyan (ipsilateral) lines show that for distractor decoding. Error bars represent SEs. B: results of the classification analyses based on the scalp distribution of θ (4–7 Hz) power. The top left panel shows the classification accuracy for target item using the scalp distribution of the contralateral (red) and ipsilateral (magenta) θ power. The bottom left panel shows the classification accuracy for distractor item based on contralateral (blue) and ipsilateral (cyan) θ power distribution. Note that the laterality was defined with respect to the item of interest. The solid black line indicates the chance level of classification. The line graph on the right shows the distribution of response offsets produced by the θ-power-based decoders. The red (contralateral) and magenta (ipsilateral) lines show the result for target decoding, and the blue (contralateral) and cyan (ipsilateral) lines show that for distractor decoding. Error bars represent SEs.
RESULTS
Experiment 1
Behavioral results.
Behavioral performance at each set size was transformed into Cowan's K using the following formula: K = set size × (hit rate − false alarm rate) separately for the short and long SOAs (Cowan 2001). This allowed us to estimate the number of task-relevant colored squares represented in VWM at each set size. When the SOA between the cue and memory array was short, the mean K estimate was 0.9 (SE: 0.01), 1.7 (SE: 0.04), 2.1 (SE: 0.12), and 2.2 (SE: 0.17), for set sizes 1, 2, 4, and 8, respectively. For the long SOA conditions, the mean K estimate was 0.9 (SE: 0.02), 1.7 (SE: 0.04), 2.3 (SE: 0.12), and 1.8 (SE: 0.13) for set sizes 1, 2, 4, and 8, respectively. Repeated-measures ANOVA showed that there was a main effect of set size due to the K estimate monotonically increasing up to set size 4, with no further increase for set size 8 [F(1,19) = 26.9, P < 0.001 for linear effect; F(1,19) = 49.0, P < 0.001 for quadratic effect]. Planned comparisons further supported the observation that K only increased up to set size 4 [t(19) = 9.6, P < 0.001 for set size 1 vs. 4; t(19) = 3.6, P < 0.001 for set size 2 vs. 4; t(19) = 0.6, not significant (NS) for set size 4 vs. 8 in short SOA conditions; t(19) = 11.5, P < 0.001 for set size 1 vs. 4; t(19) = 4.1, P < 0.01 for set size 2 vs. 4; and t(19) = −3.7, P < 0.001 for set size 4 vs. 8 in long SOA conditions, showing a significantly smaller K estimate for set size 8 than 4]. There was no main effect of SOA [F(1,19) = 0.7, NS], meaning that the participants were just as able to selectively remember the target items with both short and long SOAs. These results are in line with previously reported results using bilateral change-detection tasks.
CDA results.
Figure 2 shows the difference waves between the contralateral and ipsilateral parietoocciptical channels. The contralateral delay activity emerged 400 ms after the onset of the stimulus. The CDA monotonically increased up to set size 4 and reached a plateau for both short and long SOAs, revealing the classical capacity-defined set size function. Repeated measure ANOVA supported this observation with a significant effect of set size [F(1,19) = 20.5, P < 0.001 for linear effect; F(1,19) = 10.3, P < 0.001 for quadratic effect]. Planned pairwise comparisons supported this observation [t(19) = 4.8, P < 0.001 for set size 1 vs. 4; t(19) = 4.2, P < 0.001 for set size 2 vs. 4; t(19) = −2.7, P = 0.01, for set size 4 vs. 8 in short SOA conditions, and the CDA was smaller for set size 8 than set size 4; t(19) = 6.7, P < 0.001 for set size 1 vs. 4; t(19) = 4.1, P < 0.001 for set size 2 vs. 4; t(19) = 0.6, NS for set size 4 vs. 8 in long SOA conditions]. There also was a main effect of SOA [F(1,19) = 6.5, P < 0.05], but, critically, there was no interaction between SOA and set size [F(1,19) = 0.1, NS]. This suggested that although SOA influenced the overall amplitude of the CDA, it did not change the set size effect of the CDA. Thus, we observed the expected pattern of ERPs during this task in which spatially specific ERPs are measured contralateral to the remembered items. Next, we turned to the question of whether the oscillatory activity of the EEG provides a measure of the spatially global representations that fMRI experiments suggest may exist.
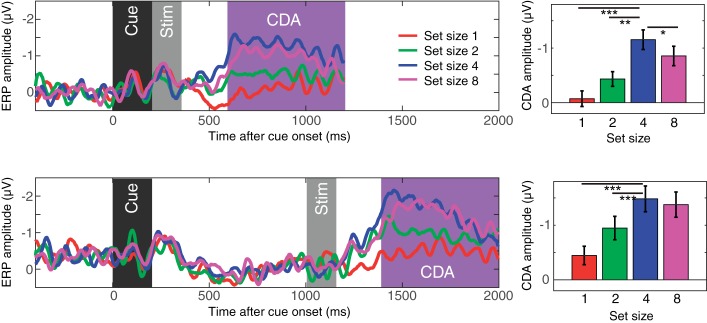
Contralateral delay activity (CDA) results from experiment 1. The top panel shows the results from the short stimulus-onset asynchrony (SOA) condition, and the bottom panel shows the results from the long SOA condition. The waveforms are the difference waves for each set size, and the time windows for the stimulus events [dark gray region for the cue; light gray region for the memory array (Stim)] and the CDA (magenta region) are highlighted. The bar graphs show mean CDA amplitudes for each set size. Error bars on the bar graphs represent SEs. Asterisks represent the results of planned t-tests (where * is <0.05, ** is <0.01, and *** is <0.001).
Bilateral oscillations exhibit a set size function mirroring behavior.
To determine whether spatially global VWM representations can be measured electrophysiologically, we analyzed frequency band oscillations from electrodes that were contralateral and ipsilateral to the remembered objects. We found that the α-band activity (8–12 Hz) was suppressed bilaterally. Moreover, as expected from a measure of VWM maintenance, this α suppression showed a set size function that changed in parallel with behavioral performance in the task. Figure 3 shows the event-related desynchronization for contralateral and ipsilateral parietooccipital channels separately. As can be seen, the capacity-limited set size function was observed across both contralateral and ipsilateral electrodes. That is, the event-related desynchronization of α monotonically increased up to set size 4 and reached the plateau for both sides in both SOA conditions. Repeated measures ANOVAs confirmed the presence of a significant effect of set size [F(1,19) = 15.3, P = 0.001 for linear effect; F(1,19) = 5.6, P = 0.029 for quadratic effect]. Planned pairwise comparisons supported this observation [ts(19) > 2.6, Ps < 0.02 for set size 1 vs. 4; ts(19) > 2.5, Ps < 0.02 for set size 2 vs. 4; ts(19) < 1.2, NS for set size 4 vs. 8 for short SOA conditions; ts(19) > 3.1, Ps < 0.001 for set size 1 vs. 4; ts(19) > 2.4, Ps < 0.02 for set size 2 vs. 4; and ts(19) < 0.6, NS for set size 4 vs. 8 for long SOA conditions]. Critically, there was no main effect of laterality [F(1,19) = 3.0, NS] or interaction between set size and laterality [F(1, 19) = 1.2, NS]. There was a main effect of SOA [F(1,19) = 19.5, P < 0.001], but this appears to reflect the neural response to the cue bleeding into the baseline activity for the short SOA conditions. Taken together, these results show that α-band activity has the defining characteristics of an electrophysiological index of spatially global VWM representations.
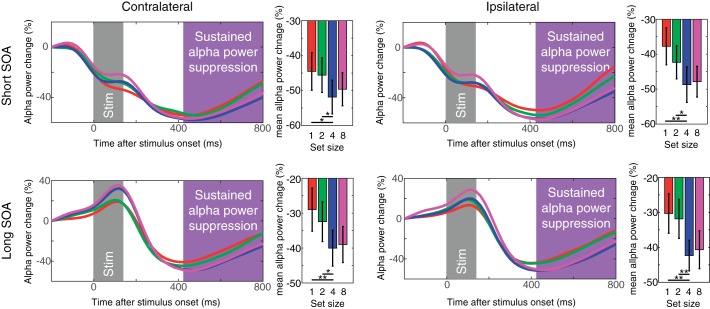
Sustained α power suppression measured in experiment 1. The top panels show the contralateral (left) and ipsilateral (right) α power suppression for short SOA conditions. The bottom panels show the same for long SOA conditions. The time windows for the stimulus onset and sustained α power suppression are highlighted as in Fig. 2 (with the α suppression window shown in magenta). Error bars on bar graphs represent SEs. The asterisks represent the results of planned t-tests (where * is <0.05 and ** is <0.01).
Next, we examined if the set size effect of parietooccipital α power suppression exhibited contralateral bias during VWM maintenance. Figure 4 shows the difference waves between contralateral and ipsilateral parietooccipital α power suppression. Repeated-measures ANOVA revealed that there was no main effect of set size [Fs(1,19) < 2.5, NS]. This is in stark contrast with the CDA that shows a lateralized distribution and, thus, further supports the idea that this parietooccipital α power suppression is a marker of spatially global VWM representations. In addition, the results shown in Fig. 4B demonstrate that the lateralized desynchronization of α was most evident before the stimulus onset (long SOA trials) and test item onset (short and long SOA trials). This suggests that the lateralized desynchronization of α indexes the orienting of attention in expectation of the upcoming event (Handel et al. 2011; Haegens et al. 2012; Van Dijk et al. 2008l; Whitmarsh et al. 2014) rather than the maintenance of VWM representations.
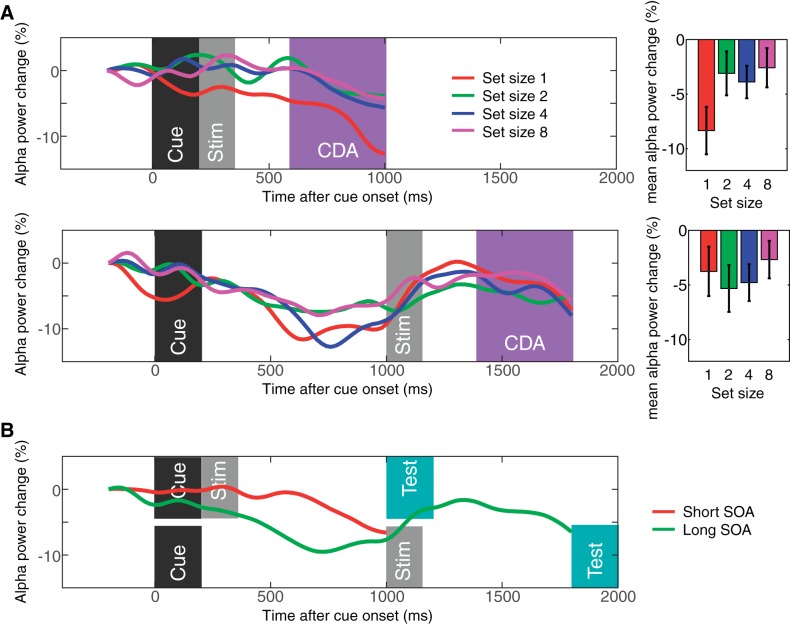
The contralateral α power suppression measured in experiment 1. A: results from the short SOA condition (top) and long SOA condition (bottom). The waveforms are the difference waves for each set size, and the time windows for the stimulus events and CDA are highlighted as in Fig. 2. Note that the time points along the x-axis indicate the center of the 400-ms time window. The bar graphs show the mean α power suppression during the CDA time window for each set size. B: time course of the contralateral α power suppression for short and long SOA conditions averaged across all set sizes. Error bars represent SEs.
Experiment 2
An alternative explanation for the ipsilateral desynchronization of α activity (8–12 Hz) that we observed in experiment 1 is that it indexes the suppression of the task-irrelevant items in the uncued hemifield (Sauseng et al. 2009). That is, instead of the bilateral α desynchronization being due to spatially global representations maintained in VWM, it is possible that ipsilateral desynchronizations show the characteristic set size function because the number of items in the task-irrelevant hemifield increased as the task-relevant set size increased. To dissociate the number of task-irrelevant objects from the number of task-relevant objects we manipulated the number of distractors presented independently of the number of task-relevant objects in the cued hemifield. If ipsilateral desynchronization of α is due to spatially global VWM representations, then we should see the capacity-defined set size function on ipsilateral channels regardless of the number of distractors. In contrast, if ipsilateral desynchronization of α is due to the suppression of distractors, then our distractor manipulation should destroy the ipsilateral set size function.
Behavioral results.
Similar to experiment 1, behavioral performance was first converted to Cowan's K for each set size across the three different distractor conditions. In the matched distractor condition, the mean K estimates were 0.92 (SE: 0.01), 1.97 (SE: 0.14), and 1.68 (SE: 0.20) for set size 1, 4, and 8, respectively. In the one-distractor condition, the mean K estimates were 0.92 (SE: 0.01), 2.14 (SE: 0.15), and 2.03 (SE: 0.23) for set size 1, 4, and 8, respectively. In the eight-distractor condition, the mean K estimates were 0.92 (SE: 0.01), 2.05 (SE: 0.16), and 1.86 (SE: 0.19) for set size 1, 4, and 8, respectively. That is, the K estimate reached a plateau at set size 4 in all distractor conditions. Repeated-measures ANOVA for each distractor condition confirmed the main effects of set size [Fs(1,19) > 14.7, P < 0.005 for linear effect; Fs(1,19) > 25.0, P < 0.001 for quadratic effect]. Planned pairwise comparisons supported these observations [ts(19) > 8.0, P < 0.0001 for set size 1 vs. 4; ts(19) < 1.2, NS for set size 4 vs. 8 for one- and eight-distractor conditions and t(19) = 2.3, P < 0.05 for matched distractor condition showing higher K for set size 4 than 8].
CDA analysis.
Figure 5 shows the difference waves (contralateral − ipsilateral parietooccipital responses) for each distractor condition. As can be seen, the CDA showed the capacity-defined set size functions across all distractor conditions. That is, the CDA reached asymptote at set size 4 in all distractor conditions. When the mean CDA amplitudes were calculated as the mean amplitude from 400-1,000 ms after the stimulus onset and entered into separate repeated-measures ANOVA for each distractor condition, we found a significant main effects of set size [Fs(1,19) > 22.0, P < 0.005 for linear effect; Fs(1,19) > 12.9, P < 0.005 for quadratic effect]. Planned pairwise comparisons supported the observation that the CDA increased from set size 1 to 4 but not from set size 4 to 8 [ts(19) > 4.8, P < 0.001 for set size 1 vs. 4; ts(19) < 1.9, NS for set size 4 vs. 8]. To better examine the effect of distractors on the CDA, we ran an additional two-way ANOVA (factors of set size and distractor condition) excluding the matched distractor condition. This revealed a significant main effect of set size [F(2, 38) = 32.9, P < 0.001] as well as distractor condition [F(1, 19) = 16.5, P < 0.01]. Critically however, these two factors did not interact [F(2, 38) = 0.4, NS]. This suggests that our distractor load manipulation did not affect the nature of the capacity-defined set size function of the CDA.

CDA and contralateral α power suppression measured in experiment 2. Shown are the CDA results for matched distractor (top), one-distractor (middle), and eight-distractor (bottom) conditions. The waveforms are the difference waves for each set size, and the time windows for the stimulus event and CDA are highlighted accordingly. The bar graphs show mean CDA amplitudes during the highlighted window for each set size. Error bars on bar graphs represent SEs. The asterisks represent the results of planned t-tests (where *** is <0.001).
The spatially global α power suppression shows the capacity-defined set size effect irrespective of the number of distractors.
We examined the sustained α power suppression (8–12 Hz) to determine if it shows the capacity-defined set size effect on both contralateral and ipsilateral electrodes regardless of the number of the distractors. This should be the case if the spatially global α power suppression truly indexes the spatially global VWM representations. As shown in Fig. 6, the sustained α power suppression reached an asymptote at set size 4 across hemispheres in all distractor conditions. Repeated-measures ANOVAs statistically confirmed this observation [Fs(1,19)>5.6, Ps < 0.05 for linear effect; Fs(1,19) > 7.5, Ps < 0.03 for quadratic effects] as well as planned pairwise comparisons [ts(19) > 2.56, Ps < 0.02 for set size 1 vs. 4; ts(19) < 1.5, NS for set size 4 vs. 8 except for ipsilateral channels in matched distractor condition; t(19) = 2.3, P < 0.05 showing smaller α power suppression for set size 8 than 4]. Critically, there was no interaction between set size and laterality [Fs(1,19) < 1, NS for linear effect; Fs(1,19) < 3.4, NS for quadratic effect]. To better examine the effect of distractors on the bilateral α power suppression, we ran an additional three-way ANOVA (with the factors of set size, distractor condition, and laterality) excluding the matched distractor condition. The analysis revealed a main effect of set size [F(2,38) = 10.4, P < 0.001] and laterality [F(1,19) = 4.8, P < 0.05] but not distractor condition [F(1,19) = 1.5, NS]. Once again, there was no interaction across three factors (Fs < 1, NS). This suggests that our distractor load manipulation did not affect the nature of the capacity-defined set size function of the spatially global α power suppression.
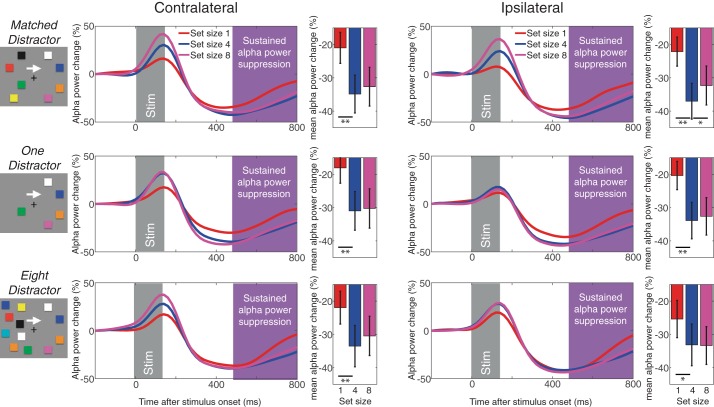
Sustained α power suppression measured in experiment 2. The top panels shows the contralateral (left) and ipsilateral (right) α power suppression for matched distractor conditions. The middle panels show the same for one-distractor condition, and the bottom panels do for the eight-distractor condition. The time windows for the stimulus onset and sustained α power suppression are highlighted accordingly. The bar graphs show the mean α power for each set size. Error bars on the bar graphs represent SEs. The asterisks represent the results of planned t-tests (where * is <0.05 and ** is <0.01).
The lateralized α power suppression indexes orienting of attention.
Similar to experiment 1, we analyzed the lateralized α power suppression. In all distractor conditions, mean lateralized α power suppression amplitudes during the CDA interval (mean α power difference from 400–800 ms after the stimulus onset) did not show the capacity-defined set size function [Fs(1,19)< 1.6, NS for linear effect; Fs(1,19) < 2.2, NS for quadratic effect except for the eight-distractor condition; F(1,19) = 7.7, P < 0.05]. Figure 7 shows the lateralized α power suppression for each distractor condition. As can be seen, the lateralized α power suppression was most evident before the onset of the stimulus and test item in all distractor conditions. These results replicated the results in experiment 1 and further support the interpretation that the lateralized α power suppression indexes the orienting of attention in expectation of upcoming events, not the active maintenance of representations in VWM.
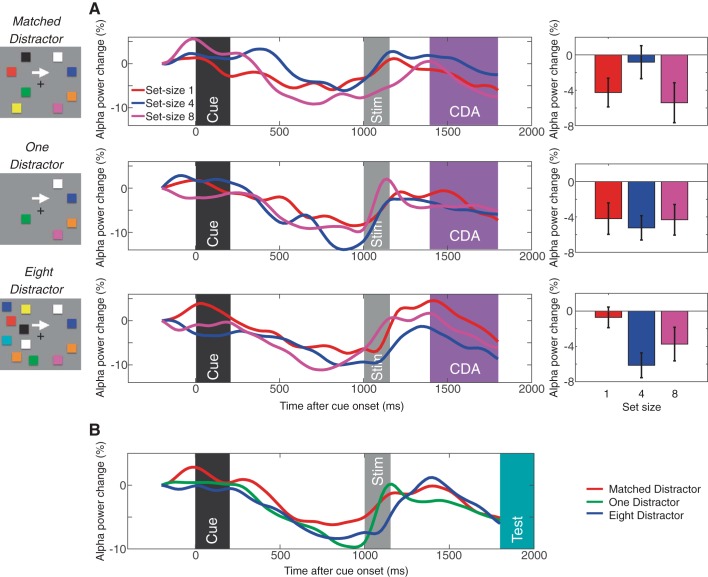
Contralateral α power suppression measured in experiment 2. A: lateralized α power suppression for matched distractor (top), one-distractor (middle), and eight-distractor (bottom) conditions. The waveforms are the difference waves (contralateral − ipsilateral) for each set size, and the time windows for the stimulus event and CDA are highlighted accordingly. The bar graphs show the mean lateralized α power suppression during the CDA window for each set size. B: time course of the contralateral α power suppression for matched, one-distractor, and eight-distractor conditions averaged across all set sizes. The events and time windows are highlighted as in A (with the addition of the test stimulus presentation shown in turquoise). Error bars on the bar graphs represent SEs.
Experiment 3
The goal of experiment 3 was to determine if the contents of VWM can be decoded from the distribution of both lateralized ERPs (i.e., the early sensory components and the CDA) and the spatially global oscillatory correlate of VWM (i.e., α power suppression). If these neural correlates truly index lateralized and spatially global VWM representations maintained in the brain, then it should be possible to use the scalp distribution of each signal during the retention interval to read out the contents of VWM using decoding analyses.
Behavioral results.
We examined the precision of subjects' behavioral report of the remembered orientation. Our dependent measure was the mean of the magnitude of response errors (i.e., the response offset in degrees from the orientation shown in the memory sample). The mean magnitude of the response offset was 5.6° (SE: 0.25). This indicates that subjects were able to maintain the target orientation with high precision.
Decoding the contents of VWM with lateralized ERPs.
Figure 8 shows the time course of the classification accuracy for VWM content using the scalp distribution of difference waves between channels that were contralateral and ipsilateral to the task-relevant hemifields. As can be seen, the content of VWM was reliably decoded during stimulus encoding and throughout the retention interval, whereas the distractor identity was not. To statistically evaluate the decoding performance, we calculated the mean decoding accuracy in a window from 100–400 ms after the stimulus onset for visual ERPs and 400-1,000 ms for the CDA. A separate repeated-measures ANOVA revealed that there was a main effect of object identity for both visual ERPs [F(1,23) = 18.7, P < 0.001] and the CDA [F(1,23) = 18.5, P < 0.001], showing that the content of VWM was selectively decoded from the scalp distribution of lateralized visual ERPs and the CDA. We then examined the pattern of errors that classifiers made (i.e., classification offsets). As can be seen from the distribution of classification offsets in Fig. 8, the classification performance had a graded profile. This shows that when the classifiers made errors, they tended to choose nearby orientations over more distant ones (Brouwer and Heeger 2009; Ester et al. 2013).

Event-related potential (ERP)-based classification results from experiment 3. The top left and bottom left panels show the classification accuracy for the target (red) and distractor (blue) using the scalp distribution of the difference waves (i.e., contralateral-ipsilateral amplitudes) and electrooculogram channels, respectively. Note that the laterality was defined with respect to the cued hemifield. The solid black line indicates the chance level of classification. The top right and bottom right panels show the distribution of response offsets produced by the visually evoked potential (VEP)-based decoder and CDA-based decoder, respectively. The red lines show the result for target decoding, and the blue lines show that for distractor decoding. Error bars represent SEs.
We also examined if content of VWM can be decoded from small but systematic eye movements (Fig. 8). The results of the decoding analysis using EOG channels (VEOG and HEOG channels) revealed that this was not the case. More precisely, the classification accuracy for the VWM content (i.e., cued item) fluctuated around chance level throughout the retention interval, and it was not significantly above chance in any time window (Ps > 0.05).
Decoding the content of VWM with α oscillations.
Figure 8 shows the time course of the classification accuracy for both contralateral and ipsilateral α power (8–12 Hz). As can be seen, the content of VWM was reliably decoded from both the contralateral and ipsilateral scalp distributions of α power, and this was sustained across the retention interval. Critically, these scalp distributions of α power consistently failed to decode the distractor orientation throughout the retention interval. To provide statistical support for these observations, we calculated mean classification accuracy from 400–1,000 ms after the stimulus onset. Two-way repeated-measures ANOVA revealed a significant main effect of object identity [i.e., target vs. distractor, F(1,23) = 13.5, P < 0.01] but no main effect of laterality [i.e., contralateral vs. ipsilateral, F(1,23) = 0.37, NS] nor interaction between object identity and laterality [F(1,23) = 0.74, NS]. That is, the target orientation was reliably decoded by both contralateral and ipsilateral scalp distributions of α power, but the distractor orientation was not.
As can be seen from the distribution of classification offsets, the classification performance had a graded profile showing that when the classifiers made errors, they tended to choose nearby orientations over more distant ones (Brouwer and Heeger 2009; Ester et al. 2013). Critically, contralateral and ipsilateral scalp distributions of α power were equally good at decoding the content of VWM [F(7,161) = 0.2, NS for the main effect of laterality; F(7,161) = 0.58, NS for interaction between laterality and offset]. These results directly support the hypothesis that bilateral α desychronization provides an index of VWM representations.
Finally, to validate that the VWM decodablity is the result of spatially global VWM representations but not of two independent hemisphere-specific VWM representations, we examined if accurate outputs of contralateral and ipsilateral decoders are temporally synchronized. Here, we found that the probability that both contralateral and ipsilateral decoders output accurate responses was statistically higher than the chance level estimated under the assumption that contralateral and ipsilateral decoders made independent predictions [t(23) = 8.4, P < 0.001]. This buttresses our claim that the bilateral α power signal reflects the existence of spatially global VWM representations.
The decoding analysis also showed a transient response in the θ band (4–7 Hz) power that appears to encode the stimuli. Figure 9 shows the time course of the classification accuracy from this parietooccipital θ activity. As can be seen, the ipsilateral and contralateral distribution of θ power in the time window from 100–400 ms after the stimulus onset reliably classified stimulus identity. Interestingly, distractor orientation was also decodable from the contralateral θ power to distractor side (i.e., ipsilateral to target) [t(23) = 3.0, P < 0.01] but not from ipsilateral θ power. Repeated-measures ANOVA confirmed this observation by showing that there was a main effect of object identity [i.e., target vs. distractor, F(1, 23) = 9.0, P < 0.01] and a main effect of laterality [i.e., contralateral vs. ipsilateral, F(1, 23) = 12.0, P < 0.01] but no interaction [F(1, 23) = 0.9, NS]. The fact that the θ response was transient, however, questions its validity as a neural correlate of storage in VWM because it did not continue through the retention interval. Instead, its transient nature makes it a great candidate for a neural correlate of stimulus encoding, which we will discuss below.
Relationship between lateralized and spatially global VWM representations.
Next, we examined the relationships among the neural measures of VWM. If spatially global VWM representations truly exist, then the quality of contralateral and ipsilateral readout of such representations should be correlated. In other words, we would expect that those who show high contralateral decodability should also show high ipsilateral decodability. As shown in Fig. 10, this was precisely the case. For α-based decoding, those who showed higher contralateral decoding accuracy showed higher ipsilateral decoding accuracy (r = 0.47, P < 0.05). Interestingly, the decoding performance using spatially global oscillatory activity was not related to performance using lateralized activity observed at the same time scale. That is, the CDA-based decoding performance was not correlated with contralateral or ipsilateral α-based decoding performance (rs < 0.16, NS). Importantly, this lack of correlation between CDA-based decoding performance and α-based decoding performance was not due to unreliable measurement of CDA-based decoding performance because the CDA-based decoding showed a reliable relationship with decoding performance using early visual ERPs (from 100–400 ms after the stimulus) (r = 0.50, P < 0.05). This is consistent with the idea that there exist lateralized and spatially global VWM representations that are dissociable (Fukuda et al. 2015).

Results of correlational analyses from experiment 3. A: correlations between neural correlates of spatially global and lateralized VWM representations. The left scatterplot shows the correlation between decoding accuracies based on the scalp distributions of contralateral and ipsilateral α (8–12 Hz) power. The right two scatterplots show the relationship between the decoding accuracies between the CDA-based and contralateral α-power-based decoders (red) and between the CDA-based and ipsilateral α-power-based decoders (blue). B: correlations between neural correlates of perceptual encoding. The left scatterplot shows the correlation between decoding accuracies based on the scalp distributions of contralateral and ipsilateral θ (4–7 Hz) power. The right two scatterplots show the relationship between the decoding accuracies between the VEP-based and contralateral θ-power-based decoders (red) and between the VEP-based and ipsilateral θ-power-based decoders (blue). C: correlation between VEP-based decoding performance and CDA-based decoding performance. The asterisks represent the statistical significance of correlations (where * is <0.05).
Interestingly, however, neural measures observed during perceptual encoding showed some interrelations. θ-based decoding performance was correlated across hemispheres (r = 0.49, P < 0.05), showing its spatially global nature of perceptual encoding. When correlated with decoding performance using early visual ERPs, the contralateral decoding revealed a significant relationship (r = 0.51, P < 0.05), whereas ipsilateral decoding did not (r = 0.13, NS). This makes sense given the contralateral bias for θ-based decoding and the more robust distractor representation in the ipsilateral θ distribution. Together, this is consistent with the idea that θ power response at least partially reflects the initial volley of sensory activity in the visual system that generates early visual ERPs (e.g., the visual N1).
DISCUSSION
In the present study, we sought to understand the relationship between neuroimaging and electrophysiological findings about the spatial nature of VWM representations. In experiments 1 and 2, we used the hallmark of VWM capacity limitations, the increase in the number of objects remembered up to an asymptote, to identify both spatially specific and spatially global VWM representations. We reasoned that the electrophysiological activity underlying the representation of objects in VWM should increase monotonically up to the capacity limit of VWM and remain at that level even at higher set sizes. As expected, the spatially specific CDA demonstrated this capacity-defined set size effect. However, we also found a spatially global electrophysiological signal that showed this same capacity limit. Specifically, we found bilateral α-band desychronization that changed in magnitude with additional items in memory, until VWM capacity was reached. Finally, the scalp distribution of this spatially global α power suppression in either hemisphere, as well as that of the CDA, was sufficient to read out the object represented in VWM. These results indicate that both spatially specific and spatially global VWM representations are maintained in the brain and that they can be measured simultaneously.
One remaining question is what exactly it is that the scalp distribution of the CDA and the α power decoded in experiment 3. Although we asked participants to remember the orientation of the bars, presumably by storing this object representation in VWM, participants could have simply kept their attention on where the bar met the ring. The present study does not allow us to distinguish between these alternative explanations of the effects. One major difficulty in making such a distinction is the intricate relationship between the definition of spatial working memory and sustained spatial attention (Chun 2011). Foster and colleagues (2016) have recently attempted this dissociation by differentiating the stimulus location and the location at which participants reported the stimulus. Indeed, they successfully showed that the scalp distribution of α power decoded the stimulus location even in a task in which participants reported all different stimulus locations at a single test location. Although this result seems to indicate that the scalp distribution of α power is related to working memory rather than the locus of spatial attention, future studies are necessary to come to a definitive conclusion.
Selective Maintenance of Spatially Global VWM Representations
Our study provides novel findings about the nature of the spatially global VWM representations. In previous fMRI studies in which the content of VWM was successfully decoded from patterns of multivoxel activity, the target stimulus was either presented in isolation or with another target item (Ester et al. 2009; Pratte and Tong 2014). Thus, it was unclear if a spatially global representation is constructed for any visually presented object without regard to its task relevance.
Our study tested for effects of task relevance by simultaneously presenting a distractor. The finding that neither contralateral nor ipsilateral scalp distribution of α power reliably decoded the distractor identity supports the selectivity of this spatially global VWM activity for the items that are being held in memory. Although there was a bias toward the target item, the transient contralateral θ power distribution reliably classified the identity of both target and distractor representations. This suggests that the distractor information was perceptually encoded but was not maintained in VWM over the retention interval. It would be interesting for a future study to examine the difference in these neighboring frequency responses. For example, why was the distractor item was decodable from the transient contralateral θ power response? One hypothesis is that this θ response reflects the automatic perceptual encoding of visual stimuli and top-down mechanisms act on this automatic processing to bias the maintenance of the target item over the distractor item. Another hypothesis is that this θ response is under voluntary control and underlies the selective encoding of the target item on a majority of the trials, but subjects erroneously encoded the distractor item and then removed it from VWM on a small number of trials due to occasional lapses of attention (Adam et al. 2015). As a consequence, the transient θ power response reflects the distractor items and, thus, is sensitive to the identity of the distractor. Future work focused on this oscillatory signature will be needed to distinguish between these hypotheses that fall out of the present study.
Dissociable Correlates of Two Types of VWM Representations
Our study showed that electrophysiological correlates of contralateral and spatially global VWM representations are dissociable. The existence of the two types of VWM representations could explain the contralateral superiority in MVP classification to decode the content of VWM. In Pratte and Tong (2014), they found that contralateral MVP in primary visual areas classified the content of VWM significantly better than its ipsilateral counterpart. Given that the contralateral hemisphere has both spatially specific and spatially global VWM representations, it is reasonable that contralateral MVP can classify the content of VWM better than ipsilateral MVP.
The discovery of dissociable neural correlates for contralateral and spatially global VWM will lead to a more precise characterization of VWM as well as its relationship with other cognitive abilities. Recently, many studies have used the ERP correlate of the contralateral VWM representation (i.e., the CDA) to infer the nature of VWM representation and its relationship with other cognitive abilities (Fukuda and Vogel 2009; Luria and Vogel 2011; Reinhart and Woodman 2015; Spronk et al. 2013; Tsubomi et al. 2013; Unsworth et al. 2014b; Vogel et al. 2005). It will be interesting to examine the similarities and differences that our spatially global VWM representations exhibit compared with the lateralized representations, since the comparison may result in a more holistic understanding of VWM and how it is used in complex cognitive processing.
GRANTS
This research was supported by National Eye Institute Grants R01-EY-019882;, R01-EY-025275;, P30-EY-08126;, and T32-EY-007135.
AUTHOR CONTRIBUTIONS
K.F. and M.-S.K. conception and design of research; K.F. performed experiments; K.F. analyzed data; K.F., M.-S.K., and G.F.W. interpreted results of experiments; K.F. prepared figures; K.F. drafted manuscript; K.F., M.-S.K., and G.F.W. edited and revised manuscript; K.F., M.-S.K., and G.F.W. approved final version of manuscript.
REFERENCES
- Adam KC, Mance I, Fukuda K, Vogel EK. The contribution of attentional lapses to individual differences in visual working memory capacity. J Cogn Neurosci 27: 1–16, 2015. [Europe PMC free article] [Abstract] [Google Scholar]
- Brouwer GJ, Heeger DJ. Decoding and reconstructing color from responses in human visual cortex. J Neurosci 29: 13992–14003, 2009. [Europe PMC free article] [Abstract] [Google Scholar]
- Chun MM. Visual working memory as visual attention sustained internally over time. Neuropsychologia 49: 1407–1409, 2011. [Abstract] [Google Scholar]
- Cowan N. The magical number 4 in short-term memory: a reconsideration of mental storage capacity. Behav Brain Sci 24: 87–185, 2001. [Abstract] [Google Scholar]
- Ester EF, Anderson DE, Serences JT, Awh E. A neural measure of precision in visual working memory. J Cogn Neurosci 25: 754–761, 2013. [Europe PMC free article] [Abstract] [Google Scholar]
- Ester EF, Serences JT, Awh E. Spatially global representations in human primary visual cortex during working memory maintenance. J Neurosci 29: 15258–15265, 2009. [Europe PMC free article] [Abstract] [Google Scholar]
- Foster JJ, Sutterer DW, Serences JT, Vogel EK, Awh E. The topography of alpha-band activity tracks the content of spatial working memory. J Neurophysiol 115: 168–177, 2016. [Europe PMC free article] [Abstract] [Google Scholar]
- Fukuda K, Mance I, Vogel EK. α Power modulation and event-related slow wave provide dissociable correlates of visual working memory. J Neurosci 35: 14009–14016, 2015. [Europe PMC free article] [Abstract] [Google Scholar]
- Fukuda K, Vogel E, Mayr U, Awh E. Quantity, not quality: the relationship between fluid intelligence and working memory capacity. Psychon Bull Rev 17: 673–679, 2010. [Europe PMC free article] [Abstract] [Google Scholar]
- Fukuda K, Vogel EK. Human variation in overriding attentional capture. J Neurosci 29: 8726–8733, 2009. [Abstract] [Google Scholar]
- Haegens S, Luther L, Jensen O. Somatosensory anticipatory alpha activity increases to suppress distracting input. J Cogn Neurosci 24: 677–685, 2012. [Abstract] [Google Scholar]
- Handel BF, Haarmeier T, Jensen O. Alpha oscillations correlate with the successful inhibition of unattended stimuli. J Cogn Neurosci 23: 2494–2502, 2011. [Abstract] [Google Scholar]
- Harrison SA, Tong F. Decoding reveals the contents of visual working memory in early visual areas. Nature 458: 632–635, 2009. [Europe PMC free article] [Abstract] [Google Scholar]
- Luria R, Vogel EK. Visual search demands dictate reliance on working memory storage. J Neurosci 31: 6199–6207, 2011. [Europe PMC free article] [Abstract] [Google Scholar]
- Pratte MS, Tong F. Spatial specificity of working memory representations in the early visual cortex. J Vis 14: 22, 2014. [Europe PMC free article] [Abstract] [Google Scholar]
- Reinhart RM, Woodman GF. Enhancing long-term memory with stimulation tunes visual attention in one trial. Proc Natl Acad Sci USA 112: 625–630, 2015. [Europe PMC free article] [Abstract] [Google Scholar]
- Sauseng P, Klimesch W, Heise KF, Gruber WR, Holz E, Karim AA, Glennon M, Gerloff C, Birbaumer N, Hummel FC. Brain oscillatory substrates of visual short-term memory capacity. Curr Biol 19: 1846–1852, 2009. [Abstract] [Google Scholar]
- Serences JT, Ester EF, Vogel EK, Awh E. Stimulus-specific delay activity in human primary visual cortex. Psychol Sci 20: 207–214, 2009. [Europe PMC free article] [Abstract] [Google Scholar]
- Spronk M, Vogel EK, Jonkman LM. No behavioral or ERP evidence for a developmental lag in visual working memory capacity or filtering in adolescents and adults with ADHD. PloS One 8: e62673, 2013. [Europe PMC free article] [Abstract] [Google Scholar]
- Tsubomi H, Fukuda K, Watanabe K, Vogel EK. Neural limits to representing objects still within view. J Neurosci 33: 8257–8263, 2013. [Europe PMC free article] [Abstract] [Google Scholar]
- Unsworth N, Fukuda K, Awh E, Vogel EK. Working memory and fluid intelligence: capacity, attention control, and secondary memory retrieval. Cogn Psychol 71: 1–26, 2014a. [Europe PMC free article] [Abstract] [Google Scholar]
- Unsworth N, Fukuda K, Awh E, Vogel EK. Working memory delay activity predicts individual differences in cognitive abilities. J Cogn Neurosci 27: 1–13, 2014b. [Europe PMC free article] [Abstract] [Google Scholar]
- Van Dijk H, Schoffelen JM, Oostenveld R, Jensen O. Prestimulus oscillatory activity in the alpha band predicts visual discrimination ability. J Neurosci 28: 1816–1823, 2008. [Abstract] [Google Scholar]
- Vogel EK, Machizawa MG. Neural activity predicts individual differences in visual working memory capacity. Nature 428: 748–751, 2004. [Abstract] [Google Scholar]
- Vogel EK, McCollough AW, Machizawa MG. Neural measures reveal individual differences in controlling access to working memory. Nature 438: 500–503, 2005. [Abstract] [Google Scholar]
- Whitmarsh S, Barendregt H, Schoffelen JM, Jensen O. Metacognitive awareness of covert somatosensory attention corresponds to contralateral alpha power. Neuroimage 85: 803–809, 2014. [Abstract] [Google Scholar]
Articles from Journal of Neurophysiology are provided here courtesy of American Physiological Society
Full text links
Read article at publisher's site: https://doi.org/10.1152/jn.00991.2015
Read article for free, from open access legal sources, via Unpaywall:
https://www.physiology.org/doi/pdf/10.1152/jn.00991.2015
Citations & impact
Impact metrics
Article citations
Comparing Neural Correlates of Memory Encoding and Maintenance for Foveal and Peripheral Stimuli.
J Cogn Neurosci, 36(9):1807-1826, 01 Sep 2024
Cited by: 0 articles | PMID: 38940724 | PMCID: PMC11324249
Shared structure facilitates working memory of multiple sequences.
Elife, 12:RP93158, 24 Jul 2024
Cited by: 1 article | PMID: 39046319 | PMCID: PMC11268885
Decomposing the multiple encoding benefit in visual long-term memory: Primary contributions by the number of encoding opportunities.
Mem Cognit, 02 Jul 2024
Cited by: 0 articles | PMID: 38956012
Discriminating orientation information with phase consistency in alpha and low-gamma frequency bands: an EEG study.
Sci Rep, 14(1):12007, 25 May 2024
Cited by: 0 articles | PMID: 38796618 | PMCID: PMC11127946
The association between working memory precision and the nonlinear dynamics of frontal and parieto-occipital EEG activity.
Sci Rep, 13(1):14252, 31 Aug 2023
Cited by: 1 article | PMID: 37653059 | PMCID: PMC10471634
Go to all (38) article citations
Similar Articles
To arrive at the top five similar articles we use a word-weighted algorithm to compare words from the Title and Abstract of each citation.
α Power Modulation and Event-Related Slow Wave Provide Dissociable Correlates of Visual Working Memory.
J Neurosci, 35(41):14009-14016, 01 Oct 2015
Cited by: 57 articles | PMID: 26468201 | PMCID: PMC4604234
ERPs and alpha oscillations track the encoding and maintenance of object-based representations in visual working memory.
Psychophysiology, 61(7):e14557, 08 Mar 2024
Cited by: 0 articles | PMID: 38459638
Flexible Coding of Visual Working Memory Representations during Distraction.
J Neurosci, 38(23):5267-5276, 08 May 2018
Cited by: 50 articles | PMID: 29739867 | PMCID: PMC5990978
The contralateral delay activity as a neural measure of visual working memory.
Neurosci Biobehav Rev, 62:100-108, 21 Jan 2016
Cited by: 102 articles | PMID: 26802451 | PMCID: PMC4869985
Review Free full text in Europe PMC
Funding
Funders who supported this work.
HHS | NIH | National Eye Institute (NEI) (4)
Grant ID: R01-EY019882
Grant ID: R01-EY025275
Grant ID: T32-EY007135
Grant ID: P30-EY08126
NEI NIH HHS (3)
Grant ID: R01 EY025275
Grant ID: T32 EY007135
Grant ID: R01 EY019882