Abstract
Free full text

DNA methylation patterns in luminal breast cancers differ from non‐luminal subtypes and can identify relapse risk independent of other clinical variables
Abstract
The diversity of breast cancers reflects variations in underlying biology and affects the clinical implications for patients. Gene expression studies have identified five major subtypes– Luminal A, Luminal B, basal‐like, ErbB2+ and Normal‐Like. We set out to determine the role of DNA methylation in subtypes by performing genome‐wide scans of CpG methylation in breast cancer samples with known expression‐based subtypes. Unsupervised hierarchical clustering using a set of most varying loci clustered the tumors into a Luminal A majority (82%) cluster, Basal‐like/ErbB2+ majority (86%) cluster and a non‐specific cluster with samples that were also inconclusive in their expression‐based subtype correlations. Contributing methylation loci were both gene associated loci (30%) and non‐gene associated (70%), suggesting subtype dependant genome‐wide alterations in the methylation landscape. The methylation patterns of significant differentially methylated genes in luminal A tumors are similar to those identified in CD24 + luminal epithelial cells and the patterns in basal‐like tumors similar to CD44 + breast progenitor cells. CpG islands in the HOXA cluster and other homeobox (IRX2, DLX2, NKX2‐2) genes were significantly more methylated in Luminal A tumors. A significant number of genes (2853, p < 0.05) exhibited expression–methylation correlation, implying possible functional effects of methylation on gene expression. Furthermore, analysis of these tumors by using follow‐up survival data identified differential methylation of islands proximal to genes involved in Cell Cycle and Proliferation (Ki‐67, UBE2C, KIF2C, HDAC4), angiogenesis (VEGF, BTG1, KLF5), cell fate commitment (SPRY1, OLIG2, LHX2 and LHX5) as having prognostic value independent of subtypes and other clinical factors.
1. Introduction
Breast cancer is the most frequently diagnosed cancer in women in the United States accounting for 26% of newly diagnosed cases in 2008. It is the major cause of death among adult women, and lifetime risk of dying from breast cancer is 33 per thousand among women from high income countries. Women in high income countries have a higher risk than women from middle or low income countries, reflecting different exposure to known risk factors such as hormonal exposure and weight, age at menarche, number of pregnancies brought to term, and extent of breast feeding. Only about 10% of breast cancer cases are attributed to known hereditary factors, such as mutations in BRCA1 and BRCA2. Breast carcinomas are classified using clinical (tumor size, lymph node status) and histo‐pathological (grade, hormone receptor status) metrics to evaluate likely aggressiveness and identify optimal courses of treatment. The classical prognostic factors that are typically used in the clinic are node status, tumor size and tumor grade. Estrogen receptor status and ErbB2 status are therapy response predictors but do not have significant prognostic value independent of therapy.
For some time, the major characteristic differentiating breast carcinomas for treatment purposes has been estrogen receptor (ER) status. More recently, sophisticated molecular analyses including measurements of gene expression and genomic aberrations have refined breast cancer classfication. Most classifications mirror the separation seen by ER status but with additional information identifying smaller groups with homogenous molecular alterations and/or clinical behavior. The most widely accepted molecular classification of breast carcinomas is the “Intrinsic Classification” based on gene expression first proposed by Perou and Sorlie in 2000 (Perou et al., 2000; Sorlie et al., 2003). They identified five major subtypes of breast tumors: Luminal A and Luminal B (dominated by ER positive samples and with expression of genes typical of glandular epithelium); Basal‐like (ER negative samples with expression of myoepithelial associated genes); ErbB2+ (heterogeneous ER status, but often amplified for ErbB2+ and nearby genes, and a strong resemblance to Basal‐like expression); and Normal‐like (tumors with expression patterns close to normal breast tissue). Of the five subclasses, the Luminal A and Basal‐like subtype are the most clearly defined. Several studies have shown that they represent tumors with different aberrations at the genomic level as well, and these groups correspond reasonably well to clinical characterization on the basis of ER and HER2 status, as well as proliferation markers or histological grade (Bergamaschi et al., 2006). The intrinsic subtypes have also been associated with different prognostic implications with the Luminal A subtype having better prognosis than the Basal‐like and ErbB2+ subtypes.
Several recent studies have reported on the epigenetic influences in breast cancer (Hinshelwood and Clark, 2008). These include several classically studied breast cancer genes such as ESR1, CDH1 and CDKN2A (Birgisdottir et al., 2006; Caldeira et al., 2006). Flanagan et al., (Flanagan, Cocciardi et al.) used Affymetrix promoter arrays and methyl DNA immune‐precipitation to study 33 familial breast cancers. They found DNA methylation patterns are significantly associated with BRCA mutation status, although they could not determine association with subtypes in their small sample set.
We set out to determine if the gene expression‐based subtypes have underlying epigenetic differences. Epigenetic modifications both at the chromatin and DNA level affect the structure and the expression of genes encoded in the DNA. The most widely studied epigenetic modification is the cytosine methylation in the context of the dinucleotide CpG. In embryonic stem cells such modifications is of major importance in regulating genes important for cell differentiation and function. Altered regulation of CpG methylation is also implicated in many diseases. Specifically, in cancer, methylation of CpG islands proximal to tumor suppressors such as p16, RASSF1A, and BRCA1, is a frequent event (Merlo et al., 1995; Rice et al., 1998; Dammann et al., 2000). Several high throughput microarray based methods have been described that employ methylation‐sensitive restriction enzymes for detection of methylation (Huang et al., 1999; Khulan et al., 2006; Ordway et al., 2006; Irizarry et al., 2008). We recently reported one such method, called Methylation Oligonucleotide Microarray Analysis (MOMA) (Kamalakaran et al., 2009). MOMA allows for high throughput analysis of thousands of genomic loci including most CpG islands, and requires as little as 100 ng of sample DNA. The large number loci that can be interrogated using MOMA allows an unbiased investigation of genome‐wide methylation patterns as well as an in‐depth search for loci whose methylation have clinical importance in breast cancer.
The Oslo Micrometastases Study (OMS) (Wiedswang et al., 2003) has been the subject of one of the largest concentration of parallel molecular analysis techniques on a clinical dataset. The full study comprises over 900 breast cancer cases with information about presence of disseminated tumor cells (DTC), and associated clinical information such as node status, tumor size, estrogen receptor status, grade as well as a median of 85 months in follow‐up. A subset of approximately 140 patients is represented with fresh frozen samples from the primary tumor, matched blood, and micrometastases and has been used in parallel pilot studies of immunohistochemisty (Bergamaschi et al., 2006), whole genome mRNA expression (Naume et al., 2007), arrayCGH, whole genome SNP and SNP‐CGH, whole genome miRNA expression analyses and high throughput sequencing. In this paper we report the results of one of two independent DNA methylation studies performed on this information‐rich set employing the genome‐wide MOMA method. The MOMA method provides a global view of methylation state of the genome, covers most of the CpG islands and provides resolution at a sub‐CpG island level dependant on restriction fragment generated by representation. However, some classically studied CpG islands associated with cancer‐related genes such as p16 and RASSF1A are missed by MOMA because of excessive fragmentation by the representational enzyme. To address this issue, a complementary and parallel study was carried out using Illumina Golden Gate array methodology and the results are reported in the concurrently submitted paper by Rønneberg et al The Illumina method addresses a much smaller but focused portion of the genome (807 cancer‐related genes), but provides single nucleotide resolution for 1505 CpG sites in these genes. Together, these two studies provide a detailed survey of epigenetics in breast cancer, their relationship to the molecular subtypes and other clinical factors such as hormone status and TP53 mutational status and their implications for relapse risk.
2. Results
We performed MOMA (Methylation Oligonucleotide Microarray Analysis) on 119 breast samples (108 frozen breast tumors plus 11 normal adjacent samples collected during surgery). The primary tumors from the Oslo Micrometastases Study (OMS) (Wiedswang et al., 2003) were from stage I to stage III patients, and came with a variety of clinical, pathological and molecular data and have been described previously. A summary of the clinical information on the samples is provided in Table 1. MOMA has been described and validated using cell lines, breast and ovarian tumor tissues previously (Kamalakaran et al., 2009). Briefly, the sample DNA is digested with MspI to make a representation, ligated with adapters and split into two pools. One pool is restricted with the methylation‐specific restriction enzyme McrBC, while the other pool is a mock treated control. The two pools are then amplified, fluorescently labelled and hybridized onto a microarray. The ratio of intensity of the mock‐treated sample over McrBC‐treated sample provides an estimate of methylation. The methylation states of each fragment are determined by using an expectation maximization algorithm to assign probability of each fragment belonging into each of three distinct states – unmethylated (−1 state), partially methylated (0 state), and methylated (+1 state). A fragment is determined to be differentially methylated if it switches states from one state to another across samples (−1 to 0 or 0 to +1 or −1 to +1).
Table 1
Clinical Characteristics of tumors profiled in this study.
Sample Category | Sample Number |
---|---|
Samples | |
1. Tumors | 108 |
2. Normal | 11 |
Expression Subtype | |
1. Luminal A | 40 |
2. Luminal B | 13 |
3. ErbB2‐Like | 19 |
4. Basal | 12 |
5. Normal‐Like | 14 |
6. Not Available | 10 |
TOTAL | 108 |
Hormone Receptor Status | |
1. Positive | 66 |
2. Negative | 35 |
3. Not Available | 7 |
TOTAL | 108 |
Tumor Grade | |
1. Grade I | 14 |
2. Grade II | 52 |
3. Grade III | 40 |
4. Not Available | 2 |
TOTAL | 108 |
Tumor Size (Category)![]() | |
1. pT1 | 46 |
2. pT2 | 49 |
3. pT3 | 6 |
4. pT4 | 4 |
5. Not Available | 3 |
TOTAL | 108 |
Lymph Node Status ![]() | |
1. pN0 | 43 |
2. pN1 | 31 |
3. pN2 | 18 |
4. pN3 | 8 |
5. Not Available | 8 |
6. TOTAL | 108 |
We first identified loci whose methylation state differed in breast tumors when compared to normal tissue to determine baseline differences. We used the t‐statistic to identify consistently altered loci between tumors and normal tissue. A list of the top 100 gene associated fragments that are significantly differentially methylated between tumors and normal tissue is provided in Supplemental Table 1 and was employed in the hierarchical clustering described in the following section. Some of our results confirmed previous findings for specific genes, such as the CpG island proximal to RUNX3 (p‐value = 8.4 e−7) and PITX2 (p‐value = 2.4 e−29) which have been shown to be inactivated in breast cancer (Maier et al., 2007; Harbeck et al., 2008; Subramaniam et al., 2009). We also identified several strong novel candidate biomarkers for breast cancer. Additionally, we found evidence that methylation of CpG islands upstream of certain microRNAs is correlated with tumorigenesis in this sample set. The CpG islands within 5 kb of miRNAs miR196a1 (p‐value = 0.00058), miR335 (p‐value = 2.2e‐14), miR124a3 (p‐value = 1.4e‐9) and miR423 (p‐value = 4.19e‐13) all show increased methylation in tumors when compared to normal tissue. Expression of one of these, miR335, has been previously shown to be lost in a majority of primary breast tumors and this loss of expression leads to an increased likelihood of metastasis (Tavazoie et al., 2008). The methylation status of three such candidates (GPR10, DRD5, CDKN1C) in a set of normal tissues and breast tumors is plotted in Figure 1a. These three candidates were then evaluated for significant methylation in tumor tissue using an independent sample set using bisulfite sequencing. All three candidates showed significantly increased methylation in tumor tissue while maintaining minimal methylation in matched normal tissues ( Figure 1b).
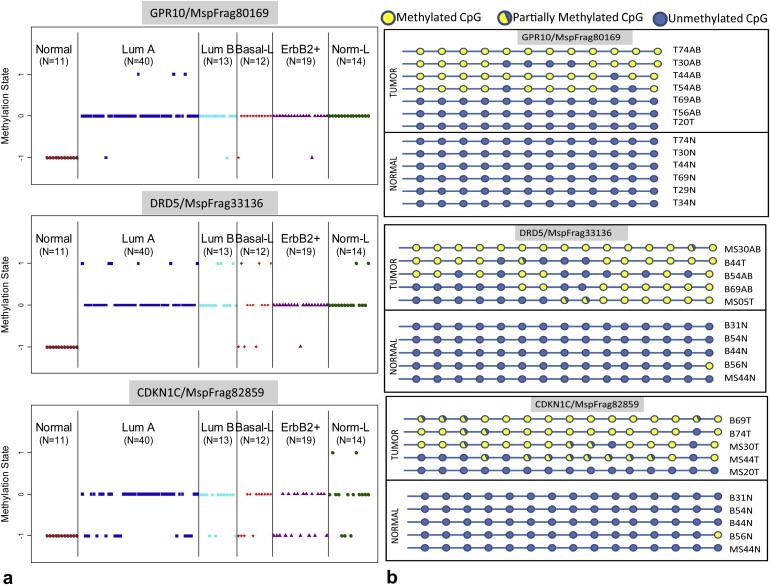
(a) Differentially methylated loci between tumors and normal tissue of 3 loci proximal to genes GPR10, DRD5 and CDKN1C. The samples are grouped by expression subtype (b) Bisulfite sequencing of selected loci in independent sample set validates significant methylation in tumors compared to normal tissue.
We next investigated DNA methylation in the clinical context of breast cancer subtypes, histology and prognosis. The 108 tumors with known expression‐based subtypes were divided into a discovery set (83 samples) for clustering analysis and a validation set (25 samples). We determined the features to be used for clustering in two steps consisting of non‐overlapping sets. The top 500 loci that varied most by standard deviation across 83 tumor samples were chosen to maximize the methylation diversity in the set (Supplemental Table 2 ). Conversely, the 100 loci described above (Supplemental Table 1) that distinguished tumors from normal tissue contain little information concerning subtype diversity, but serve as unifying characteristics that are common to all breast cancers.
We used these 600 features to cluster the 83 tumors and 11 normal samples for which the expression subtype data was available. Hierarchical clustering of the samples based on these six hundred loci gave us clustering that is remarkably similar to the one produced by expression analysis. Figure 2a shows the clustering of samples based on methylation and overlays the known expression cluster of each sample. As expected, the normal breast tissue samples clustered tightly. The expression subtype was determined by identifying which of the five centroids each sample correlates most to as described by Sorlie et al. (Sorlie et al., 2003). Cluster I was dominated by samples with a high correlation to the Luminal A centroid and anti‐correlation to the Basal‐like centroid. This is in contrast to cluster II where the samples were highly correlated to the Basal‐like centroid and anti‐correlated to the Luminal A centroid. Samples in cluster III were dominated by low correlation to each of the centroids. Most of the Luminal A samples were in cluster I (22/30 samples) and the Basal‐like samples were in cluster II (7/8 samples), cluster III had a mixture of subtypes, but 5 of 10 Normal‐like samples were in this low correlation class. We computed the absolute of the difference between the correlation values to the Luminal A centroid and the Basal‐like centroid as a measure of confidence in assignment of the expression subtypes. This confidence measure is significantly greater in the samples which cluster together in the methylation‐based clustering (Methylation clusters I and II) than in samples in the third methylation cluster using a Mann–Whitney test (p‐value = 0.0013). Finally, changing the number of loci used from top 500 most variant to top 250 or top 1000 did not significantly alter the clustering and retained the separation of the luminal A samples from basal‐like/ErB2+ samples (data not shown).
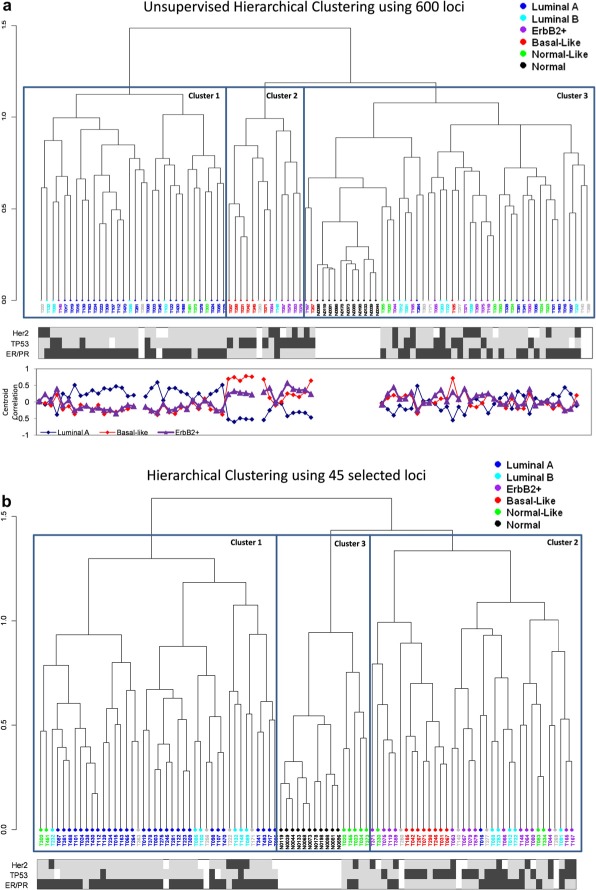
(a) Unsupervised clustering of breast tumors using methylation data groups samples similarly to those obtained by expression based analysis. Luminal A (Blue) subtypes (Cluster 1) form a distinctly separate group from the basal‐like(red) and ErbB2+(purple) subtypes. ER status, TP53 mutational status and Her status by FISH are plotted under the dendrogram (Black = positive, Gray = Negative, white = NA). Correlations to Luminal, Basal and ErbB2+ Centroid derived from expression data are plotted under the heatmap. Clusters I and II have unambiguous assignment of expression subtype, while cluster III contains samples with samples having weak correlations to multiple centroids. (b) fragment subset clusters luminal and basal groups. (c) Addition of 25 validation samples to the clustering retains the separation of luminal subtypes from basal/ErbB2 enriched subtypes.
We then investigated the possibility of improving the clustering based on expression subtypes using a selected subset of loci. We used a semi‐supervised version of our evolutionary search tool, a Genetic Algorithm (GA) based feature selection method (Schaffer et al., 2005). In this approach, we used the GA to select and evaluate subsets of loci for uniformity of the cluster members' expression subtype. This subset selection resulted in many smaller subsets outperforming the original 600‐loci set as a stratification tool. We show in Figure 2b one such subset which included just 45 of the original 600 loci. This subset substantially improves clustering of the subtypes, most notably the basal samples. Finally we added 25 new samples as validation into the clustering algorithm and we were able to cluster 9/9 Luminal A samples in the Luminal cluster, 9/10 Basal‐like/ErbB2+ in the Basal‐like/ErbB2+ cluster and 5/5 Normal‐like samples in the Normal‐like cluster (Figure 2c).
Interestingly, these chosen loci were not related to the genes that were identified in the expression subset as intrinsic gene set. Of the 500 most varying loci, 146 loci were found to be within 5000 bp of the transcriptional start site of a known gene. Many of these genes have been implicated in breast cancer, notably the homeobox gene clusters (HOXA2, HOXB13, DLX3), cell surface receptors (EGFR, the WNT family receptor, FZD8, toll‐like receptor, TLR2), keratins (KRT7) and forkhead proteins (FOXF1). Supplemental Figure 1 shows a subset of these genes and their functional categories.
The remainder were not near any annotated gene transcriptional start site. We looked at the evolutionary conservation and regulatory potential for these loci to evaluate the possibility that these could have any unannotated regulatory functions. Using data from the multi‐species conservation derived regulatory potential of the genome (Taylor et al., 2006), we compared the loci that possess discriminatory power to cluster breast cancer subtypes over a randomly chosen subset. These islands that contribute to clustering turned out to be not significantly more conserved than the rest of the genome ( Supplemental Figure 2 ). The absence of any clear increased conservation or regulatory regions in loci that have subtype‐specific methylation is perhaps surprising. The CpG islands which vary the most among tumors are clearly able to differentiate between Luminal and Basal‐like tumors. This observation leads us to the possibility that the etiology of the different tumor subtypes maybe reflected in the genome‐wide methylation patterns. When we investigated the possibility that the luminal and basal subtypes have different global methylation levels, we determined that the overall methylation state in each sample was comparable. We find no evidence of a “methylator phenotype” in breast cancer as has been seen in colorectal cancer (Toyota et al., 1999a; Toyota et al., 1999b). The overall levels of methylation (as determined by the number of loci in each of the “−1”,“0” and “+1” states) in luminal and basal tumors remain the same, but distinct patterns of methylation separate the two types.
We then specifically looked for genes that are differentially methylated in the various subtypes. In this analysis we used a t‐test to identify fragments that were most different between Luminal A expression subtype and the Basal‐like or ErbB2+ expression subtypes. We identified over 637 loci that were significant (p‐value<0.01 after correcting for multiple‐testing), including 360 loci that were mappable to a gene using our previously defined criteria (Supplemental Table 3 ). Interestingly, 56% of the significant loci were found to be near genes, compared to only 30% in the most varying loci used in the unsupervised clustering analysis. A histogram analysis of the distance of these fragments to the transcriptional start site of their associated genes showed that most of the significant fragments were within conventionally defined promoter regions with more than 50% of our significant genes within 500b of the TSS. ( Supplemental Figure 3 ). The table includes many gene families implicated in cancer and differentiation – HOX A family (HOXA2, HOXA6, HOXA7, HOXA10, HOXA11, HOXC10, HOXC5, HOXD13), FOX family (FOXC1, FOXD4, FOXD4L1, FOXD4L3, FOXF2, FOXP4, FOXQ1), growth factors and growth factor receptors (EGFR, FGF9, FGF19), matrix proteins (COL14A1, COL16A1, COL7A1, CLDN10, CLDN5) and protocadherins (PCDH10, PCDHAC2, PCDHGA10, PCDHGA11). The HOX genes have been previously described to have aberrant methylation in a variety of cancers, with the HOX A cluster aberrantly methylated in breast cancer (Novak et al., 2006). Indeed, we find many HOX genes to be significantly altered in their methylation status. Importantly when we looked at the subtypes of cancer, the HOXA gene alterations were more common in tumors belonging to the Luminal A expression subtype than the others. Increased methylation of HOXA2, HOXA7, HOXA10 and HOXA11 was observed in a majority of Luminal tumors, while their levels stayed similar to normal breast tissue in the other subtypes ( Figure 3a). A heatmap of the average methylation levels of a few interesting candidates from the list of differential methylated genes with subtype‐specific methylation are shown in Figure 3b. Interestingly, a majority of the significant loci have higher levels of methylation in luminal samples when compared to the basal‐like/ErbB2‐plus samples.

(a) Deregulation of methylation at HOX A genes in Luminal cancer subtypes (b) Heatmap of average methylation values of 100 selected genes groups by subtype. Transcription factors belonging to Homeobox family (HOXA, IRX, LHX1), forkhead family (FOXC1, FOXD4, FOXP2), cell adhesion molecules (KRT7, COL7A1, COL14A1, COL16A1) and protocadherins (PCDHAC2, PCDHGA10) have increased methylation levels in luminal tumors when compared to basal and ErbB2 subtypes.
2.1. DNA methylation loci as prognostic factors
One of the critical factors in managing treatment for breast cancer is identifying those at most risk for metastatic disease. We used distant disease free survival (DDFS) as the clinical end point to measure tumor aggressiveness. The classical clinical prognostic factors such as node status and tumor size significantly stratified patients into good and poor prognosis groups, while receptor status and adjuvant therapy status did not possess significant prognostic value. The distributions and prognostic value of the clinical variables are summarized in Supplemental Table 4. We then used this clinical data to identify genomic loci whose methylation status correlated with tumor aggressiveness. Loci were first filtered by choosing only those that were likely to be informative by requiring that the minority methylation state of a given locus contains at least 15 samples. This resulted in a total of 34,371 loci, of which 11,459 loci were found to be within 5000 bp from a transcriptional start site and 22,912 loci were not near any transcriptional start site.
We evaluated each of the above loci for their ability to stratify patients into good or poor prognosis groups depending on their methylation status. Using the Kaplan–Meier estimator of survival as the underlying statistical model, we identified the association of methylation states with DDFS. The Kaplan–Meier estimator calculates the probability of no systemic recurrence at a given time by using the time to systemic recurrence from retrospective data. Survival curves were estimated for each state of a given locus and the log‐rank (Mantel‐Haenzel) test was used to determine whether the two survival curves were significantly different from each other. We estimated statistical significance based on 1000 permutations of the time to distant metastasis data and chose loci that achieved a significance level less than 0.05 after multiple‐testing correction. This led to a total of 2559 loci chosen as significantly stratifying the patients into good and poor prognosis groups.
To find which of the above loci are providing prognostic information independent of other clinico‐pathological variables, we performed multivariate Cox regression analysis using other significant clinical variables. Of the 2559 significant loci, a total of 921 loci remained significant when included in a multivariate Cox regression with the other clinical variables. Of these 921 loci, 490 were found to be within 5,000bp from a transcriptional start site while 431 were deemed intergenic. It is notable that the ratio of genic to intergenic loci amongst the prognostic factors is significantly different compared to the ratio of the complete list of 34,371 loci included initially for survival analysis (p‐value < 10−3). Gene ontology enrichment analysis of the 490 genic loci revealed significant (p‐value<0.01 after multiple‐testing correction) enrichment of genes related to transcription factor activity, regulation of MAP kinase activity, cell proliferation, cell death, angiogenesis and neuronal development (Beissbarth and Speed, 2004). This suggests that loci associated with poor prognosis are more likely to be functionally important in the metastasis cascade, thus opening up the possibility of identifying potential biomarkers for prognosis as well as targets for treatment.
Kaplan–Meier Survival curves are plotted for a set of interesting candidates in Figure 4. These genes are involved in cancer‐related molecular functions such as cell death (TOP1 p‐value = 5e‐04; SEPT4 p‐value = 6e‐03), cell cycle (UBE2C p‐value = 9e‐04; MAPK12 p‐value = 1e‐03; TUBB3 p‐value = 3e‐02), cell fate commitment (KLF4 p‐value = 9e‐03; FGF12 p‐value = 4e‐03) and transcription factor activity (ELK1, p‐value = 5e‐03). The list of prognostic genes organized according to their significant gene ontology terms are presented in Table 2. Notably, the methylation state of a gene amongst the samples with poor prognosis is different from its state in normal breast tissue samples. The complete list of genes and intergenic loci whose methylation status correlated significantly with relapse likelihood is given in Supplemental Table 5.
Table 2
Hazard ratios of selected fragments along with their GO category.
Significant Gene Ontology Term (p‐value) | Gene Name | Methylation State in Normal Tissue | Poor Prognosis Methylation State | Significance of Survival Difference(p‐value) | Significance of Multivariate Cox Coefficient (p‐value) | Multivariate Hazard Ratio (lower 0.95, upper 0.95) |
---|---|---|---|---|---|---|
Cell Death(1e‐03) | TOP1 | M | UM | 2e‐05 | 5e‐04 | 3.677 (1.75, 7.72) |
TDGF1 | UM | M | 6e‐05 | 9e‐04 | 4.08 (1.77, 9.38) | |
CIDEB | UM | M | 5e‐05 | 2e‐03 | 4.02 (1.63, 9.89) | |
UNC5A | UM | M | 4e‐03 | 0.027 | 2.74 (1.12, 6.7) | |
Cell Fate Commitment(6e‐04) | SPRY1 | M | UM | 1e‐04 | 2e‐03 | 4.0 (1.65, 9.73) |
FGF12 | UM | M | 1e‐04 | 4e‐03 | 3.31 (1.47, 7.49) | |
KLF4 | M | UM | 1e‐03 | 9e‐03 | 2.63 (1.27, 5.46) | |
OLIG2 | UM | M | 5e‐03 | 0.023 | 2.56 (1.14, 5.76) | |
Cell Proliferation(6e‐08) | KI‐67 | M | UM | 5e‐03 | 0.004 | 3.43 (1.48, 7.93) |
HDAC4 | M | UM | 1e‐03 | 0.016 | 2.62 (1.19, 5.75) | |
Cell Cycle Process(7e‐04) | KIF2C | M | UM | 2e‐03 | 8e‐03 | 2.65 (1.28,5.49) |
UBE2C | M | UM | 1e‐05 | 9e‐04 | 3.57 (1.68, 7.59) | |
MAPK12 | M | UM | 9e‐04 | 1e‐03 | 3.51 (1.60, 7.67) | |
TUBB3 | M | M | 9e‐03 | 0.035 | 3.19 (1.09, 9.39) | |
Vasculature Development(8e‐04) | VEGF | M | UM | 8e‐03 | 7e‐03 | 3.01 (1.34, 6.75) |
KLF5 | M | UM | 4e‐03 | 4e‐03 | 3.56 (1.49, 8.53) | |
BTG1 | M | UM | 2e‐03 | 0.013 | 2.65 (1.22, 5.74) | |
Transcription Factor Activity(1e‐04) | LHX5 | UM | M | 6e‐03 | 0.032 | 2.94 (1.10, 7.87) |
LHX2 | M | UM | 4e‐03 | 0.028 | 2.32 (1.09, 4.91) | |
ONECUT2 | M | UM | 8e‐03 | 0.029 | 2.74 (1.11, 6.79) | |
ONECUT1 | UM | M | 8e‐03 | 0.023 | 2.8 (1.15, 6.83) | |
FOXH1 | UM | M | 8e‐05 | 7e‐05 | 5.09 (2.28, 11.36) | |
ELK1 | UM | M | 1e‐03 | 5e‐03 | 3.53 (1.46, 8.53) | |
JUN | M | UM | 4e‐03 | 0.025 | 2.44 (1.12, 5.33) | |
GSC | M | UM | 3e‐04 | 2e‐03 | 3.69 (1.61, 8.45) |
We find demethylation of the Topoisomerase I (TOP I) promoter increased the likelihood of relapse in those patients. Other candidate genes include Goosecoid (GSC, p‐value = 3e‐04), which has been previously shown to promote metastasis through its role in epithelial‐mesenchymal‐transitions (Hartwell et al., 2006) and whose demethylation is found to be associated with poor prognosis in our study; demethylation of Vascular endothelial growth factor, VEGF (p‐value = 8e‐03), whose role in angiogenesis is well known is also associated with poor prognosis; and ONECUT1 (p‐value = 8e‐03), whose methylation has been previously associated with cervical cancer and is associated with poor prognosis in our study. We found that the demethylation of TP53BP2 (p‐value = 1e‐03), a protein that binds to TP53 leads to significantly poorer prognosis independent of treatment and other clinical variables. Due to its reported role in regulating the apoptotic function of TP53 (Sullivan and Lu, 2007), we investigated the relationship of TP53BP2 methylation and relapse risk in TP53 wild type and TP53 mutated populations. Interestingly the prognostic value of TP53BP2 methylation status was completely eliminated in TP53 mutated populations, revealing an effect modifying link between TP53 mutation and TP53BP2 methylation based prognosis ( Figure 5 ).
2.2. Effects of methylation on Gene expression
The OMS study samples have been profiled using cDNA arrays to measure RNA levels in each sample relative to a pooled RNA reference and expression data are publicly available. The absence of absolute expression levels precluded a sample by sample correlation to the absolute methylation levels identified in this study. However, it is possible to pool the samples according to their methylation states and identify the difference in relative expression levels between these groups. For each locus, we grouped samples according to their methylation state (−1,0,+1). We then tested if there is a difference between expression levels of a given gene between 2 methylation states using the one‐sided Wilcoxon signed rank test. We used a definition of a fragment being within 5 kbp upstream to 2 kbp downstream of a transcriptional start site to identify that fragment as being associated with the gene. 9487 unique genes from the expression data set had at least one fragment mapped to this region. We analyzed the effects of methylation of these loci on expression levels of associated genes. Of these, 8393 genes had at least 10 samples exhibiting at least 2 methylation states thereby enabling us to compare relative expression level differences with methylation state. 2853 (33%) genes showed a significant anti‐correlation of the expression levels to the methylation state of the corresponding fragment (p‐value<0.05). 146 of the 500 fragments used in the unsupervised clustering were gene associated by criteria described above. Of these 146, 79 were present in the expression data. 33 of those 79 genes showed significant anti‐correlation of gene expression with methylation. Additionally, 313 genes that were significant in the survival analyses had expression data and 137 of those were found to have significant anti‐correlation between gene expression and methylation status. Of the 362 genes with significant differential methylation between basal‐like/ErbB2+ and luminal subtypes, 200 could be analyzed for correlation to expression. 118 of these genes showed significant anti‐correlation of gene expression levels to methylation states.The mean expression values of the samples in each methylation state are plotted for 50 genes in a heatmap in Figure 6.

Correlation of expression levels to methylation states of the associated fragments. Heatmap of the mean of the expression values for a selected list of 50 genes with significant anti‐correlation to methylation states is plotted. White colors indicate no samples were present in that methylation state for the gene. The number of individual samples in each state varies by gene and thus is not plotted.
3. Discussion
In this study, we performed genome‐wide scans of CpG island methylation patterns in over 100 breast tumors and normal breast tissues. The primary finding of our analysis is that luminal breast tumors have different methylation profiles when compared to other breast tumor subtypes. The pattern of methylation in Luminal tumors is distinctly different from tumors of basal‐like or ErbB2+ origin. We find that this difference is reflected throughout the CpG islands in the genome and is not limited to functional genes. We compared our results with those of the parallel study of Rønneberg et al using the Illumina Golden Gate array and reported concurrently along with this study. Our own clustering results produced three clusters (Figure 2c). Cluster I consists of mostly Luminal A subtypes, Cluster II has ErbB2+ and basal subtypes and cluster III has normal samples together with Normal‐Like and some Luminal subtypes. We determined that 72 samples were analyzed in both studies. The Rønneberg et al study also identified three methylation based clusters. Two of these clusters consisted of a majority of Luminal A expression subtypes (Clusters I and III) and one with Basal‐like and ErbB2+ subtypes (Cluster II). There were significant additional differences in ER and TP53 mutational status between Cluster I and II but not with the Cluster III. When we looked at the overlaps between our Luminal A cluster (Cluster I) with those of Rønneberg et al., 32/34 samples were identified in the Luminal majority clusters (Clusters I and III in Rønneberg et al.). Additionally, 23/28 samples in Cluster II in our study were also identified as belonging to cluster II in the Rønneberg study. These overlaps provide strong evidence of the distinct methylation differences between Luminal A subtype and the basal/ErbB2+ subtypes. Our results predict that there are fundamental changes occurring in luminal tumors that are then reflected in the genome‐wide methylation patterns. Interestingly, luminal origin tumors had significantly more methylation in fragments associated with transcription factors implicated in development and differentiation – notably the HOX A and homeobox genes and forkhead family.
These findings complement recent reports on DNA methylation patterns seen in different cell types in breast tissue (Bloushtain‐Qimron et al., 2008). Bloushtain‐Qimron et al. identified methylation patterns from FACS sorted cells from normal breast tissue; CD24+ (Luminal), MUC1+ (Luminal progenitor), CD10+ (myoepithelial progenitor) and CD44+ (multipotent progenitor). They determined that CD24 + luminal cells have increased methylation at a number of development related transcription factors when compared to CD44 + basal progenitor cells. Our own data show significant overlaps of the methylation patterns in luminal subtypes with those found in CD24 + breast cells and patterns in basal subtype overlap with multipotent CD44 + progenitor cells. A comparison of differentially methylated genes between the Bloushtain‐Qimron study and our own results showed remarkable agreement – 10 of the top genes identified in their study are consistent with our results. Table 3 shows overlaps between our study and those of Bloushtain‐Qimron et al. These findings suggest that the genome‐wide methylation pattern of the tumors reflects the methylation pattern of the cell of origin. Supporting this hypothesis is the finding that FOXC1 and HOXA10, two of the four markers identified by Bloushtain‐Qimron et al. are among the top most differentially methylated markers in our study. During the preparation of this manuscript, two new studies, (Flanagan et al; Holm et al.) reported their findings on DNA methylation patterns in breast cancer. The study by Holm et. al. showed significant differences in the DNA methylation patterns of luminal A, luminal B and basal tumors using the Illumina Beadstudio Methylation Module. These results complement our own findings, although their platform covered only 807 cancer‐related genes compared to the genome‐wide CpG islands platform used in our study. Due to this limitation, they did not identify the novel findings in our own study that DNA methylation patterns in the breast cancer subtypes are not limited only to cancer‐related genes but reflected genomewide in both gene associated and non‐gene associated CpG islands. The study by Flanagan et al., determined DNA methylation profiles in 33 familial breast cancer samples. This study primarily focused on identifying differential methylation patterns driven by BRCA1 mutation status and did not report methylation patterns being associated with subtypes.
Table 3
Overlap among methylation patterns identified in Bloushtain‐Qimron study with MOMA patterns identified in this study. “UM“ refers to unmethylated state and “M” methylated state. A”X” denotes a finding of significant correlation in our study.
Gene Name | Function | Diff Methylation Cell type 1 | Diff. Methyl. MOMA | Expression Methylation Correlation | ||
---|---|---|---|---|---|---|
CD24 + Luminal | CD44 + Progenitor | Luminal | Basal | |||
DDN | M | UM | M | UM | X | |
GATA6 | Stem Cell/WNT signaling | M | UM | X | ||
TCF7L1 | WNT pathway | M | UM | M | UM | X |
FOXC1 | Development | M | UM | M | UM | X |
FOXF2 | Development | M | UM | M | UM | X |
SOX13 | Development | M | UM | X | ||
LHX1 | Homeobox | M | UM | M | UM | |
LHX3 | Homeobox | M | UM | X | ||
HOXA10 | Homeobox | M | UM | M | UM | |
HOXA11 | Homeobox | M | UM | M | UM | |
DLX2 | Homeobox | M | UM | X | ||
NKX2‐8 | Homeobox | M | UM | |||
NKX2‐2 | Homeobox | M | UM | |||
NKX2‐5 | Homeobox | M | UM | |||
IRX2 | Homeobox | M | UM | |||
IRX5 | Homeobox | M | UM |
Finally we discovered candidate loci for cancer prognosis with molecular functions associated with cancer ‐ Cell Cycle and Proliferation (Ki‐67, UBE2C, KIF2C, HDAC4), vasculature development and angiogenesis (VEGF, BTG1, KLF5), transcription factors with roles in cell fate commitment (SPRY1, OLIG2, LHX2 and LHX5). Interestingly over‐expression of proliferation markers such as Ki‐67 and UBE2C are already being considered as prognostic markers (de Azambuja et al., 2007; Loussouarn et al., 2009) and correspondingly their methylation levels are lower in patients with poor prognosis. Topoisomerase I, which has previously implicated in chemotherapy resistance, was found to be also a risk factor for recurrence. The methylation of TOP1 may also have clinical implications for patients. 30% of breast tumors in our study have an unmethylated promoter and these patients were observed to have a higher likelihood of relapse Topoisomerase expression has been linked to the development of resistance to topoisomerase inhibitors (Burgess et al., 2008). Demethylation of TP53BP2 leads to significantly poorer prognosis (p‐value = 1e‐03) independent of treatment and other clinical variables. TP53BP2, which interacts with p53 has been implicated in gastric cancer (Ju et al., 2005). The existence of this effect in only p53 wild type tumors is intriguing and further study is needed to determine the functional nature of this link and likely mechanism of the interaction. An exhaustive analysis of all significant genes for interaction between themselves and p53 mutation status would provide further insight into the mechanisms of disease progression. Similarly, the interaction between all individual methylation loci which have prognostic ability have to be evaluated in future studies to identify combination markers which have better performance. The interaction between the individual loci may also provide insight into the different molecular pathways that are affected by treatment.
In summary, our study has found evidence for a strong association of DNA methylation within breast cancer subtypes. The question of whether this association is causal should be the focus of future studies – especially the high number of significantly methylated genes in luminal A subtypes in comparison with the basal or ErbB2+ subtypes. This is especially intriguing since we could not find any difference in the overall methylation levels among the subtypes. While this could be due to innate differences between cell types that may give rise to Luminal A and basal subtypes, there is no convincing evidence that points to the differences in the cell type of origin in the subtypes. A focused study addressing these questions would greatly advance our understanding of the breast cancer subtypes and their implications for treating patients with efficacy.
4. Methods
4.1. Samples
Samples for this study were obtained from a variety of sources. Samples for MOMA analysis were obtained from the Oslo Micrometastases Study, ranged from stages I to stage III patients, and have been described previously (Wiedswang et al., 2003). A summary of the clinical information on the samples are provided in Table 1. Normal breast samples for comparison with MOMA were obtained from the Cooperative Human Tissue Network collection.
DNA samples for validation by bisulfite sequencing were obtained from breast tumor and adjacent normal tissues from women who were undergoing surgery for breast cancer in Karnataka state in India. The breast tissues were subjected to histochemical staining to evaluate the proportion of tumor tissues (>85%). All the tumor tissues were of infiltrating ductal carcinoma and subjected to immunohistochemical staining for ER/PR/Her2 analysis. The subjects were of the age group from 25 to 70 years. This study was approved by Institutional Ethics Committee of Manipal University and samples were obtained with informed consent.
4.2. Methylation array and detection Coverage
All annotated CpG islands were obtained from the UCSC genome browser. These islands were predicted using the published Gardiner‐Garden and Frommer definition and involves the following criteria: length >= 200bp, %GC >= 50%, observed/expected CpG >= 0.6. There are 27,325 CpG islands in the range represented by 159,436 MspI fragments on the array. Arrays were manufactured by Nimblegen Systems Inc using the 390 K format to the following specifications. The CpG island annotation from human genome build 33 (hg17) was used to design a 50 mer tiling array. The 50 mers were shifted on either side of the island sequence coordinates to evenly distribute the island. The 390 K format has 367,658 available features which would not fit all islands with a 50 mer tiling.
4.3. Sample preparation and hybridization
Representations have been described previously (Lucito et al., 2003), with the following changes. The primary restriction endonuclease used is MspI. After the digestion the following linkers were ligated: MspI24mer CAGCATCGAGACTGAACGCAGCAG, and MspI12mer CGCTGCTGCGTT. The 12 mer is not phosphorylated and does not ligate. After ligation the material is cleaned by phenol‐chloroform, precipitated, centrifuged, and resuspended. The material is divided in two, half being digested by the endonuclease McrBC and the other half being mock digested according to specification by New England Biolabs. The digestion time is 3 h. As few as four 250‐μL tubes were used for each sample pair for amplification of the representation in a 100ul volume reaction. The cycle conditions were 95 °C for 1 min, 72 °C for 3 min, for 15 cycles, followed by a 10‐min extension at 72 °C. The contents of the tubes for each pair were pooled when completed. Representations were cleaned by phenol‐chloroform extraction, precipitated, resuspended, and the concentration determined. Representations were run on a gel to check for content, the McrBC digested representation being approximately 100‐150bp shorter on average than the mock. DNA was labelled as described with minor changes (Lucito et al., 2003). Briefly, 2 μg of DNA template was placed (dissolved in TE at pH 8) in a 0.2‐mL PCR tube. 5 μL of random nanomers (Sigma Genosys) were added brought up to 25 μL with dH2O, and mixed. The tubes were placed in Tetrad at 100 °C for 5 min, then on ice for 5 min. To this 5 μL of NEB Buffer2, 5 μL of dNTPs (0.6 nm dCTP, 1.2 nm dATP, dTTP, dGTP), 5 μL of label (Cy3‐dCTP or Cy5‐dCTP) from GE Healthcare, 2 μL of NEB Klenow fragment, and 2 μL dH2O was added. Procedures for hybridization and washing were followed as reported previously (Lucito et al., 2003) with the exception that the oven temperature for hybridization was increased to 50 °C.
4.4. Bisulfite sequencing
Two micro gram equivalent genomic DNA from matched normal and tumor tissue biopsy samples were used for bisulfite treatment using EZ DNA methylation Kit (Zymo research, USA) according to the manufacturer's instructions. The primers were designed by Methyl primer express V.1 (Applied Biosystems, USA). The primer sequence, amplicon length and annealing temperatures are mentioned in the Supplemental Table 6. In brief 100 ng of bisulfite treated DNA was amplified in a 25uL reaction volume containing 100 ng each of forward and reverse primer. PCR reaction contained 1XPCR buffer, 200uM dNTPs, 2 units/uL Taq Polymerase (Finnzyme, USA), using the following PCR conditions: 95 °C for 5 mins (95 °C for 30sec, respective annealing temp for 1min, 72 °C for 1min)X35 Cycles and 72 °C for 10 mins. Amplifications were performed in a Veriti Thermocycler (Applied Biosystems, USA). Following electrophoresis PCR products were gel purified, precipitated with ethanol and ammonium acetate and dissolved in sterile MQ water. PCR product was directly sequenced in ABI3130 Genetic analyzer (Applied Biosystem, USA) according to manufacturer's instructions using big dye terminator kit. The sequences were aligned and checked manually with the original unconverted sequence to find out the methylated and unmethylated CpG present at low level and also to confirm the extent of bisulfite conversion. The percentage of methylation at each CpG sites were calculated using the formula peak height for Cytosine/sum of peak height cytosine + thymine. Primers and annealing conditions used are provided in Supplemental Table 6.
4.5. Data analysis and statistics
Microarray images were scanned on a GenePix 4000 B scanner and data was extracted using Nimblescan software (Nimblegen Systems Inc). For each probe, the geometric mean of the ratios (GeoMeanRatio) of control over McrBc‐treated samples were then calculated for each experiment and its associated dye swap. The probe intensity ratios for each fragment were averaged. The intensity ratios can be described as belonging to one of the three following categories. (a) When the log ratio is positive, the fragment's status is expected to be methylated, since there is a depletion of the fragment in the McrBC‐treated pool over the mock treated control. (b) When the log ratio is negative, the fragment is expected to be unmethylated since the amplification of the depleted McrBC pool leads to overrepresentation of the individual fragments from a reduced complexity pool. (c) When the log ratio is around zero, the fragment status cannot be inferred solely from that sample alone. This is because a fragment that has negative log ratio in its unmethylated state can move to a log ratio of around zero when methylated. But a fragment that has positive log ratio when methylated will have a zero log ratio when unmethylated. We applied a standard expectation maximization algorithm for estimating parameters for a mixture of three normal distributions. This EM algorithm was modified to include the constraint that any point can belong only to distributions whose means are adjacent to the point. This allowed us to avoid a situation where a single distribution with a high standard deviation is the most likely source for points that lie nearer to another normal. We assigned a value to each point of −1, 0, or 1 if its most likely source is from the normal distribution with the low, middle, or high mean respectively. We performed this procedure for each sample which then provided each fragment a probability of being assigned to a 0, −1 or +1 state. These probabilities were then discretized into 0, +1 or −1 using a threshold of 0.66.
To establish concordance rates of MOMA analysis and ensure that our MOMA analysis pipeline is sound, we compared a set of fragments that were evaluated both by MOMA technology and by capture‐array bisulfate sequencing by Hodges, E et al (Hodges et al., 2009) in the SKN1 fibroblast and MDAMB231 breast cancer cell lines. We started with 291 CpG islands mappable between both technologies. We obtained 815 fragments that were called as methylated or unmethylated by sequencing and were binned into 0,‐1 or +1 in our MOMA analysis pipeline. In the SKN1 cell line, all 313 (100%) fragments that were called as unmethylated by MOMA (−1) were confirmed to be unmethylated by sequencing. Of the 156 fragments called as methylated by MOMA (+1), 124 (80%) were confirmed by sequencing. The correlation coefficient of this analysis was 0.86. The other fragments were called in the intermediate state (0) by MOMA. In our MOMA analysis, we use their states in other samples/cell lines to infer relative methylation levels. 58 of these fragments were also determined by MOMA to be in the methylated (+1) state in the MDAMB231 cell line. We therefore inferred that these fragments had relatively low levels of methylation in SKN1 cells. Confirming this, 81% of these fragments were determined to be unmethylated by the sequencing approach.
4.6. Semi‐supervised feature subset selection
We used a semi‐supervised genetic algorithm directed clustering approach to identify a reduced subset of loci that would classify the expression. For this purpose we used our evolutionary search tool, a feature selection method on a Genetic Algorithm (GA) (Schaffer et al., 2005). The GA is executed in a cross‐validation scheme designed to avoid overfitting. First, we implement a series of outer validation loops where in each execution of the GA, a portion of the samples is put aside. Of the 86 normal and tumor samples used, in each repetition of the GA a learning set of 77 samples are randomly selected with care to preserve proportional representation of all breast cancer subtypes. Each GA execution is terminated through a control within the method that detects when the feature pool can no longer be effectively recombined into new feature subsets. In post processing, all discovered feature subsets are re evaluated and filtered out to eliminate those that failed to perform consistently throughout the internal loop where they were evaluated. Of the resulting feature subsets, we selected the feature subset that resulted in best hierarchical clustering on all 86 learning samples. A sequence of 50 outer loop repetitions was executed for this subset selection.
The GA repeatedly evaluates populations of 100 feature subsets (i.e. loci subsets) to evolve feature subsets that best stratify the samples into subtypes. In each iteration 100 such subsets (or individuals) and up to 100 additional subsets (or offspring) based on recombination of these individuals are evaluated and assigned a fitness value. The fitness value is computed by a fitness function which here computes homogeneity of hierarchical clustering based on the feature subset. It takes as input a subset of the methylation data obtained based on a subset of the features and a set of samples with their corresponding subtype annotation based on gene expression. First, hierarchical clustering with Pearson correlation and complete linkage is performed on this data. The resulting clustering is then characterized by the homogeneity of individual clusters when the dendrogram is cut to provide 16 clusters. Based on the subtype annotation, we obtain the number of samples in each cluster that are of the subtype that makes the majority of the annotations. The sum of the majority samples from all clusters is the fitness function. Clusters of size one or two were not considered for homogeneity and as such reduced the total count. Feature subsets are compared based on their fitness values and subset sizes. The GA aims first at maximizing the fitness value and then minimizing the subset size: given two subsets of equal fitness performance, the subset with fewer features is promoted to the next iteration. Feature subset size variation in individuals is introduced in the process of creating offspring (see publication for details on offspring creation).
4.7. Survival analysis
Relapse free survival data was available for a total of 108 samples in the dataset and was correlated with the methylation status of each locus. Loci that did not have at least 15 samples in the minority state were eliminated from consideration for survival analysis. Kaplan–Meier curves were estimated for each methylation state of the selected loci and the differences between survival curves were estimated using the Mantel–Haenszel test. In order to ensure that loci did not stratify the samples purely by chance, we performed 1000 independent permutations of the clinical data and recalculated the survival curve differences for all loci. p‐values for individual loci were estimated by evaluating how often their performance was equaled or exceeded in the 1000 random permutation trials. We then derived q‐values from the estimated p‐values by correcting for multiple‐testing with a false discovery rate of 5%. Loci with q‐values lower than 0.05 were chosen for further analysis. In order to ensure that the selected loci are independent of other clinical factors, we performed multivariate Cox regression analysis on each of the selected loci along with node status, tumor size, age, tumor grade, estrogen receptor status, ErbB2 status, systemic adjuvant therapy, and hormone therapy. Loci whose Cox regression coefficient remained statistically significant and did not change by more than 20% when included with the above pathophysiological variables were chosen as being independent prognostic indicators. The methylation state of any given locus in normal tissues was assigned as the methylation state of that locus which accounted for at least 80% of the normal samples. The above survival analysis was carried out using the survival, multtest and qvalue packages in R.
4.8. Expression–methylation correlation
We used a one‐sided Wilcoxon signed rank test to check the alternate hypothesis that the expression level of a gene is significantly greater when the fragment related to the gene (−5 kb to +2 kb of tss) is in a lower methylation state. Each fragment can have increasing levels of methylation denoted by “−1”, “0” or “+1” states based on the Expectation‐Maximization analysis. Accordingly, for each fragment related to a gene, we performed three separate tests in which we compared expression levels of samples with methylation states (i) “−1” to “0”, (ii) “0” to “+1 and (iii) “−1” to “+1”. The test was only performed if each group in a given comparison had at least 10 samples. P‐value threshold was set at 0.05 for a given test to be declared significant. We still needed to account for the possibility that the number of significant correlations between methylation status and expression was not due to pure chance. We therefore permuted the sample identifiers of the methylation profiles and repeated the estimation of correlation across all loci. For each of the 1000 permuted datasets, we estimated the p‐values of the correlations between methylation status and expression values for all the loci. This distribution allowed us to calculate the number of likely correlations that would occur by pure chance. We used this background distribution and a false discovery rate of 0.05, which led to the identification of loci with statistically significant correlations between their methylation status and expression levels.
Supporting information
The following are the Supplementary data related to this article:
Supplementary data
Supplementary data
Supplementary material 1.
Supplementary data related to this article can be found online at 10.1016/j.molonc.2010.11.002.
Notes
Kamalakaran Sitharthan, Varadan Vinay, Giercksky Russnes Hege E., Levy Dan, Kendall Jude, Janevski Angel, Riggs Michael, Banerjee Nilanjana, Synnestvedt Marit, Schlichting Ellen, Kåresen Rolf, Shama Prasada K., Rotti Harish, Rao Ramachandra, Rao Laxmi, Eric Tang Man-Hung, Satyamoorthy K., Lucito Robert, Wigler Michael, Dimitrova Nevenka, Naume Bjorn, Borresen-Dale Anne-Lise, Hicks James B., (2011), DNA methylation patterns in luminal breast cancers differ from non‐luminal subtypes and can identify relapse risk independent of other clinical variables, Molecular Oncology, 5, 10.1016/j.molonc.2010.11.002. [Europe PMC free article] [Abstract] [Google Scholar]
Contributor Information
Sitharthan Kamalakaran, Email: moc.spilihp@khtrahtis.
Vinay Varadan, Email: [email protected].
Hege E. Giercksky Russnes, Email: ude.dravrah.icfd@senssur_egeh.
Dan Levy, Email: ude.lhsc@yvel.
Jude Kendall, Email: ude.lhsc@lladnek.
Angel Janevski, Email: [email protected].
Michael Riggs, Email: ude.lhsc@sggir.
Nilanjana Banerjee, Email: [email protected].
Ellen Schlichting, Email: [email protected].
Rolf Kåresen, Email: [email protected].
Man-Hung Eric Tang, Email: ude.lhsc@gnatm.
Robert Lucito, Email: ude.lhsc@oticul.
Michael Wigler, Email: ude.lhsc@relgiw.
Nevenka Dimitrova, Email: [email protected].
Bjorn Naume, Email: [email protected].
Anne-Lise Borresen-Dale, Email: on.oiu.muidar@bla.
James B. Hicks, Email: ude.lhsc@skcih.
References
- Beissbarth T., Speed T.P., 2004. GOstat: find statistically overrepresented Gene Ontologies within a group of genes. Bioinformatics. 20, (9) 1464–1465. [Abstract] [Google Scholar]
- Bergamaschi A., Kim Y.H., 2006. Distinct patterns of DNA copy number alteration are associated with different clinicopathological features and gene-expression subtypes of breast cancer. Genes Chromosomes Cancer. 45, (11) 1033–1040. [Abstract] [Google Scholar]
- Birgisdottir V., Stefansson O.A., 2006. Epigenetic silencing and deletion of the BRCA1 gene in sporadic breast cancer. Breast Cancer Res. 8, (4) R38 [Europe PMC free article] [Abstract] [Google Scholar]
- Bloushtain-Qimron N., Yao J., 2008. Cell type-specific DNA methylation patterns in the human breast. Proc. Natl. Acad. Sci. U S A. 105, (37) 14076–14081. [Abstract] [Google Scholar]
- Burgess D.J., Doles J., 2008. Topoisomerase levels determine chemotherapy response in vitro and in vivo. Proc. Natl. Acad. Sci. U S A. 105, (26) 9053–9058. [Abstract] [Google Scholar]
- Caldeira J.R., Prando E.C., 2006. CDH1 promoter hypermethylation and E-cadherin protein expression in infiltrating breast cancer. BMC Cancer. 6, 48 [Europe PMC free article] [Abstract] [Google Scholar]
- Dammann R., Li C., 2000. Epigenetic inactivation of a RAS association domain family protein from the lung tumour suppressor locus 3p21.3. Nat. Genet. 25, (3) 315–319. [Abstract] [Google Scholar]
- de Azambuja E., Cardoso F., 2007. Ki-67 as prognostic marker in early breast cancer: a meta-analysis of published studies involving 12,155 patients. Br. J. Cancer. 96, (10) 1504–1513. [Europe PMC free article] [Abstract] [Google Scholar]
- Flanagan, J. M., S. Cocciardi, et al. "DNA methylome of familial breast cancer identifies distinct profiles defined by mutation status." Am J Hum Genet 86(3): 420–433. [Europe PMC free article] [Abstract]
- Harbeck N., Nimmrich I., 2008. Multicenter study using paraffin-embedded tumor tissue testing PITX2 DNA methylation as a marker for outcome prediction in tamoxifen-treated, node-negative breast cancer patients. J. Clin. Oncol. 26, (31) 5036–5042. [Abstract] [Google Scholar]
- Hartwell K.A., Muir B., 2006. The Spemann organizer gene, Goosecoid, promotes tumor metastasis. Proc. Natl. Acad. Sci. U S A. 103, (50) 18969–18974. [Europe PMC free article] [Abstract] [Google Scholar]
- Hinshelwood R.A., Clark S.J., 2008. Breast cancer epigenetics: normal human mammary epithelial cells as a model system. J. Mol. Med. 86, (12) 1315–1328. [Abstract] [Google Scholar]
- Hodges E., Smith A.D., 2009. High definition profiling of mammalian DNA methylation by array capture and single molecule bisulfite sequencing. Genome Res. 19, (9) 1593–1605. [Europe PMC free article] [Abstract] [Google Scholar]
- Holm, K., C. Hegardt, et al. "Molecular subtypes of breast cancer are associated with characteristic DNA methylation patterns." Breast Cancer Res 12(3): R36. [Europe PMC free article] [Abstract]
- Huang T.H., Perry M.R., 1999. Methylation profiling of CpG islands in human breast cancer cells. Hum. Mol. Genet. 8, (3) 459–470. [Abstract] [Google Scholar]
- Irizarry R.A., Ladd-Acosta C., 2008. Comprehensive high-throughput arrays for relative methylation (CHARM). Genome Res. 18, (5) 780–790. [Europe PMC free article] [Abstract] [Google Scholar]
- Ju H., Lee K.A., 2005. TP53BP2 locus is associated with gastric cancer susceptibility. Int. J. Cancer. 117, (6) 957–960. [Abstract] [Google Scholar]
- Kamalakaran S., Kendall J., 2009. Methylation detection oligonucleotide microarray analysis: a high-resolution method for detection of CpG island methylation. Nucleic Acids Res. [Europe PMC free article] [Abstract] [Google Scholar]
- Khulan B., Thompson R.F., 2006. Comparative isoschizomer profiling of cytosine methylation: the HELP assay. Genome Res. 16, (8) 1046–1055. [Europe PMC free article] [Abstract] [Google Scholar]
- Loussouarn D., Campion L., 2009. Validation of UBE2C protein as a prognostic marker in node-positive breast cancer. Br. J. Cancer. 101, (1) 166–173. [Europe PMC free article] [Abstract] [Google Scholar]
- Lucito R., Healy J., 2003. Representational oligonucleotide microarray analysis: a high-resolution method to detect genome copy number variation. Genome Res. 13, (10) 2291–2305. [Europe PMC free article] [Abstract] [Google Scholar]
- Maier S., Nimmrich I., 2007. DNA-methylation of the homeodomain transcription factor PITX2 reliably predicts risk of distant disease recurrence in tamoxifen-treated, node-negative breast cancer patients–Technical and clinical validation in a multi-centre setting in collaboration with the European Organisation for Research and Treatment of Cancer (EORTC) PathoBiology group. Eur. J. Cancer. 43, (11) 1679–1686. [Abstract] [Google Scholar]
- Merlo A., Herman J.G., 1995. 5' CpG island methylation is associated with transcriptional silencing of the tumour suppressor p16/CDKN2/MTS1 in human cancers. Nat. Med. 1, (7) 686–692. [Abstract] [Google Scholar]
- Naume B., Zhao X., 2007. Presence of bone marrow micrometastasis is associated with different recurrence risk within molecular subtypes of breast cancer. Mol. Oncol. 1, (2) 160–171. [Europe PMC free article] [Abstract] [Google Scholar]
- Novak P., Jensen T., 2006. Epigenetic inactivation of the HOXA gene cluster in breast cancer. Cancer Res. 66, (22) 10664–10670. [Abstract] [Google Scholar]
- Ordway J.M., Bedell J.A., 2006. Comprehensive DNA methylation profiling in a human cancer genome identifies novel epigenetic targets. Carcinogenesis. 27, (12) 2409–2423. [Abstract] [Google Scholar]
- Perou C.M., Sorlie T., 2000. Molecular portraits of human breast tumours. Nature. 406, (6797) 747–752. [Abstract] [Google Scholar]
- Rice J.C., Massey-Brown K.S., 1998. Aberrant methylation of the BRCA1 CpG island promoter is associated with decreased BRCA1 mRNA in sporadic breast cancer cells. Oncogene. 17, (14) 1807–1812. [Abstract] [Google Scholar]
- Schaffer, J. D., A. Janevski, et al. (2005). A Genetic Algorithm Approach for Discovering Diagnostic Patterns in Molecular Measurement Data. Computational Intelligence in Bioinformatics and Computational Biology, 2005. CIBCB '05. Proceedings of the 2005 IEEE Symposium on.
- Sorlie T., Tibshirani R., 2003. Repeated observation of breast tumor subtypes in independent gene expression data sets. Proc. Natl. Acad. Sci. U S A. 100, (14) 8418–8423. [Europe PMC free article] [Abstract] [Google Scholar]
- Subramaniam M.M., Chan J.Y., 2009. RUNX3 inactivation by frequent promoter hypermethylation and protein mislocalization constitute an early event in breast cancer progression. Breast Cancer Res. Treat. 113, (1) 113–121. [Abstract] [Google Scholar]
- Sullivan A., Lu X., 2007. ASPP: a new family of oncogenes and tumour suppressor genes. Br. J. Cancer. 96, (2) 196–200. [Europe PMC free article] [Abstract] [Google Scholar]
- Tavazoie S.F., Alarcon C., 2008. Endogenous human microRNAs that suppress breast cancer metastasis. Nature. 451, (7175) 147–152. [Europe PMC free article] [Abstract] [Google Scholar]
- Taylor J., Tyekucheva S., 2006. ESPERR: learning strong and weak signals in genomic sequence alignments to identify functional elements. Genome Res. 16, (12) 1596–1604. [Europe PMC free article] [Abstract] [Google Scholar]
- Toyota M., Ahuja N., 1999. CpG island methylator phenotype in colorectal cancer. Proc. Natl. Acad. Sci. U S A. 96, (15) 8681–8686. [Europe PMC free article] [Abstract] [Google Scholar]
- Toyota M., Ahuja N., 1999. Aberrant methylation in gastric cancer associated with the CpG island methylator phenotype. Cancer Res. 59, (21) 5438–5442. [Abstract] [Google Scholar]
- Wiedswang G., Borgen E., 2003. Detection of isolated tumor cells in BM from breast-cancer patients: significance of anterior and posterior iliac crest aspirations and the number of mononuclear cells analyzed. Cytotherapy. 5, (1) 40–45. [Abstract] [Google Scholar]
Articles from Molecular Oncology are provided here courtesy of Wiley
Full text links
Read article at publisher's site: https://doi.org/10.1016/j.molonc.2010.11.002
Read article for free, from open access legal sources, via Unpaywall:
https://europepmc.org/articles/pmc5528275?pdf=render
Citations & impact
Impact metrics
Article citations
Computational deconvolution of DNA methylation data from mixed DNA samples.
Brief Bioinform, 25(3):bbae234, 01 Mar 2024
Cited by: 0 articles | PMID: 38762790 | PMCID: PMC11102637
Review Free full text in Europe PMC
Molecular Characterization and Subtyping of Breast Cancer Cell Lines Provide Novel Insights into Cancer Relevant Genes.
Cells, 13(4):301, 06 Feb 2024
Cited by: 2 articles | PMID: 38391914 | PMCID: PMC10886524
Comprehensive analysis of HOXC8 associated with tumor microenvironment characteristics in colorectal cancer.
Heliyon, 9(11):e21346, 20 Oct 2023
Cited by: 0 articles | PMID: 37885723 | PMCID: PMC10598528
DNA methylation differences in noncoding regions in ER negative breast tumors between Black and White women.
Front Oncol, 13:1167815, 24 May 2023
Cited by: 0 articles | PMID: 37293596 | PMCID: PMC10244512
Mechanisms behind context-dependent role of glucocorticoids in breast cancer progression.
Cancer Metastasis Rev, 41(4):803-832, 27 Jun 2022
Cited by: 8 articles | PMID: 35761157 | PMCID: PMC9758252
Review Free full text in Europe PMC
Go to all (89) article citations
Data
Data behind the article
This data has been text mined from the article, or deposited into data resources.
BioStudies: supplemental material and supporting data
Similar Articles
To arrive at the top five similar articles we use a word-weighted algorithm to compare words from the Title and Abstract of each citation.
Distinct patterns of promoter CpG island methylation of breast cancer subtypes are associated with stem cell phenotypes.
Mod Pathol, 25(2):185-196, 28 Oct 2011
Cited by: 34 articles | PMID: 22037257
Expression and methylation patterns partition luminal-A breast tumors into distinct prognostic subgroups.
Breast Cancer Res, 18(1):74, 07 Jul 2016
Cited by: 45 articles | PMID: 27386846 | PMCID: PMC4936004
An integrated genomics analysis of epigenetic subtypes in human breast tumors links DNA methylation patterns to chromatin states in normal mammary cells.
Breast Cancer Res, 18(1):27, 29 Feb 2016
Cited by: 46 articles | PMID: 26923702 | PMCID: PMC4770527
Molecular subtypes of breast cancer are associated with characteristic DNA methylation patterns.
Breast Cancer Res, 12(3):R36, 18 Jun 2010
Cited by: 191 articles | PMID: 20565864 | PMCID: PMC2917031