Abstract
Background
Anxious hypervigilance is marked by sensitized sensory-perceptual processes and attentional biases to potential danger cues in the environment. How this is realized at the neurocomputational level is unknown but could clarify the brain mechanisms disrupted in psychiatric conditions such as posttraumatic stress disorder. Predictive coding, instantiated by dynamic causal models, provides a promising framework to ground these state-related changes in the dynamic interactions of reciprocally connected brain areas.Methods
Anxiety states were elicited in healthy participants (n = 19) by exposure to the threat of unpredictable, aversive shocks while undergoing magnetoencephalography. An auditory oddball sequence was presented to measure cortical responses related to deviance detection, and dynamic causal models quantified deviance-related changes in effective connectivity. Participants were also administered alprazolam (double-blinded, placebo-controlled crossover) to determine whether the cortical effects of threat-induced anxiety are reversed by acute anxiolytic treatment.Results
Deviant tones elicited increased auditory cortical responses under threat. Bayesian analyses revealed that hypervigilant responding was best explained by increased postsynaptic gain in primary auditory cortex activity as well as modulation of feedforward, but not feedback, coupling within a temporofrontal cortical network. Increasing inhibitory gamma-aminobutyric acidergic action with alprazolam reduced anxiety and restored feedback modulation within the network.Conclusions
Threat-induced anxiety produced unbalanced feedforward signaling in response to deviations in predicable sensory input. Amplifying ascending sensory prediction error signals may optimize stimulus detection in the face of impending threats. At the same time, diminished descending sensory prediction signals impede perceptual learning and may, therefore, underpin some of the deleterious effects of anxiety on higher-order cognition.Free full text

The un-predictive brain under threat: a neuro-computational account of anxious hypervigilance
Abstract
Background
Anxious hypervigilance is marked by sensitized sensory-perceptual processes and attentional biases to potential danger cues in the environment. How this is realized at the neuro-computational level is unknown, but could clarify the brain mechanisms disrupted in psychiatric conditions such as PTSD. Predictive coding, instantiated by dynamic causal models (DCM), provides a promising framework to ground these state-related changes in the dynamic interactions of reciprocally-connected brain areas.
Methods
Anxiety states were elicited in healthy participants (N=19) by exposure to the threat of unpredictable, aversive shocks while undergoing magnetoencephalography. An auditory oddball sequence was presented to measure cortical responses related to deviance detection, and DCM quantified deviance-related changes in effective connectivity. Participants were also administered alprazolam (double-blinded, placebo-controlled crossover) to determine whether the cortical effects of threat-induced anxiety are reversed by acute anxiolytic treatment.
Results
Deviant tones elicited increased auditory cortical responses under threat. Bayesian analyses revealed that hypervigilant responding was best explained by increased post-synaptic gain in A1 activity as well as modulation of feedforward, but not feedback, coupling within a temporo-frontal cortical network. Increasing inhibitory GABA (γ-aminobutyric acid)-ergic action with alprazolam reduced anxiety and restored feedback modulation within the network.
Conclusions
Threat-induced anxiety produced unbalanced feedforward signalling in response to deviations in predicable sensory input. Amplifying ascending sensory prediction error signals may optimize stimulus detection in the face of impending threats. At the same time, diminished descending sensory prediction signals impede perceptual learning and may, therefore, underpin some of the deleterious effects of anxiety on higher-order cognition.
Anxiety triggers a cautious and vigilant stance toward the environment in novel, ambiguous and uncertain settings (1–2). Such a state of hypervigilance is clearly advantageous in the case of real threats, but when extreme and persistent, it can overwhelm and incapacitate. Indeed, exaggerated sensory-perceptual responding is considered a central component of hyperarousal in PTSD (3), which likely increases distractibility and disrupts higher cognitive processes (4). Experimental work has amassed considerable evidence that anxious hypervigilance takes the form of heightened stimulus-driven processing and biased attention toward threat signals (5–9). For example, the mismatch negativity (MMN), a neuroelectric response to unexpected (oddball) stimulus events, has been shown to be increased in individuals with PTSD (10–11) and by inducing anxiety in psychiatrically healthy individuals (9). A closer look at this MMN effect may provide a useful entry point to further our limited understanding of the brain mechanisms underlying anxious hypervigilance. To provide a more integrative and mechanistic account, we took a first step toward studying anxiety’s effects on the brain network dynamics underlying deviance (or change) detection from the theoretical perspective of predictive coding.
The brain has long been portrayed as a hypothesis tester that anticipates environmental change and modifies its expectations when they are violated (12). Predictive coding, which carries on this idea of active perceptual inference, is a contemporary neuro-computational framework predicated on bidirectional communication within brain networks, where feedback and feedforward signalling embody prediction and prediction error, respectively (13–14). Within this framework, dynamic causal modeling (DCM (15)) has emerged as a powerful method for characterising brain network dynamics (i.e., effective connectivity) underlying noisy empirical data obtained with electroencephalography (EEG) and magnetoencephalography (MEG). DCM has been extensively applied to data from oddball (or mismatch negativity, MMN) paradigms, a simple and elegant procedure for eliciting brain responses to improbable (and therefore, unpredicted) stimuli. Stimulus deviance detection has been consistently shown to involve modulation of both feedforward and feedback connectivity within an extended, hierarchically-organized cortical network (16–18). This empirical evidence, together with neuronal modeling of oddball stimulus processing (19), supports the view that perceptual learning is a Bayesian-like process of updating predictions (i.e., modulation of feedback activity) to minimize future prediction error.
Here we extend this neuro-computational approach to affective neuroscience to offer a novel account of the effects of anxiety on early perceptual processing. Although anxiety-related changes in stimulus deviance responding have been shown previously (9–11), the network dynamics underpinning these cases of hypervigilant responding were not examined. In Cornwell et al. (9), healthy individuals were exposed to a threat of unpredictable shock procedure, a well-validated method for inducing sustained anxiety (20) as demonstrated by a wealth of prior studies (e.g., 21–23). In the present study, we adopted the same experimental strategy of using a MMN paradigm to elicit well-characterized auditory prediction errors under threat of shock to study modulation of intrinsic and extrinsic connectivity during the propagation of prediction errors up the auditory hierarchy.
From the perspective of predictive coding, anxious hypervigilance manifests as increased sensory precision. Computationally, precision quantifies reliability or salience afforded to ascending (sensory) prediction errors, enabling them to update posterior beliefs more rapidly and efficiently (24). Physiologically, this effect is thought to be mediated by neuromodulation of the postsynaptic gain of cells reporting prediction error (e.g., superficial pyramidal cells) (24). Consequently, we predicted that threat-induced anxiety would increase intrinsic gain of responses to prediction errors in early auditory cortex and augment the influence of ascending connections that convey prediction errors, relative to descending connections that convey predictions. In addition, we down-regulated threat-induced anxiety by administering a fast-acting anxiolytic compound (the benzodiazepine alprazolam) before introducing the threat of shock condition using a placebo-controlled crossover design. With this pharmacological manipulation, we sought to determine whether the changes in intrinsic and extrinsic connectivity observed under threat can be reversed by reducing anxiety.
Method and Materials
Subjects
Nineteen healthy participants (7 women, mean age ± SD = 29 ± 7 y) completed two testing MEG sessions and a single MRI session. Target sample size was based on previously published data using the same MEG paradigm (9). All participants received physical and psychiatric exams to ensure their health status and that they were not taking psychoactive medications. They were also screened for metallic implants and other contraindications associated with MRI scans. The procedures were approved by the Combined Neurosciences Institutional Review Board of the National Institutes of Health (ClinicalTrials.gov Identifier: NCT00047853). Informed consent was obtained from each participant prior to testing. Additional participants were tested but excluded from the analyses because of either excessive head movement during the MEG recordings (N = 2) or did not complete both treatment conditions (N = 4).
Drug administration
Participants received a single oral 1 mg dose of alprazolam (Xanax®) or an inactive placebo in a double-blind randomized crossover design. Alprazolam is a well-known and clinically-effective anxiolytic compound that acts as a positive allosteric modulator at GABAA receptors, which in turn increases cortical inhibitory processes (25). Single administration of alprazolam has been shown to be effective in reducing threat-induced anxiety in the laboratory as measured by startle reflex potentiation and subjective report in humans (22). Alprazolam or placebo was administered by a nurse 90–120 min before the MEG recordings. MEG sessions were conducted at least 7 days apart (mean ± SD = 26 ± 15 days). Experimenter blinding was maintained until data collection was completed. Of the 19 participants included in the following analyses, ten participants received alprazolam for the first testing session, and nine participants received placebo for the first testing session.
Threat of shock auditory oddball procedure
The task procedures are similar to those described previously (9). Participants completed two runs in which three pure tones (duration = 100 ms, stimulus onset asynchrony = 600 ms) were presented binaurally at a comfortable loudness through plastic tubes inserted into each ear: a standard frequency tone (1000 Hz) with a 80% probability and two deviant frequency tones (936 Hz, 1064 Hz) with 10% probabilities. Periodically the tone sequences were interrupted by a voice recording indicating one of two contexts: “Shock at any time in the next 30 seconds” (THREAT) and “No shock in the next 30 seconds, you are safe” (SAFE). THREAT and SAFE contexts alternated 10 times in each run with the first context counterbalanced across subjects. Participants listened to the tones without responding and were explicitly told that there was no link between the tones and timing of shocks during THREAT contexts. One shock was administered to the wrist at the end of the final THREAT context of the first run during the first testing session and one at the end of the first THREAT context of the second run during the second testing session. Administering shocks sparingly has been shown to be highly effective in inducing sustained anticipatory anxiety (9, 26), in part because it reduces habituation to a moderately-aversive stimulus. Using a constant current stimulator, shock level was set on an individual basis before the MEG recordings using a work-up procedure (1–3 sample shocks) to identify a moderately-uncomfortable physical intensity (3–5 mA). Subjective anxiety was reported for THREAT and SAFE contexts after each run on a 0–10 scale (“no anxiety” to “highly anxious”). There was a 2–5 m break in between runs.
MEG acquisition
Whole-head MEG recordings were made following placebo or alprazolam administration in two separate sessions with a CTF-OMEGA 275-channel magnetometer (VSM MedTech Ltd., Canada) in a magnetically-shielded room (Vacuumschmelze, Germany). Gradiometer data were digitized at 1200 Hz across a bandwidth of 0–300 Hz (notch filter = 60 Hz) with synthetic 3rd gradient balancing enabled for active noise cancellation. Continuous recordings of relative head position were made with three energized coils attached to the nasion and left and right preauricular fiducial sites. Participants showing over 6.5 mm displacement from the start of any recording were excluded from analysis. A high-resolution anatomical T1-weighted MRI was obtained in a separate session for source-space modeling.
Event-related adaptive beamformer imaging
We followed a similar analytic approach as described previously (9). Specifically, we used a variant of synthetic aperture magnetometry (SAM) for mapping evoked signals across the brain (SAMerf) (27). To increase signal-to-noise, SAMerf uses time-domain averaging of reconstructed source activity after each beamformer (i.e., spatial filter) is specified from the raw sensor covariance. For each run within a recording session, covariance matrices were generated from a broad time-frequency window (2–30 Hz, 0–500 ms relative to tone onset), combining 100 deviant and 100 standard tone epochs, for THREAT and SAFE contexts separately (For two participants, we discarded 10% and 40% of the epochs from one run, respectively, due to significant head movements in the latter part of the recording). For both contexts, we included only standard tone epochs that immediately preceded deviant tone epochs for a balanced comparison. Beamformer coefficients were calculated at 6.5 mm steps across source space using the vector lead-field formulation of Sekihara and colleagues (28) with a multiple, local spheres head model derived from individual anatomical MRIs. Data were projected through each beamformer and averaged in the time-domain. Source power was integrated over 100–250 ms post-stimulus onset to compare evoked responses between tones (i.e., deviant/standard). Source images for each context were averaged across the two runs within each session.
Using Analysis of Functional NeuroImages (AFNI) (29), MRIs and beamformer source images were warped to standardized Talairach space. Group analyses were constrained to a priori regions of interest (ROIs). Spherical masks (7-mm radii) were centered at coordinates (in MNI space) obtained from Garrido et al. (16) and voxels contained within each mask were averaged and extracted to obtain evoked power estimates. Six ROIs were studied (Figure 1C): bilateral A1, bilateral superior temporal gyrus (STG) and bilateral inferior frontal gyrus (IFG). An omnibus MANOVA was first conducted to determine any main or interactional effects among the three factors (ROI × Treatment × Context). Follow-up 2 × 2 repeated-measures ANOVAs were carried out for each ROI and evaluated using a modified-Bonferroni correction procedure that controlled family-wise error at α < .05 (30).
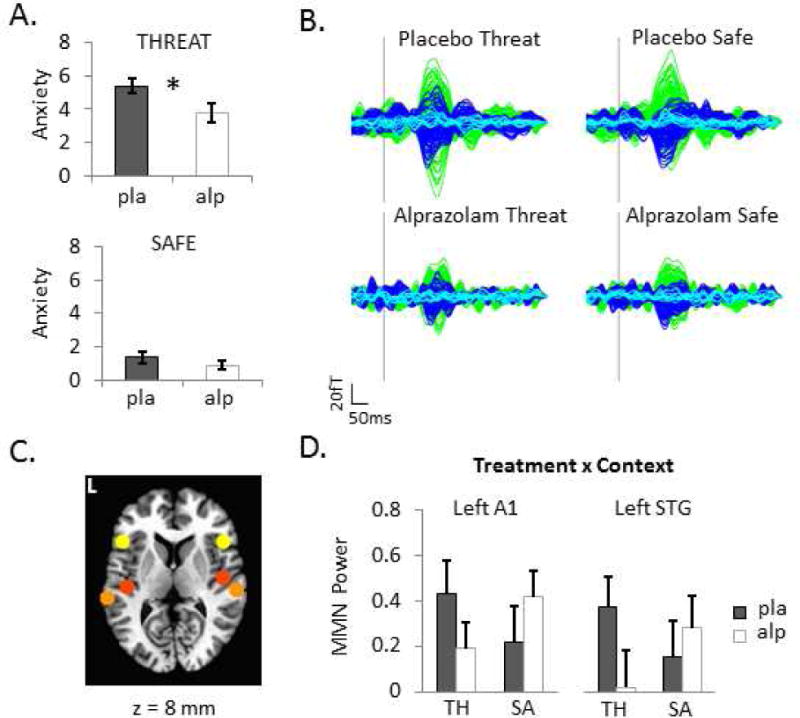
A. Retrospective anxiety reports for each context after receiving placebo and 1mg alprazolam on a 0–10 scale. B. Group-averaged difference waves (deviant minus standard tones) exhibiting the magnetic mismatch negativity (MMNm) response by Treatment and Context. Axial gradiometer traces are color coded by hemisphere (green = left, dark blue = right, light blue = midline). C. Six a priori regions of interest (ROIs) to which event-related beamformer source analyses were constrained. Coordinates (MNI space) for each spherical ROI depicted on the standard MRI are as follows: left IFG (yellow) = −46, 20, 8 mm; right IFG = 46, 20, 8 mm; left A1 (red) = −42, −22, 7 mm; right A1 = 46, −14, 8; left STG (orange) = −61, −32, 8 mm; right STG = 59, −25, 8 mm. D. Left primary auditory cortex (A1) and left superior temporal gyrus (STG) ROIs showing significant Treatment by Context interactions (p < .05, corrected). Bar graph shows group-averaged evoked power estimates (log10-transformed deviant/standard power ratio or ‘MMN power’) integrated over 100–250 ms post-stimulus onset. Error bars are s.e.m. pla=placebo, alp=alprazolam, TH=THREAT, SA=SAFE.
Dynamic causal modeling
The theoretical and conceptual basis of DCM has been extensively described and evaluated in previous studies (e.g., 15, 31). For M/EEG data, DCM is a generative model of evoked responses that is useful to test hypotheses about directional coupling or effective connectivity among brain areas (32). Each source is modeled as a simplified neural mass comprised of three distinct (excitatory and inhibitory) neuronal populations dynamically coupled (33). Bayesian model inversion entails quantifying model evidence (favoring fit accuracy and penalizing complexity) and estimating coupling parameters. Data preprocessing and modeling was implemented in Statistical Parametric Mapping (SPM v12) and followed the same source model and sequence outlined in Garrido et al. (16). Briefly, raw data were high pass filtered (.61 Hz cutoff), downsampled (200 Hz) and low pass filtered (40 Hz cutoff) before concatenating runs within each session and robust averaging epochs for each stimulus separately. A low-pass filter (40 Hz cutoff) was re-applied to the averaged data to remove high-frequency artifacts that can be introduced by robust averaging. Data were reduced to eight spatial modes for the post-stimulus period between 0–250ms before model inversion, and a multiple, local spheres volume conductor was used to calculate the forward solution. We modeled the experimental effects of the threat factor and differences in responses to deviant and standard tones in terms of changes in intrinsic (within source) and extrinsic (between sources) coupling within the DCM. Specifically, we used a 2 × 2 factorial design to model changes (i.e., log scaling) in connectivity in each of the four cells. This enabled us to compare different models of the experimental effects in terms of condition-specific changes in different combinations of connectivity.
For each subject, 16 DCMs were inverted per treatment condition. In addition to the eight models with 6-node symmetric architectures (Supplementary Figure S1), a complementary set of eight DCMs with 5-node asymmetric architectures that excluded the left IFG were also tested. Although previous evidence favors the latter architecture (16), previous whole-brain event-related beamformer analyses (9) hinted at the possibility that with the inclusion of the threat of shock, 6-node symmetrical architectures may perform better. The models differed only with respect to whether the strength of between-source (extrinsic) or within-A1 (intrinsic) coupling was free to vary as a function of each stimulus (2 contexts × 2 tone stimuli). Intrinsic coupling modulation represents changes in the overall excitability of a source independently of any extrinsic modulation (e.g., local adaptation) (18). In addition to feedforward, feedback and intrinsic A1 connections, we included lateral connections between bilateral A1 and bilateral STG (not depicted in figures), but these remained fixed across the models.
Model space was explored using a random-effects approach at the family-level (34). In the first step, we directly compared 5-node architectures to 6-node architectures (Supplementary Figure S2). Within the winning family, we proceeded with additional partitions of the model subspace to determine separately whether modulation (vs. no modulation) of each type of connection improves model fit. For example to determine whether feedback modulation improves model fit, we compared a family of models with no feedback modulation (‘null’, ‘f’, ‘i’, and ‘f-i’ in Supplementary Figure S1) to a family of models with feedback modulation (‘b’, ‘fb’, ‘b-i’, and ‘fb-i’). To attempt to replicate the modeling results, we followed the exact same preprocessing and modeling steps for the previously-collected data from Cornwell et al. (9).
Posterior connectivity estimates were obtained by Bayesian model averaging (34) to allow for closer inspection of connectivity changes within THREAT versus SAFE contexts. Repeated-measures ANOVAs tested for Treatment × Context × Stimulus interactions starting from an omnibus analysis that included one additional factor (Hemisphere) for intrinsic A1 connections or two additional factors (Hemisphere and Hierarchical level) for feedforward and feedback connections. Residual values were inspected to test model assumptions (e.g., heteroscedasticity, outliers), but no clear violations were observed. Follow up interaction contrasts and simple effect analyses were conducted only for significant interactions (α < .05) to protect against Type-I error inflation.
Results
Contextual and pharmacological manipulation of anxiety
Participants were exposed to two conditions during MEG scanning, a THREAT context in which aversive shocks could be delivered unpredictably without immediate warning and a SAFE context in which no shocks could be delivered. MEG scanning commenced approximately 90–120 m after participants had been orally administered 1 mg of the benzodiazepine alprazolam or an inactive placebo on two separate occasions (double-blinded). As expected, participants reported feeling less anxious during THREAT, but no different during SAFE, following alprazolam administration compared to placebo (Figure 1A; FTreatment-by-Context(1,18) = 5.5, p = .03). Thus, threat-induced anxiety was dampened by anxiolytic treatment.
Threat of shock heightens auditory cortical responses to deviant stimuli
We perturbed neural dynamics underlying these high and low anxiety states with a passive auditory oddball paradigm and measured stimulus deviance-related activity with whole-head MEG. Deviance-related activity is operationally defined as the difference between responses to deviant and standard stimuli, revealing the classic MMN (and its neuromagnetic counterpart, MMNm) (35). Time-locked averaging of raw sensor data, followed by subtraction of the averaged waveforms, revealed robust MMNm responses between 100–250 ms during THREAT and SAFE contexts, which were noticeably attenuated after receiving alprazolam (Figure 1B).
These waveforms were explored further in source space with event-related adaptive beamforming (SAMerf) (27), a method for computing voxel-wise estimates of evoked power. Spherical masks (7-mm radii) were used to extract mean log10-transformed power ratios (deviant/standard) from each of the six a priori ROIs (Figure 1C). An omnibus MANOVA revealed a significant three-way (region × treatment × context) interaction, Wilks Λ = .48, F5,14 = 3.01, p = .047. Follow-up repeated-measures ANOVAs (modified-Bonferroni corrected) for individual ROIs revealed a significant interaction in left A1 and left STG, FTreatment-by-Context-by-Stimulus(1,18) = 9.25, p = .007 and FTreatment-by-Context-by-Stimulus(1,18) = 8.10, p = .011, respectively, both showing a larger reduction in deviance-related activity after alprazolam administration relative to placebo during THREAT compared to SAFE contexts (Figure 1D). No other ROIs exhibited significant interactions or main effects.
Anxiety causes unbalanced feedforward processing of deviant stimuli
With DCM, we explored directional coupling among key temporal and frontal cortical nodes that responded to stimulus deviance. Eight network models were compared having the same 6-node hierarchical structure encompassing bilateral A1, bilateral STG and bilateral IFG (Supplementary Figures S1–S2). Source location priors were taken from Garrido et al. (16) (Figure 1C). With three sequential partitions of the 8-model space, we made family-level inferences on whether modulation of feedforward, feedback and intrinsic A1 connections improved model fit for each treatment condition (full model space description in Supplementary Figure S1).
In the placebo condition, models that allowed for intrinsic A1 modulation clearly outperformed those that did not, as did those specifying modulation of feedforward connections (Figure 2A). However, feedback changes did not increase model evidence, meaning that responses to stimulus deviance could be adequately explained by enabling of, and only of, forward or ascending connections (i.e., an unbalanced feedforward model). This finding notably diverges from previous modeling results that have consistently shown that stimulus deviance drives changes in feedforward and feedback coupling as well as increased intrinsic gain in A1 nodes under a wide range of affectively-neutral conditions (16, 36–37). By contrast, but in line with these previous DCM results, the best fitting models in the alprazolam treatment condition were those that allowed for changes in feedforward and feedback connections (Figure 2B). Thus, by reducing anxiety pharmacologically, we found that the normal balance in extrinsic modulation was restored even with the threat of shock still looming over participants.
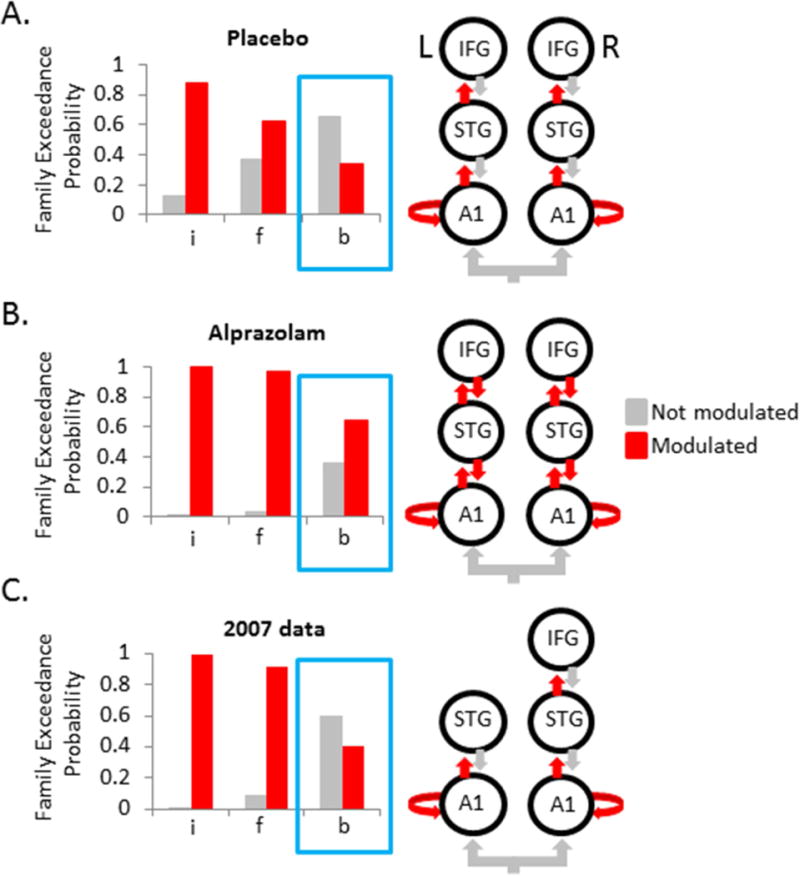
A. Family-level random-effects Bayesian analyses revealed that after placebo treatment best fitting models were those that allowed modulation of intrinsic A1 (i) and feedforward (f) connectivity but not feedback (b) connectivity. B. After alprazolam treatment, best fitting models allowed modulation of intrinsic A1 and balanced feedforward/feedback connectivity. C. The lack of feedback modulation in the placebo condition was replicated in an independent dataset (9) using a 5-node architecture. A1 = primary auditory cortex, IFG = inferior frontal gyrus, STG = superior temporal gyrus.
To bolster confidence in this anxiety-related disruption in feedback coupling, we attempted to replicate the findings in an independent dataset. Specifically, we fit the same set of DCMs to previously-collected data (N=16) from Cornwell et al. (9). That study used identical anxiety-induction and stimulus procedures without a pharmacological manipulation. For those data, we used a 5-node asymmetrical network that excluded left IFG based on a preliminary result indicating that these models fit the data better than models with a 6-node symmetrical network (Supplementary Figure S2). The same pattern observed in the placebo condition emerged in this second dataset with evidence being highest for models with modulation of intrinsic A1 and feedforward connections, but not feedback connections (Figure 2C). This was also true when the inferior set of 6-node models was used for this second dataset (data not shown), reinforcing the link between threat-induced anxiety and diminished feedback connectivity.
Right frontal and temporal cortical nodes show reduced feedback coupling
To compare coupling gain estimates between THREAT and SAFE contexts for each treatment condition, posterior connectivity parameters were obtained by Bayesian model averaging and analysed with classical parametric statistics (28). For feedback connections, a repeated-measures ANOVA revealed that alprazolam increased feedback coupling to deviant tones during THREAT, particularly between right IFG and right STG (FTreatment-by-Context-by-Stimulus(1,18) = 4.69, p = .04, Figure 3). This finding lends further support to the ‘de-coupling’ of feedback mechanisms (18% reduction, on average) in response to stimulus deviance in a threat-induced anxiety state, which is then restored by anxiolytic treatment. Moreover, alprazolam reduced intrinsic gain in bilateral A1 to deviant tones under THREAT (FTreatment-by-Context-by-Stimulus(1,18) = 4.89, p = .04; Figure 4), suggesting that alprazolam also attenuates the anxiety-related boost (16% gain increase, on average) in primary auditory cortical excitability to stimulus deviance. Feedforward connectivity parameters did not vary as a function of treatment.
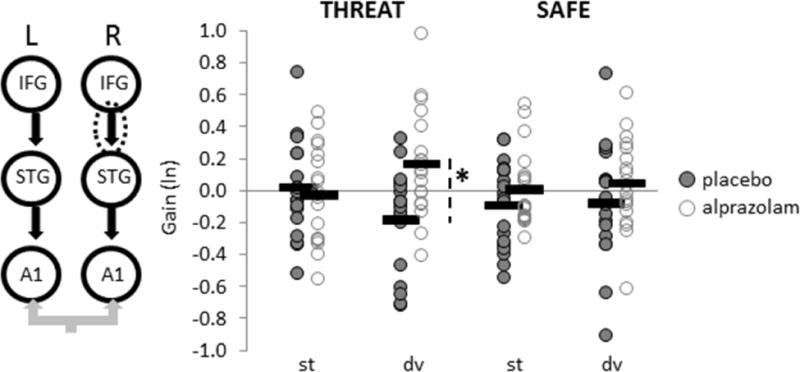
Feedback connectivity parameters obtained by Bayesian model averaging are graphed by treatment, context and stimulus for the right fronto-temporal connection (circled). Coupling gains for each participant (circles) and group means (bars) are displayed. dv = deviant tone, st = standard tone, *p=.006
Discussion
By exposing participants in a threat-induced anxiety state to simple auditory oddball stimuli, we observed a radical change in network dynamics underlying early perceptual processing. Within a DCM analytic framework, Bayesian model comparisons revealed that deviant stimulus responding under threat of shock could be adequately explained by changes only in intrinsic A1 gain and feedforward signalling within a bilateral temporo-frontal cortical network. In other words, models that allowed for feedback changes did not increase model evidence over simpler models that did not, suggesting that the balance in reciprocal network communication was temporarily skewed by anxiety states. This anxiety-induced transformation to a biased feedforward system was replicated in a set of previously-collected data and was reversed pharmacologically by an anxiolytic compound. It is worth emphasizing that, in previous DCM studies of oddball stimulus processing, a balanced feedforward/feedback network has consistently emerged with the highest model evidence, even when potent pharmacological manipulations were used (e.g., ketamine (37)) or vegetative patients were tested (36). Thus, although only a limited set of models were evaluated here, the unique effect of anxiety states emerged from within the same model space explored extensively in prior studies.
These results point to a potential neuro-computational link between anxiety’s adaptive and maladaptive cognitive effects. Predictive coding postulates that perceptual learning emerges from a complex interplay of feedforward and feedback signalling (13–14). By amplifying prediction error through increased primary cortical excitability and reduced feedback modulation from prefrontal cortices, sensory-perceptual processes may become tightly coupled to local stimulus variations, supporting rapid, but relatively indiscriminate responding in threatening situations. Thus, sensitivity becomes favored over specificity in an anxiety state (8), streamlining the link between stimulus detection and action to promote self-preservation. However, without updating sensory predictions through feedback signalling, higher-order stimulus patterns that emerge at longer timescales may not be properly encoded (i.e., stimulus transition probabilities that emerge after two or more occurrences of the deviant tone), leading to impaired perceptual learning. This could have pervasive, downstream effects on cognitive processes that are predicated on more elaborative perceptual processing and controlled behavioral responding, including discriminative Pavlovian fear conditioning (38), working memory (39–40) and response inhibition (41), all of which show evidence of being negatively impacted by high anxiety.
Although we did not study a patient population, the transient effects of threat of unpredictable shocks in psychiatrically healthy individuals are thought to capture essential features of pathological anxiety (42–43). This is supported by extensive evidence that clinically anxious populations are especially sensitive to unpredictable (as opposed to predictable) shock conditions (44–45) and that anxiolytic compounds, including benzodiazepines and selective serotonin re-update inhibitors, are effective at reducing anxiety-potentiated startle reactivity during sustained periods of unpredictable threat (21, 46). Accordingly, our translational approach is consistent with a dimensional perspective of psychiatric disorders (47) that aims to identify underlying mechanisms that range from normal to abnormal from which we can make predictions for future studies in clinically anxious or traumatized individuals. For instance, a similar transformation toward biased feedforward processing could underlie the persistent state of hypervigilance in patients diagnosed with PTSD. This would explain the elevated MMN that has been observed in these patients under ostensibly benign (i.e., no threat) experimental conditions (10–11) together with the phenomenology of feeling generally threatened in PTSD (48). Recent evidence of an elevated MMN in panic disorder may reflect a similar alteration in network dynamics (49).
Finally, these findings present a potential neurobiological target for the development of more selective therapeutics to reduce anxious hypervigilance and improve cognition. As a first step, we showed that acute administration of a well-established, rapid-acting anxiolytic compound effectively normalized anxiety-induced sensitization of sensory-perceptual processing by restoring the balance in feedforward and feedback signalling. Thus, boosting GABAergic signalling with a benzodiazepine provides one route by which to temporarily increase inhibitory tone and counteract heightened stimulus-driven responding in threat-induced anxiety states (50). More preferable to this short-term fix would be to selectively target the lasting neuromodulatory (e.g., noradrenergic) influences on cortical excitability and hypervigilance that may underlie chronic anxiety and stress (51). Nevertheless, by showing that brain network dynamics underlying hypervigilant responding can be altered pharmacologically, we have laid out a noninvasive assay of synaptic neuromodulation that might provide a sensitive means for testing novel compounds targeting the implicit pathophysiology underlying anxiety disorders.
Acknowledgments
This work was funded in part by the Intramural Research Program of the National Institute of Mental Health (NIMH, ZIAMH002798; Protocol 02-M-0321; NCT00047853) and a NARSAD Young Investigator Award from the Brain and Behavior Research Fund (B.R.C). M.I.G. acknowledges a University of Queensland Fellowship (2016000071). Thank you to Lynne Liebermann for assistance with data collection, to members of the NIMH MEG Core Facility for quality assurance and troubleshooting and to the nursing staff at the NIH Clinical Center Outpatient Clinic for drug administration and patient monitoring.
Footnotes
Publisher's Disclaimer: This is a PDF file of an unedited manuscript that has been accepted for publication. As a service to our customers we are providing this early version of the manuscript. The manuscript will undergo copyediting, typesetting, and review of the resulting proof before it is published in its final citable form. Please note that during the production process errors may be discovered which could affect the content, and all legal disclaimers that apply to the journal pertain.
ClinicalTrials.gov Identifier: NCT00047853
Financial Disclosures
The authors report no biomedical financial interests or potential conflicts of interest.
Supplementary Material
Supplementary information is available at Biological Psychiatry’s website.
References
Full text links
Read article at publisher's site: https://doi.org/10.1016/j.biopsych.2017.06.031
Read article for free, from open access legal sources, via Unpaywall:
https://europepmc.org/articles/pmc5584579?pdf=render
Citations & impact
Impact metrics
Article citations
Exploring the use of visual predictions in social scenarios while under anticipatory threat.
Sci Rep, 14(1):10913, 13 May 2024
Cited by: 0 articles | PMID: 38740937 | PMCID: PMC11091092
Pre-scan state anxiety is associated with greater right amygdala-hippocampal response to fearful versus happy faces among trait-anxious Latina girls.
BMC Psychiatry, 24(1):1, 02 Jan 2024
Cited by: 1 article | PMID: 38167015 | PMCID: PMC10759434
Time-resolved dynamic computational modeling of human EEG recordings reveals gradients of generative mechanisms for the MMN response.
PLoS Comput Biol, 19(12):e1010557, 13 Dec 2023
Cited by: 0 articles | PMID: 38091350 | PMCID: PMC10752554
A neural oscillatory signature of sustained anxiety.
Cogn Affect Behav Neurosci, 23(6):1534-1544, 25 Oct 2023
Cited by: 1 article | PMID: 37880568 | PMCID: PMC10684633
Trait and State Anxiety Effects on Mismatch Negativity and Sensory Gating Event-Related Potentials.
Brain Sci, 13(10):1421, 07 Oct 2023
Cited by: 1 article | PMID: 37891790 | PMCID: PMC10605251
Go to all (30) article citations
Data
Data behind the article
This data has been text mined from the article, or deposited into data resources.
BioStudies: supplemental material and supporting data
Clinical Trials
- (2 citations) ClinicalTrials.gov - NCT00047853
Similar Articles
To arrive at the top five similar articles we use a word-weighted algorithm to compare words from the Title and Abstract of each citation.
Neural responses to auditory stimulus deviance under threat of electric shock revealed by spatially-filtered magnetoencephalography.
Neuroimage, 37(1):282-289, 18 May 2007
Cited by: 70 articles | PMID: 17566766 | PMCID: PMC2717627
Benzodiazepines counteract rostral anterior cingulate cortex activation induced by cholecystokinin-tetrapeptide in humans.
Biol Psychiatry, 73(4):337-344, 08 Oct 2012
Cited by: 9 articles | PMID: 23059050
Auditory perceptual learning is not affected by anticipatory anxiety in the healthy population except for highly anxious individuals: EEG evidence.
Clin Neurophysiol, 130(7):1135-1143, 27 Apr 2019
Cited by: 4 articles | PMID: 31085447
Exploring the function of selective attention and hypervigilance for threat in anxiety.
Clin Psychol Rev, 34(1):1-13, 05 Nov 2013
Cited by: 62 articles | PMID: 24286750
Review
Funding
Funders who supported this work.
Brain and Behavior Research Foundation
Core
Intramural NIH HHS (2)
Grant ID: Z01 MH002798-06
Grant ID: ZIA MH002798
NIH Clinical Center
National Alliance for Research on Schizophrenia and Depression
National Institute of Mental Health (3)
Grant ID: 02-M-0321
Grant ID: NCT00047853
Grant ID: ZIAMH002798
National Institutes of Health
Past Global Changes (1)
Grant ID: https://clinicaltrials.gov/ct2/show/NCT00047853
University of Queensland (1)
Grant ID: 2016000071