Abstract
Background
Timely influenza surveillance is important to monitor influenza epidemics.Objectives
(i) To calculate the epidemic threshold for influenza-like illness (ILI) and acute respiratory infections (ARI) in 19 countries, as well as the thresholds for different levels of intensity. (ii) To evaluate the performance of these thresholds.Methods
The moving epidemic method (MEM) has been developed to determine the baseline influenza activity and an epidemic threshold. False alerts, detection lags and timeliness of the detection of epidemics were calculated. The performance was evaluated using a cross-validation procedure.Results
The overall sensitivity of the MEM threshold was 71·8% and the specificity was 95·5%. The median of the timeliness was 1 week (range: 0-4·5).Conclusions
The method produced a robust and specific signal to detect influenza epidemics. The good balance between the sensitivity and specificity of the epidemic threshold to detect seasonal epidemics and avoid false alerts has advantages for public health purposes. This method may serve as standard to define the start of the annual influenza epidemic in countries in Europe.Free full text

Influenza surveillance in Europe: establishing epidemic thresholds by the Moving Epidemic Method
Abstract
Please cite this paper as: Vega et al. (2012) Influenza surveillance in Europe: establishing epidemic thresholds by the moving epidemic method. Influenza and Other Respiratory Viruses 7(4), 546–558.
Background Timely influenza surveillance is important to monitor influenza epidemics.
Objectives (i) To calculate the epidemic threshold for influenza‐like illness (ILI) and acute respiratory infections (ARI) in 19 countries, as well as the thresholds for different levels of intensity. (ii) To evaluate the performance of these thresholds.
Methods The moving epidemic method (MEM) has been developed to determine the baseline influenza activity and an epidemic threshold. False alerts, detection lags and timeliness of the detection of epidemics were calculated. The performance was evaluated using a cross‐validation procedure.
Results The overall sensitivity of the MEM threshold was 71·8% and the specificity was 95·5%. The median of the timeliness was 1
week (range: 0–4·5).
Conclusions The method produced a robust and specific signal to detect influenza epidemics. The good balance between the sensitivity and specificity of the epidemic threshold to detect seasonal epidemics and avoid false alerts has advantages for public health purposes. This method may serve as standard to define the start of the annual influenza epidemic in countries in Europe.
Introduction
Influenza causes respiratory infections in humans, leads to increased consultations in general practice and is an important global cause of hospital admissions and mortality. 1 , 2 While attack rates are highest among children, mortality rates are typically highest in elderly populations and among persons suffering from chronic and underlying conditions. 3 , 4 Influenza produces substantial direct healthcare costs, and even greater indirect costs associated with the loss of both labour and school days. 5 , 6 , 7 Consequently, influenza is an important burden on human health and a challenge for health services, making it a priority for surveillance activities, which can help to better prioritise the efforts in prevention and control.
Objectives of influenza surveillance include monitoring annual epidemics and detecting and characterising circulating viruses. 8 Additionally, timely detection of the start of the seasonal epidemic is needed to alert health services and to mitigate morbidity, mortality and economic costs. Lessons from the past human outbreak of an avian influenza A(H5N1) virus infection in Hong Kong in 1997 9 and the recent experience of the influenza A(H1N1)pdm09 pandemic declared by the WHO on June 2009 10 have intensified the efforts of international and national health authorities to further enhance surveillance systems to provide a more standard and accurate estimation of influenza dynamics at the local and country level.
Although influenza can appear sporadically in populations, influenza epidemics usually occur seasonally in temperate regions of the world, typically during autumn and winter months, with a great variation in the epidemiological characteristics. The onset, duration, intensity and geographical spread of influenza activity are unpredictable and depend on multiple factors such as the characteristics of virus strains, population susceptibility and climatic and environmental factors. 11
High‐quality influenza surveillance can inform vaccine decision‐making and help policy‐makers prioritise resources for persons most at risk of severe outcomes. Timely detection of the onset of epidemics has the potential to inform empirical antiviral therapy for hospitalised patients with suspected influenza, 12 which can further reduce the health and economic burden of influenza. Accurately identifying the start of influenza epidemic requires routine and timely data collection and a standard procedure to analyse these data continuously.
A wide variety of statistical methods are available for defining influenza epidemics, detecting influenza outbreaks, and to assess the intensity of epidemics. In the last two decades, Serfling‐based models and dynamic linear models requiring only recent data 13 , 14 , 15 , 16 have been used for this purpose. Most of these models are based on time series methods, and they often use subjective criteria, such as a discretional virus isolations rate or manual removal of epidemic peaks, to establish a non‐epidemic period. 17 , 18 , 19 In the United States, for instance, periods with <10% of specimens testing positive for influenza are considered non‐epidemics periods 20 and have been used to establish national and regional baselines. Cowling et al. 21 estimated the sensitivity, specificity and the timeliness of an influenza alert, taking the period where the isolation rate was above a 20% as epidemic. Regardless of the method used, some important issues remain unresolved such as the evaluation of the validity of a given method in the absence of a gold standard or how to handle the early warning signals from statistical surveillance systems. 22
Nowadays, influenza sentinel networks exist at regional and national levels in most of Europe, optimising surveillance through the integration of epidemiological and virological data with high sensitivity, specificity, accuracy and timeliness. 23 However, these systems, which are based on the epidemiological surveillance of patients attending primary healthcare services, lack clear criteria to detect the start of the influenza epidemic and to assess the intensity and spread of influenza. Attempts at searching for a standard epidemic threshold have not satisfied all expectations 24 , 25 or are difficult to apply worldwide, because of the complexity of the mathematical modelling and the variety of criteria to calculate the baseline or epidemic threshold. An automated method using simple data is needed to define the onset of the seasonal epidemic and to assess the intensity of influenza activity.
A model to detect seasonal epidemics, to monitor their intensity and to compare their spatial spread has been used in Spain since 2003 with reliable results in several European countries. 26 , 27 A modified version of this approach named MEM (Moving Epidemic Method)’ is being implemented by the World Health Organisation and the European Centres for Disease Prevention and Control to monitor influenza circulation in the European countries. The main purpose of the method is to define the baseline influenza activity in historical data and to establish an epidemic threshold above which the weekly rates are considered to be in the epidemic period. In this study, we describe the epidemic threshold, three thresholds for different levels of intensity calculated by MEM, the modelled 2009–2010 season, and we evaluate the performance of the epidemic threshold for detecting the start of the influenza epidemic in 19 European countries.
Material and methods
Selected data
Weekly aggregate numbers of influenza‐like illness (ILI) and acute respiratory infections (ARI) for 19 European countries or regions were included in the analysis. Romania provided both ILI and ARI data independently. Most countries reported population‐based rates. The data sets were generally collected through sentinel surveillance networks, 28 , 29 but four countries provided data from nationwide or a combination of notification systems. Castilla y León, a large region in the upper centre of Spain, has also been particularly included because data are provided by one unique sentinel network with homogeneous and standard methodological criteria since 1996.
Countries were selected according to the following criteria:
- 1
ILI was registered independently from ARI.
- 2
Historical data were available for five or more surveillance seasons.
- 3
Weekly rates were estimated at least for 33
weeks in every season, from week 40 of 1
year to the week 20 of the following year. For the 2009–2010, the season the period was extended from the 30th week of 2009 to the 20th week of 2010 because of the circulation of the influenza A (H1N1)pdm09 virus.
- 4
The number of sites reporting data and the population under surveillance remained stable over time.
Data quality control
Missing values in weekly rates at the beginning or at the end of the surveillance period did not affect the method and were excluded from calculations. Missing weekly data during the surveillance period were imputed with a smooth regression over the full season’s data using a small window parameter. 30
The Moving Epidemic Method
The purpose of the MEM is to model influenza epidemics based upon historical data from a specific country or region. The method has three main steps: First, the length, start and the end of the annual epidemics are determined, splitting the season in three periods: a pre‐epidemic, an epidemic and a post‐epidemic period. Secondly, the baseline and the epidemic thresholds are calculated using the pre‐epidemic and post‐epidemic values of historical seasons. Finally, thresholds for different levels of intensity are computed.
In the first step, for each season separately, the length of the epidemic period is estimated as the minimum number of consecutive weeks with the maximum accumulated rates percentage (MAP).
The MAP curve draws the maximum cumulated rate for a period of a given length r, expressed as percentage of the total rate of the jth season.
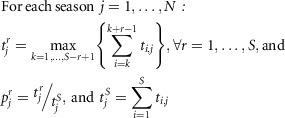
Where t i,j is the ith rate of the jth season, N is the number of seasons, and S is the number of surveillance weeks per season.
Then the MAP curve is smoothed, using a smoothing regression (
over r), selecting automatically the window parameter.
30
,
31
The optimum number of weeks for each season is determined calculating the increment in the percentage from one number of weeks to the next one.

It is represented as the slope of the MAP curve, a dispersion graph of over r.
When this increment is lower than the predefined criteria, the optimum is found.

Where is the optimum duration of the jth season and δ an inner parameter that depends on the country, ranges from 2% to 4% and maximises sensitivity and specificity.
Once the optimum duration of the epidemic is found, the timing is determined by the value k
* that maximises the expression used to calculate the .

The epidemic starts on week k
* and ends on week .
Weeks before the epidemic period are the pre‐epidemic period (1 to k
*−1), and weeks after are the post‐epidemic period ( to S).
In the second step, the baseline and threshold using pre‐epidemic values of historical seasons are calculated. The baseline is the arithmetic mean of all pre‐epidemic rates of all historical seasons (pooled together as a single sample). The post‐epidemic baseline is the mean of all post‐epidemic rates.
To calculate the threshold, only a set of pre‐epidemic values is considered. For each season, the highest n values from the pre‐epidemic period are taken, where n=
30/number of seasons.

Where t (i),j is the ith pre‐epidemic highest rate.
With all these n×
N values, a one‐tailed point 95% confidence interval is calculated.

Where z
0.05 is the 5% percentile of a standard normal distribution, and S
t the standard deviation of the sample, and .is the arithmetic mean of the selected pre‐epidemic values.

The upper limit of the confidence interval is the epidemic threshold.

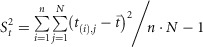
Additionally, a post‐epidemic threshold is calculated using the same procedure.
Thresholds for different levels of intensity are calculated using the n highest values of each epidemic period to build a sample of n×
N rates. With the sample, the geometric mean and 50%, 90% and 95% one‐sided confidence intervals are calculated. These upper limits are the threshold for the different levels of intensity of the epidemic.
An R language implementation of the algorithm is publicly available through an R library called ‘mem’ that can be downloaded for free at The Comprehensive R Archive Network ‐CRAN‐ in http://www.r‐project.org/.
Descriptive data analysis
For each country, we describe the thresholds (pre‐epidemic and post‐epidemic) based on the historical data from the beginning of surveillance in the country up to the end of the 2008–2009 season, as well as the thresholds for different levels of intensity. We compared these thresholds with the modelled periods of the 2009–2010 season (pre‐epidemic, epidemic and post‐epidemic). Rates in the pre‐epidemic period were expected to be lower than the pre‐epidemic threshold rate, while rates within the epidemic period are expected to be higher. Finally, rates in the post‐epidemic period were expected to be lower than the post‐epidemic threshold rate. We calculated the number of false alerts, the detection lag and timeliness of the 2009–2010 season.
The following definitions were used:
- 1
Alert week: The first week of the 2009–2010 season with a rate above the pre‐epidemic threshold.
- 2
False alert: A weekly rate from the modelled pre‐epidemic period of the 2009–2010 season which is above the pre‐epidemic threshold.
- 3
Detection lag: The delay between the start of the epidemic period modelled by MEM for the 2009–2010 season and the alert week. The lag is zero if there is no delay.
- 4
Timeliness: A positive value representing the number of weeks between the alert week and the first week of the epidemic period modelled by MEM for the 2009–2010 season, either the alert week precedes the epidemic period or vice versa.
Cross‐validation analysis
The accuracy of the method was evaluated using a cross‐validation procedure. For each country, each single season was extracted from the historical records to be used as the target season. MEM calculated the beginning and the end of the epidemic period of the target season. On the basis of the remaining seasons, excluding the target season, MEM calculated the pre‐ and post‐epidemic thresholds. This procedure was repeated as often as the numbers of seasons available per country.
To assess the quality of the method, the rates of the target season inside and outside of the epidemic period determined by MEM were compared to the thresholds calculated using all historical information but the target season. To measure the performance of the threshold, the following statistics and definitions were used:
- 1
Sensitivity: The number of epidemic weeks above the pre‐epidemic threshold (before the peak) and above the post‐epidemic threshold (after the peak) divided by the number of epidemic weeks (epidemic length).
- 2
Specificity: The number of non‐epidemic weeks below the pre‐epidemic threshold (before the peak) and below the post‐epidemic threshold (after the peak) divided by the number of non‐epidemic weeks.
- 3
Positive predictive value (PPV): The number of epidemic weeks above the threshold divided by the number of weeks above the threshold.
- 4
Negative predictive value (NPV): The number of non‐epidemic weeks below the threshold divided by the number of weeks below the threshold.
- 5
Median timeliness: Median of the seasons’ timeliness
This process was undertaken for each data set provided by the countries. Statistics were calculated as overall measures of the performance of the MEM, and also separately with regard to its application to ILI and ARI surveillance data.
Influenza‐like illness and ARI mean statistics were compared using the two‐sample Student’s t‐test, except for timeliness, where the Wilcoxon rank sum test was used. P‐values and confidence intervals of the difference in means between ARI and ILI were provided. The R language was used for these calculations.
Results
The modelled 2009–2010 season
Data from 19 countries or regions, with a total of 20 data sets (15 ILI and 5 ARI), were modelled. Most of the data came from sentinel surveillance systems, based in the voluntary notification of consultations in primary care, and expressed as cases per 100000 population (Table 1). The number of seasons used in each country varied from five in Kyrgyzstan (ILI), Romania (ILI and ARI) and Kazakhstan (ARI) to 15 in the Netherlands.
Table 1
Characteristics of data sets by country
Region | Seasons | Numerator | System | Nature | Denominator | Units | Average |
---|---|---|---|---|---|---|---|
Influenza‐like illness data | |||||||
![]() | 10 | ILI | Sentinel | Voluntary | Population* | ×100![]() | – |
![]() | 14 | ILI | Sentinel | Voluntary | Population | ×100![]() | 40![]() |
![]() | 6 | ILI | Sentinel | Voluntary | Consultations | ×10![]() | 6000–20![]() |
![]() | 7 | ILI | Sentinel | Compulsory | Population | ×100![]() | 2000![]() |
![]() | 10 | ILI | Sentinel | Voluntary | Population | ×100![]() | 263![]() |
![]() | 12 | ILI | Sentinel | Voluntary | Population | ×100![]() | 1![]() ![]() |
![]() | 5 | ILI | Nationwide | Compulsory | Population | ×100![]() | 5![]() ![]() |
![]() | 6 | ILI | Sentinel | Compulsory | Population | ×100![]() | 600![]() ![]() |
![]() | 9 | ILI | Sentinel | Voluntary | Population | ×100![]() | 1![]() ![]() |
![]() | 12 | ILI | Sentinel | Voluntary | Population | ×100![]() | 56![]() |
![]() | 5 | ILI | Sentinel | Compulsory | Population | ×100![]() | 600![]() |
![]() | 10 | ILI | Sentinel | Voluntary | Population | ×100![]() | 75![]() |
![]() | 14 | ILI | Sentinel | Voluntary | Population | ×100![]() | 813![]() |
![]() | 9 | ILI | Sentinel | Voluntary | Population* | ×100![]() | – |
![]() | 15 | ILI | Sentinel | Voluntary | Population | ×100![]() | 116![]() |
Acute respiratory infection data | |||||||
![]() | 7 | ARI | Nationwide | Compulsory | Population | ×100![]() | 3![]() ![]() |
![]() | 5 | ARI | Sentinel/Nationwide | Compulsory | Population | ×100![]() | 16![]() ![]() |
![]() | 5 | ARI | Sentinel | Compulsory | Population | ×100![]() | 600![]() |
![]() | 6 | ARI | Nationwide | Compulsory | Population | ×100![]() | 44![]() ![]() ![]() |
![]() ![]() | 7 | ARI | Clustering*** | Compulsory | Population | ×100![]() | 98![]() ![]() |
*Population is estimated from the number of consultations per week. GPs have not a fixed patient list.
**Average number of consultations per week.
***Compulsory system within a sample of cities of the country.
Pre‐epidemic thresholds per 100000 population ranged from 25 (Ireland and Portugal) to 168 (Romania) for ILI data and from 189 (Kazakhstan) to 762 (Romania) for ARI data, showing the differences between the systems. Post‐epidemic thresholds were slightly different to pre‐epidemics thresholds, most of the countries with a variation in a range of ±10%, with the exception of Castilla y León (Spain), Hungary and Poland in which these differences were much higher (Table 2).
Table 2
Pre‐epidemic and post‐epidemic thresholds based on historical data until 2008‐2009, and comparison with the observed 2009–2010 season
Region | Pre‐threshold (n/100 000) | Post‐threshold (n/100 000) | Length of epidemic2009–2010 (weeks) | False alerts 2009–2010 (n) | Detection lag 2009–2010 (weeks) | Timeliness2009–2010 |
---|---|---|---|---|---|---|
Influenza‐like illness data | ||||||
![]() | 143 | 145 | 10 | 0 | 0 | 0 |
![]() | 71 | 98 | 12 | 0 | 0 | 0 |
![]() | 149 | 152 | 10 | 4 | 0 | 5 |
![]() | 150 | 92 | 12 | 0 | 0 | 0 |
![]() | 25 | 23 | 12 | 8 | 0 | 8 |
![]() | 42 | 47 | 9 | 11 | 0 | 12 |
![]() | 73 | 65 | 6 | 0 | 0 | 0 |
![]() | 55 | 56 | 7 | 13 | 0 | 13 |
![]() | 113 | 69 | 8 | 0 | 0 | 0 |
![]() | 25 | 24 | 10 | 1 | 0 | 6 |
![]() | 168 | 125 | 12 | 2 | 0 | 4 |
![]() | 27 | 32 | 7 | 0 | 0 | 0 |
![]() | 79 | 72 | 10 | 0 | 0 | 0 |
![]() | 69 | 73 | 9 | 0 | 0 | 0 |
![]() | 63 | 56 | 10 | 0 | 0 | 0 |
Acute respiratory infection data | ||||||
![]() | 457 | 402 | 18 | 0 | 2 | 2 |
![]() | 189 | 168 | 9 | 0 | 0 | 0 |
![]() | 762 | 774 | 8 | 3 | 0 | 4 |
![]() | 618 | 630 | 7 | 1 | 0 | 1 |
![]() | 573 | 537 | 4 | 4 | 0 | 6 |
The modelling results for the 2009–2010 epidemic, summarised in Table 2, are also plotted by the countries. In Castilla y León (Spain), where the method was primarily developed, MEM identified an epidemic period of 12weeks and a pre‐epidemic threshold of 71 cases per 100
000 population. The alert week (the first incidence rate above the epidemic threshold) in week 38 was the first week of the epidemic period detected by MEM. There was no detection lag, and no false alerts were observed prior to that week. However, 2
weekly rates of the epidemic period are located below the post‐epidemic threshold. The intensity of the 2009–2010 epidemic was moderated, peaking very much below the 90% level of historical epidemic values (Figure 1B).
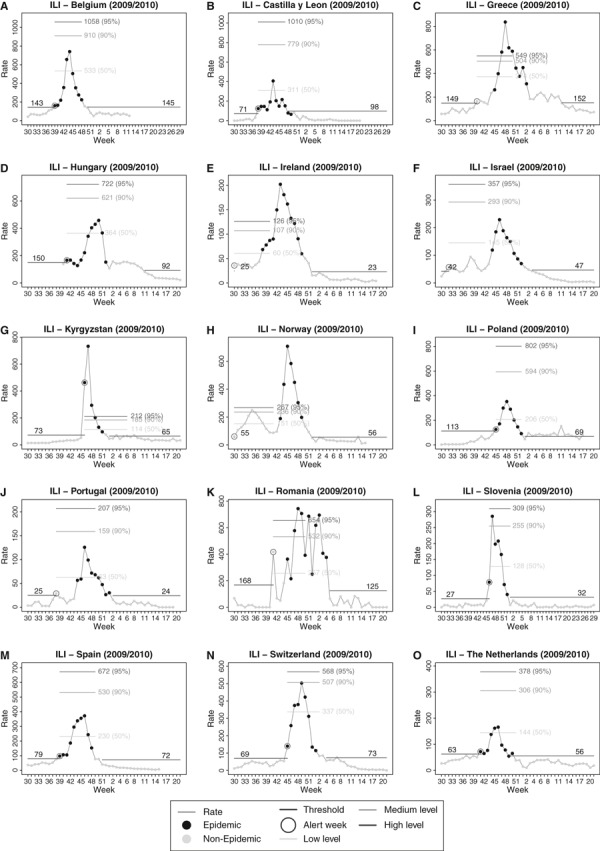
Epidemic threshold, levels of intensity and modelled influenza‐like illness season 2009–2010 by country.
In countries with similar sentinel surveillance systems, such as Belgium, Spain and Switzerland, the model also produced similar results. No false alerts or detection lags were observed, and the duration of the epidemics was 10, 10 and 9weeks, respectively. Their observed intensity remained below the 90% confidence interval. (Figure 1A,M,N). Slovenia, Poland and Kyrgyzstan showed also excellent results in terms of timeliness, although in Kyrgyzstan, rates were considerably higher in 2009–2010 than in previous historical data (Figure 1G, I, L).
High pre‐epidemic values for the pandemic season of 2009‐2010 were observed in Ireland and Romania (Figure 1E,K) and were detected by MEM as false alerts. In the cases of Israel and Norway (Figure 1F,H), this high pre‐epidemic values correspond to the first of the two waves observed in the season. In Greece and Portugal (Figure 1C,J), isolated false alerts were also detected some weeks before the epidemic period. No detection lags were observed among ILI reporting countries in the 2009–2010 season.
Among the countries providing ARI data, Albania, Kazakhstan, Romania, Russian Federation and Ukraine (Figure 2), the MEM produced high epidemic thresholds and reduced levels of intensity. The peaks of the 2009–2010 seasons exceeded the 95% confidence interval of the historical epidemic periods. The false alerts and timeliness results appeared quite similar to the ILI countries. In Albania (Figure 2A), epidemic was detected 2weeks after the start of the epidemic period, producing a detection lag according to the model.
Cross‐validation
3,3, ,44 display the historical time series of ILI and ARI used in the cross‐validation process, the epidemic periods modelled by MEM, and the alert week according to the pre‐epidemic threshold of each season. When the alert week is located beyond the first week of the epidemic period modelled by MEM, there is a delay in the detection of the seasonal epidemic. Additionally, if the alert week is inside the pre‐epidemic period modelled by MEM, there is a false alert.

Time series of influenza‐like illness by country, the epidemic periods modelled by MEM, and the alert week according with the pre‐epidemic threshold of each season.

Time series of acute respiratory infection by country and the epidemic periods modelled by MEM, and the alert week according with the pre‐epidemic threshold of each season.
The overall sensitivity of the MEM thresholds across all countries providing data was 71·8% (Table 3). The sensitivity for countries reporting ILI was slightly higher (75·7%) than for countries reporting ARI (60·1%), and this difference is borderline significant (P=
0·06). The MEM provided over 90% sensitivity in detecting the epidemic period in Belgium and Switzerland. Sensitivity was lowest in Ireland and Norway (ILI data), and in Albania, Romania and Ukraine (ARI data).
Table 3
Indicators of the model performance to detect the beginning of an epidemic period
Region | Sensitivity (%) | Specificity (%) | PPV (%)* | NPV (%)** | Median timeliness |
---|---|---|---|---|---|
All data | 71·8 | 95·5 | 91·3 | 88·6 | 1 |
Influenza‐like Illness data | 75·7 | 96·0 | 92·4 | 90·4 | 1 |
![]() | 94·6 | 98·7 | 97·3 | 97·3 | 0 |
![]() | 77·3 | 98·4 | 96·4 | 91·5 | 0 |
![]() | 85·5 | 93·6 | 90·6 | 95·2 | 0·5 |
![]() | 76·8 | 93·5 | 92·9 | 88·7 | 1 |
![]() | 55·4 | 96·1 | 93·9 | 83·8 | 2 |
![]() | 72·6 | 96·4 | 93·9 | 88·1 | 1 |
![]() | 80·0 | 92·0 | 81·5 | 88·1 | 1 |
![]() | 59·6 | 91·4 | 88·4 | 81·7 | 4·5 |
![]() | 68·4 | 94·2 | 88·9 | 90·3 | 1·5 |
![]() | 69·7 | 97·7 | 93·5 | 85·3 | 0 |
![]() | 74·1 | 95·1 | 87·5 | 91·6 | 2 |
![]() | 83·1 | 98·8 | 95·1 | 95·8 | 0·5 |
![]() | 77·5 | 98·3 | 95·9 | 90·5 | 1 |
![]() | 92·3 | 97·1 | 93·1 | 97·3 | 0 |
![]() | 68·2 | 98·6 | 96·7 | 90·8 | 2 |
Acute Respiratory Infection data | 60·1 | 94·1 | 88·2 | 83·3 | 2 |
![]() | 50·0 | 94·8 | 90·0 | 69·3 | 4 |
![]() | 72·5 | 89·6 | 83·8 | 80·2 | 1 |
![]() | 51·3 | 95·0 | 89·4 | 86·5 | 2 |
![]() | 76·6 | 95·6 | 90·2 | 95·0 | 1 |
![]() | 50·2 | 95·7 | 87·7 | 85.3 | 2 |
*Positive predictive value.
**Negative predictive value.
The specificity of the alert signal was 95·5%. The specificity was similar for both types of data (96·0% versus 94·1%), and no significant differences were found (P=
0·20). Specificity of the MEM was above 90% in all countries, with the exception of Kazakhstan (89·6%), with the highest values (over 98%) obtained for Belgium, Castilla y León, Slovenia, Spain and the Netherlands.
The positive predictive value (PPV) of observed rates above the epidemic thresholds was significantly higher (P<
0·05) for ILI data than for ARI data (92·4% versus 88·2%). No difference was observed for the negative predictive value (NPV) (90·4% versus 83·3%) (P
=
0·17). The PPV and NPV of the MEM exceeded 80% in all countries with the exception of Albania. Particularly high PPV and NPV (both above 90%) were observed in Belgium, Slovenia, Switzerland, Castilla y León, the Netherlands, Spain, Greece and Russian Federation. These were countries that also presented high sensitivity (over 75%) or specificity (over 95%) values.
The median timeliness of epidemics detection was 1week. The timeliness ranged from 0 (Belgium, Castilla y León, Portugal and Switzerland) to 4·5 (Norway), and again, the lowest values appeared in countries where other statistics of performance appeared strongest. This lag was observed to be a week longer in the countries reporting ARI than in countries reporting ILI but this difference was not statistically significant (P
=
0·13).
Discussion
The main purpose of the MEM is to calculate an epidemic threshold based on simple and reliable epidemiological data. The epidemic threshold is an ILI or ARI rate above which we define the start of the seasonal epidemic. The model was primarily developed to model influenza epidemics but, in order to ascertain its practical usefulness to calculate the threshold in ‘real world’ settings, we have applied the MEM to 20 data sets from 19 countries in the WHO European region with a diversity of surveillance systems and data types. While MEM performance varied considerably in different scenarios, the method generally produced a robust and specific signal to detect influenza epidemics and assessed the impact of seasonal respiratory infections in countries where only ARI data were available.
Unlike other methods, 16 , 20 , 24 the MEM does not include virological data. Influenza detection rates vary across the European Region depending on methods and practices in different countries (some of which have limited laboratory capacity) and may be less standardised than epidemiological measures for routine detection of the beginning of the influenza season. Influenza viruses circulate in a population at very low levels even outside of the influenza season and sporadic isolations may also have a little epidemic significance. As some countries within the European Region may not have influenza sentinel surveillance systems, viruses reported from out‐of‐season outbreaks investigations could also reduce the specificity of the method to detect the actual start of a season. Moreover, low‐intensity epidemics in rural areas with limited laboratory capacity could delay the first detection of influenza for weeks in some countries. This suggests that a model using only simple epidemiological data might be a more practical choice for a standard within a region. Nevertheless, historical ILI and ARI data should be carefully checked to eliminate the data errors or aberrant information causing peaks in the pre‐epidemic and post‐epidemic periods. Notwithstanding, if influenza weekly epidemiological data are accompanied by timely virological data, virus circulation should be taken into account to make appropriate public health decisions.
Hashimoto et al. 32 have explored the detection of epidemics in their early stage and defined periods of no activity and pre‐epidemic periods, but the threshold and limits were not modelled based on the duration and intensity of historic influenza epidemics, and the alert status varied significantly depending of the arbitrary thresholds used. 33 Cowling et al. 21 suggested that the influenza surveillance systems should incorporate a simple and reliable model with maximum sensitivity and specificity, and a minimum lag in detecting epidemics. While no system can optimise each of these attributes, influenza surveillance through well‐run sentinel networks has proven its utility and validity, and strongly enhanced the value of predictive models. The routine collection of a limited amount of high‐quality data in sentinel systems improves the sensitivity and specificity in comparison with compulsory and other non‐sample systems. 34
The MEM epidemic threshold has a sensitivity of 75·7% for ILI data and a very high specificity (overall more than 95% for ILI and ARI data). The epidemic threshold estimated by the MEM is conservative. Specificity is a more important attribute for the detection of an influenza epidemic than sensitivity, because the announcement of the start of influenza epidemic can generate media interest and triggers influenza prevention and control measures such as antiviral use, enhancements of vaccination campaigns or the implementation of non‐pharmaceutical interventions. When the epidemic alert signal is excessively specific, the detection lag increases and reduces timeliness, as observed in Albania. The NPV increases in the pre‐epidemic period, when incidence is lower. Conversely, the positive predictive value of MEM was observed to be highest during the epidemic period. The MEM seeks to achieve a balance between these values, as shown in Belgium and Slovenia (where values >95% were observed in both attributes), because it is this transition point from the pre‐epidemic to the epidemic period where values are more relevant. Timeliness is higher in ARI data than ILI data, but this difference is not statistically significant, probably due to the sample size.
Case definitions with a lower relative specificity for influenza, such as ARI, will reduce the specificity of the model to detect an influenza season. This occurs because increasing circulation of other respiratory pathogens, such as respiratory syncytial virus, is more likely to produce increasing ARI than ILI consultations. Other factors influencing outpatient consultation rates, such as public anxiety, highly sensitised clinicians 35 or the circulation of a novel influenza virus as was observed during the 2009–2010 pandemic in several European countries, 36 can also produce aberrant epidemiological data and false‐positives results. This may also results in patterns with bimodal waves as observed in some countries such as Ukraine in 2009–2010 or pre‐epidemic peaks as observed in Portugal and Greece. In these situations, complementary virological data are necessary to confirm the start of the epidemic period. The MEM model presents a limitation when there are two or more epidemics waves in the same season, as observed in the 2009–2010 pandemic season in Israel and Norway, which needs to be taken into account when evaluating the model.
The validity of the model for detecting the start of the ARI epidemics should be explored using a larger data set. A disease model deriving a baseline and threshold from pre‐epidemic disease‐free periods seems less appropriate for ARI data, where the case definition covers a greater number of pathogens, some of which may circulate widely prior to the epidemic period. 37 , 38 As the baselines are intended to register ‘background noise’ when there is no epidemic at all, there may be some problems with ARI, as this background noise caused by summer‐time respiratory viruses including parainfluenza, rhinovirus, coronavirus and adenovirus may sometimes exceed the epidemic signal. ARI data from Albania and Romania did not present a typical epidemic wave but an increase in consultation rates with several peaks, while the Russian Federation showed high post‐epidemic rates. Despite the differences between ILI and ARI, the only statistic that we observed to be significantly different between ILI and ARI systems is positive predictive value. ARI thresholds calculated by MEM could also be useful to detect an excess of disease. This heterogeneity of European data provides a basis for discussion about the appropriateness of the MEM for routine use in sentinel respiratory disease surveillance.
The mathematical approach underlying MEM also invites discussion of the duration of the epidemic period and its relationship with the calculated threshold. The shorter the duration, the higher is the epidemic threshold. In this work, the duration is determined by the slope change following the first step of the MEM procedure. The δ parameter depends on the shape and intensity of the historical influenza seasons, and it is recommended to lead particularised country analysis to find the δ value which maximises the sensitivity and specificity.
The significance of the post‐epidemic thresholds is still a point for discussion. ARI data show more differences between pre‐ and post‐epidemic baselines than ILI data, probably because we are looking at different diseases, while in influenza, true variations could occur. The large variation by country does suggest, however, methodological reasons, which will require further analysis.
Although a common approach is necessary for standard comparisons of the timing and intensity of influenza seasons across countries, the epidemic threshold and intensity levels may be modified depending on national objectives. For instance, the 50%, 90% and 95% CIs used to calculate levels of intensity and to compare the epidemics through different countries are discretional and could be changed.
In this work, we chose the parameters which, based on the authors experience, would give the most reliable results. However, these parameters may be adjusted by countries: more/less pre‐epidemic points, median and bootstrap confidence intervals, arithmetic or geometric means, etc. The variations in these parameters do not question the underlying structure of the model and allow the method to be adapted by different surveillance systems.
We selected countries with five or more years of historical data to be included in this study, but it is still open how many historical seasons should be included in building the model, even when long time series are available. Changes in case reporting, demographics, case definitions and secular trends (which are declining in several countries) 39 could affect how well the model fits the tested data. Typically, influenza mortality models make use of at least the five preceding seasons. 13 , 40 , 41 More than ten seasons may further increase accuracy but make a model susceptible to biases from secular trends.
The MEM is an open method and offers a flexible procedure for calculating a threshold for outpatient consultations. The main achievement of MEM is an algorithm splitting one wave season in three periods using pre‐epidemic information to calculate a threshold. The high sensitivity and specificity of the threshold in detecting the beginning of the epidemics in most countries, despite their diverse surveillance system and data quality, support its use for public health purposes such as early warning, health services policy or mass media and public information. MEM can be applied to historical ARI data, but the validity indicators show a somewhat poorer performance than for its application to ILI data.
One common method of analysis and interpretation of ILI data should be established in Europe. MEM could be a good option because of its intuitive concept, simple data requirements and flexibility in comparison with other sophisticated mathematical models. But other steps towards harmonisation should also be taken. These analyses suggest an added benefit from the establishment of an ILI definition with highly sensitive inclusion criteria. 42 Combined with highly specific threshold derived indicators, Europe would obtain an even more reliable and comprehensive perspective on influenza surveillance. Moreover, comparing ILI weekly data with levels of intensity calculated by MEM with a standard algorithm would allow international assessment of clinical influenza activity among European countries.
Acknowledgements
The authors would like to thank allinvestigators, the secretaries and the staff from the WHO Regional Office for Europe, the European Centre for Diseases Prevention and Control and country representatives who coordinated the participation, contributed in providing and checking country and network data, as well as reviewing and commenting local results:
WHO Regional Office for Europe: Caroline S. Brown
European Centre for Diseases Prevention and Control: Daniel Faensen, Phillip Zucs, Silvia Sarbu, Flaviu Plata, Tommi Asikainen and Johan Giesecke.
Country representatives by alphabetical country name: Albania. Institute of Public Health (Tirana): As. Prof. Silva Bino, Dr.Dritan Ulqinaku, Dr.Artan Simaku, Dr. Iris Hatibi and Dr.Alma Robo. Belgium. Scientific Institute of Public Health (Brussels): Dr. Francoise Wuillaume and Dr. Viviane Van Casteren. Castilla y León. Consejería de Sanidad. (Valladolid, Spain): Dr. Carolina Rodriguez Gay and Milagros Gil Costa. Greece. Hellenic Centre for Disease Control and Prevention (Athens): Theodore Lytras. Hungary. National Center for Epidemiology, Department of Communicable Diseases Epidemiology (Budapest): Dr. Csohán Ágnes, Dr. Molnár Zsuzsanna and Kaszás Katalin. Ireland. Health Protection Surveillance Centre, (Dublin): Dr. Lisa Domegan, Dr. Joan O Donnell and Dr. Darina O Flanagan. Israel. The Israel Center for Disease Control (Tel Hashomer): Zalman Kaufman, Dr. Michal Bromberg and the Maccabi Health Services, Israel. Kazakhstan. The State Sanitary Epidemiological surveillance committee (Bishkek): Dr. Temirgalieva Aigul; and the Department of Epidemiological SurveillanceRepublic SES of Kazahstan: Dr. Ainagul Kuatbaeva. Kyrgyzstan. Department of State Sanitary Epidemiological Surveillance Ministry of Health of the Kyrgyz Republic (Bishkek): Dr. Kaliya Kasymbekova, and Dr. Nurmatov Zuridin Norway. Norwegian Institute of Public Health (Oslo): Dr. Siri H. Hauge and Dr. Bjørn G. Iversen. Poland. Department of Influenza Research. National Influenza Center, National Institute of Public Health‐National Institute of Hygiene (Warsaw): Dr. Romanowska Magdalena and Dr. Brydak Lidia B. Portugal. Departamento de Epidemiologia, Instituto Nacional de Saúde Dr. Ricardo Jorge (Lisboa): Carlos Matias Dias, Inês Batista and the Gp’s from Rede Médicos Sentinela. Romania. National Center for Surveillance and Control of Communicable Diseases, National Institute for Public Health (Bucharest): Dr. Rodica Popescu and Dr.Odette Nicolae. Russian Federation. Research Institute of Influenza Ministry of Health and Social Development of Russian Federation (St. Petersburg): Dr. Karpova Ludmila Serafimovna and Dr. Stolarov Kiril Alexandrovich. Slovenia. National Institute of Public Health (Ljubljana): Dr. Maja Socan. Spain. National Center of Epidemiology. Carlos III Health Institute (Madrid): Dr. Amparo Larrauri Camara and Dr. Silvia Jiménez‐Jorge, on behalf of the Spanish Influenza Surveillance System (SISS). Switzerland. Federal Office of Public Health. Directorates of Public Health. Division of Communicable Diseases (Bern): Dr. Rita Born. The Netherlands. Dutch Sentinel General Practice Network, Netherlands Institute of Health Services Research (Utrecht): Dr. Gé A. Donker. Ukraine. L.V.Gromashevsky Institute of Epidemiology & Infectious Diseases Academy of Medical Science of Ukraine (Kiev): Dr. Alla Mironenko, Dr. Olga Onischenko and Dr. Olga Golubka.
We also would like to thank all researchers who contributed as scientific advisors to the MEM developmentin the past: John Paget from the Netherlands Institute of Health Services Research (Utrecht), Marisol Gutierrez from the Consejería de Sanidad (Valladolid, Spain) and Mark Witschi from the WHO Regional Office for Europe.
Finally, we would particularly like to thank the primary healthcare professionals for their dedication to global influenza surveillance.
References

Articles from Influenza and Other Respiratory Viruses are provided here courtesy of Wiley
Full text links
Read article at publisher's site: https://doi.org/10.1111/j.1750-2659.2012.00422.x
Read article for free, from open access legal sources, via Unpaywall:
https://europepmc.org/articles/pmc5855152?pdf=render
Citations & impact
Impact metrics
Article citations
Setting thresholds to determine COVID-19 activity levels using the mean standard deviation (MSD) method, England, 2022-2024.
Euro Surveill, 29(45), 01 Nov 2024
Cited by: 0 articles | PMID: 39512168 | PMCID: PMC11544723
Combined short-term exposure to meteorological, pollution factors and pertussis in different groups from Jining, China.
J Glob Health, 14:04234, 25 Oct 2024
Cited by: 0 articles | PMID: 39450617 | PMCID: PMC11503506
Immunity Debt Regarding the Aspect of Influenza in the Post-COVID-19 Era in Taiwan.
Viruses, 16(9):1468, 15 Sep 2024
Cited by: 0 articles | PMID: 39339944 | PMCID: PMC11437480
Evaluation of the design of the influenza-like illness sentinel surveillance system in Brazil.
Cad Saude Publica, 40(6):e00028823, 29 Jul 2024
Cited by: 0 articles | PMID: 39082558 | PMCID: PMC11321611
A Predictive Model of the Start of Annual Influenza Epidemics.
Microorganisms, 12(7):1257, 21 Jun 2024
Cited by: 0 articles | PMID: 39065025 | PMCID: PMC11278734
Go to all (107) article citations
Similar Articles
To arrive at the top five similar articles we use a word-weighted algorithm to compare words from the Title and Abstract of each citation.
Influenza surveillance: determining the epidemic threshold for influenza by using the Moving Epidemic Method (MEM), Montenegro, 2010/11 to 2017/18 influenza seasons.
Euro Surveill, 24(12), 01 Mar 2019
Cited by: 14 articles | PMID: 30914080 | PMCID: PMC6440585
Influenza surveillance in Europe: comparing intensity levels calculated using the moving epidemic method.
Influenza Other Respir Viruses, 9(5):234-246, 01 Sep 2015
Cited by: 70 articles | PMID: 26031655 | PMCID: PMC4548993
[Application of the moving epidemic method in the development of epidemic thresholds and tiered warning alert approachs for influenza prevention in Beijing].
Zhonghua Liu Xing Bing Xue Za Zhi, 41(2):201-206, 01 Feb 2020
Cited by: 1 article | PMID: 32164130
Harmonizing influenza primary-care surveillance in the United Kingdom: piloting two methods to assess the timing and intensity of the seasonal epidemic across several general practice-based surveillance schemes.
Epidemiol Infect, 143(1):1-12, 15 Jul 2014
Cited by: 29 articles | PMID: 25023603 | PMCID: PMC9206800
Review Free full text in Europe PMC