Abstract
Free full text

Heritability enrichment of specifically expressed genes identifies disease-relevant tissues and cell types
Abstract
We introduce an approach for identifying disease-relevant tissues and cell types by analyzing gene expression data together with genome-wide association study (GWAS) summary statistics. Our approach uses stratified LD score regression to test whether disease heritability is enriched in regions surrounding genes with the highest specific expression in a given tissue. We apply our approach to gene expression data from several sources together with GWAS summary statistics for 48 diseases and traits (average N=169K), detecting significant tissue-specific enrichments (FDR<5%) for 34 traits. In our analysis of multiple tissues, we detect a broad range of enrichments that recapitulate known biology. In our brain-specific and immune-specific analyses, significant enrichments include an enrichment of inhibitory over excitatory neurons for bipolar disorder but excitatory over inhibitory neurons for schizophrenia and body mass index. Our results demonstrate that our polygenic approach is a powerful way to leverage gene expression data for interpreting GWAS signal.
INTRODUCTION
There are many diseases whose causal tissues or cell types are uncertain or unknown. Identifying these tissues and cell types is critical for developing systems to explore gene regulatory mechanisms that contribute to disease. In recent years, researchers have been gaining an increasingly clear picture of which parts of the genome are active in a range of tissues and cell types: for example, which parts of the genome are accessible, which enhancers are active, and which genes are expressed1–3. Combining this type of information with GWAS data offers the potential to identify causal tissues and cell types for disease.
Many different types of data characterizing tissue- and cell-type-specific activity have been analyzed together with GWAS data to identify disease-relevant tissues and cell types, including histone marks4–8, DNase I hypersensitivity (DHS)9–12, eQTLs3,13, and gene expression data14–17. Of these data types, gene expression data (without genotypes or eQTLs) has the advantage of being available in the widest range of tissues and cell types. Previous studies have shown that gene expression data are informative for disease-relevant tissues and cell types, and have led to biological insights about the diseases and traits studied14–17. However, the methods applied in these studies restrict their analyses to subsets of SNPs that pass a significance threshold. To our knowledge, no previous study has modeled genome-wide polygenic signals to identify disease-relevant tissues and cell types systematically from GWAS and gene expression data.
Here, we apply stratified LD score regression7, a method for partitioning heritability from GWAS summary statistics, to sets of specifically expressed genes to identify disease-relevant tissues and cell types across 48 diseases and traits with an average GWAS sample size of 169,331. We first analyze two gene expression data sets3,17,18 containing a wide range of tissues to infer system-level enrichments. We then analyze chromatin data from the Roadmap Epigenomics and ENCODE projects1,2 across the same set of diseases and traits to validate these results. Finally, we analyze gene expression data sets that allow us to achieve higher resolution within a system3,19–21, identifying enriched brain regions, brain cell types, and immune cell types for several brain- and immune-related diseases and traits; we validate several of our immune enrichments using independent chromatin data. Our results underscore that a heritability-based framework applied to gene expression data allows us to achieve high-resolution enrichments, even for very polygenic traits.
RESULTS
Overview of methods
We analyzed the five gene expression data sets listed in Table 1, mapping mouse genes to orthologous human genes when necessary. To assess the enrichment of a focal tissue for a given trait, we follow the procedure described in Figure 1. We begin with a matrix of normalized gene expression values across genes, with samples from multiple tissues including the focal tissue. For each gene, we compute a t-statistic for specific expression in the focal tissue (Online Methods). We rank all genes by their t-statistic, and define the 10% of genes with the highest t-statistic to be the gene set corresponding to the focal tissue; we call this the set of specifically expressed genes, but we note that this includes not only genes that are strictly specifically expressed (i.e. only expressed in the focal tissue), but also genes that are weakly specifically expressed (i.e. higher average expression in the focal tissue). For a few of the data sets analyzed, we modified our approach to constructing the set of specifically expressed genes to better take advantage of the data available (Online Methods). We add 100kb windows on either side of the transcribed region of each gene in the set of specifically expressed genes to construct a genome annotation corresponding to the focal tissue. (The choice of the parameters 10% and 100kb is discussed in the Supplementary Note; our results are robust to these choices (see below).) Finally, we apply stratified LD score regression7 to GWAS summary statistics to evaluate the contribution of the focal genome annotation to trait heritability (Online Methods). We jointly model the annotation corresponding to the focal tissue, a genome annotation corresponding to all genes, and the 52 annotations in the “baseline model”7 (including genic regions, enhancer regions, and conserved regions; see Table S1). A positive regression coefficient for the focal annotation in this regression represents a positive contribution of this annotation to trait heritability, conditional on the other annotations. We report regression coefficients, normalized by mean per-SNP heritability, together with a P-value to test whether the regression coefficient is significantly positive. Stratified LD score regression requires GWAS summary statistics for the trait of interest, together with an LD reference panel (e.g. 1000 Genomes22), and has been shown to produce robust results with properly controlled type I error7. We have released open source software implementing our approach, and have also released all genome annotations derived from the publicly available gene expression data that we analyzed (see URLs). We call our approach LD score regression applied to specifically expressed genes (LDSC-SEG).
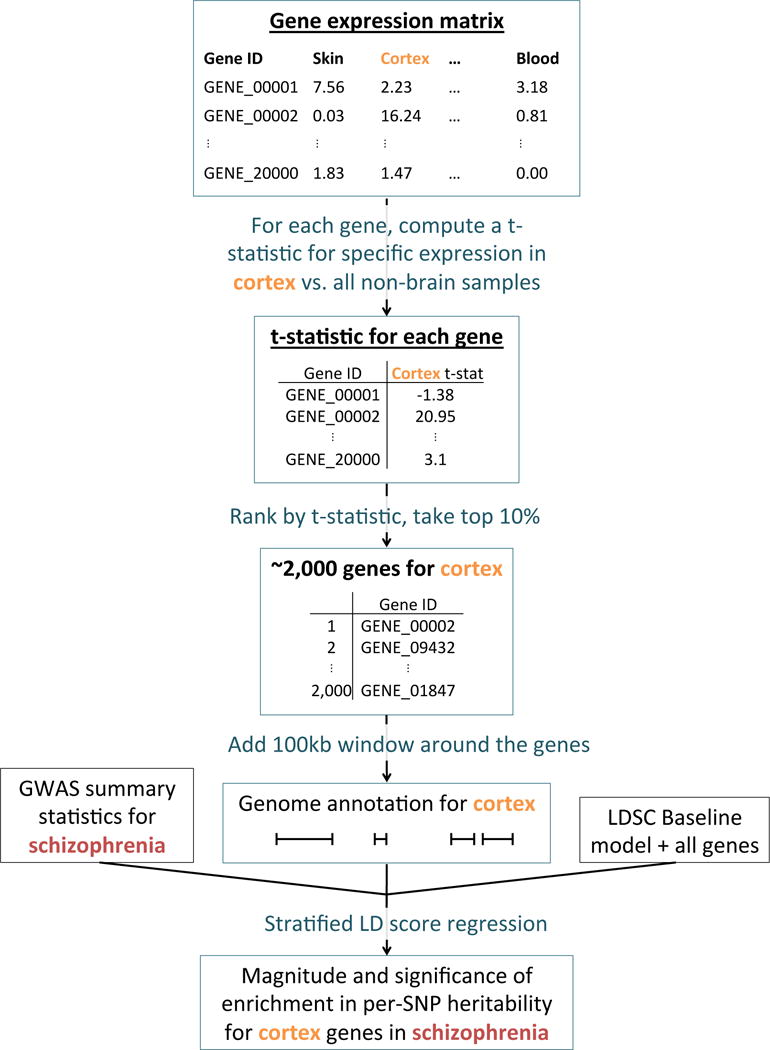
Overview of the approach. For each tissue in our gene expression data set, we compute t-statistics for differential expression for each gene. We then rank genes by t-statistic, take the top 10% of genes, and add a 100kb window to get a genome annotation. We use stratified LD score regression7 to test whether this annotation is significantly enriched for per-SNP heritability, conditional on the baseline model7 and the set of all genes.
Table 1
List of gene expression data sets used in this study. We analyzed five gene expression data sets: two (GTEx and Franke lab) containing a wide range of tissues and three (Cahoy, PsychENCODE, ImmGen) with more detailed information about a particular tissue.
Analysis of 48 complex traits across multiple tissues
We first analyzed two gene expression data sets — GTEx and a dataset which we call the Franke lab data set — and we classified the 205 tissues and cell types in these data sets into nine categories for visualization (Tables S2 and S3, Online Methods). We analyzed GWAS summary statistics for 48 diseases and traits from the UK Biobank23 (Online Methods), the Brainstorm Consortium16,24–32, and publicly available sources33–43, with an average sample size of 169,331 (Table S4), applying LDSC-SEG for each of the 205 specifically expressed gene annotations in turn. We excluded the HLA region from all analyses, due to its unusual genetic architecture and pattern of LD.
For 34 of the 48 traits, at least one tissue was significant at FDR<5% (Figure 2, Figure S1 and Tables S5 and S6). Several of our results recapitulate known biology: immunological traits exhibit immune cell-type enrichments, psychiatric traits exhibit strong brain enrichment, LDL and triglycerides exhibit liver-specific enrichments, BMI-adjusted waist-hip ratio exhibits adipose enrichment, type 2 diabetes exhibits enrichment in the pancreas, and height exhibits enrichments in a variety of tissues in a pattern similar to previous analyses of this trait44. In addition, several of our results validate very recent findings from other genetic analyses: in particular, smoking status, years of education, BMI, and age at menarche show robust brain enrichments that recapitulate results from our previous analysis of genetic data together with chromatin data7. Our results were robust to the choice of percent of genes used (10%) and to the size of the window used (100kb) (Figure S2). We assessed correlations in enrichment patterns for pairs of traits (Online Methods), and found large and significant correlations among many brain-related phenotypes, among many immune-related phenotypes, and among a third set of phenotypes including height and blood pressure that tended to have enrichments in the musculosketal/connective, cardiovascular, and other categories (Figure S3). The most significant annotation for each of these 34 traits spanned 11%-23% (mean 16%) of the genome and explained 21%-62% (mean 36%) of SNP-heritability, with enrichments varying from 1.4× to 4.7× (mean 2.3×) (Table S5).
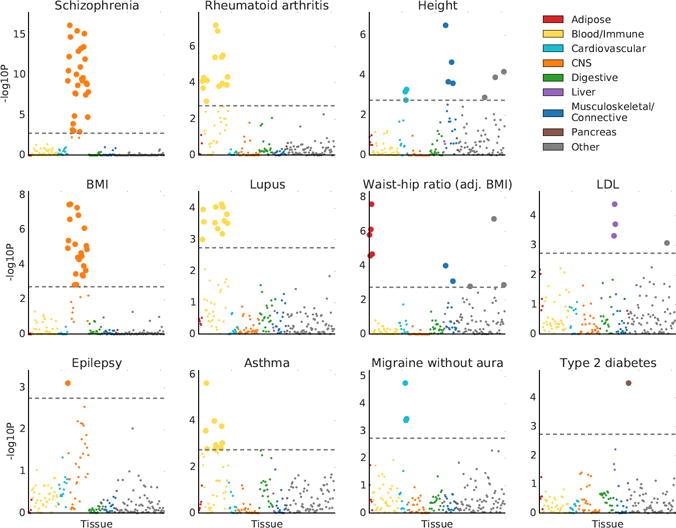
Results of the multiple-tissue analysis for selected traits. Results for the remaining traits are displayed in Figure S1. Each point represents a tissue/cell type from either the GTEx data set or the Franke lab data set. Large points pass the FDR<5% cutoff, –log10(P)=2.75. GWAS data is described in Table S4, gene expression data is described in the Online Methods and Tables S2-3, and the statistical method is described in the Overview of Methods and the Online Methods. Numerical results are reported in Table S6.
Because related tissues have highly overlapping gene sets and we fit each tissue without adjusting for the other tissues, related tissues often appear enriched as a group. In this analysis and the analysis in the next section, both focused on identifying system-level enrichments, these correlated results do not limit interpretability. In later sections, we focus on differentiating among related tissues/cell types within a system. We note also that the correlation structure among annotations can lead to a distribution of P values that is highly non-uniform (Online Methods).
Validation using independent chromatin data
We analyzed the same 48 diseases and traits using stratified LD score regression7 in conjunction with chromatin data from the Roadmap Epigenomics and ENCODE projects1,2 (see URLs) instead of gene expression data, with three goals: (1) to validate the results from our analysis of gene expression data using a different type of data from an independent source, (2) to identify new enrichments using chromatin data that we did not observe using gene expression data, and (3) to compare enrichments from the two types of data. The ENCODE data we used was from a subproject called EN-TEx, which includes epigenetic data on a set of tissues that match a subset of the tissues from the GTEx project but are from different donors. In total, we analyzed 489 tissue-specific chromatin-based annotations from peaks for six epigenetic marks (Online Methods).
We considered two types of validation for the results of the multiple-tissue analysis of gene expression described above: validation at the system level and validation at the tissue/cell-type level. For validation at the system level, we classified the top tissue or cell type for each trait with a significant enrichment into one of nine systems (Online Methods), and we considered an enrichment to be validated if a tissue or cell type from the same system passed FDR < 5% for the same phenotype in the chromatin analysis. For validation at the tissue/cell-type level, we only analyzed the 27 tissues present in both GTEx and EN-TEx, and we considered an enrichment of a tissue in GTEx to be validated if any mark in the same tissue in EN-TEx passed FDR < 5% for the same phenotype. The top enrichment from our multi-tissue analysis of gene expression was validated at the system level for 33 out of 34 phenotypes (Figure 3a, Table S5), and the top enrichment of a tissue or cell type shared between GTEx and EN-TEx was validated at the tissue/cell-type level for 13 out of 20 phenotypes, rising to 16 with a more lenient definition (Table S5, Online Methods). In many instances, the analysis of chromatin data detected more enrichments, larger enrichments, and/or enrichments at higher significance levels than the analysis of gene expression data, though this was not always the case (Figures S4-S5, Table S7, Online Methods). The enrichment correlations in this analysis showed a similar pattern to the gene expression analysis above (Figure S6).
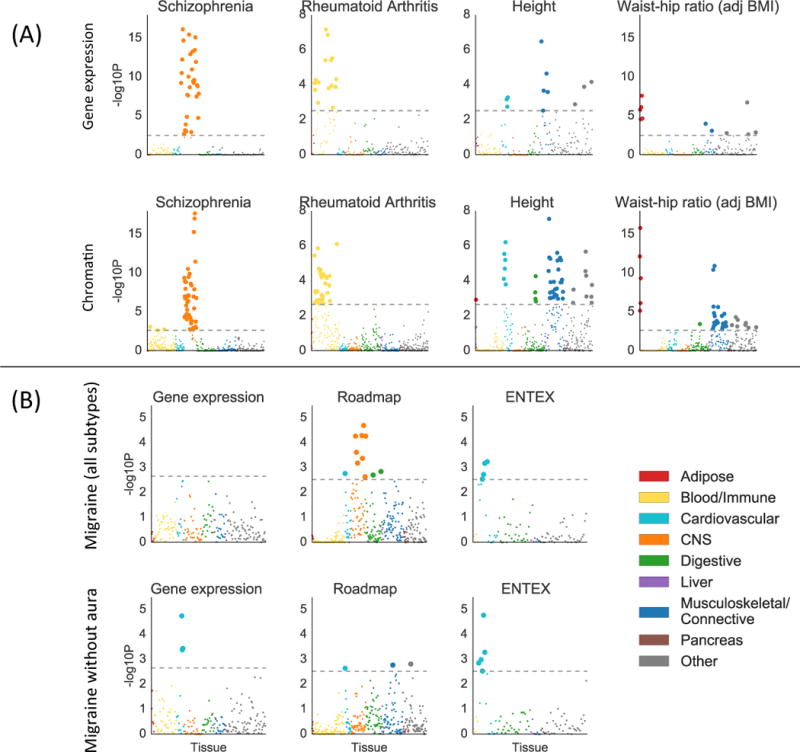
Validation of gene expression results with chromatin data. (A) Examples of validation using chromatin data (bottom) of results from gene expression data (top), for selected traits. Results using chromatin data for all traits are displayed in Figure S5, with numerical results in Table S7. For the chromatin results, each point represents a track of peaks for H3K4me3, H3K4me1, H3K9ac, H3K27ac, H3K36me3, or DHS in a single tissue/cell type. (B) Results using gene expression data (including GTEx), Roadmap, and EN-TEx, for migraine (all subtypes) and migraine without aura. For both subfigures, large points pass the FDR<5% cutoff, –log10(P)=2.85 (chromatin) or –log10(P)=2.75 (gene expression). GWAS data is described in Table S4; gene expression data and chromatin data are described in the Online Methods, Tables S2-3, and Table S7; and the statistical method is described in the Overview of Methods and the Online Methods.
There is a long-standing scientific debate as to whether migraine has a primarily neurological or vascular basis45. We analyzed GWAS summary statistics for migraine with aura, migraine without aura, and migraine (all subtypes)16. The migraine (all subtypes) data set contained the data sets for migraine with aura and for migraine without aura, as well as a large number of additional subjects whose subtype was unknown. We found cardiovascular enrichments for migraine without aura with gene expression data, and for migrane without aura and migraine (all subtypes) with EN-TEx data, consistent with previous work16 (Figure 3b). Our analysis of Roadmap data, however, yielded qualitatively different results: the strongest enrichment for migraine (all subtypes) was a neurological enrichment. The top two annotations were neurospheres and fetal brain, neither of which was present in the gene expression data we analyzed nor in EN-TEx. The correlation in enrichments between migraine (all subtypes) and migraine without aura in the gene expression analysis was estimated to be 0.48 (s.e. 0.15), and in the chromatin data was estimated to be 0.60 (s.e. 0.13). Our results are consistent with the hypothesis that migraine without aura does indeed have a vascular component, and that another subtype of migraine may have a neurological basis which is sufficiently cell-type specific that the relevant cell types are not represented in either the GTEx or Franke lab data sets. These results highlight the importance of having as many tissues and cell types as possible represented in a multiple-tissue analysis.
A major advantage of gene expression data is that it is available at finer tissue/cell-type resolution within several systems. In the within-system analyses that follow, we investigate these finer patterns of tissue/cell-type specificity.
Analysis of 12 brain-related traits using fine-scale brain expression data
We identified 12 traits with CNS enrichment at FDR<5% in our gene expression and/or chromatin analyses (Online Methods). We first investigated whether some brain regions are enriched over other brain regions for these traits using gene expression data from GTEx (Figure S7, Online Methods). The results are displayed in Figure 4a and Table S8a. We identified significant enrichments in the cortex relative to other brain regions at FDR<5% for bipolar disorder, schizophrenia, depressive symptoms, and BMI, and in the striatum for migraine. These enrichments are consistent with our understanding of the biology of these traits46–49, but to our knowledge have not previously been reported in any integrative analysis using genetic data. We also identified enrichments in cerebellum for bipolar disorder, years of education, and BMI. However, we caution that differential gene expression in samples from different brain regions can reflect the cell type composition of these brain regions as well as their function. In particular, the cerebellum is known to have a very high concentration of neurons50, and thus cerebellar enrichments could indicate either that the cerebellum is a region that is important in disease etiology, or that neurons are an important cell type. While many pairs of phenotypes had high estimated enrichment correlations in this analysis, migraine tended to have low enrichment correlations with other phenotypes (Figure S8); for example, the estimated enrichment correlation between migraine and schizophrenia was 0.06 (s.e.=0.30) while the estimated enrichment correlation between bipolar disorder and schizophrenia was 0.96 (s.e.=0.05).
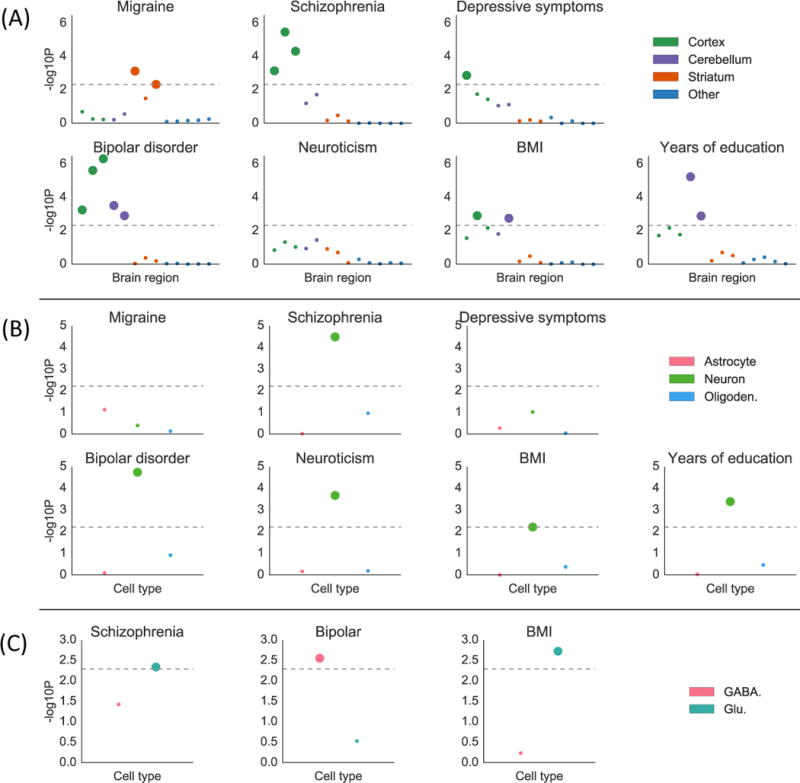
Results of the brain analysis for selected traits. Numerical results for all traits are reported in Table S8. (A) Results from within-brain analysis of 13 brain regions in GTEx, classified into four groups, for seven of 12 brain-related traits. Large points passed the FDR<5% cutoff, –log10(P)=2.34. (B) Results from the data of Cahoy et al. on three brain cell types for seven of 12 brain-related traits. Large points passed the FDR<5% cutoff, –log10(P)=2.22. (C) Results from PyschENCODE data on two neuronal subtypes for three of five neuron-related traits. Large points passed the Bonferroni significance threshold in this analysis, –log10(P)=2.06. GWAS data is described in Table S4, gene expression data is described in the Online Methods and Table S8, and the statistical method is described in the Overview of Methods and the Online Methods.
To address the question of the relative importance of brain cell types, as opposed to brain regions, we analyzed the same set of traits using a publicly available data set of specifically expressed genes identified from different brain cell types purified from mouse forebrain19 (Online Methods). The results of this analysis are displayed in Figure 4b and Table S8b. We identified neuronal enrichments at FDR<5% for five traits: bipolar disorder, schizophrenia, years of education, BMI, and neuroticism. The other cell types did not exhibit significant enrichment for any of the 12 brain-related traits. The enrichment of neurons for all three of the traits with enrichment in cerebellum in the brain-region analysis supports the hypothesis that analyses of brain regions may be confounded by cell-type composition.
To more precisely characterize the neuronal enrichments, we analyzed the five traits with neuronal enrichment at FDR<5% using t-statistics computed by the PsychENCODE consortium20 on differential expression in glutamatergic (excitatory) vs. GABAergic (inhibitory) neurons (Online Methods). The results are displayed in Figure 4c and Table S8c; we used Bonferroni correction in this analysis, as we were testing only 5×2=10 hypotheses. For bipolar disorder, genes that are specifically expressed in GABAergic neurons exhibited heritability enrichment, while genes specific to glutamatergic neurons did not. This result supports the theory that pathology in GABAergic neurons can contribute causally to risk for bipolar disorder51,52. For BMI and schizophrenia, on the other hand, we found significant enrichment in glutamatergic neurons but not in GABAergic neurons.
We were unable to validate the results of these analyses using independent chromatin data. For the two analyses of brain cell types, this was because we were not aware of any available data sets with analogous chromatin data. For the analysis of brain regions, this was because the chromatin annotations that we analyzed were highly correlated across different brain regions and thus some phenotypes showed enrichment in nearly every brain region; we did not consider these non-specific enrichments to be a meaningful validation of our region-specific results using gene expression data.
Analysis of 25 immune-related traits using immune cell expression data
We identified 25 traits with immune enrichment at FDR<5% in our gene expression and/or chromatin analyses (Online Methods). We investigated cell-type-specific enrichments for these traits using gene expression data from the ImmGen project21, which contains microarray data on in 292 immune cell types from mice (Online Methods). This data set contains data for many immune cell types that are not available in the multiple-tissue analysis, and because we compute t-statistics within the data set—i.e., each immune cell vs. other immune cells—the gene sets are less overlapping than those of immune cell types in the multiple-tissue analysis.
We identified enrichments at FDR<5% for 16 traits. Results are displayed in Figure 5, Figure S9 and Tables S9 and S10, and reveal highly trait-specific patterns of enrichment. For primary biliary cirrhosis, the largest and most significant enrichment was in B cells, consistent with literature on the importance of B cells for this trait54. Alzheimer’s disease exhibits enrichment in myeloid cells, as seen previously from genetics55. Asthma and eczema both exhibited enrichment in T and NKT cells; several subclasses of T cells have been shown to be important in asthma,57 and a previous study using chromatin data found an enrichment in T cells for asthma but not in other immune cell types6. Rheumatoid arthritis, Crohn’s disease, inflammatory bowel disease, and multiple sclerosis all exhibited enrichments in a variety of cell types, consistent with complex etiologies for these diseases that involve many different immune cell types58–60. Schizophrenia and bipolar disorder both exhibited an enrichment in T cells. Patients with bipolar disorder have been shown to have a reduction in certain types of T cells, but have equal levels of B cells, NK cells, and monocytes compared to controls61. T cell levels have been shown to vary between schizophrenia cases and controls, but existing literature is not consistent in its description of the direction of effect62. Note that our analysis excludes the HLA region; a previous analysis of the HLA region for schizophrenia implicated the complement system through its role in synaptic pruning, a signal that is distinct from the signal we observe here63. Finally, we identified an enrichment in stromal cells for both diastolic and systolic blood pressure. For each of these two traits, we identified enrichments in the musculoskeletal/connective category in the multiple-tissue analysis that were stronger than the immune enrichments in that analysis, and thus we hypothesize that the enrichment in stromal cells is not providing better resolution on the immune enrichment but instead reflects the more general importance of connective tissue. In enrichment correlation analyses, schizophrenia and bipolar disorder clustered with immunological diseases, while metabolic traits, neurological diseases, and other psychiatric diseases did not (Figure S10).
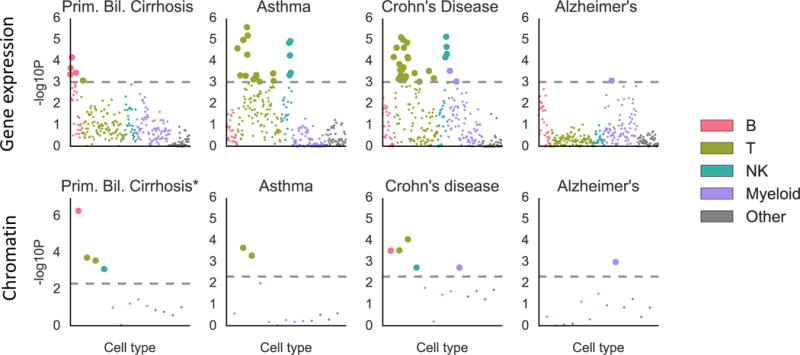
Results of the analysis of ImmGen gene expression data (top) and hematopoiesis ATAC-seq data (bottom) for selected traits. Results for the remaining traits are displayed in Figure S9. Large points passed the FDR<5% cutoff, –log10(P)=3.03 (Gene expression) or –log10(P)=2.32 (Chromatin). Numerical results are reported in Table S10. GWAS data is described in Table S4, gene expression and chromatin data is described in the Online Methods and Table S10, and the statistical method is described in the Overview of Methods and the Online Methods.
To validate these results, we analyzed ATAC-seq (chromatin) data from 13 cell types spanning the hematopoietic hierarchy in humans64. We validated 10 out of 14 top results (Table S9, Online Methods). The only immunological disease whose result was not validated was lupus; the top result for lupus in the ImmGen analysis was a myeloid cell type, while the largest and most significant enrichment in the hematopoiesis data set was a B cell enrichment, consistent with other genetic studies of this trait14.
DISCUSSION
We have shown that applying stratified LD score regression to sets of specifically expressed genes identifies disease-relevant tissues and cell types. Our approach, LDSC-SEG, allows us to take advantage of the large amount of gene expression data available—including fine-grained data for which we do not currently have a comparable chromatin counterpart—to ask questions ranging in resolution from whether a trait is brain-related to whether excitatory or inhibitory neurons are more important for disease etiology. Our results improve our understanding of the phenotypes studied here, highlight the power of GWAS as a source of biological insight, and may also be useful for choosing the relevant tissue or cell type for in-vitro experiments to further elucidate molecular mechanisms underlying genome-wide significant loci identified in genome-wide association studies.
There are several key differences between LDSC-SEG, which relies on gene expression data without genotypes or eQTLs, and approaches that require eQTL data3,13 (Online Methods, Figure S11, Supplementary Note). Our polygenic approach also differs from other gene expression-based approaches such as SNPsea14,15 and DEPICT17, which restrict their analyses to subsets of SNPs that pass a significance threshold (Supplementary Note, Figures S12-S16, Tables S11-S15).
We cannot conclusively say whether gene expression or chromatin data is preferable when both types of data are available in the same tissues and cell types (Online Methods, Figure S4, Figure S17, Table S10, Table S16). Instead, we conclude that the question of which type of data is preferable may depend on complex factors such as which chromatin marks were analyzed, the sample size with which the specifically expressed genes are called, and the overall quality of the data set. When gene expression and chromatin data are available on the same set of tissues or cell types, it may be possible to combine these types of data to improve power, for example by restricting an annotation to tissue-specific chromatin marks near specifically expressed genes, or by combining the P-values from separate analyses of the two types of data. We defer a thorough exploration of this set of possibilities to future work.
Our work is based on the assumption that a tissue or cell type is important for a particular disease if and only if SNPs near genes with high specific expression in that tissue/cell type are enriched for heritability. This assumption leads to several limitations of our approach. First, when analyzing gene expression data from different tissues, cell type composition can confound the analysis, as we demonstrated in our comparison of brain regions; this makes enrichments of organs such as the esophagus or uterus hard to interpret. Second, tissues/cell types with similar gene expression profiles to a causal tissue/cell type will be identified as relevant to disease, just as SNPs in LD with a causal SNP will be identified as associated to disease in a GWAS; thus, significant tissues/cell types should be cautiously interpreted as the “best proxy” for the truly causal tissue/cell type, which may be unobserved. Third, our focus on nearby SNPs prevents us from leveraging signal from regulatory SNPs that act at longer distances. Our approach is also fundamentally limited by the availability of gene expression data and cannot rule out the importance of a given cell type; for example, if the tissue/cell type that is most relevant for a disease occurs in a stage of development or under a stimulus that has not been assayed, then we may not identify enrichments in that tissue/cell type. We would also like to highlight that for most of these phenotypes there is likely not just one causal tissue/cell type, but many.
Our use of a heritability-based approach has advantages but also leads to some limitations. First, our approach will not detect strong but highly localized signals. Second, power increases only modestly with sample size at very large sample sizes (Supplementary Note). Also, because our approach uses stratified LD score regression, it cannot be applied to custom array data, it requires a sequenced reference panel that matches the population studied in the GWAS, and can be affected by model misspecification7. Recent augmentations to the baseline model65 have been shown to help ameliorate model misspecification, but we leave further investigation of this in the context of cell-type-specific analyses to future work.
Another limitation of our method is that its results may be difficult to validate. We undertook a type of validation using independent chromatin data, when there was comparable chromatin data available. However, this type of validation involves a number of challenges. First, we often do not have chromatin data in the same tissues and cell types as the gene expression data. Second, it is not clear that we should always expect results to replicate; for example, it is biologically plausible that SNPs near specifically expressed genes in the relevant tissue are enriched, while SNPs in H3K36me3 peaks called in the tissue are not. Third, our gene expression annotations represent relative activity—we select genes with higher expression in the focal tissue compared to other tissues—while the chromatin annotations that we use here represent absolute activity (although relative chromatin annotations are also possible6,66). Despite these limitations, replicating an enrichment for a particular system, tissue, or cell type using independent chromatin data can provide a strong validation for gene expression results.
Our power to identify disease-relevant tissues and cell types will improve as large GWAS sample sizes become available for more phenotypes, and as gene expression data is generated in new tissues and cell types. This will help advance our understanding of disease biology and lay the groundwork for future experiments exploring specific variants and mechanisms.
ONLINE METHODS
Computing t-statistics
When computing the t-statistic of each gene for a focal tissue, we excluded all samples from similar tissues category (described for each data set below). For example, when computing the t-statistic of specific expression for each gene in cortex using GTEx data, we compared expression in cortex samples to expression in all other samples, excluding other brain regions. We chose to exclude other brain regions because we wanted to include genes that are more highly expressed in brain tissues than in non-brain tissues, even if they are not specific to cortex within the brain. This procedure results in a higher correlation among the t-statistics for the different brain regions; in a separate analysis, we compute within-brain t-statistics to disentangle this signal.
Thus, for a focal tissue (e.g., cortex) in a larger tissue category (e.g., brain), we computed the t-statistic for gene g as follows. We first constructed a design matrix X where each row corresponds to a sample either in cortex or outside of the brain. The first column of X has a 1 for every cortex sample and a -1 for every non-brain sample. The remaining columns are an intercept and covariates (see below). The outcome Y in our model is expression. We fit this model via ordinary least squares, and compute a t-statistic for the first explanatory variable in the standard way:
where MSE is the mean squared error of the fitted model; i.e.,
where N is the number of rows in X. This gives us a t-statistic for each gene for the focal tissue. We then select the top 10% of genes, add a 100kb window around their transcribed regions, and apply stratified LD score regression to the resulting genome annotations as described below.
For visualization purposes and discussion of results, it is often useful to color tissues or cell types according to a categorization; the categorization for visualization is not always the same as the categorization for computing t-statistics. We give the categorization for visualization in the supplementary tables listed in the respective figure captions.
Modifications of our approach
For some analyses, we modified our approach to constructing sets of specifically expressed genes to better take advantage of the data available.
Franke lab data set. The values in the publicly available matrix are not a quantification of expression intensity, but rather a quantification of differential expression relative to other tissues in this data set17,18. Thus, it was not appropriate to compute t-statistics in this data set. We used the original values in place of our t-statistics, then proceeded as described in Figure 1.
Cahoy data set. The data set of Cahoy et al. had available sets of specifically expressed genes for the three cell types that each had between 1,700 and 2,100 genes. We took these to be the gene sets for the three cell types, then proceeded as in the standard approach, adding a 100kb window and applying stratified LD score regression.
PsychENCODE data set. The PsychENCODE data set had available t-statistics for GABAergic neurons vs. Glutamatergic neurons. We used these t-statistics, rather than computing our own.
For the other data sets we analyzed (GTEx, GTEx brain regions, ImmGen), we used the approach described in Figure 1. We view it as an advantage of our method that it can be flexibly adapted to many different types of data.
Application of stratified LD score regression
Stratified LD score regression7 is a method for partitioning heritability. Given (potentially overlapping) genomic annotations
(If the genomic annotations are real-valued rather than subsets of SNPs, we can replace
Under this model, the expected marginal chi-square association statistic for SNP i reflects the causal contributions not only of SNP i but of SNPs in LD with SNP i. Specifically,
where N is the GWAS sample size, a is a constant that reflects population structure and other sources of confounding,68 and
The regression coefficient
We analyzed autosomes only and excluded the HLA from all analyses. In each analysis, we jointly fit the following annotations:
The annotation created for our focal tissue by adding 100kb windows around the top 10% of genes ranked by t-statistic.
An identical annotation created for all genes included in the gene expression data set being analyzed.
The baseline model with 52 functional categories, described previously7 and listed in Table S1.
GTEx data set
We downloaded the RNA-seq read counts from GTEx v6p (see URLs), removed genes for which fewer than 4 samples had at least one read count per million, removed samples for which fewer than 100 genes had at least one read count per million, and applied TPM normalization69. We analyzed 53 tissues with an average of 161 samples per tissue. We used the “SMTSD” variable (“Tissue Type, more specific detail of tissue type”) to define our tissues and the “SMTS” variable (“Tissue Type, area from which the tissue sample was taken”) to define the tissue categories for t-statistic computation (Table S2). We used age and sex as covariates for our t-statistics.
Franke lab data set
The Franke lab data set is an aggregation of publicly available microarray gene expression data sets comprising 37,427 samples in human, mouse, and rat17,18. We downloaded the publicly available gene expression data from the DEPICT website (see URLs). The available gene expression values already quantify relative expression for a tissue/cell-type rather than absolute expression for a single sample17,18, and so we used these values in place of our t-statistics. We determined that several pairs of tissues had values that were correlated at r2>0.99, including several that had r2=1. We pruned our data so that no two tissues had r2>0.99. Most of the closely correlated pairs were also biologically closely related so that the interpretation did not depend on which tissue we chose to keep (e.g., plasma and plasma cells, joint and joint capsule). For pairs of tissues where one tissue was more specific than the second, we kept the more specific pair (e.g., nose vs. nasal mucosa, quadriceps muscle vs. skeletal muscle). There were two clusters of highly correlated tissues for which we decided to remove the entire cluster, not keeping any of the tissues, because these clusters had very strong but biologically implausible correlations. The first such cluster was made up of eyelids, conjunctiva, anterior eye segment, tarsal bones, foot bones, and bones of the lower extremity. The second such cluster was made up of connective tissue, bone and bones, skeleton, and bone marrow. After pruning, this data set contained 152 tissues, listed in Table S3.
UK Biobank data
We analyzed data from the full N=500K UK Biobank release23 for 13 traits (P.R. Loh et al., unpublished data). The summary statistics were generated using BOLT-LMM v2.3, an unpublished extension of BOLT-LMM70.
Enrichment correlation
For a pair of phenotypes and a set of tissues/cell types, we defined the enrichment correlation to be the correlation between the regression coefficients corresponding to each tissue/cell type. We estimated the enrichment correlation by correlating the estimates of the regression coefficients, and we quantified uncertainty via block jackknife over 200 sets of consecutive SNPs. We note that when the number of tissues/cell types included is small, the true underlying enrichment correlation may be large even though there is no relationship between the two phenotypes, so we only estimate enrichment correlations when there are at least 10 tissues or cell types.
Distribution of P-values
The correlation structure among annotations can lead to a distribution of P values that is highly non-uniform with many P-values close to 0 or 1 (Figure 2). This is caused by our one-sided test for enrichment, testing whether the regression coefficient—which represents the change in per-SNP heritability due to a given annotation, beyond what is explained by the set of all genes as well as the baseline model—is positive. The P-values near 0 occur due to correlated annotations with true signal, and the P-values near 1 occur due to annotations without true signal that, conditional on the baseline model, are negatively correlated to annotations with true signal as a consequence of our construction of sets of specifically expressed genes; these annotations thus have negative regression coefficients.
Chromatin-based annotations
We downloaded narrow peaks from the Roadmap Epigenomics consortium for DNase hypersensitivity and five activating histone marks: H3K27ac, H3K4me3, H3K4me1, H3K9ac, and H3K36me3 (see URLs). Each of these six features was present in a subset of the 88 primary cell types/tissues, for a total of 397 cell-type-/tissue-specific annotations. We also analyzed peaks called using Homer from EN-TEx, a subgroup of the ENCODE project, for four activating histone marks: H3K27ac, H3K4m3, H3K4me1, and H3K36me3. Each of these four features was present in a subset of 27 tissues that were also included in the GTEx data set, for a total of 93 cell-type-/tissue-specific annotations. For each of these two datasets, of each of the annotations, we tested for enrichment by adding the annotation to the baseline model (see Table S1), together with the union of cell-type-specific annotations within each mark and the average of cell-type-specific annotations within each mark. A positive regression coefficient for a tissue-/cell-type-specific annotation represents a positive contribution of the annotation to per-SNP heritability, conditional on the other annotations. We again computed a P-value to test whether the regression coefficient was positive.
Our analysis of chromatin in this work differs from our previous analysis of chromatin data7 in three ways. First, we use a larger range of marks and tissues/cell types: every track available from the Roadmap Epigenomics website (see URLs) for any of six activating marks, H3K27ac, H3K4me1, H3K4me3, H3K9ac, H3K36me3, and DHS, in any of the 88 primary tissues and cell types available, in addition to recent EN-TEx data. Second, for our analysis of Roadmap data, we used narrow peaks from Roadmap for all of the marks. Previously, we analyzed H3K27ac data from one source6 and H3K4me1, H3K4me3, and H3K9ac data from another source5,12; now that there is a single standard source with uniformly processed data for all Roadmap data, we have switched to using this data. Finally, we controlled more strictly for confounders by including the average across cell types of the cell-type-specific annotations for a given mark as an annotation in the model, so that annotations that tend to fall in areas that are more active overall are not falsely interpreted as cell-type-specific signal.
Classification of tissues/cell types for system-level validation of the results of the multiple-tissue analysis of gene expression
We used the classification for visualization used in Figure 2, classifying the top tissue or cell type for each trait with a significant enrichment into one of the eight systems (excluding “Other”) in the Figure 2 legend. There were three phenotypes whose top tissue fell in the “Other” category; two of these we classified into a new “Reproductive” category. The last one, serous membrane, did not have any comparable tissues in our chromatin data and so we instead attempted to replicate the second most significant result for that phenotype.
Multiple-tissue validation results
The top enrichment from our multi-tissue analysis of gene expression was validated at the system level for 33 out of 34 phenotypes, and at the tissue level for 13 out of 20 (Results). If we allowed an enrichment of any artery sample in GTEx to be validated by an enrichment of any artery sample in EN-TEx (instead of requiring strict matching of aorta, tibial artery, and coronary artery), the number of validations rose from 13 to 16. Of the four remaining results that were not validated, three were an enrichment in lung for an immunological disease; for all three diseases, the top enrichment in the analysis of gene expression (not restricting to tissues shared between GTEx and EN-TEx) was an immune category from the Franke lab dataset, and the top enrichment in the analysis of chromatin data was an immune category in the Roadmap dataset. We hypothesize that the lung samples analyzed in GTEx may have contained substantial amounts of blood and thus exhibited a gene expression signature reflecting immune activity; this is supported by a GO enrichment analysis of the lung gene set, in which the top three results were related to antigen presentation, immune response, and cytokine-mediated signaling, respectively.
Heritability enrichments of chromatin-based annotations
Aggregating all results of the Roadmap and EN-TEx chromatin analyses, at least one tissue was significant at FDR<5% for 44 of the 48 traits (Figure S5 and Tables S5 and S7). Averaging across the most significant annotation for each of these 44 traits, the tissue-specific chromatin annotation spanned 3.3% of the genome and explained 43% of the SNP-heritability (Table S5). The sizes of the annotation ranged from 0.8% to 7.8%, and the estimates of enrichment varied from 3.5× to 33×, representing much more variability than for the top annotations in the multiple-tissue gene expression analysis. Because the annotations were much smaller, the estimates of proportion of heritability tended to be much noisier.
Phenotypes with CNS enrichment
The following 12 traits had CNS enrichment at FDR<5% in either the multiple tissue analysis of gene expression or in the analysis of chromatin data above: schizophrenia, bipolar disorder, Tourette syndrome, epilepsy, generalized epilepsy, ADHD, migraine, depressive symptoms, BMI, smoking status, years of education, and neuroticism. The nervous system has been implicated, either with genetic evidence or non-genetic evidence, for each of these traits7,34,24,32,45,71–73.
Analysis of 13 brain regions using data from GTEx
While the multiple-tissue analysis included annotations for many different brain regions, the gene sets for the different brain regions were often highly overlapping so that for many traits, many brain regions were identified as enriched. For example, nearly every brain region in either the GTEx or Franke lab data was found to be enriched at FDR<5% in schizophrenia (Figure 2). To differentiate among brain regions, we restricted ourselves to gene expression data only from samples from the brain in the GTEx data. We computed t-statistics within the brain-only data set; e.g. we computed t-statistics for cortex vs. other brain regions instead of cortex vs. other tissues in GTEx, and we used these new t-statistics to construct and test gene sets as in the multiple-tissue analysis. In this analysis, we set each tissue to be its own category for computation of t-statistics, and we used age and sex as covariates. Individual-level data was not available for the Franke lab data set, and thus we could not compute within-brain t-statistics for this data set.
An alternative approach would be to undertake a joint analysis of the original 13 annotations from the multiple-tissue analysis. However, joint analysis of 13 highly correlated annotations is likely to be underpowered, while re-computing t-statistics within the brain allows us to construct new annotations with lower correlations (Figure S7), increasing our power. Moreover, differential expression within the brain may allow us to isolate signals from cell types or processes that are unique to a single brain region, separately from the cell types or processes that are unique to the brain but shared among brain regions. Thus, we use differential expression within the brain, rather than joint analysis of the original annotations, to differentiate among brain regions.
Data on three brain cell types from Cahoy et al.19
The authors of Cahoy et al.19 purified neurons, astrocytes, and oligodendrocytes from mouse forebrain, and made lists of specifically expressed genes available for each of these three cell types, which we downloaded (see URLs). To obtain a list of all genes, we also downloaded a list of all genes that passed quality control in their analysis (Table S3b of Cahoy et al.). We mapped from mouse to human genes using orthologs from ENSEMBL (see URLs).
Data on two neuron types from PsychENCODE20
PsychENCODE20 generated RNAseq data from the nuclei of GABAergic and Glutamatergic neurons from the dorsolateral prefrontal cortex of four neurotypical human donors, and computed t-statistics using limma74. We used these t-statistics.
Phenotypes with immune enrichment
Twenty-five traits had immune enrichment at FDR<5% in either the multiple tissue analysis of gene expression or in the analysis of chromatin data. This includes many immunological disorders: celiac disease, Crohn’s disease, inflammatory bowel disease, lupus, primary biliary cirrhosis, rheumatoid arthritis, type 1 diabetes, ulcerative colitis, asthma, eczema, and multiple sclerosis. It also includes Alzheimer’s and Parkinson’s diseases, which are neurodegenerative diseases with an immune component previously identified from genetics75,76, as well as several brain-related traits—ADHD, anorexia nervosa, bipolar disorder, schizophrenia, Tourette syndrome, and neuroticism—and HDL, LDL, triglycerides, diastolic and systolic blood pressure, hypertension, and BMI. Several of the brain-related traits have been previously suggested to have an immune component32,77,78; HDL, LDL, and triglycerides have been linked to immune activation79–82; immune cells are causally involved in blood pressure and hypertension83; and obesity, in addition to contributing to inflammation84, can also be induced in mice through alterations of the immune system85.
Data on 292 immune cell types from ImmGen
We downloaded publicly available microarray gene expression data on 292 immune cell types from the ImmGen Consortium (see URLs). We used both Phase 1 (GSE15907) and Phase 2 (GSE37448) data. The data on GEO were on an exponential scale, so we log transformed the data and mapped to human genes using ENSEMBL orthologs. We defined tissue categories for t-statistic computation using the classification on the main page of immgen.org of cell types into categories: B cells, gamma delta T cells, alpha beta T cells, innate lymphocytes, myeloid cells, stromal cells, and stem cells (Table S10). The classification at immgen.org also has a “T cell activation” category that we collapsed into the alpha beta T cell category because it had data on alpha beta T cells at different stages of activation. We did not include any covariates.
Validation of immune results
To validate the results of the ImmGen analysis, we analyzed ATAC-seq peaks from 13 cell types spanning the hematopoietic hierarchy in humans64. The 13 cell types did not allow us to validate at very high resolution; instead, we classified all cell types from ImmGen and from the hematopoiesis data set using the classification for visualization of Figure 5 into five categories: B cells, T cells, NK cells, myeloid cells, and other cells. There were no stromal cells in the hematopoiesis data set and it was not possible to validate the enrichments for diastolic and systolic blood pressure; this left us with 14 phenotypes with an enrichment at FDR<5% in the ImmGen analysis where the top result fell into one of the first four categories (excluding “Other”). We considered one of these 14 results to be validated if any cell type in the same category from the hematopoiesis data set passed FDR<5%. The four phenotypes whose top results did not replicate were Lupus, schizophrenia, bipolar disorder, and neuroticism.
Differences between LDSC-SEG and eQTL-based approaches
Our approach differs in several key ways from approaches that require eQTL data3,13. First, our approach can be applied to expression data sets such as the Franke lab data set, the Cahoy data set, the PsychENCODE data set, and the ImmGen data set that do not have genotypes or eQTLs available (Table 1). Second, methods based on eQTLs require gene expression sample sizes that are large enough to detect eQTLs. In an analysis of data from the GTEx project, we determined that we could identify strong enrichments such as brain enrichment for schizophrenia with just one brain sample, though subtler enrichments had decreasing levels of significance as the gene expression data were down-sampled (Figure S11, Supplementary Note). Results from our analysis of ImmGen data, which has 2.8 samples per cell type on average, confirm that LDSC-SEG can identify significant enrichments even when the gene expression data has a small number of samples per tissue/cell type, in contrast to eQTL-based methods. Finally, we note that a recent study86 tested 30 phenotypes for tissue-specific enrichment in 44 tissues from GTEx using the TWAS approach87 but concluded that their results “did not suggest tissue-specific enrichment at the current sample sizes.” We share their hypothesis that this is because eQTLs are often shared across tissues even when overall expression levels are very different.
Comparison of gene expression and chromatin for cell-type specific analysis
Our estimated enrichments were higher for the chromatin-based annotations than for the gene expression-based annotations, but the gene expression-based annotations are larger and have less LD to the rest of the genome. Some chromatin marks tend to be more cell type-specific than overall gene expression, but our specifically expressed gene sets have low correlation across tissues (Figure S17). There were two instances in which we had gene expression and chromatin data on the same set of tissues/cell types, and we compared the P-values in our analyses of these data sets. First, we compared our results from GTEx (gene expression) and EN-TEx (chromatin) for the tissues shared between these two data sets in the multiple-tissue analysis, and we found that the two data sets had comparable distributions of P-values (Figure S4). On the other hand, the hematopoietic data set that we analyzed64 had matched ATAC-seq and RNA-seq data, and while our analysis of the ATAC-seq peaks lead to significant enrichments for many traits (Figure 5, Table S10), the RNA-seq data set yielded only a single enrichment for a single trait (Table S16).
Data availability
We have released all genome annotations derived from the publicly available gene expression data that we analyzed at http://data.broadinstitute.org/ alkesgroup/LDSCORE/. This includes all annotations used in Figures 2–5 with the exception of the annotations derived from the PsychENCODE data in Figure 4c, for which we did not have permission to release annotations.
Code availability
Open source software implementing our approach is available at http://www.github.com/bulik/ldsc.
Acknowledgments
We are thankful to R Herbst, E Hodis, F Hormozdiari, M Kanai, T Pers, S Riesenfeld, J Ulirsch, and A Veres for helpful comments. This research has been conducted using the UK Biobank Resource (Application Number: 16549). This research was funded by NIH grants R01 MH107649, R01 MH109978, U01 CA194393, and U01 HG009379. HKF was supported by the Fannie and John Hertz Foundation and by Eric and Wendy Schmidt. The data on neuron types were generated as part of the PsychENCODE Consortium, supported by: U01MH103339, U01MH103365, U01MH103392, U01MH103340, U01MH103346, R01MH105472, R01MH094714, R01MH105898, R21MH102791, R21MH105881, R21MH103877, and P50MH106934 awarded to: Schahram Akbarian (Icahn School of Medicine at Mount Sinai), Gregory Crawford (Duke), Stella Dracheva (Icahn School of Medicine at Mount Sinai), Peggy Farnham (USC), Mark Gerstein (Yale), Daniel Geschwind (UCLA), Thomas M. Hyde (LIBD), Andrew Jaffe (LIBD), James A. Knowles (USC), Chunyu Liu (UIC), Dalila Pinto (Icahn School of Medicine at Mount Sinai), Nenad Sestan (Yale), Pamela Sklar (Icahn School of Medicine at Mount Sinai), Matthew State (UCSF), Patrick Sullivan (UNC), Flora Vaccarino (Yale), Sherman Weissman (Yale), Kevin White (UChicago) and Peter Zandi (JHU).
Footnotes
AUTHOR CONTRIBUTIONS
H.K.F. and A.L.P. designed the study. H.K.F., Y.A.R., K.S., and S.P. analyzed data. H.K.F. and A.L.P. wrote the manuscript with assistance from Y.A.R., V.A., K.S., A.G., A.B., S.G., P.R.L., C.L., N.S., G.G., A.S., E.M., S.P., J.R.B.P., J.D.B., B.E.B., S.R., S.M., and B.M.N.COMPETING FINANCIAL INTERESTS
The authors declare no competing financial interests.
URLs
LDSC software, including LDSC-SEG: https://github.com/bulik/ldsc.
Gene sets and LD scores from this paper: https://data.broadinstitute.org/alkesgroup/LDSCORE/.
GTEx: http://www.gtexportal.org)
Franke lab data: https://data.broadinstitute.org/mpg/depict/depict_download/tissue_expression.
Cahoy et al. data: http://jneurosci.org/content/suppl/2008/01/03/28.1.264.DC1, see Tables S4-S6.
PsychENCODE: https://www.synapse.org//#!Synapse:syn4921369/wiki/235539.
ImmGen, https://www.immgen.org/
Roadmap Epigenomics: http://www.roadmapepigenomics.org.
GERA data set (database of Genotypes and Phenotypes (dbGaP), phs000674.v1.p1): http://www-ncbi-nlm-nih-gov.libproxy.mit.edu/projects/gap/cgi-bin/study.cgi?study_id=phs000674.v1.p1.
makegenes.sh: https://github.com/freeseek/gwaspipeline
References
Full text links
Read article at publisher's site: https://doi.org/10.1038/s41588-018-0081-4
Read article for free, from open access legal sources, via Unpaywall:
https://europepmc.org/articles/pmc5896795?pdf=render
Citations & impact
Impact metrics
Citations of article over time
Alternative metrics
Smart citations by scite.ai
Explore citation contexts and check if this article has been
supported or disputed.
https://scite.ai/reports/10.1038/s41588-018-0081-4
Article citations
Discovery and prioritization of genetic determinants of kidney function in 297,355 individuals from Taiwan and Japan.
Nat Commun, 15(1):9317, 29 Oct 2024
Cited by: 0 articles | PMID: 39472450 | PMCID: PMC11522641
Temporally distinct 3D multi-omic dynamics in the developing human brain.
Nature, 635(8038):481-489, 09 Oct 2024
Cited by: 0 articles | PMID: 39385032 | PMCID: PMC11560841
Dissecting shared genetic architecture between depression and body mass index.
BMC Med, 22(1):455, 11 Oct 2024
Cited by: 0 articles | PMID: 39394142 | PMCID: PMC11481102
A cell type-aware framework for nominating non-coding variants in Mendelian regulatory disorders.
Nat Commun, 15(1):8268, 27 Sep 2024
Cited by: 1 article | PMID: 39333082 | PMCID: PMC11436875
Multivariate genomic analysis of 5 million people elucidates the genetic architecture of shared components of the metabolic syndrome.
Nat Genet, 56(11):2380-2391, 30 Sep 2024
Cited by: 0 articles | PMID: 39349817 | PMCID: PMC11549047
Go to all (482) article citations
Data
Data behind the article
This data has been text mined from the article, or deposited into data resources.
BioStudies: supplemental material and supporting data
dbGaP - The database of Genotypes and Phenotypes
- (2 citations) dbGaP - phs000674
Similar Articles
To arrive at the top five similar articles we use a word-weighted algorithm to compare words from the Title and Abstract of each citation.
Leveraging mouse chromatin data for heritability enrichment informs common disease architecture and reveals cortical layer contributions to schizophrenia.
Genome Res, 30(4):528-539, 17 Apr 2020
Cited by: 14 articles | PMID: 32303558 | PMCID: PMC7197474
Exploring shared genetic bases and causal relationships of schizophrenia and bipolar disorder with 28 cardiovascular and metabolic traits.
Psychol Med, 49(8):1286-1298, 26 Jul 2018
Cited by: 30 articles | PMID: 30045777
Genome-wide association study followed by trans-ancestry meta-analysis identify 17 new risk loci for schizophrenia.
BMC Med, 19(1):177, 12 Aug 2021
Cited by: 11 articles | PMID: 34380480 | PMCID: PMC8359304
Polygenic risk scores in imaging genetics: Usefulness and applications.
J Psychopharmacol, 29(8):867-871, 05 May 2015
Cited by: 35 articles | PMID: 25944849
Review
Funding
Funders who supported this work.
Medical Research Council (1)
Child Growth and development
Dr Kenneth Ong, University of Cambridge
Grant ID: MC_UU_12015/2
NCI NIH HHS (2)
Grant ID: U01 CA194393
Grant ID: R01 CA194393
NHGRI NIH HHS (2)
Grant ID: T32 HG002295
Grant ID: U01 HG009379
NIAMS NIH HHS (2)
Grant ID: R01 AR063759
Grant ID: UH2 AR067677
NIGMS NIH HHS (1)
Grant ID: T32 GM007753
NIMH NIH HHS (14)
Grant ID: P50 MH106934
Grant ID: R01 MH105472
Grant ID: R01 MH105898
Grant ID: R01 MH109978
Grant ID: U01 MH103340
Grant ID: U01 MH103346
Grant ID: U01 MH103365
Grant ID: R01 MH094714
Grant ID: R01 MH107649
Grant ID: R21 MH105881
Grant ID: R21 MH102791
Grant ID: R21 MH103877
Grant ID: U01 MH103339
Grant ID: U01 MH103392
Novo Nordisk Fonden (1)
Grant ID: NNF16OC0022018