Abstract
Free full text

Time-Dependent Associations Between Body Composition, Physical Activity, and Current Asthma in Women: A Marginal Structural Modeling Analysis
Associated Data
Abstract
The role of obesity in adult asthma is well-known and has been partly attributed to a confounding role of physical inactivity. However, the interrelationships between obesity, physical activity, and asthma have been incompletely addressed, probably because their time-dependent and bidirectional nature represents a methodologically challenging research question. We aimed to estimate the independent causal effects of body mass index (BMI; weight (kg)/height (m)2) and physical activity on current asthma using marginal structural models (MSMs). MSMs were applied to 15,353 adult women from a 2011 case-control study of asthma (Asthma-E3N) nested within the French E3N study (Etude Epidémiologique auprès de Femmes de la Mutuelle Générale de l'Education Nationale). Three time periods (1997-2000-2002, 2000-2002-2005, and 2002-2005-2011) were defined, where exposures (BMI and physical activity) were measured at time t, outcome (current asthma) was measured at time t + 1, and covariates were measured at time t − 1 or at baseline. A strong significant and positive dose-response relationship between BMI and current asthma was observed (odds ratios were 0.90 (95% confidence interval (CI): 0.79, 1.03), 1.29 (95% CI: 1.17, 1.42), and 1.87 (95% CI: 1.60, 2.18) for the BMI groups <20.0, 25.0–29.9, and ≥30.0, respectively, versus the normal-weight group (BMI 20.0–24.9)). We found no association between physical activity and current asthma. Our results suggest an independent causal deleterious effect of overweight and obesity on current asthma, whereas no independent causal effect of physical activity was found.
The prevalence of asthma has largely increased for the last 40 years, and asthma has been classified as the 28th greatest (out of 291 diseases) cause of disability-adjusted life years worldwide (1). There is consistent evidence that obesity is associated with an increased risk of asthma in adults, especially women (2, 3). The mechanisms that underlie the obesity-asthma relationship are still unclear. First, the observed association could be due to effects of obesity on lung mechanics, inflammation, or sex hormones, which in turn would lead to increased asthma risk (2). Second, it has been argued that this association could be explained by shared risk factors for obesity and asthma, such as poor diet or a sedentary lifestyle (4). Disentangling the role of physical activity in asthma is specifically relevant because the prevalence of a sedentary lifestyle has been increasing in the last few decades as well. Although several mechanisms have been proposed to support the hypothesis of a role of (in)activity in asthma (5), the evidence linking physical activity to asthma is inconclusive (6–9), with the issue of possible reverse causation (i.e., asthma symptoms’ limiting physical activity) needing to be taken into account (10).
An additional difficulty in research about obesity, physical activity, and asthma is that physical activity and body composition (and therefore obesity) can influence each other (11, 12). In addition, both body composition and physical activity are factors that vary over time. Therefore, in longitudinal studies aiming to assess the association of body composition with asthma, physical activity may be considered as a time-dependent confounder, and vice versa (13, 14). However, the complex interrelationships between body composition, physical activity, and asthma remain poorly studied (15) and require the use of statistical techniques that, unlike standard statistical methods, provide unbiased results in the presence of time-dependent confounding (16, 17). Marginal structural models (MSMs) address time-dependent confounding and at the same time allow estimation of causal effects in observational studies (18), by mimicking a hypothetical randomized experiment via creation of a pseudo-population in which exposed and nonexposed subjects are exchangeable (within levels of the available confounders) (19). This approach still has limited application in respiratory epidemiology (14, 20).
To our knowledge, no study has yet investigated jointly the roles of body composition and physical activity in asthma among adults while addressing time-dependent confounding. Therefore, we aimed to estimate the independent causal effects of physical activity and body composition on current asthma, using MSMs.
METHODS
Study design and periods of interest
The Etude Epidémiologique auprès de Femmes de la Mutuelle Générale de l'Education Nationale (E3N study) is a prospective cohort study being carried out among women in a French national health insurance plan covering mostly teachers (Mutuelle Générale de l'Education Nationale) and the French component of the European Prospective Investigation into Cancer and Nutrition (21). E3N was initiated in 1990 with 98,997 female members of the Mutuelle Générale de l'Education Nationale; the women were aged 40–65 years at baseline. Since 1990, information on lifestyle and medical history has been collected approximately every 2 years by means of self-administered questionnaires. Since 1992, all questionnaires have included a simple repeated question regarding asthma: “Have you ever had an asthma attack?”. In order to improve the respiratory characterization of women from the E3N study, a nested case-control study on asthma (the Asthma-E3N study) was performed in 2011. It included the 7,100 women who had ever had an asthma attack (i.e., women who reported having “asthma” at least once on the main E3N questionnaires) between 1992 and 2005 and 14,200 aged-matched “women without asthma,” who were randomly selected from E3N women who had never reported asthma.
In the current project, we used retrospective information from previous surveys carried out as part of the E3N study, among participants in the Asthma-E3N nested case-control study. To assess the effects of body composition and physical activity at time t on current asthma at time t + 1, we used data on body mass index (BMI; weight (kg)/height (m)2) and physical activity collected in 2000, 2002, and 2005 and data on current asthma collected in 2002, 2005, and 2011. To take into account history of exposure, we hypothesized that BMI at time t depends on physical activity at time t, as well as BMI, physical activity (11), menopausal status (22), and smoking status (23, 24) at time t − 1 (1997, 2000, and 2002, respectively). We hypothesized that physical activity at time t depends on physical activity, BMI (12), asthma status (10), menopausal status (22), and smoking status (24) at time t − 1. These relationships are depicted in Figure Figure1.1. Overall, 3 time periods could be evaluated, each consisting of 3 time points (t − 1, t, and t + 1): The first period was 1997-2000-2002, the second period was 2000-2002-2005, and the third period was 2002-2005-2011.
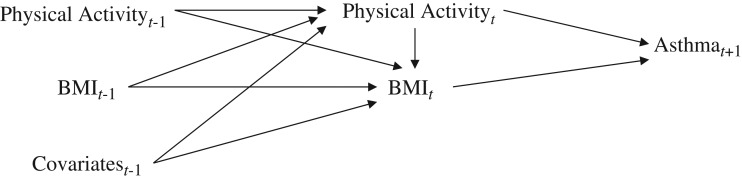
Relationship between time-varying exposures (body mass index (BMI; weight (kg)/height (m)2) and physical activity), the outcome (current asthma), and time-varying covariates (age, education, diet, menopause, smoking status, ever having an asthma attack) in a study of body composition, physical activity, and current asthma, Asthma-E3N Study, 2000–2011. E3N, Etude Epidémiologique auprès de Femmes de la Mutuelle Générale de l'Education Nationale.
Study population
Among the 21,300 women who received the Asthma-E3N questionnaire, only those who responded to at least 4 consecutive questionnaires (i.e., either E3N questionnaires 5 (administered in 1997), 6 (2000), 7 (2002), and 8 (2005) or E3N questionnaires 6, 7, and 8 and the Asthma-E3N questionnaire (2011)) were included in our analysis (n = 17,928), so that at least 2 time periods were evaluated. Because energy intake is an important variable in the relationship between physical activity and body composition, we excluded women who did not complete the food frequency questionnaire, which was administered only in 1993 (n = 2,575). In the end, 15,353 women were included (see Web Figure 1, available at http://aje.oxfordjournals.org/).
In comparison with included women, women excluded from the study population had a significantly higher BMI, used hormone replacement therapy less frequently after menopause, had a lower educational level, and more frequently were current smokers (Web Table 1). Excluded women were also older and reported ever having asthma more frequently (Web Table 1).
Assessment of asthma
Current asthma, the outcome of interest, was defined according to standardized international recommendations (25, 26) as the presence of asthma attacks (i.e., a positive answer to the question “Have you had an asthma attack in the last 12 months?”) and/or receipt of asthma treatment (inhaled bronchodilators or inhaled corticosteroids) in the last 12 months. Asthma attacks were self-reported at all time points. Information on asthma treatment was obtained through a comprehensive drug reimbursement database for the years 2005 and 2011 (27), and asthma treatment was assessed by questionnaire in 2002 (28). Ever having an asthma attack (“ever asthma”) was used as a time-dependent covariate and was computed from answers given to the repeated question on “ever asthma” up to time t − 1 of each period. As was done previously (27), 3 classes were used: “never asthma,” “inconsistent ever asthma” (i.e., at least 1 negative answer to the asthma question after a positive one), and “consistent ever asthma” (i.e., no negative answer to the asthma question after a positive one).
Assessment of body composition
Body composition was assessed using BMI, which was calculated using self-reported height and weight obtained from the main questionnaires sent in 1997, 2000, 2002, and 2005. For BMI considered as a time-dependent exposure, we used the data obtained in 2000, 2002, and 2005 and classified BMI into 4 categories: underweight (<20.0), normal-weight (20.0–24.9), overweight (25.0–29.9), and obese (≥30.0).
For BMI considered as a time-dependent covariate, we used the data obtained in 1997, 2000, and 2002 and employed BMI as a continuous variable to improve convergence (because BMI at time t − 1 is highly correlated with BMI at time t). We defined BMI used as a time-dependent covariate at time t − 1 of a given period as the mean of BMI at t − 1 and BMI at t − 2. To deal with missing data (overall missingness: 3% of observations), if either BMI at t − 1 or BMI at t − 2 was missing, we considered the other variable alone (allowing the use of BMI from the 1995 survey, if needed).
Assessment of physical activity
Physical activity was assessed from the questionnaires sent in 1997, 2002, and 2005 (the questionnaire sent in 2000 did not include questions on physical activity), using data from several questions on different activities. Each specific activity was assigned an intensity code, expressed as the metabolic equivalent of task (MET) based on the ratio of work metabolic rate (i.e., during the activity) to a standard resting metabolic rate (29). We assigned values of 3 METs for walking, 6 METs for moderate recreational activities, 9 METs for vigorous recreational activities, 8 METs for climbing stairs, 2.5 METs for light household activities, and 4 METs for heavy household activities. The amount of time spent per week in each activity was multiplied by its intensity, providing us with an energy expenditure value (MET-hours/week) for each activity. Finally, all MET-hours/week values were added to obtain a weekly total estimate of energy expenditure, as commonly done (29). For the year 2000, we estimated the amount of physical activity as the mean amount of physical activity between 1997 and 2002 (1 questionnaire before and 1 questionnaire after). Because different questions on specific physical activities (e.g., walking, cycling, gardening, cooking) were asked on each questionnaire, the mean physical activity level also differed from one questionnaire to another. Thus, the amount of physical activity was converted into tertiles for each period (a low, moderate, or high level of physical activity).
For physical activity considered as a time-dependent exposure at time t of a given period, we used the physical activity score converted into tertiles in 2000, 2002, and 2005. For physical activity considered as a time-dependent covariate at time t − 1 of a given period, we used the physical activity score converted into tertiles in 1997, 2000, and 2002. To deal with missing data for physical activity used as a time-dependent covariate at time t − 1 of a given period (overall missingness: 2% of observations), we imputed data from the previous questionnaire assessing physical activity (allowing the use of physical activity from the 1993 survey, if needed).
Assessment of time-dependent covariates
Smoking status was considered in 1997, 2000, and 2002 and categorized into 3 classes: never smoker, former smoker, and active smoker. Menopausal status was considered in 1997, 2000, and 2002 and categorized into 3 classes based on age at menopause and information on use of hormone replacement therapy: no menopause, menopause without hormone replacement therapy, and menopause with hormone replacement therapy.
Assessment of fixed covariates
Age was assessed in 1997 and used as a continuous variable. Educational level was assessed in 1990 and categorized into 4 classes: less than high school, high school to level 2 university diploma (equivalent to a US associate's degree), level 3 or 4 university diploma (equivalent to a US bachelor's degree), and level 5 university diploma or more (equivalent to US graduate-level education). Diet was assessed by food frequency questionnaire in 1993, and total energy intake (kcal/day) was used as a continuous variable.
Statistical analysis
Associations estimated in observational studies cannot usually be interpreted as causal effects, because the exposed and unexposed subjects are rarely exchangeable (i.e., exposed and unexposed subjects rarely share the same set of confounder values). The use of MSMs allows the creation of a pseudo-population in which exposed and nonexposed subjects are exchangeable (within levels of the available confounders) and time-dependent confounding has been eliminated, thus leading to unbiased associations that can be interpreted as causal effects (18). For that purpose, each subject is assigned a weight proportional to the inverse of the probability that each subject had his or her own exposure (physical activity or BMI) history at a given time (tth time period), given a chosen set of covariates (30). Standardized weights (SWs) for physical activity/BMI were stabilized and calculated as follows:
and
where SW1(T) and SW2(T) represent the stabilized weights for physical activity and BMI, respectively, at time T; E1 and E2 represent physical activity and BMI, respectively; L represents a vector of time-dependent covariates (smoking status and menopausal status for BMI and smoking status, menopausal status, and asthma for physical activity); and V represents a vector of time-independent (fixed) covariates (age at baseline, total energy intake, and educational level).
The probabilities in the numerator and denominator were estimated through “exposure models”—that is, multinomial logistic regression models for the probability of exposure at each time t. Thus, for each subject, at each given time, a weight for physical activity (i.e., SW1) and a weight for BMI (i.e., SW2) were estimated. The variables included in the exposure models are presented in Table Table1.1. In order to achieve weight stabilization, the set of baseline covariates V was also included in the weight numerator model (in addition to the weight denominator model). MSMs have been shown to be sensitive to the “positivity assumption,” which requires that there be a positive number of subjects (at least 1) in each exposure category, for all levels of the confounders and in all time periods. Because the estimated weight distribution may serve as an indicator of the “positivity assumption” (31), we examined the distribution of weights. The mean of the weights was 1.00. The range of weights was 0.57–1.75 for physical activity, 0.48–1.76 for BMI, and 0.44–1.68 for the total weight. The first and 99th percentiles were 0.81–1.24 for physical activity, 0.85–1.19 for BMI, and 0.75–1.28 for the total weight.
Table 1.
Variables Included in Exposure Models Assessing the Associations of Body Mass Index and Physical Activity With Asthma, Asthma-E3N Study, 2000–2011
Exposure Model | Included Variables |
---|---|
BMIa (t) | |
![]() | Age, time-period indicator, total energy intake, educational level, and BMI (t − 1) |
![]() | Age, time-period indicator, total energy intake, educational level, physical activity (t), physical activity (t − 1), BMI (t − 1), menopausal status (t − 1), and smoking status (t − 1) |
Physical activity (t) | |
![]() | Age, time-period indicator, total energy intake, educational level, and physical activity (t − 1) |
![]() | Age, time-period indicator, total energy intake, educational level, physical activity (t − 1), asthma (t − 1), BMI (t − 1), menopausal status (t − 1), and smoking status (t − 1) |
Abbreviations: BMI, body mass index; E3N, Etude Epidémiologique auprès de Femmes de la Mutuelle Générale de l'Education Nationale.
a Weight (kg)/height (m)2.
The second step consists of estimating the independent effects of physical activity and BMI on current asthma in the reweighted pseudo-population using a joint model. As previously proposed (32, 33), we used a weighted pooled multinomial logistic model in which the final weight was calculated, at each time and for each subject, as the product of SW1 and SW2. Because we did not adjust for the set of baseline covariates V through the weights, these variables were added as covariates in the final model (31).
We performed several sensitivity analyses. First, to evaluate whether observed associations could have been driven by a small number of persons with extreme weights, we used weight truncation (i.e., we reset the values of weights greater than the 99th percentile to the 99th percentile value and the values of weights lower than the first percentile to the first percentile value). Second, and because of the potential overlap between the diagnosis of chronic obstructive pulmonary disease and the diagnosis of asthma, we performed analyses restricted to never smokers (n = 8,062). Third, to consider the possibility that there are important effects of current physical activity status (versus past physical activity status) on body composition, we tested a second model for the calculation of weight for BMI at time t, including only physical activity, BMI, menopausal status, and smoking status at time t − 1 but not physical activity at time t.
RESULTS
The characteristics of the study participants in 2000 (baseline) are presented in Table Table2.2. The women had a mean age of 59 years; 8.5% were active smokers, the mean physical activity level was 71.1 MET-hours/week (standard deviation, 39.3), and the prevalences of overweight and obesity were 23.5% and 6.1%, respectively. The prevalences of current asthma were 11.7%, 16.6%, and 16.0% in 2002, 2005, and 2011, respectively.
Table 2.
Baseline Characteristics of Participants in a Study of Body Composition, Physical Activity, and Current Asthma (n = 15,353), Asthma-E3N Study, 2000
Characteristic | No. of Missing Values | Mean (SD) | % |
---|---|---|---|
Age, years | 0 | 59.2 (6.3) | |
Educational levela | 569 | ||
![]() | 10.7 | ||
![]() ![]() | 51.2 | ||
![]() ![]() | 19.0 | ||
![]() ![]() | 19.2 | ||
Smoking status | 2 | ||
![]() | 52.5 | ||
![]() | 39.0 | ||
![]() | 8.5 | ||
Menopausal status | 136 | ||
![]() | 10.1 | ||
![]() | 20.8 | ||
![]() | 69.1 | ||
Total energy intake, kcal/day | 2,226 (557) | ||
Physical activity, MET-hours/week | 1,005 | 71.1 (39.3) | |
BMIb (continuous) | 149 | 23.7 (3.7) | |
BMI category | 149 | ||
![]() | 12.2 | ||
![]() | 58.2 | ||
![]() | 23.5 | ||
![]() | 6.1 | ||
Ever having an asthma attack | 1 | ||
![]() | 77.3 | ||
![]() | 17.3 | ||
![]() | 5.5 |
Abbreviations: BMI, body mass index; E3N, Etude Epidémiologique auprès de Femmes de la Mutuelle Générale de l'Education Nationale; HRT, hormone replacement therapy; MET, metabolic equivalent of task; SD, standard deviation.
a Measured in 1990.
b Weight (kg)/height (m)2.
We observed a strong significant and positive dose-response association between BMI and current asthma: Odds ratios were 0.90 (95% confidence interval (CI): 0.79, 1.03) for underweight versus normal BMI, 1.29 (95% CI: 1.17, 1.42) for overweight versus normal BMI, and 1.87 (95% CI: 1.60, 2.18) for obesity versus normal BMI (Figure (Figure2).2). We found no association between physical activity and current asthma: Odds ratios were 1.01 (95% CI: 0.93, 1.11) and 1.00 (95% CI: 0.91, 1.10), for moderate and high physical activity levels, respectively, versus a low level of physical activity.
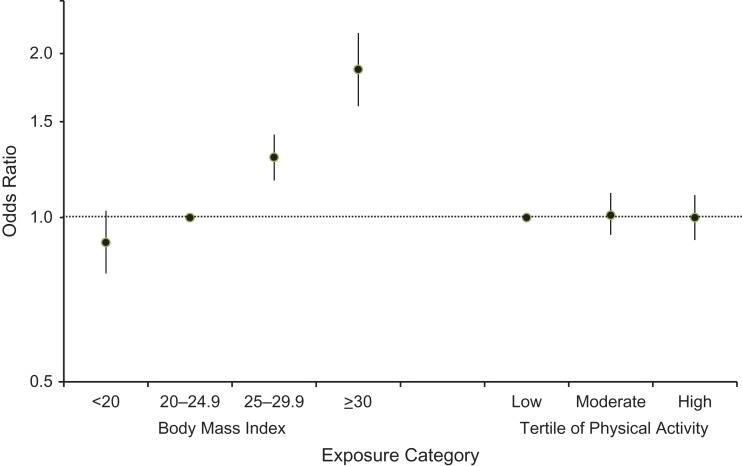
Independent associations of body mass index (weight (kg)/height (m)2) and physical activity (MET-hours/week) with current asthma among 15,353 adult women, Asthma-E3N Study, 2000–2011. Bars, 95% confidence intervals. E3N, Etude Epidémiologique auprès de Femmes de la Mutuelle Générale de l'Education Nationale; MET, metabolic equivalent of task.
When extreme weights were truncated, the odds ratios for the associations between physical activity and current asthma and between BMI and current asthma remained very similar (Table (Table3).3). In the analysis restricted to the 8,062 women who had never smoked, the association between BMI and current asthma remained significant, although the magnitude of the estimates decreased slightly (Table (Table3).3). The analysis using the model not including current physical activity status for the calculation of weight for BMI yielded similar results.
Table 3.
Odds Ratios for the Joint Association of Body Mass Index and Physical Activity With Current Asthma From Marginal Structural Models, After Truncation of Extreme Weights and Among Nonsmokers (Sensitivity Analyses), Asthma-E3N Study, 2000–2011
Exposure | Weight Truncationa | Nonsmokers (n = 8,062) | ||
---|---|---|---|---|
OR | 95% CI | OR | 95% CI | |
Body mass indexb | ||||
![]() | 0.90 | 0.79, 1.03 | 0.86 | 0.71, 1.05 |
![]() | 1.00 | Referent | 1.00 | Referent |
![]() | 1.29 | 1.17, 1.42 | 1.22 | 1.07, 1.40 |
![]() | 1.86 | 1.60, 2.17 | 1.56 | 1.25, 1.96 |
Tertile of physical activity | ||||
![]() | 1.00 | Referent | 1.00 | Referent |
![]() | 1.01 | 0.93, 1.11 | 1.03 | 0.92, 1.16 |
![]() | 1.00 | 0.91, 1.10 | 0.97 | 0.85, 1.11 |
Abbreviations: CI, confidence interval; E3N, Etude Epidémiologique auprès de Femmes de la Mutuelle Générale de l'Education Nationale; OR, odds ratio.
a The values of weights greater than the 99th percentile (i.e., 1.28) were reset to 1.28, and the values of weights lower than the first percentile (i.e., 0.75) were reset to 0.75.
b Weight (kg)/height (m)2.
DISCUSSION
In this longitudinal study, we applied MSMs to disentangle the independent associations of body composition and physical activity with current asthma, while dealing appropriately with their time-dependent interrelationships. Our results suggested that in these women, body composition had an effect on current asthma independently of physical activity, while physical activity showed no effect on current asthma.
Several previous studies have investigated the joint roles of physical activity and body composition as determinants of asthma (13, 34–41), but most of them (34–39, 41) used standard statistical methods, which may have provided biased results in the presence of time-dependent confounding (16, 17). This was not the case in the current study. Our results extend, for asthma activity, the results observed for asthma incidence in 2 previous studies (13, 38). The study by Beckett et al. (38), conducted in adults, found that gains in BMI increased the risk of asthma incidence only in women, while decreased physical activity was not associated with asthma incidence. However, the results of this study could have been biased because it used standard statistical methods. On the contrary, the study by Garcia-Aymerich et al. (13) assessed the 10-year risk of adult-onset asthma in 76,470 women from the Nurses’ Health Study after hypothetical interventions on BMI and physical activity, using the parametric g-formula, which is another method that adjusts for time-dependent confounding (13). That study showed a significant reduction in hypothetical asthma risk associated with a weight loss intervention and a nonsignificant reduction associated with intervention on physical activity level, which is consistent with our results. Overall, evidence supports the conclusion that after controlling for time-dependent confounding due to physical activity, overweight and obesity are still associated with asthma risk in women. Our results regarding the role of obesity in asthma are also supported by animal and human physiological studies (2, 42, 43). The observed lack of effect for physical activity, once time-dependent confounding by body composition has been considered, could suggest that previously reported beneficial effects of physical activity on asthma (8) could be partly mediated by the beneficial effect of physical activity on body composition (32).
MSMs have been increasingly used in the past decade (32, 33, 44–48), but their use to study causes of respiratory health outcomes is still limited (46, 47). Garcia-Aymerich et al. (46) investigated the independent causal effect of physical activity on the development and course of chronic obstructive pulmonary disease using MSMs to adjust for time-dependent confounding, including obesity. That study showed a significant association between regular physical activity and reduced risk of chronic obstructive pulmonary disease development and hospitalization, suggesting a protective effect of physical activity that is independent of body composition. To our knowledge, only 1 study has jointly assessed the independent effects of physical activity and body composition on a health outcome (i.e., functional limitation in the elderly); in that study, Tager et al. (32) concluded that physical activity has beneficial effects on the functioning of elderly persons through changes in body composition.
A limitation of our study was the potential misclassification of asthma, as it was based on self-reporting of asthma attacks and our assessment of current asthma treatment between 2002 and 2011 was heterogeneous. However, we previously reported good consistency between self-reports of asthma attacks and objective data on asthma medication use among E3N women over time (27). Our study was limited by self-reported assessment of covariates and exposures. However, self-reported BMI was found to be highly correlated (correlation = 0.92) with the objective measure obtained by a skilled technician, as previously validated in a subsample of 152 women from the E3N study (49). The potential misclassification bias that could arise from the inclusion of patients with chronic obstructive pulmonary disease as asthmatics can be disregarded, since similar results were obtained when we conducted our analyses among never smokers. The fact that women included in our analyses were “healthier” than excluded women could have led to a selection bias. However, these differences were relatively small in numerical terms and thus were partly a consequence of the large population size. Regarding diet, because it was assessed only once, we could not consider it as a time-dependent exposure. However, the existence of an effect of diet on asthma, as opposed to the role of obesity or, to a lesser extent, physical activity, is far from clear. Thus, potential residual confounding by time-dependent diet would not be expected.
Nonpositivity may be structural if, for a given level of a confounder, subjects are by definition all unexposed (or all exposed) (31); this did not occur in our analyses. Nonpositivity may also occur at random, especially if the number of covariates included in the exposure model is high or if some of the categorical covariates have many levels. However, in the present study, the distribution of weights (mean close to 1, small range) was not indicative of bias in the exposure models (18, 31). When weights are truncated, associations observed using an MSM are expected to be closer to those observed with a standard model, since time-dependent confounding is less controlled for (31). Results obtained after weight truncation suggest that there was not a substantial amount of time-dependent confounding in our study, but this could not have been known without the use of MSMs. Another limitation of our study was the deterministic approach we used to deal with missing data. However, fewer than 3% of observations had missing data for physical activity or BMI, which we assumed to be missing “completely at random” or “at random” (50).
Strengths of this study included its longitudinal design; the availability of repeated measurements for outcome, risk factors, and covariates; and the use of MSMs, which was appropriate to this methodologically challenging research question and also provided causal estimates for the associations of interest. Another strength of this study was the assessment of 2 exposures that were studied conjointly, which has rarely been done (32, 33).
In conclusion, this study suggests that overweight and obesity are causally related to an increased risk of asthma symptoms in women, independently of physical activity. These results are in accordance with current international guidelines which recommend that obese people lose weight, by following a healthy balanced diet and practicing regular exercise (51).
ACKNOWLEDGMENTS
Author affiliations: Aging and Chronic Diseases: Epidemiological and Public Health Approaches, Unit 1168, National Institute of Health and Medical Research (INSERM), Villejuif, France (Annabelle Bédard, Nicole Le Moual, Margaux Sanchez, Orianne Dumas, Raphaëlle Varraso); Mixed Research Unit 1168, University of Versailles St.-Quentin-en-Yvelines, Montigny le Bretonneux, France (Annabelle Bédard, Nicole Le Moual, Margaux Sanchez, Orianne Dumas, Raphaëlle Varraso); Center for Research in Environmental Epidemiology (CREAL), Barcelona, Spain (Ignasi Serra, Xavier Basagaña, Judith Garcia-Aymerich); Pompeu Fabra University, Barcelona, Spain (Ignasi Serra, Xavier Basagaña, Judith Garcia-Aymerich); Biomedical Research Networking Centers in Public Health (CIBERESP), Barcelona, Spain (Ignasi Serra, Xavier Basagaña, Judith Garcia-Aymerich); Gustave Roussy Institute, Lifestyle, Genes, and Health: Integrated Trans-Generational Epidemiology, Unit 1018, Center for Research in Epidemiology and Population Health, INSERM, Villejuif, France (Françoise Clavel-Chapelon); Mixed Research Unit 1018, Paris-Sud University, Villejuif, France (Françoise Clavel-Chapelon); Team of Environmental Epidemiology Applied to Reproduction and Respiratory Health, Albert Bonniot Institute, Grenoble Alpes University, Grenoble, France (Valérie Siroux); Team of Environmental Epidemiology Applied to Reproduction and Respiratory Health, Albert Bonniot Institute, INSERM, Grenoble, France (Valérie Siroux); and Team of Environmental Epidemiology Applied to Reproduction and Respiratory Health, Albert Bonniot Institute, Grenoble University Hospital Center, Grenoble, France (Valérie Siroux).
This research was funded by a CENESA (Causal Effect of Nutritional, Environmental, and Social Factors on Asthma in the E3N Study) grant (Institute of Public Health Research 2013). The study benefited from the joint support of the Direction Gale de la Santé; the Mission recherche de la Direction de la Recherche, des Études, de l’Évaluation, et des Statistiques; the Caisse nationale d'Assurance maladie des Travailleurs salariés; the Régime social des Indépendants; and the Caisse nationale de Solidarité pour l'Autonomie. The main E3N study is supported by the Mutuelle Générale de l'Education Nationale, the French League Against Cancer, the Gustave Roussy Institute, and INSERM.
We thank Marie Fangon, Maryvonne Niravong, and Lyan Hoang (Mixed Research Unit 1018, Center for Research in Epidemiology and Population Health, INSERM) for implementation of the study.
Conflict of interest: none declared.
REFERENCES
Articles from American Journal of Epidemiology are provided here courtesy of Oxford University Press
Full text links
Read article at publisher's site: https://doi.org/10.1093/aje/kwx038
Read article for free, from open access legal sources, via Unpaywall:
https://academic.oup.com/aje/article-pdf/186/1/21/26575716/kwx038.pdf
Citations & impact
Impact metrics
Citations of article over time
Alternative metrics
Smart citations by scite.ai
Explore citation contexts and check if this article has been
supported or disputed.
https://scite.ai/reports/10.1093/aje/kwx038
Article citations
Physical activity, sedentary behaviour, and childhood asthma: a European collaborative analysis.
BMJ Open Respir Res, 11(1):e001630, 15 Aug 2024
Cited by: 0 articles | PMID: 39147399 | PMCID: PMC11331876
Plant-Based Diets and the Incidence of Asthma Symptoms among Elderly Women, and the Mediating Role of Body Mass Index.
Nutrients, 15(1):52, 22 Dec 2022
Cited by: 2 articles | PMID: 36615710 | PMCID: PMC9824479
All Types Obesity and Physical Inactivity Associated with the Risk of Activity of Daily Living Limitations Among People with Asthma.
J Multidiscip Healthc, 15:1573-1583, 22 Jul 2022
Cited by: 0 articles | PMID: 35909421 | PMCID: PMC9326037
Healthy diet associated with better asthma outcomes in elderly women of the French Asthma-E3N study.
Eur J Nutr, 61(5):2555-2569, 27 Feb 2022
Cited by: 3 articles | PMID: 35220443 | PMCID: PMC9279211
The Role of Nutritional Factors in Asthma: Challenges and Opportunities for Epidemiological Research.
Int J Environ Res Public Health, 18(6):3013, 15 Mar 2021
Cited by: 12 articles | PMID: 33804200 | PMCID: PMC7999662
Review Free full text in Europe PMC
Go to all (10) article citations
Data
Data behind the article
This data has been text mined from the article, or deposited into data resources.
BioStudies: supplemental material and supporting data
Similar Articles
To arrive at the top five similar articles we use a word-weighted algorithm to compare words from the Title and Abstract of each citation.
Birth weight, body silhouette over the life course, and incident diabetes in 91,453 middle-aged women from the French Etude Epidemiologique de Femmes de la Mutuelle Generale de l'Education Nationale (E3N) Cohort.
Diabetes Care, 33(2):298-303, 16 Nov 2009
Cited by: 34 articles | PMID: 19918011 | PMCID: PMC2809270
Cohort Profile: The French E3N Cohort Study.
Int J Epidemiol, 44(3):801-809, 10 Sep 2014
Cited by: 73 articles | PMID: 25212479
Fatty acid consumption and incident type 2 diabetes: an 18-year follow-up in the female E3N (Etude Epidémiologique auprès des femmes de la Mutuelle Générale de l'Education Nationale) prospective cohort study.
Br J Nutr, 116(10):1807-1815, 15 Nov 2016
Cited by: 13 articles | PMID: 27842617
Population attributable fractions of the main type 2 diabetes mellitus risk factors in women: Findings from the French E3N cohort.
J Diabetes, 11(3):242-253, 23 Sep 2018
Cited by: 11 articles | PMID: 30098121
Funding
Funders who supported this work.