Abstract
Free full text

Who Can Tolerate a Marginal Kidney? Predicting Survival After Deceased-Donor Kidney Transplantation by Donor-Recipient Combination
Abstract
The impact of donor quality on post-kidney transplant (KT) survival may vary by candidate condition. Characterizing this variation would increase access to KT without sacrificing outcomes. We developed a tool to estimate post-KT survival for combinations of donor quality and candidate condition. We studied deceased-donor KT recipients (N=120,818) and waitlisted candidates (N=376,272) between 2005 and 2016 using the Scientific Registry of Transplant Recipients. Donor quality and candidate condition were measured using Kidney Donor Profile Index (KDPI) and Estimate Post-Transplant Survival (EPTS). We estimated 5-year post-KT survival by combinations of KDPI and EPTS using random forests, and waitlist survival by EPTS using Weibull regressions. Survival benefit was defined as absolute reduction in mortality risk with KT. For candidates with EPTS=80, 5-year waitlist survival was 47.6%, and 5-year post-KT survival was 78.9% after receiving kidneys with KDPI=20 and 70.7% with KDPI=80. The impact of KDPI on survival benefit varied greatly by EPTS. For candidates with low EPTS (e.g.<40), KDPI had limited impact on survival benefit. For candidates with middle or high EPTS (e.g.>40), survival benefit decreased with higher KDPI, but was still substantial even with KDPI=100 (>16 percentage-points). Our prediction tool (www.transplantmodels.com/kdpi-epts) can support individualized decision-making on kidney offers in clinical practice.
INTRODUCTION
Deceased-donor kidney transplant (DDKT) candidates wait a median of 7.6 years for DDKT due to a severe shortage of kidneys available for transplantation (1). However, half of the kidneys from marginal donors, who are older or have medical comorbidities, are discarded after being procured for transplantation (2). Previous studies demonstrated that KT with a marginal kidney confers substantial survival benefit over remaining on waitlist (3–5). Increased utilization of marginal kidneys is a possible solution to mitigate the organ shortage (6).
An important challenge hindering further utilization of marginal kidneys is that an individual candidate’s outcome after DDKT with a marginal kidney may vary. Previous studies reported that certain subgroups, such as younger or non-diabetic recipients, may not receive clear survival benefit from KT with a marginal kidney (3,7). Nonetheless, these examples are not granular enough to be used in practice for making an individualized decision to accept or decline a marginal kidney. Identifying whether an individual candidate would obtain survival benefit is of utmost importance to safely increase marginal kidney utilization. Ongoing concerns include the fact that healthier candidates, who would likely survive for a decade even without immediate KT, may gain little from KT with marginal kidneys (7,8). On the other hand, unhealthy candidates may not have the physiological reserve to tolerate KT with marginal kidneys (9). Little is known about how candidate condition modifies the effect of donor quality on post-KT outcomes, i.e., the interaction between marginal donor quality and candidate condition.
This knowledge gap is a potential reason for the underutilization of marginal kidneys, given that uncertainty leads to risk aversion in clinical practice (10,11). A marginal kidney offer should be considered more favorably for a candidate who is predicted to receive substantial survival benefit with such a kidney. However, there is no tool to guide this prediction. This uncertainty prompts transplant centers to make a “safe” decision to wait for healthier, lower-risk kidney offers rather than to accept the marginal kidney offer in hand. Characterizing the interaction between donor quality and candidate condition could facilitate the use of marginal kidneys and expand access to KT for candidates who could tolerate such kidneys, without sacrificing outcomes.
To address this knowledge gap, we aimed to create a simple and intuitive online tool to estimate the post-KT survival by donor quality, specifically to candidate condition. The purpose of this tool was to support evaluation of marginal kidney offers by predicting whether the offered kidney would provide sufficient survival benefit to the candidate under consideration. We used a machine learning method on national registry data to achieve the highest possible prediction performance.
METHODS
Data Source
This study used data from the Scientific Registry of Transplant Recipients (SRTR). The SRTR data system includes data on all donors, waitlisted candidates, and transplant recipients in the US, submitted by the members of the Organ Procurement and Transplantation Network (OPTN). The Health Resources and Services Administration (HRSA), US Department of Health and Human Services, provides oversight to the activities of the OPTN and SRTR contractors.
Study population
The recipient cohort included all adult (aged ≥ 18 years) deceased-donor kidney-only transplant recipients between January 1, 2005 and December 31, 2016 (n=120,818). The candidate cohort included all adult candidates who were actively registered on the OPTN waitlist between January 1, 2005 and December 31, 2016 (n=376,272); multiple registrations for a single candidate were consolidated. Deaths were ascertained by the OPTN and SRTR from multiple sources, including the Social Security Death Master File and the Centers for Medicare & Medicaid Services (CMS) data (12).
Post-kidney transplant survival
Our primary outcome was post-KT survival, which was defined as the time from KT to death, censoring for the end of the study. Our exposures were the Kidney Donor Profile Index (KDPI) and the Estimated Post-Transplant Survival (EPTS) as metrics of donor and candidate risk (13,14). KDPI is calculated from 10 donor variables and EPTS is from 4 recipient variables. Like other long-term outcome prediction models in kidney transplantation, the predictive performance of KDPI and EPTS is not ideal, with Harrell’s c-statistics around 0.6 (15,16). Albeit this shortcoming, KDPI and EPTS are relevant to our study because they are currently used in the allocation of deceased donor kidneys in the United States. Clinicians can readily obtain KDPI of the offered kidneys and EPTS of the candidates from the allocation system, and are familiar with the concepts of these risk metrics. As such, using these two scores, as opposed to directly modeling on donor and recipient clinical characteristics, grants simplicity and improves usability while maintaining clinical relevance. KDPI and EPTS were calculated as per the 2016 OPTN mapping tables (13,14).
We used the random forests algorithm to predict the post-KT survival by KDPI and EPTS. Random forests can handle complex interactions between multiple predictors with no knowledge or assumptions on how the predictors will interact with each other (17,18). Hence, it allows a more efficient and objective approach to examine the interaction between KDPI and EPTS compared to the generalized linear regression. We used the approach of Ishwaran and colleagues to implement random forests for survival data (19). With this approach, we estimated the survival function over the entire follow-up period. However, our tool presents the survival estimates only at 5- and 10-year post-KT to ensure simplicity and interpretability in clinical practice. Of note, random forests do not provide uncertainty measures, such as standard errors in generalized linear models. Ishwaran and colleagues suggested using the c-statistic as an overall measure of predictive performance (19). The c-statistic of our model was 0.637, which is slightly higher than that of the original KDRI model.
Waitlist survival
We assigned a hypothetical “entry date” to all candidates. Each candidate’s entry date was randomly chosen from the candidate’s follow up period, which began on the first date of active waitlisting and ended on the first date of death, KT, removal from the waitlist, or the end of the study (December 31, 2016). If the candidate was already actively listed at the beginning of the study, the follow up period for the candidate started from the beginning of the study (January 1, 2005). Waitlist survival was defined as the time from the entry date to death, censoring for KT, removal from the waitlist, or the end of the study. We calculated EPTS as of the entry date for the candidate cohort.
Our aim was to predict a candidate’s survival on waitlist from the time point when the candidate receives (and declines) a kidney offer. However, date of kidney offer was not available for all candidates included in our analysis. We assumed that the actual timing when the candidate declines an offer is trivial in the association between EPTS and future survival because EPTS already reflects temporality via two time-varying variables - candidate age and time on dialysis. For example, a 50-year old candidate who spent 3 years on dialysis would show better survival than a 51-year old counterpart who spent 4 years on dialysis. However, because the EPTS of the latter would be accordingly higher to reflect the additional risk accumulated over the 1-year gap, the association between EPTS and survival will be consistent regardless of when they received the kidney offer. We tested this assumption with a sensitivity analysis in which we characterized the association between EPTS and survival at 4 different time points: dialysis initiation and 3, 6, and 9 years since dialysis initiation. Our sensitivity analysis showed that the association between EPTS and survival is consistent across different time points, suggesting that our method can correctly predict survival by EPTS. (Supplementary Figure 1)
We predicted waitlist survival by EPTS using a Weibull model. Unlike the Cox proportional hazard model, the Weibull model can directly estimate the survival function of the individuals (20). In accordance to our post-KT survival analysis, our tool presents the 5- and 10-year waitlist survival. We used the natural cubic splines to characterize the association between EPTS and waitlist survival in a flexible, non-linear functional form (21). KT is a competing event to death: we used the cause-specific hazard method, as opposed to the subdistribution hazard method, in order to predict the survival should the candidate remain on waitlist without receiving a KT (22–24).
Survival benefit
Survival benefit was assessed by calculating the absolute risk reduction in mortality. We estimated the risk difference in percentage points by subtracting post-KT mortality from waitlist mortality. We also estimated relative risk reduction, or risk ratio, by dividing waitlist mortality by post-KT mortality. For both measures, greater values indicate greater survival benefit from KT. Survival benefit was estimated at 5- and 10-year post-KT.
Missing data
In the recipient cohort, there were 2473 missing values for time on dialysis, 936 missing values for recipient diabetes status, 30 missing values for donor serum creatinine, two missing values for donation after cardiac death, and one missing value for donor height. In the candidate cohort, there were 2998 missing values for diabetes status. Missing values for the donor variables were imputed from other variables constituting KDPI, and those for the recipient/candidate variables were from other variables constituting EPTS.
Statistical analysis
Data preparation and calculation of EPTS and KDPI were performed in Stata/MP 15 for Linux. All other statistical analyses including estimation of post-kidney transplant survival and waitlist survival were performed in R 3.4.2.
RESULTS
Population characteristics
In the recipient cohort (n=120,818), 33.1% were African American, 39.7% were female, 14.7% had previous transplants, and 18.1% had peak panel reactive antibody (PRA) ≥ 80. Median recipient age was 54 years and median time on dialysis prior to KT was 3.4 years. These patients received kidneys from donors of whom 39.8% were female, 14.1% were African American, and 15.2% donated after cardiac death. Median donor age was 40 years. The median (interquartile range) of KDPI and EPTS were 49 (25, 71) and 45 (20, 74), respectively. The candidate cohort (n=376,272) had a similar profile as the recipient cohort: 30.0% were African American, 39.5% were female, 11.9% had previous transplants, and 14.0% had peak PRA ≥ 80. Median age at waitlisting was 54 years (Table 1).
Table 1
Population characteristics
Recipient cohort (n=120,818) | Candidate cohort (n=376,272) | |
---|---|---|
Recipient/candidate factors | ||
Age at transplant, y | 54 (44, 63) | |
Age at waitlisting, y | 53 (43, 61) | |
Race | ||
![]() | 43.0% | 44.5% |
![]() | 33.1% | 30.0% |
![]() | 15.6% | 17.0% |
![]() | 8.4% | 8.5% |
Female sex | 39.7% | 39.5% |
Diabetes | 35.0% | 42.5% |
Hypertension | 87.9% | 87.7% |
Peak PRA | ||
![]() | 56.1% | 64.6% |
![]() | 25.7% | 21.4% |
![]() | 18.1% | 14.0% |
Previous Transplants | 14.7% | 11.9% |
Years on dialysis | 3.4 (1.6, 5.6) | |
EPTS | 45 (20, 74) | |
Donor factors | ||
Age, y | 40 (25, 51) | |
Female sex | 39.8% | |
Race | ||
![]() | 68.8% | |
![]() | 14.1% | |
![]() | 13.7% | |
![]() | 3.3% | |
Weight, kg | 78.9 (66.0, 93.0) | |
Height, cm | 171.0 (163.0, 179.0) | |
Diabetes | 7.3% | |
Hypertension | 28.0% | |
Serum Creatinine, mg/dl | 0.9 (0.7, 1.3) | |
Donation after cardiac death | 15.2% | |
Hepatitis C | 2.6% | |
KDPI | 49 (25, 71) | |
Cold ischemic time, hr | 16.9 (11.5, 23.0) |
PRA, panel reactive antibody; EPTS, Estimated Post-transplant Survival; and KDPI, Kidney Donor Profile Index.
Post-kidney transplant survival
In the recipient cohort, the predicted post-KT survival was 84.3% (95% confidence interval [CI], 84.1%–84.6%) at 5 years and 64.4% (95% CI, 64.0%–64.9%) at 10 years. The predicted 5-year survival by KDPI and EPTS ranged from 53.0% with KDPI of 100 and EPTS of 100 to 97.4% with KDPI of 28 and EPTS of 1. The contour plot in Figure 1 depicts the post-KT survival based on EPTS and KDPI.

Predicted survival with waitlist versus kidney transplantation at 5 and 10 years by Estimated Post-transplant Survival (EPTS) and Kidney Donor Profile Index (KDPI). The “Waitlist” panels show the predicted waitlist survival by EPTS (y-axis), and the “Transplantation” panels show the predicted post-kidney transplant (KT) survival by EPTS (y-axis) and KDPI (x-axis). Survival estimates at each KDPI-EPTS combination are shown in numbers and colors, blue color indicating higher survival and darker red indicating lower survival. For example, the predicted 5-year waitlist survival for a candidate with EPTS of 80 is 47.6% (See (a) 5-year survival; “Waitlist” panel). The predicted 5-year post-KT survival is 78.9% if this candidate receives a kidney with KDPI of 20, and 70.7% with KDPI of 80 (See (a) 5-year survival; “Transplantation” panel).
The impact of increasing KDPI on post-KT survival varied by EPTS. For a candidate with EPTS of 20, the predicted 5-year post-KT survival was 93.9% with KDPI of 20 and 89.1% with KDPI of 80. For a candidate with EPTS of 80, the predicted 5-year post-KT survival was 78.9% with KDPI of 20 and 70.7% with KDPI of 80 (Table 2).
Table 2
Examples of 5-year waitlist survival, 5-year post-transplant survival, and survival benefit by different values of Estimated Post-transplant Survival (EPTS) and Kidney Donor Profile Index (KDPI)
EPTS | Waitlist | Transplant, KDPI=20 | Transplant, KDPI=80 | ||||
---|---|---|---|---|---|---|---|
| |||||||
5-year Survival | 5-year Survival | Risk Difference | Risk Ratio | 5-year Survival | Risk Difference | Risk Ratio | |
20 | 74.4% | 93.9% | 19.5 pp | 4.2 | 89.1% | 14.7 pp | 2.3 |
80 | 47.6% | 78.9% | 31.2 pp | 2.5 | 70.7% | 23.1 pp | 1.8 |
Risk differences were calculated in percentage points by subtracting transplant mortality from waitlist mortality. Risk ratios were calculated by dividing waitlist mortality by transplant mortality. PP, percentage point; EPTS, Estimated Post-transplant Survival; and KDPI, Kidney Donor Profile Index.
Waitlist survival
In the candidate cohort, the predicated wait-list survival was 59.2% (95% CI, 58.8%–59.6%) at 5 years and 35.2% (95% CI, 33.7%–36.9%) at 10 years. The predicted 5-year survival monotonically decreased as EPTS increased, from 87.1% with EPTS of 1 to 36.8% with EPTS of 100. The predicted 5-year survival was 74.4% with EPTS of 20 and 47.6% with EPTS of 80 (Table 2).
Survival benefit
The risk difference in 5-year mortality ranged from 2.1 percentage points with EPTS of 1 and KDPI of 100 to 32.2 percentage points with EPTS of 96 and KDPI of 1 (Figure 2a). Risk difference decreased with higher KDPI: the decrease was only slight with low EPTS but notable with middle or high EPTS. For a candidate with EPTS of 20, 5-year mortality decreased by 19.5 percentage points with a kidney that has KDPI of 20 and by 14.7 percentage points with KDPI of 80. For a candidate with EPTS of 80, 5-year mortality decreased by 31.2 percentage points with KDPI of 20 and by 23.1 percentage points with KDPI of 80 (Table 2). Survival benefit was generally greater with higher EPTS. Even with a kidney that has KDPI of 100, 5-year mortality decreased by 16.5 percentage points for candidates with EPTS of 60 and 16.7 percentage points with EPTS of 90.
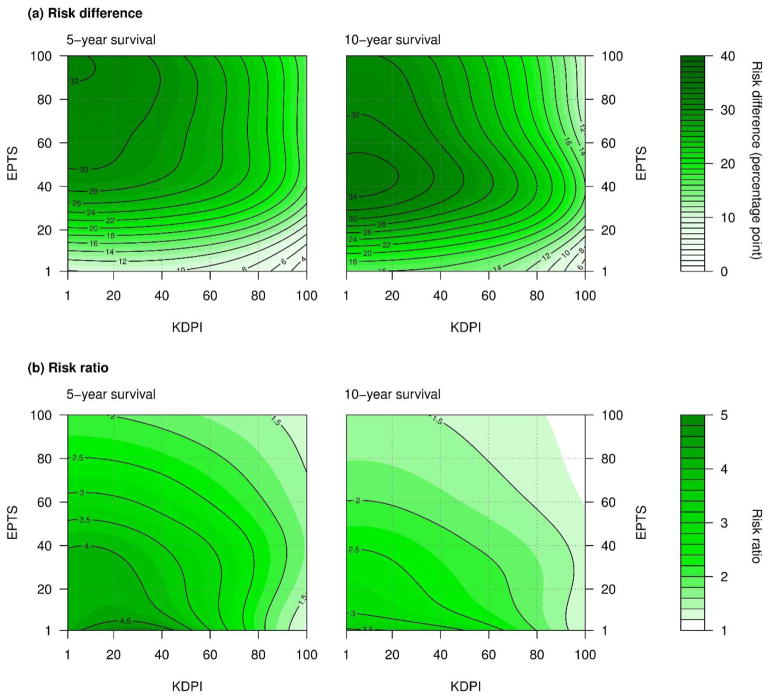
Survival benefit of receiving a kidney transplant versus remaining on waitlist by Estimated Post-transplant Survival (EPTS) and Kidney Donor Profile Index (KDPI). Survival benefit was defined as the reduction in mortality risk with a kidney transplant. Darker color indicates a greater survival benefit. (a) Risk difference, defined as waitlist mortality minus post-kidney transplant (KT) mortality, decreased with higher KDPI. The decrease was only slight with low EPTS and more pronounced with middle or high EPTS. However, even with the highest values of KDPI, individuals with middle or high EPTS are expected to receive substantial survival benefit (>16 percentage-points). (b) Risk ratio, defined as waitlist mortality divided by post-KT mortality, decreased as KDPI increased, particularly when EPTS was low.
The relative reduction in the 5-year mortality risk, measured in risk ratio, ranged from 1.2 with EPTS of 1 and KDPI of 100 to 5.0 with EPTS of 1 and KDPI of 28. Risk ratio also decreased with higher KDPI, more rapidly for candidates with lower EPTS. For a candidate with EPTS of 20, 5-year mortality decreased by 4.2-fold with KDPI of 20 and by 2.3-fold with KDPI of 80. For a candidate with EPTS of 80, 5-year mortality decreased by 2.5-fold with KDPI of 20 and by 1.8-fold with KDPI of 80.
DISCUSSION
In this study of a large nationwide registry of transplant recipients, we estimated post-KT survival and waitlist survival by KDPI and EPTS, using a machine learning algorithm that can address interactions between the two predictors. Receiving KT was associated with a lower mortality risk than remaining on waitlist in all combinations of KDPI and EPTS, with reduction in 5-year mortality ranging from 2.1 to 32.2 percentage points. More importantly, the effect of KDPI on survival benefit was modified by EPTS, suggesting that the potential impact of marginal donor quality should be assessed according to the candidate’s condition. The survival estimates from our models are presented on simple contour plots included in this manuscript. We also created an online tool (www.transplantmodels.com/kdpi-epts) that offers an interactive visualization of the impact of KDPI on survival benefit for a specific value of EPTS (Figure 3).
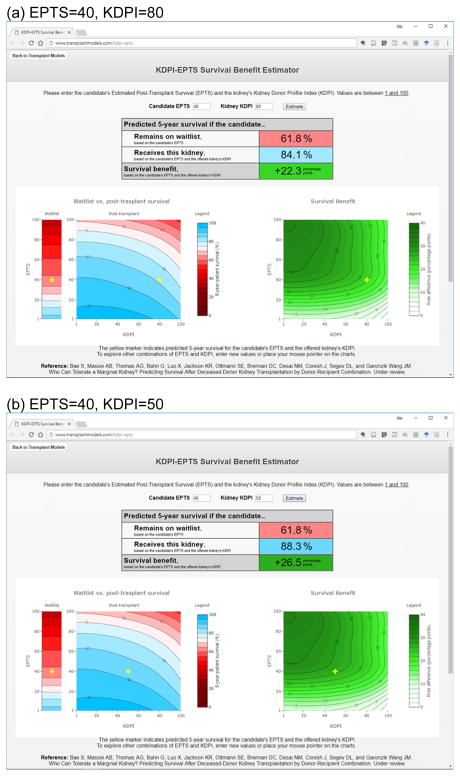
Demonstration of the online risk calculator. (a) EPTS=40, KDPI=80. (b) EPTS=40, KDPI=50. This tool predicts waitlist survival, post-transplant survival, and survival benefit by EPTS and KDPI. In this example, the predicted 5-year waitlist survival of a candidate with EPTS of 40 is 61.8%. Suppose this candidate receives a kidney offer with KDPI of 80. (a) Based on the candidate’s EPTS and the kidney’s KDPI, the predicted post-transplant survival is 84.1%. (b) If this candidate receives the “median” kidney with KDPI of 50, the predicted post-transplant survival is 88.3%. For this candidate, receiving a lower-KDPI kidney results in only a subtle improvement in survival, lending support to accepting the currently offered kidney rather than declining it.
This tool is intended to assist transplant providers with an individualized evaluation of marginal kidney offers. The important question when considering a marginal kidney offer is whether undergoing transplantation with the offered kidney would result in a more favorable survival outcome for the candidate compared to declining the offer in hopes of receiving a lower-KDPI kidney in the future. Using our tool, one can estimate (a) post-KT survival with the offered kidney, (b) waitlist survival, and (c) post-KT survival with a potential kidney with lower KDPI, for the candidate under consideration. If the waitlist survival is predicted to be acceptably high and the post-KT survival would substantially improve with a lower-KDPI kidney, it could be a reasonable decision to decline the currently offered marginal kidney.
Nonetheless, our findings show that declining a marginal kidney offer would not be beneficial in most cases. As shown in Figure 2a, KDPI has little impact in the low and middle range (e.g. <80) among candidates with low EPTS (e.g. <40). For example, the 5-year mortality for a candidate with EPTS of 40 is predicted to decrease by 22.3 percentage points when receiving a kidney with KDPI of 80 and by 26.5 percentage points with KDPI of 50 (i.e. the “median” kidney). The insignificant difference in survival benefit between the two options lends support to accepting a kidney with KDPI of 80 as opposed to declining the offer to wait for a kidney with lower KDPI (Figure 3). On the other hand, candidates with middle and high EPTS (e.g. >40) would receive substantial survival benefit even with kidneys with the highest KDPI. A kidney with KDPI of 100 can still reduce the 5-year mortality by 16.5 percentage points for a candidate with EPTS of 60. This estimate is comparable to the predicted survival benefit with KDPI of 1 and EPTS of 15 (16.4 percentage points). In other words, the survival benefit that middle- and high-EPTS candidates would receive from the highest-KDPI kidneys is comparable to the survival benefit that low-EPTS candidates would receive from the lowest-KDPI kidneys.
Our tool can improve the process of evaluating a kidney offer in several ways. First, the estimates from our tool are specific to the candidate’s condition. We found that the impact of marginal kidney quality on post-KT survival varies substantially by the recipient’s health condition. Second, our tool predicts survival benefit not only with the currently offered kidney but also with potential better-quality kidneys that may become available in the future. A comparison of these estimates may help deciding whether to accept the current kidney offer or to decline it and wait for another. Lastly, the simplicity of our model opens a possibility for shared decision making. Individual candidates waiting for KT have different expectations on their waitlist survival and post-KT survival (25). Hence, candidates would have their own perspectives on how much survival benefit is sufficient to justify the surgical stress, the life-long immunosuppression, and the financial burden associated with KT. Our tool is an easy way to facilitate such discussions between the clinician and the candidate.
Several recent studies have proposed statistical tools to support clinicians making acceptance/decline decisions on kidney offers. Wey and colleagues (26) created a tool to predict if a candidate who declined a kidney offer would subsequently receive a KT and maintain a functioning graft at 3 years after declining the initial kidney offer. This tool is based on candidate characteristics, KDPI of the offered kidney, estimated cold ischemic time, and local kidney supply. Bertsimas and colleagues (27) developed a model to predict whether a candidate would receive a lower-KDPI kidney offer within certain time periods after declining the initial offer. This model is based on local kidney supply, candidate characteristics, and previous kidney offers. Compared to these approaches, our tool is much simpler to use while maintaining predictive accuracy. Our tool requires only two user inputs, EPTS and KDPI, which are supplied to transplant providers by OPTN. In addition, our tool predicts survival benefit for all possible values of KDPI and demonstrates if a lower-KDPI kidney would confer meaningfully superior survival benefit than the currently offered kidney. Taken together, our tool can serve as a quick initial reference to determine whether waiting for a better kidney would be meaningful at all for the specific candidate under consideration, even before consulting more complex models discussed above to predict the candidate’s chance of receiving a better kidney in the future.
Our tool may also lead to an improved utilization of high-KDPI kidneys. Despite the severe shortage of kidneys available for transplantation, the utilization of high-KDPI kidneys is very low. Half of the kidneys with KDPI 81–100 are discarded, despite the fact that they were initially deemed acceptable and procured for KT (2). Even among the kidneys that are eventually transplanted, finding a transplant center that is willing to accept a marginal kidney is often a lengthy and challenging process. A recent study (28) reported that a quarter of the kidneys that were eventually transplanted were declined for 73 times or more before being accepted. Donor quality was the most frequently stated reason for decline: 30.7% of the offers were declined due to “donor age or quality”, and 13.3% were bypassed, i.e. automatically declined, because “minimal acceptance criteria were not met”. Our findings on the interaction between donor quality and candidate condition may possibly reduce unnecessary discard of marginal, yet viable kidneys. Using our tool, clinicians can assess whether the offered marginal kidney would confer sufficient survival benefit to the individual candidate under consideration, rather than taking a risk-averse approach that could remove an opportunity for KT from those who could have benefitted from it.
While we used absolute risk reduction as our primary measure of survival benefit, we also estimated relative risk reduction. Absolute risk reduction is generally more relevant to guiding clinical decisions, whereas relative risk reduction is to characterizing associations (29,30). The risk ratios shown in Figure 2b provide an overview of the interaction between EPTS and KDPI on survival benefit. The impact of KDPI on survival benefit appears to be the mildest when KDPI is low and EPTS is high (the upper left quadrant in Figure 2b) and the greatest when KDPI is high and EPTS is low (the lower right quadrant in Figure 2b). This finding supports that declining high-KDPI kidney offers and waiting for lower-KDPI kidneys could still be a viable strategy for some low-EPTS candidates.
Our study has several limitations. First of all, the combinations of KDPI and EPTS observed in our study is a result of a selection process; in other words, some clinician at some point thought this combination was good, and the bad combinations might have more likely been declined by savvy clinicians. Hence, some of the extreme cases, such as very low EPTS and very high KDPI, might be results of circumstances that necessitate unusual (not commonly practiced or seen) combinations of KDPI and EPTS. Also, the extremes are likely more extrapolative than the middles. Estimates from such cases should be interpreted with caution. Similarly, our prediction model is based on the data of transplanted kidneys. Applying this model to kidneys with extremely high KDPI requires caution since a select subset of such kidneys are transplanted. Lastly, our tool does not include several key factors such as center-specific (or even provider-specific) organ supply and aggressiveness, and the clinical and socioeconomic factors that do not constitute KDPI or EPTS (e.g. histocompatibility between the donor and the recipient and the recipient’s health insurance status). While these are important predictors of post-KT survival, we decided to focus on KDPI and EPTS since our scope was to create a simple tool that can serve as a quick reference for accepting or declining marginal kidney offers.
In conclusion, we developed a unique, simple, provider-friendly tool to predict waitlist survival and post-KT survival for any given combination of donor quality and candidate condition. Our tool provides an individualized prediction of survival benefit from the offered kidney as well as potential better-quality kidneys, assisting clinicians determining whether to accept the currently offered kidney or to wait for a better-quality kidney offer. Our tool is also simple to use and easy to understand, as it only requires EPTS and KDPI from the user and presents the estimates visually. We expect that this tool may reduce unnecessary discards, and assist with appropriate recipient selection for all kidneys from deceased donors.
Supplementary Material
Supp figS1-2
Figure S1: The association of EPTS with 5-/10-year waitlist survival remains similar regardless of time on dialysis.
Figure S2: Distribution of KDPI and EPTS in the study population. (a) Histogram of EPTS. (b) Histogram of KDPI. (c) Heatmap of KDPI-EPTS combination. Darker shade indicates greater number of cases with the KDPI-EPTS combination.
Supp legends
Acknowledgments
This work was supported by grant number K24DK101828 (Segev) from the National Institute of Diabetes and Digestive and Kidney Diseases (NIDDK) and by Mogam Science Scholarship Foundation (Bae). The analyses described here are the responsibility of the authors alone and do not necessarily reflect the views or policies of the Department of Health and Human Services, nor does mention of trade names, commercial products or organizations imply endorsement by the U.S. Government. The data reported here have been supplied by the Minneapolis Medical Research Foundation (MMRF) as the contractor for the Scientific Registry of Transplant Recipients (SRTR). The interpretation and reporting of these data are the responsibility of the author(s) and in no way should be seen as an official policy of or interpretation by the SRTR or the U.S. Government.
Abbreviations
CI | Confidence interval |
CMS | Centers for Medicare and Medicaid Services |
DDKT | Deceased-donor kidney transplant |
EPTS | Estimated Post-Transplant Survival |
HRSA | Health Resources and Services Administration |
KDPI | Kidney Donor Profile Index |
OPTN | Organ Procurement and Transplantation Network |
SRTR | Scientific Registry of Transplant Recipients |
Footnotes
DISCLOSURE
The authors of this manuscript have no conflicts of interest to disclose as described by the American Journal of Transplantation.
Additional Supporting Information may be found online in the supporting information tab for this article.
References
Full text links
Read article at publisher's site: https://doi.org/10.1111/ajt.14978
Read article for free, from open access legal sources, via Unpaywall:
http://www.amjtransplant.org/article/S1600613522089468/pdf
Citations & impact
Impact metrics
Article citations
Present and Future Applications of Artificial Intelligence in Kidney Transplantation.
J Clin Med, 13(19):5939, 05 Oct 2024
Cited by: 0 articles | PMID: 39407999 | PMCID: PMC11478249
Review Free full text in Europe PMC
Program Report: Expanding the Deceased Donor Pool in Manitoba With an Age-Targeted Kidney Transplant Program.
Can J Kidney Health Dis, 11:20543581241287288, 13 Oct 2024
Cited by: 0 articles | PMID: 39403069 | PMCID: PMC11472366
Advancing Kidney Transplantation: A Machine Learning Approach to Enhance Donor-Recipient Matching.
Diagnostics (Basel), 14(19):2119, 25 Sep 2024
Cited by: 0 articles | PMID: 39410523 | PMCID: PMC11475881
Unlocking the benefits of transplantation with kidneys from older donors.
Nat Rev Nephrol, 20(9):562-563, 01 Sep 2024
Cited by: 1 article | PMID: 38890547
Association of Race and Ethnicity With High Longevity Deceased Donor Kidney Transplantation Under the US Kidney Allocation System.
Am J Kidney Dis, 84(4):416-426, 16 Apr 2024
Cited by: 0 articles | PMID: 38636649
Go to all (45) article citations
Data
Data behind the article
This data has been text mined from the article, or deposited into data resources.
BioStudies: supplemental material and supporting data
Similar Articles
To arrive at the top five similar articles we use a word-weighted algorithm to compare words from the Title and Abstract of each citation.
Association of Race and Ethnicity With High Longevity Deceased Donor Kidney Transplantation Under the US Kidney Allocation System.
Am J Kidney Dis, 84(4):416-426, 16 Apr 2024
Cited by: 0 articles | PMID: 38636649
Regional Disparities in Transplantation With Deceased Donor Kidneys With Kidney Donor Profile Index Less Than 20% Among Candidates With Top 20% Estimated Post Transplant Survival.
Prog Transplant, 29(4):354-360, 10 Sep 2019
Cited by: 7 articles | PMID: 31506000 | PMCID: PMC8415134
Allocation of the Highest Quality Kidneys and Transplant Outcomes Under the New Kidney Allocation System.
Am J Kidney Dis, 73(5):605-614, 28 Mar 2019
Cited by: 10 articles | PMID: 30929853
The UNOS Renal Transplant Registry: Review of the Last Decade.
Clin Transpl, 1-12, 01 Jan 2014
Cited by: 13 articles | PMID: 26281122
Review
Funding
Funders who supported this work.
Mogam Science Scholarship Foundation
NIDDK NIH HHS (2)
Grant ID: K23 DK115908
Grant ID: K24 DK101828
National Institute of Diabetes and Digestive and Kidney Diseases (1)
Grant ID: K24DK101828