Abstract
Free full text

Paradoxical effects of obesity on T cell function during tumor progression and PD-1 checkpoint blockade
Abstract
The recent successes of immunotherapy have shifted the paradigm in cancer treatment but since only a percentage of patients respond, it is imperative to identify factors impacting outcome. Obesity is reaching pandemic proportions and is a major risk factor for certain malignancies, but the impact of obesity on immune responses, in general, and in cancer immunotherapy, in particular, is poorly understood. Here we demonstrate, across multiple species and tumor models, that obesity results in increased immune aging, tumor progression and PD-1-mediated T cell dysfunction which is driven, at least in part, by leptin. Strikingly however, obesity is also associated with increased efficacy of PD-1/PD-L1 blockade in both tumor-bearing mice and clinical cancer patients. These findings advance our understanding of obesity-induced immune dysfunction and its consequences in cancer and highlight obesity as a biomarker for some cancer immunotherapies. These data indicate a paradoxical impact of obesity on cancer. There is heightened immune dysfunction and tumor progression but also greater anti-tumor efficacy and survival following checkpoint blockade which directly targets some of the pathways activated in obesity.
Introduction
Recent advances in our understanding of the mechanisms of immune regulation have led to major clinical breakthroughs in cancer, including the use of inhibitors of the PD-1/PD-L1 (PD-(L)1) axis (i.e. “checkpoint blockade”)1–3. PD-(L)1 signaling is central to both initial T cell priming as well as later T cell exhaustion which occurs with aging or chronic antigen stimulation resulting in impairment of proliferative and functional abilities4. Blockade of this pathway markedly augments T cell responses in a variety of viral and cancer models5–8. However, despite the success of PD-(L)1 blockade in multiple malignancies including melanoma, lung, renal, and bladder cancer, these therapies fail to generate sustained benefits in the majority of patients. Extensive efforts are underway to elucidate biomarkers and mechanisms of response9. Many studies have focused on the tumor microenvironment as well as mutational or antigenic load, but patient-associated factors such as sex, age, body mass index (BMI) and immunological history (i.e. pathogen exposure) are also likely to profoundly impact immune responses and yet are poorly understood.
Obesity, defined by increased BMI (≥30kg/m2) reflecting visceral fat accumulation10, is reaching pandemic proportions. Obesity has been associated with numerous co-morbidities such as diabetes, heart disease and cancer10–12, and represents a significant societal burden accounting for > 20% of the total annual U.S. healthcare expenditure13. Although obesity is characterized by a “meta-inflammatory” state with dysregulated immune responses and “inflammaging”12, little is understood about the impact of obesity on immune responses during cancer progression and immunotherapy. This is confounded by the use, in most pre-clinical cancer models, of young lean mice that fail to recapitulate the clinical scenario of the elderly cancer patient. Surprisingly, recent clinical analyses demonstrate that obesity is associated with improved response and survival of cancer patients treated with targeted therapy and checkpoint blockade immunotherapy, although a mechanistic link was not elucidated14,15. In this study, we investigated the impact of obesity on T cell responses and demonstrate a significant impact of obesity on the PD-(L)1 axis, immune aging and dysfunction across multiple species and cancer models. In particular, we demonstrate a marked effect of obesity on tumor progression in mice as well as on clinical outcomes in cancer patients treated with PD-(L)1 checkpoint blockade stratified by body mass. These studies highlight the contrasting/paradoxical effects, both positive and negative, of obesity on cancer immune responses in the context of immunotherapy.
Results
Obesity-related T cell dysfunction across multiple species.
We investigated T cell phenotype and function in control diet mice (control, 10% fat diet) versus diet-induced obese mice (DIO, 60% fat diet) at 6 months (Supplementary Fig. 1a-g) and 11–12 months (Fig. 1a-f and Supplementary Fig. 2–3) of age. DIO mice had a marked increase in subcutaneous and visceral adipose tissue as demonstrated by magnetic resonance imaging (Supplementary Fig. 1a-b). Non-fasting glucose and hemoglobin A1c levels were within normal limits in both DIO and control mice (Supplementary Fig. 1b-c, 2a). At 6 months of age DIO mice had an increased frequency of PD-1+ T cells in the liver (Supplementary Fig. 1f-g). By 11–12 month of age DIO mice exhibited a significant increase in dysfunctional exhausted T cells in peripheral blood, liver, and spleen. Specifically, DIO mice had an increase in the frequency of memory T cells in the peripheral blood (Supplementary Fig. 2b) and liver (Fig. 1b). Further analysis showed a > 2-fold increase in PD-1 expression on DIO versus control T cells (Fig. 1c and Supplementary Fig. 2c). Similar results were seen when examining CD4+ and CD8+ T cell subsets in 6 and 11–12-month old mice (Supplementary Fig. 1f-g, 2d-e). To determine if increased PD-1 expression in DIO mice was indicative of T cell dysfunction, we examined hallmarks of exhaustion, including diminished proliferative function and cytokine production. Ex vivo stimulated polyclonal T cells from DIO mice demonstrated reduced proliferative capacity, as well as reduced IFNγ and TNFα production compared to control (Fig. 1d-f), and, again, similar results were seen when examining CD4+ and CD8+ T cell subsets (Supplementary Fig. 3). We next assessed the impact of obesity in outbred non-human primates (NHP, rhesus macaques) and humans and observed a remarkably similar pattern. T cells from spontaneously obese NHP demonstrated a significant increase in the memory pool and PD-1 expression, while also showing reduced proliferative capacity as compared to non-obese NHP (Fig. 1g-h and Supplementary Fig. 4a-f). Healthy human volunteers were categorized into obese (BMI≥30) and non-obese (BMI<30) cohorts (Supplementary Fig. 4g and Supplementary Table 1). T cells from these obese donors also demonstrated a significant increase in PD-1 expression and decreased proliferative function compared to non-obese donors (Fig. 1i-j). Thus, across multiple species, we observed increased T cell exhaustion and dysfunction in obese subjects providing novel data to support an obesity associated phenotype of immune dysfunction.
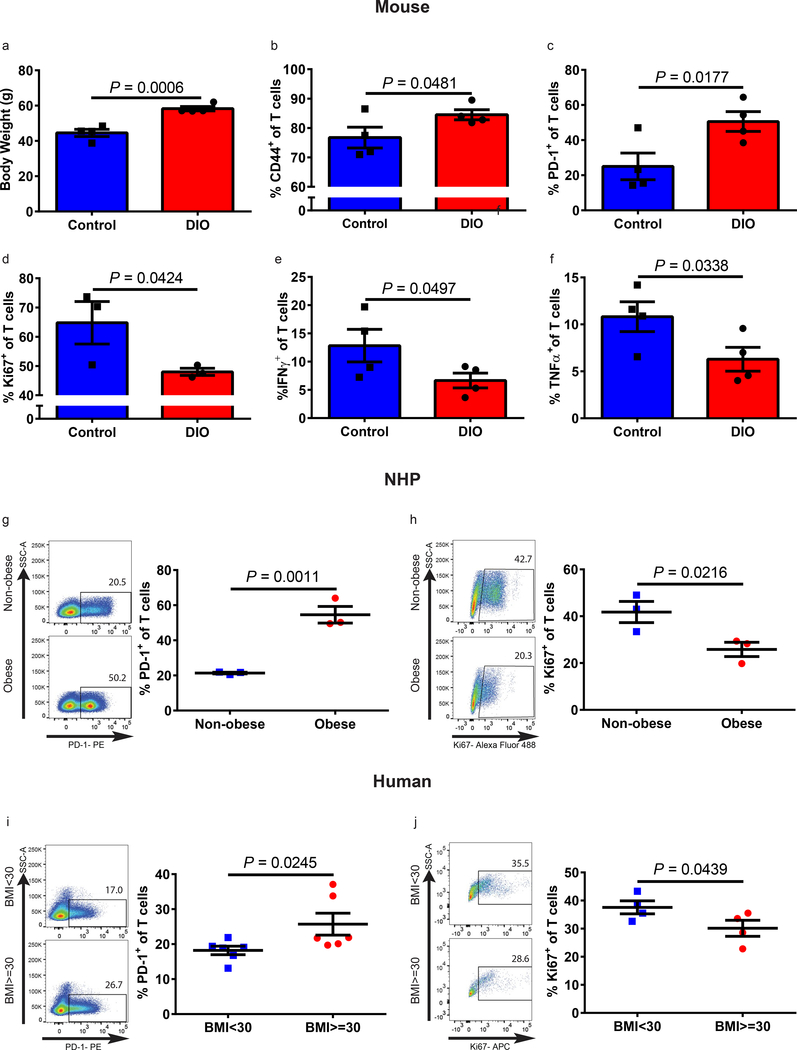
a) Body weights of 11–12-month-old male control and DIO mice (n=4/group). Frequency of b) CD44+ and c) PD-1+ T cells in the liver of 11–12-month-old male control and DIO mice (n=4/group) assessed by flow cytometry. Frequency of d) Ki67 (n=3/group), e) IFNγ (n=4/group), and f) TNFα (n=4/group) expressing T cells in the liver of 11–12-month-old male control and DIO mice after ex vivo stimulation. g) Representative flow plots and frequency of PD-1+ T cells in the peripheral blood of non-obese and obese rhesus macaques (n=3/group). h) Representative flow plots and frequency of Ki67 expressing T cells following ex vivo stimulation of rhesus macaque PBMCs (n=3/group). i) Representative flow plots and frequency of PD-1+ T cells in the peripheral blood of non-obese (BMI<30) and obese (BMI≥30) human healthy volunteers (n=6/group). j) Representative flow plots and frequency of Ki67 expressing T cells following ex vivo stimulation of healthy human PBMCs (n=4/group). Data in this figure are all depicted as mean ±s.e.m., with all individual points shown. One-tailed unpaired Student’s t-test p values shown.
Obesity promotes tumor growth and T cell exhaustion
We next studied the consequences of obesity on tumor immunity. A confounding issue in preclinical cancer modeling is the direct impact that obesity has on tumor growth kinetics16, which may obscure the effects of obesity on the immune response to the tumor. B16 melanoma tumors grew significantly faster in 6-month old DIO mice compared to control counterparts (Fig. 2a and Supplementary Fig. 5a). Tumors in DIO mice exhibited increased metabolic activity as demonstrated by increased 18F-fluorodeoxyglucose (FDG) uptake on positron emission tomography (PET)/computed tomography (CT) scans (Fig. 2b and Supplementary Fig.5b). We observed greater ulceration, necrosis, and invasion into subcutaneous fat by melanoma tumors in DIO mice (Supplementary Fig. 5c). Consistent with our previous findings in non-tumor bearing mice, T cells in the tumor microenvironment (TME) of DIO mice demonstrated features of exhaustion. Specifically, DIO mice had a significantly higher frequency of tumor-infiltrating CD8+ T cells (CD8+ TILs) expressing PD-1, Tim3 and Lag3 and lower proliferation by Ki67 compared to control mice as assessed by flow cytometry (Fig. 2c-f and Supplementary Fig. 5d). Since regulatory T-cells (Tregs) play a role in the TME and have been noted to be altered in obesity17, we also examined Tregs in the TME. We did not observe differences in the frequency of Tregs between DIO and control mice (Supplementary Fig. 5e), nor was there a difference in PD-L1 expression in the TME (Supplementary Fig. 5f). Interestingly, Cpt1a gene expression, a master regulator of fatty acid oxidation (FAO), was upregulated in both tumors and CD8+ T cells of DIO mice (Supplementary Fig.5g). Cpt1a was recently identified as being upregulated in early stage exhausted T cells following LCMV infection and has been suggested as a potential driver of the metabolic changes leading to exhaustion18. We observed similar results with 4T1 breast carcinoma cells in DIO BALB/c mice in terms of tumor growth (Supplementary Fig. 5h-j) and TIL PD-1 expression (Fig. 2g) demonstrating these phenomena across strains and tumor types.
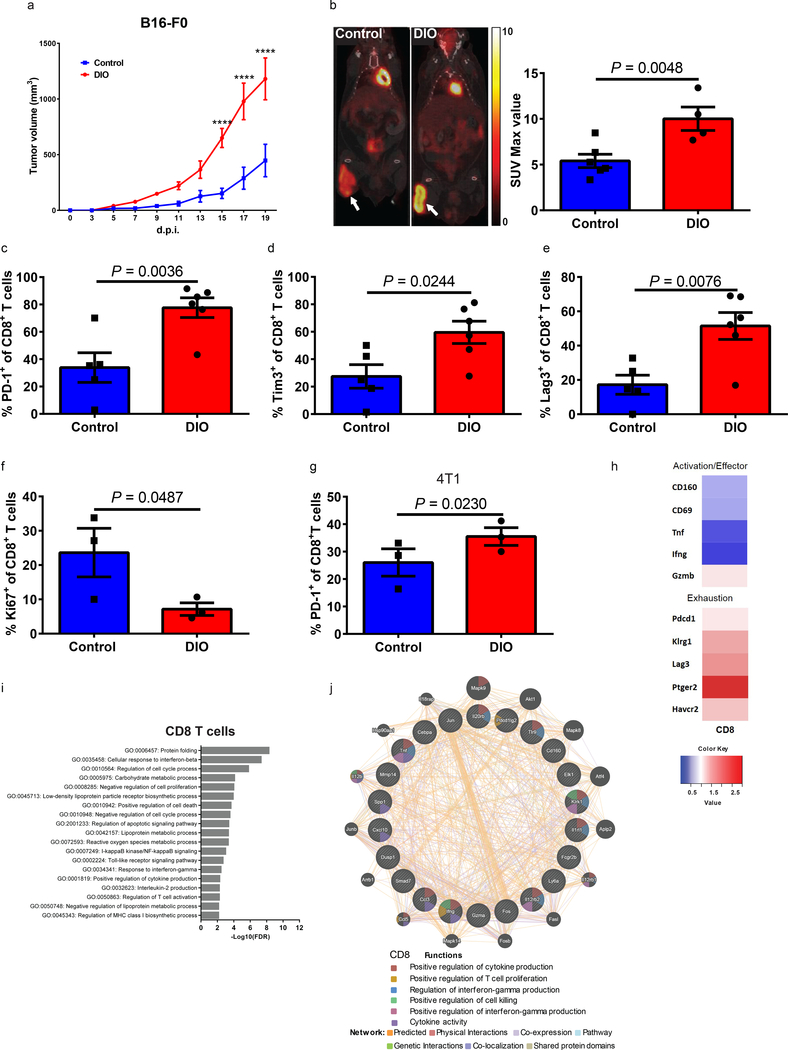
a) Tumor volume of B16-F0 melanoma subcutaneously inoculated in 6-month-old control (n=4) and DIO (n=5) C57BL/6 male mice. Tumor volumes depicted as mean ±s.e.m. Two-way ANOVA with Tukey post-hoc test p-values shown. b) Representative PET-CT images and quantification of tumor burden comparing 6-month-old B16-F0-tumor-bearing control (n=6) and DIO (n=4) mice 18 days post inoculation (d.p.i.). Frequency of c) PD-1, d) Tim3, and e) Lag3 on tumor-infiltrating CD8+ T cells from 6-month-old B16-F0-bearing control (n=5) and DIO (n=6) male mice at 16 d.p.i. f) Frequency of Ki67 on tumor-infiltrating CD8+ T cells from 6-month-old B16-F0-bearing control and DIO male mice (n=3/group). g) Frequency of PD-1 on tumor-infiltrating CD8+ T cells from 4T1-bearing control and DIO female mice (n=3/group) at 23 d.p.i. b-g) Data are depicted as mean ±s.e.m., with all individual points shown. One-tailed unpaired Student’s t-test p-values shown. h) Heat maps generated from RNAseq data showing differentially expressed activation/effector and exhaustion genes (≥1.5 fold difference in CD44+CD8+ T cells isolated from the spleens and lymph nodes of 6-month-old B16-F0-bearing control and DIO C57BL/6 male mice at 16 d.p.i. Data shown as fold change over controls (n=3/group). i) Candidate genes based on FDR cut-off of 1.5-fold change functionally categorized. The most significant pathways of candidate genes were analyzed using G:Profiler by the g:GOST comprehensive method (p<0.05) (n=3/group). j) Network analysis of down-regulated genes (DIO relative to control) in CD8+ T cells from enriched gene sets using GeneMANIA (n=3/group). **** p<0.001
In addition to differences in the TME, the frequency of PD-1+ T cells was significantly increased systemically in the liver, spleen, and draining lymph nodes (dLN) of tumor bearing DIO mice (Supplementary Fig. 6). This is in contrast to non-tumor bearing 6-month-old DIO mice where differences were observed only in the liver (Supplementary Fig. 1) indicating that tumor challenge accelerated the exhaustion phenotype in 6-month-old DIO mice inducing an exhausted systemic phenotype resembling 11–12-month old DIO mice. This coincided with a higher expression of Eomes and a lower expression of T-bet on PD-1+CD8+ T cells in liver, spleen, and dLN of 6-month-old tumor bearing DIO mice compared to age-matched tumor bearing controls (Supplementary Fig. 6c,e,g-h). Elevated expression of transcription factor Eomes and decreased levels of T-bet are well known markers of T cell exhaustion19–21. We observed the frequency of PD-1+ T cells in the visceral fat to be generally higher than in other organs (Supplementary Fig. 6i-j), which is in agreement with recent reports22.
We next defined the molecular differences between sorted (>95% purity) CD8+ memory T cells from the spleens and lymph nodes of B16-bearing control and DIO mice using transcriptomic analysis. Categorical clustering adjusted from previously described canonical T cell exhaustion and effector markers23 corroborated the results of our tumor T cell-phenotyping, demonstrating upregulation of exhaustion-related transcripts and downregulation of effector-related transcripts in T cells from DIO mice (Fig. 2h). Differential profile analysis also demonstrated marked global transcriptional differences of CD8+ T cells from control versus DIO mice (Supplementary Fig. 7a). This functional categorical clustering of markers was generated based on previously published data 24. T cells from tumor-bearing DIO mice upregulated anergy-, senescence-, and TGF-β-associated genes as well as genes previously associated with obesity, diabetes and insulin-resistance22 (Supplementary Fig.7a). Using ontology and network analysis, we further observed global, log fold, differences in T cell gene expression from DIO versus control mice in genes involved in metabolism, T cell activation, Toll-like receptor signaling, regulation of chemotaxis and protein folding similarly (Fig. 2i, Benjamini-Hochberg FDR <0.05). Similar results were obtained using represented ontologies of either down-regulated (Fig. 2j) or up-regulated genes (Supplementary Fig.7b) through network analysis. These results suggest that T cells from tumor-bearing DIO mice exhibit a transcriptomic profile which is markedly different than T cells from tumor-bearing control mice, findings consistent with increased exhaustion and inflammation.
Leptin levels are correlated with PD-1 expression
Obesity has been demonstrated to induce a meta-inflammatory state with increased pro-inflammatory cytokines such as TNFα and IL-6, as well as elevated levels of glucose, insulin, fatty acids and leptin. Leptin, which is characteristically present at high levels in obesity, has not been previously linked to PD-1 expression, but studies suggest that it can affect T cell function25–27. The RNAseq analysis on T cells from B16-bearing control and DIO mice revealed differential expression of pathways involved in fat metabolism and T cell signaling and showed marked changes in the expression of genes previously described to be involved with leptin signaling28 including several IFN-inducible GTPases 29 (Supplementary Fig. 8a). In healthy human volunteers (Supplementary Table 1), we observed significantly increased serum leptin levels in obese donors (Fig. 3a), which correlated with PD-1 expression on CD8+ T cells (Fig. 3b and Supplementary Fig. 8b). Similarly, leptin levels were elevated in DIO mice, and this corresponded with an elevated frequency of PD-1 on CD8+ T cells (Fig. 3c-d and Supplementary Fig. 1e-f). Using ad libitum (AL)-fed mice, which gain weight and visceral adipose tissue as they age, and age-matched calorie restricted (CR) mice, we next determined whether limiting body weight and adipose tissue could minimize leptin levels and T cell exhaustion. CR mice had decreased visceral adiposity than AL mice as measured by magnetic resonance imaging (Fig. 3e), and, also, weighed significantly less and had lower leptin levels (Supplementary Fig. 8c-d). The reduced obesity and leptin levels in CR mice were accompanied by a significantly lower frequency of PD-1+CD8+ T cells in the liver and spleen as well as a lower frequency of Eomes+PD-1+CD8+ T cells (Fig. 3f and Supplementary Fig. 8c-i) demonstrating that reduction of body fat resulted in lower leptin and T cell dysfunction with age. We next examined T cell phenotype in leptin-deficient ob/ob30 and leptin receptor (ObR)-deficient db/db mice31, both of which are obese due to lack of the satiety signal. As expected, db/db mice weighed significantly more than age-matched wild type (WT) control mice (Supplementary Fig.9a), but the frequency of CD8+ T cells expressing PD-1 in db/db mice was significantly lower than that of WT control mice (Fig. 3g). The ob/ob mice were weight-matched to wild type DIO mice and significantly heavier than WT control mice (Supplementary Fig. 9b). The frequency of CD8+ T cells expressing PD-1 from ob/ob mice trended lower than that of DIO mice with a similar weight but trended higher than that of control mice (Supplementary Fig. 9c-d). These results suggest that leptin is not required for PD-1 expression on CD8+ T cells but that in the setting of obesity intact leptin signaling contributes to the PD-1+ phenotype. We next examined the fate of T cells from WT (ObR+) and db/db (ObR-) mice on regular diet subject to allogeneic activation by adoptive transfer into immunodeficient but H2-disparate (H2d) NSG mice (Supplementary Fig. 9e). Comparing the number of CD8+ T cells originally transferred into NSG mice to the number of donor cells found in the spleen (Supplementary Fig. 9f) and liver (Supplementary Fig. 9g) at 13 days post transfer, we observed that WT cells minimally expanded in marked contrast to db/db T cells which expanded robustly. This increased expansion of db/db CD8+ T cells was associated with lower PD-1 expression (Fig. 3h-i). Comparing PD-1 frequency of transferred cells pre- and post-transfer we find that both WT and db/db CD8+ T cells increased PD-1 expression upon transfer and chronic allogenic stimulation, but the extent of that increase was drastically more pronounced in WT CD8+ T cells which had a 40-fold increase (Supplementary Fig. 9h). These results suggest that T cells with intact leptin signaling are more susceptible to PD-1 upregulation and proliferative dysfunction with chronic stimulation in vivo whereas leptin unresponsive T cells remain functional with limited PD-1 upregulation.
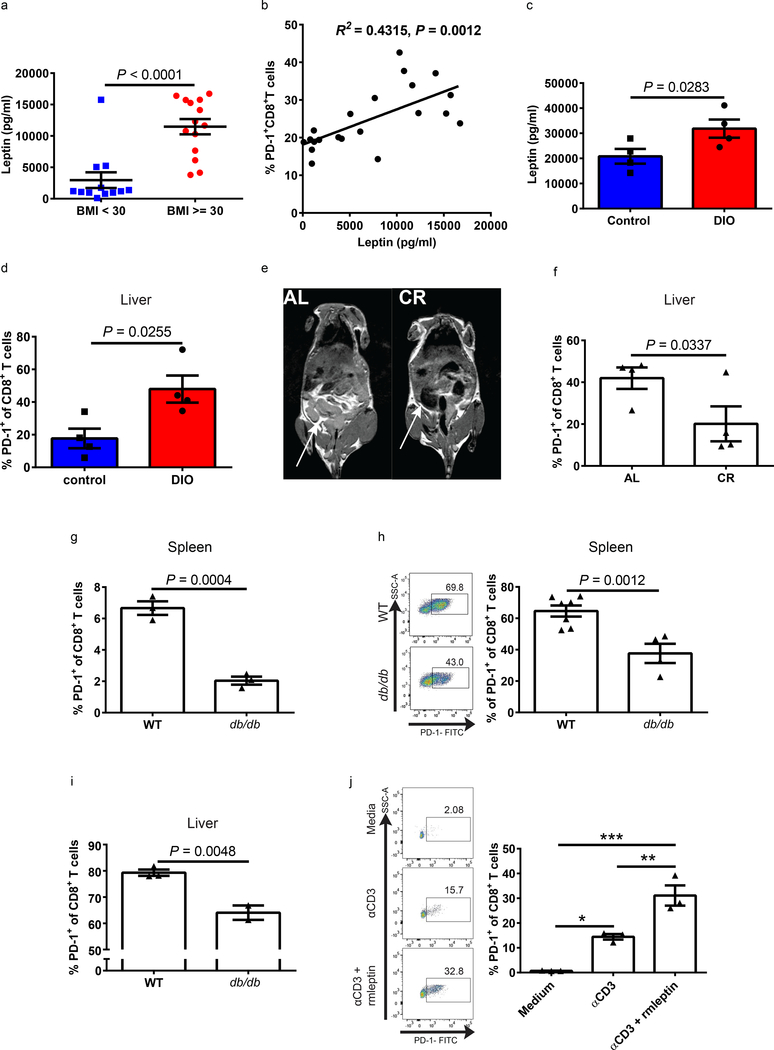
a) Leptin levels in non-obese (BMI<30, n=14) and obese (BMI≥30, n=12) healthy human volunteers. b) Linear regression analysis of leptin levels and PD-1 expression on CD8+ T cells in peripheral blood of human volunteers (n=21). c) Leptin levels and d) PD-1 expression on liver CD8+ T cells in 11–12-month-old control and DIO male mice (n=4/group). e) T1-weighted MRI demonstrating subcutaneous and visceral fat (fat appears bright white, white arrows) in 19-month-old ad-libitum (AL) fed and age-matched calorie restricted (CR) male mice. f) Frequency of PD-1 expressing CD8+ T cells in the liver of 19-month-old AL and CR male mice (n=4/group). g) Frequency of PD-1+CD8+ T cells from spleens of WT and db/db (9-month-old) male mice (n=3/group). Representative flow plots and frequency of PD-1 on h) splenic (n=7 in WT group, n=4 in db/db group) and i) liver (n=3 in WT group, n=2 in db/db group) CD8+ T cells of NSG mice 13 days post-transfer. a, c-d, f-i) Data are depicted as mean ±s.e.m., with all individual points shown. One-tailed unpaired Student’s t-test p-values shown. j) Representative flow plots and frequency of PD-1 expression on splenic CD8+ T cells ex vivo stimulated with αCD3, αCD3 and recombinant mouse leptin (αCD3+rmleptin), or unstimulated for 24 hrs (n=3 technical replicates). Data are depicted as mean ±s.e.m., with all individual points shown. One-way ANOVA with Tukey post-hoc test used to compare groups. *p<0.05, **p<0.01.
To further link leptin to PD-1 expression and T cell exhaustion we evaluated in vitro the effects of leptin on T cell activation directly. Mirroring the in vivo results above with allogeneic stimulation, PD-1expression on CD8+ T cells was increased upon stimulation with anti-CD3 (αCD3), and this upregulation was dramatically enhanced with addition of recombinant mouse leptin (rmleptin, Fig. 3j). This increased PD-1 expression correlated with upregulation of phospho-STAT3 (pSTAT3, Supplementary Fig. 10a-b), a major downstream mediator of leptin signaling, which is also known to induce PD-1 expression on T cells through distal regulatory elements that interact with the PD-1 gene promoter32. Importantly, the addition of a STAT3 inhibitor significantly decreased both the upregulation of pSTAT3 and PD-1 induced by stimulation and leptin (Supplementary Fig. 10a-b). Leptin can increase T cell activation33,34 and obesity can cause leptin resistance35,36. An alternate explanation for the T cell phenotype of DIO mice could be that leptin increases T cell activation and in obesity T cells develop leptin resistance. Therefore, we examined the induction of pSTAT3 by leptin stimulation in T cells from control and DIO mice. There were no differences in the expression of pSTAT3 on CD8+ T cells from control and DIO mice (Supplementary Fig. 10c), indicating that CD8+ T cells in DIO mice are not resistant to leptin, in agreement with published reports on DIO mice. Our studies with sorted human T cells in vitro revealed that mitogen-driven proliferation of memory T cells, but not naïve T cells, was inhibited by leptin, also in agreement with previous findings37(Supplementary Fig. 10d). Collectively, our findings implicate leptin as a link between obesity and increased PD-1 expression and exhaustion on memory T cells.
We then examined the effect of leptin on T cell exhaustion in tumor-bearing models using leptin-deficient mice. We treated B16-tumor bearing ob/ob mice with rmleptin and examined tumor growth and immune activity (Supplementary Fig. 11a). Although ob/ob mice treated with exogenous rmleptin continuously lost weight, tumors in these mice grew faster (Supplementary Fig. 11b-c). Importantly, leptin treatment resulted in increased CD8+ T cell exhaustion in the tumor environment with significantly decreased IFNγ on PD-1+CD8+ T cells (Fig. 4a), increased numbers of PD-1+CD8+ T cells, decreased expression of Ki67, and significantly increased expression of Eomes at the mRNA level (Supplementary Fig. 11d-f). Exogenous rmleptin also significantly affected CD8+ T cells outside of the TME as demonstrated in the liver. Leptin upregulated PD-1 expression at the mRNA and protein level (Supplementary Fig. 11g-h), as well as mRNA expression of HAVCR2 (Tim3) and Cpt1a (Supplementary Fig. 11i-j), resulting in diminished proliferation of PD-1+CD8+ T cells (Fig. 4b). To examine the consequences of obesity and leptin signaling on the anti-tumor immune response, we adoptively transferred T cells from WT (ObR+) or db/db mice into control or DIO immune deficient C57BL/6 Rag2−/− mice (which lack T cells) bearing B16-F0 tumors (Fig. 4c-d and Supplementary Fig. 12a). Consistent with our previous data, tumor growth was accelerated in DIO Rag2 −/− mice compared to control Rag2 −/− (Supplementary Fig. 12b-c) after adoptive transfer of WT T cells. Importantly, tumor growth kinetics were similar whether db/db T cells were transferred into control or DIO Rag2 −/− C57BL/6 mice, whereas tumors grew faster in DIO Rag2 −/− mice that received WT T cells compared to control Rag2 −/− mice that received WT T cells (Fig. 4e and Supplementary Fig. 12d). This finding suggests that the obese environment, with elevated leptin, impairs the anti-tumor responses of transferred WT CD8+ T cells, but not leptin-resistant db/db CD8+ T cells. This is confirmed by TME immunophenotyping, which demonstrates significantly higher expression of PD-1 on WT adoptively transferred CD8+ T cells in DIO Rag2−/− mice versus db/db T cells in the DIO Rag2−/− mice or versus WT or db/db T cells in control Rag2−/− hosts (Fig. 4f and Supplementary Fig. 12e). Importantly, ex vivo analysis of TNFα and IFNγ production also demonstrated improved functionality of db/db (compared to WT) TILs isolated from the TME of DIO Rag2 −/− mice whereas WT and db/db TILs from tumors in control Rag2 −/− had equivalent functionality (Fig. 4g-i and Supplementary Fig. 12f-h). These results indicate a role of leptin signaling in increasing PD-1 expression and promoting T cell exhaustion in tumor-bearing mice, which affects anti-tumor immunity.
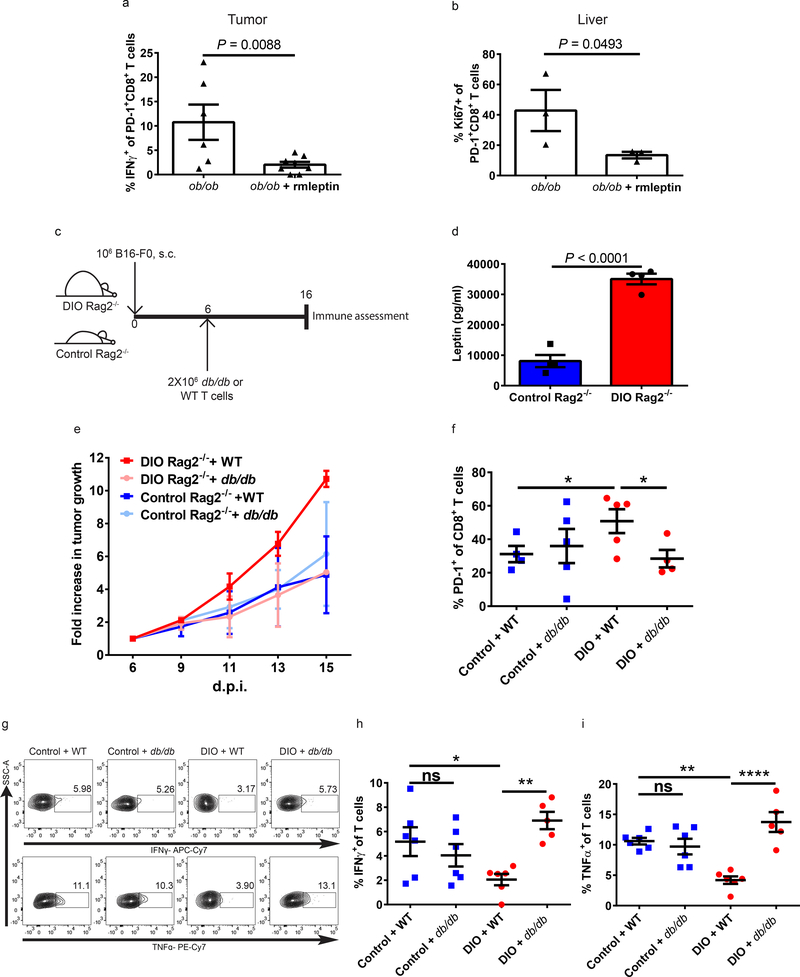
a) Frequency of IFNγ+ tumor-infiltrating CD8+PD-1+ T cells after ex vivo stimulation (n=3/group, 2 technical replicates in ob/ob group, 2–3 technical replicates in ob/ob+rmleptin group) and b) frequency of Ki67 on CD8+PD-1+ T cells in the liver of 3-month-old ob/ob male B16-F0-bearing mice treated with or without rmleptin (n=3/group). c) Schema of C57BL/6 Rag2−/− mice challenged with B16 melanoma cells followed by adoptive transfer of T cells from either WT or db/db C57BL/6 mice. d) Serum leptin levels of 5-month-old control and DIO C57BL/6 Rag2−/− male mice (n=4/group). a-b, d) Data are depicted as mean ± s.e.m., with all individual points shown. One-tailed unpaired Student’s t-test used to compare groups. e) Fold change of B16-F0 tumor growth in control and DIO Rag2−/− mice after adoptive transfer of T cells from either WT or db/db C57BL/6 mice (fold change compared to tumor size at time of adoptive transfer) (n=2/group). f) Frequency of PD-1 expression on tumor-infiltrating CD8+ T cells from B16-F0-bearing control (n=4 in WT group, n=5 in db/db group) and DIO (n=5 in WT group, n=4 in db/db group) Rag2−/− mice with adoptive transfer of T cells from either WT or db/db C57BL/6 mice. g) Representative flow staining and frequency of h) IFNγ and i) TNFα producing tumor-infiltrating T cells after ex vivo stimulation collected from B16-F0-bearing control and DIO Rag2−/− mice with adoptive transfer of T cells from either WT or db/db mice (n=2/group, 2–3 technical replicates). f, h-i) Data are depicted as mean ±s.e.m., with all individual points shown. One-way ANOVA with Tukey post-hoc test used to compare groups. *p<0.05, **p<0.01, **** p<0.001.
Improved efficacy of PD-1 checkpoint blockade in DIO mice
Building on our observations that CD8+ T cells have increased exhaustion and dysfunction in obese, cancer-bearing subjects, we assessed the impact of PD-1 blockade on tumor growth. Administration of anti-PD-1 (αPD-1) as a monotherapy (Supplementary Fig. 13a) had minimal to no effect on control mice but surprisingly led to significant and marked reduction in B16 tumor burden and a significant improvement in survival in DIO mice (Fig. 5a-b and Supplementary Fig. 13b-c). Similar results were also observed in 3LL-tumor-bearing DIO mice (Fig. 5c and Supplementary Fig. 13d-e). Of note, in agreement with our other models demonstrating accelerated tumor growth in obese models, 3LL tumors also grew faster in DIO mice. These results demonstrate that in models where αPD-1 monotherapy has limited effect (B16)38, or where there is some susceptibility to monotherapy (3LL)39, DIO mice displayed significantly greater therapeutic efficacy than control recipients. The improved responsiveness in DIO mice was associated with a significant increase in the total number of tumor-infiltrating T cells and increased frequency of CD8+ T cells in the TME (Fig. 5d-e and Supplementary Fig. 13f-g), and an increased CD8 to CD4 T cell ratio (Supplementary Fig. 13h) in response to therapy. In DIO mice, αPD-1 therapy also reduced the frequency of PD-1+ T cells in the TME (Supplementary Fig. 13i-j). Similarly, ex vivo functional assessment of splenic CD8+ T cells from aPD-1-treated tumor-bearing DIO mice demonstrated significantly increased TNFα- and IFNγ production on CD8+ T cells, compared with controls (Supplementary Fig. 14a-b). Overall, αPD-1 therapy rescued CD8+ T cells from exhaustion and significantly improved survival in DIO mice. Similar anti-tumor effects were also observed in a melanoma metastasis model (B16-F10, Supplementary Fig. 14c). Importantly, αPD-1 treatment significantly inhibited metastases in B16-bearing DIO mice, but not in control mice (Fig. 5f). Further examination of the effects of αPD-1 in DIO mice revealed significant inhibition of both visceral and lung metastases (Fig. 5g-j). Importantly, despite the risks of severe/lethal toxicities after systemic immune-stimulatory therapies in obese mice40, adverse events were not observed after αPD-1 checkpoint blockade (Supplementary Fig. 14d-g), implying an uncoupling of heightened treatment response and risk of toxicity in the setting of obesity that may be contingent on the type of immunotherapy applied.
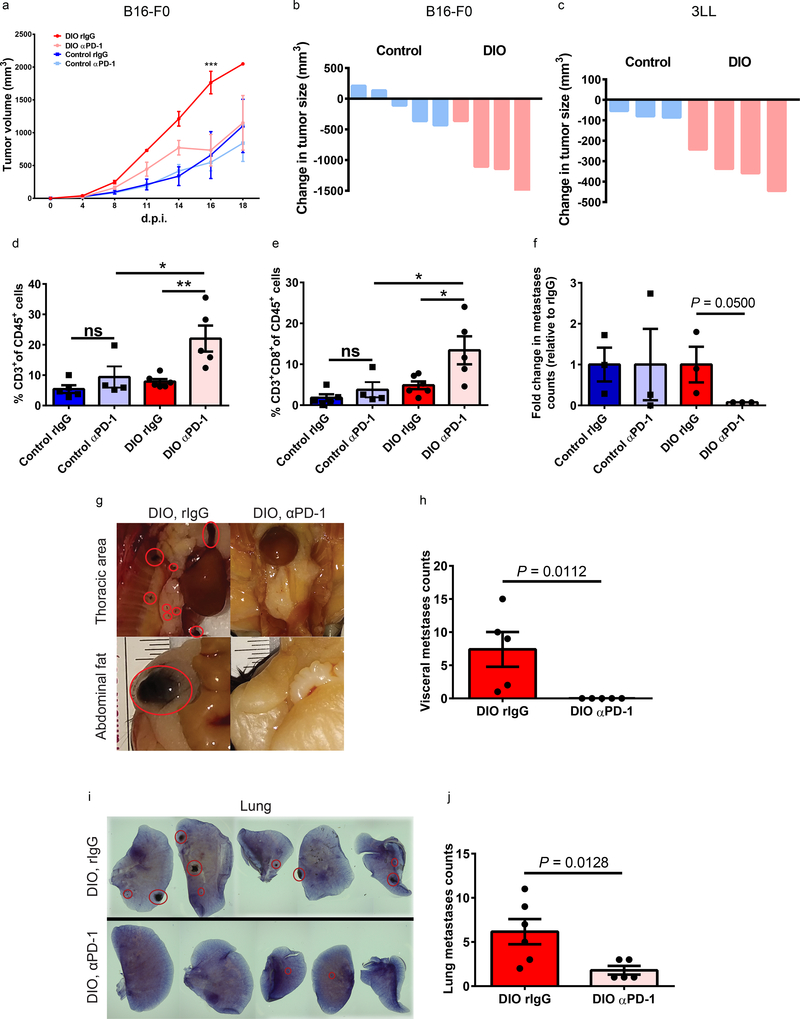
a) Volume of B16-F0 melanoma in 6-month-old control and DIO C57BL/6 male mice with and without αPD-1 treatment (n=4 in control and DIO rIgG groups, n=5 in control and DIO αPD-1 groups). Tumor volumes are depicted as mean ±s.e.m. Two-way ANOVA with Tukey post-hoc test used to compare groups. Waterfall plots of the volumes of b) B16-F0 (n=5 in control group, n=4 in DIO group) at 16 d.p.i. and c) 3LL lung carcinoma (n=3 in control group, n=4 in DIO group) at day 11 d.p.i. in 6-month-old control and DIO C57BL/6 male mice with and without αPD-1 treatment (graphed as change in tumor volume compared to non-treatment controls). Frequency of tumor-infiltrating d) CD3+ cells and e) CD8+ T cells as a percentage of CD45+ cells in the tumor (n=6 in DIO rIgG group, n=5 in control rIgG and DIO αPD-1 groups, n=4 in control αPD-1 group). Data are depicted as mean ±s.e.m., with all individual points shown. One-way ANOVA with Tukey post-hoc test used to compare groups. f) Fold change of total metastases in intravenously injected B16-F10-bearing control and DIO mice with and without αPD-1 treatment (graphed as change in tumor metastases counts compared to non-treatment controls) 28 d.p.i. (n=3/group). Data are depicted as mean ±s.e.m., with all individual points shown. Mann-Whitney test used to compare DIO rIgG and αPD-1 groups. g) Representative pictures and h) quantification of metastases in visceral fat of non-treated and treated B16-F10-bearing DIO mice (n=5/group). Data are depicted as mean ±s.e.m., with all individual points shown. One-tailed unpaired Student’s t-test p-value shown. i) Representative staining and j) quantification of lung metastases in non-treated and treated B16-F10-bearing DIO mice (n=6 in rIgG group, n=5 in αPD-1 group). Data are depicted as mean ±s.e.m., with all individual points shown. One-tailed unpaired Student’s t-test p-value shown. *p<0.05, **p<0.01, ***p<0.005.
Impact of obesity on PD-1 expression and effects of checkpoint blockade in obese cancer patients
Since significant anti-tumor effects were observed with αPD-1 therapy in obese mouse models, we examined T cell phenotypes in the TME of obese human cancer patients. In a large cohort of 152 human colorectal cancers (CRCs), we observed significantly fewer tumor-infiltrating-T cells in obese patients (Fig. 6a-b). Since CRC patients have a poor response rate to checkpoint blockade, we also examined a more responsive tumor type – melanoma. In a cohort of 251 melanoma patients with annotated clinical data from the cancer genome atlas (TCGA) a 1.57-fold (p=0.019) increase in mean PD-1 expression was noted in tumors of obese (BMI≥30) patients (data not shown). Among patients older than 60 (n=126), the effect of obesity on exhaustion marker expression in the TME was even more pronounced with a 3-fold increase in PDCD1 (PD-1) in obese patients (Fig. 6c, p=3.2×10−5), 2-fold increase in HAVCR2 (Tim3) (Fig 6d, p=2.0×10−4), 3-fold increase in LAG3 (Fig 6e, p=2.0×10−5), 2.3-fold increase in TIGIT (Fig 6f, p=1.3×10−3), 2.2-fold increase in TBX21 (T-bet) (Fig 6g, p=9.0×10−4), and 1.79 fold increase in EOMES (Fig 6h, p=6.9×10−3). These results generally mirrored the TME phenotype in DIO mouse tumors, with no change in FOXP3, leptin receptor, CTLA-4 and CD274 (PD-L1) expression (Supplementary Fig. 15a-d). Most importantly, we observed a striking improvement in the clinical outcomes of obese (BMI≥30) versus non-obese (BMI<30) patients, in a cohort of 250 human patients treated with αPD-(L)1 checkpoint blockade for a variety of cancers (Supplementary Table 2). There was a statistically significant improvement in progression-free survival (median: 237 versus 141 days, p=0.0034) and overall survival (median: 523 versus 361 days, p=0.0492) in obese patients (Figure 6i-j). As in obese mice, no increases in immune-related adverse effects were observed in obese patients (Supplementary Table 2). Using a Cox proportional hazards model to adjust for ECOG performance status, line of treatment, age, sex, and cancer type, BMI category was still significantly associated with better progression-free survival (HR=0.61, 95%CI=0.42–0.89, p=0.01) and overall survival after αPD-(L)1 therapy (HR=0.594,95%CI=0.35–0.99, p=0.048).
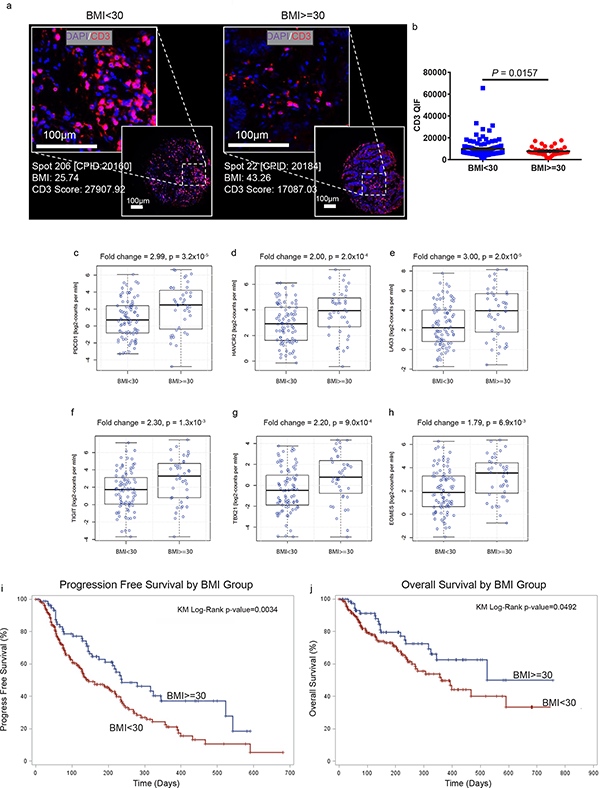
a) Representative immunofuorescent staining and b) bar graph of CD3+ infiltrates in the TME of human CRCs (n=113 in BMI<30 group, n=39 in BMI>=30 group). Data are depicted as mean ±s.e.m., with all individual points shown. One-tailed unpaired Student’s t-test p-value shown. Human TCGA data analysis of c) PDCD1 (PD-1), d) HAVCR2 (Tim3), e) LAG3, f) TIGIT, g) TBX21 (T-bet), and h) EOMES expression in melanoma tumors in non-obese (n=86) versus obese (n=40) patients. The line within each notch box represents the median. The lower and upper boundaries of the box indicate first and third quartiles respectively. The whiskers indicate the minimum and maximum values. P‐value was calculated via DESeq2 (Wald‐Test) to compare groups. i) PFS and j) OS of 250 human cancer patients treated with PD-(L)1 checkpoint blockade and stratified by BMI (n= 169 in BMI<30, n=81 in BMI>=30). i-j) Kaplan-Meier estimates of PFS and OS.
Discussion
In this report, we demonstrate for the first time, across multiple species and tumor models, that obesity increases T cell aging resulting in higher PD-1 expression and dysfunction, which is driven, at least in part, by leptin signaling. We also observed increased tumor progression in the setting of obesity, and this was likely due to this immunosuppression as well as direct (metabolic and hormonal41) effects. However, the PD-1-mediated T cell dysfunction in obesity remarkably leaves their tumors markedly more responsive to checkpoint blockade. Importantly, these preclinical findings are corroborated by clinical data demonstrating significantly improved outcomes in obese cancer patients treated with PD-1/PD-L1 inhibitors.
Our findings validate and provide mechanism for a recent report in obese melanoma patients showing improved outcomes with immunotherapy14. In that study the effect was limited to male melanoma patients, whereas our clinical data extends across cancer types and demonstrates a positive effect even when controlling for sex. Although our study focuses on PD-1 mediated T cell dysfunction induced by obesity, sex, as well as other factors such as age, genetics, metabolic dysregulation, gut microbiome, dietary differences, and the duration of obesity likely confound the effects of obesity on the immune system and warrant further study. Age, in particular, is known to increase the memory pool of T cells and may be a key confounder particularly in specific-pathogen-free (SPF) laboratory mice which experience limited exposure to immune challenges. In our studies 6-month-old DIO mice did not display the heightened phenotypic exhaustion seen in 11-month-old DIO mice, unless challenged with a tumor. This is consistent with the concept that obesity results in an immune aging effect, termed “inflammaging” 12, which is accentuated by immune challenge. Thus age, sex, exposure to immune challenge, and obesity may all play critical roles in shaping immune responses. Furthermore, obesity likely operates via multiple pathways aside from the PD-(L)1 axis to induce T cell dysfunction and promote tumor growth, and these phenomena are likely also influenced by diet. We observed elevated Cpt1a expression - a master regulator of fatty acid oxidation (FAO) and driver of metabolic changes leading to exhaustion18. Other factors associated with obesity, such as free fatty acids, insulin/IGF1, and proinflammatory cytokines such as TNF and IL6, all likely contribute to this phenotype outside of body fat. As a result, it will be important to ascertain the effects of different diets as well as duration of exposure needed to observe possible immune-altering effects.
In our study, tumor bearing DIO mice showed higher expression of Eomes than T-bet, which supports an exhausted CD8+ T cell profile4,42. Although some studies suggest that T-bethi but not Eomeshi exhausted T cells respond better to PD-1 blockade4, it has been demonstrated that blocking PD-(L)1 axis can reinvigorate PD-1hiT-betloEomes+ CD8+ T cells43.
We focused on leptin as a mechanism of obesity induced T cell dysfunction given that STAT3, a major downstream transcription factor of the leptin receptor44,45, has known binding sites in the promoter region of PD-146,47 and has been implicated in driving expression of PD-1 in human and murine cancers48. However, it is important to recognize that these molecules have broad pleiotropic effects, and other pathways are likely implicated. This was demonstrated by the effects of administering leptin to ob/ob mice where significant metabolic effects also occurred.
While leptin has been well-documented to impact T cells, particularly Tregs, the literature also suggests that leptin increases T cell activation26,34, which may be explained by the opposing effects of leptin on naïve versus memory T cell populations as previously reported 37. PD-1 plays critical roles both in early T cell activation as well as in later chronic stimulation where the exhaustion phenotype predominates. Thus, differences between our findings and others are likely explained by the T cell populations examined as well as the time-points assessed. The impact of leptin and obesity on other immune cells is also likely very important given the recent report linking leptin in tumor-bearing DIO mice with PD-L1 expression in myeloid-derived suppressor cells (MDSC)49. Our results also suggest that targeting the leptin receptor on activated T cells or CAR T cells in immunotherapy may be of use to augment T cell function particularly in high leptin circumstances.
Overall, our data suggest that obesity associated “inflammaging” results in increased T cell aging and induction of normal suppressive pathways to counter this chronic inflammatory state. In obesity, PD-1 mediated immune suppression may be a mechanism to protect against possible autoreactive or hyperactive T cell responses induced by chronic inflammation. Importantly, the linkage of obesity and leptin to PD-1 and T cell dysfunction in cancer progression appears remarkably robust in both mouse and human studies. It remains to be determined if obesity increases PD-1+ CD8 T cells in a broad range of human cancers and whether this mechanism also contributes to the increased incidence of cancer in obese patients. Furthermore, it remains to be delineated clinically whether the environment in the obese state results in greater T cell activation and function once checkpoint blockade is applied. It is unclear if obesity may impact the survival of cancer patients by other non-immune factors as well. In that regard, obesity should not necessarily be regarded as a positive prognostic factor in cancer but rather as a potential mediator of immune dysfunction and tumor progression that can be successfully reversed by PD-(L)1 checkpoint inhibition resulting in heightened efficacy.
Methods
Please also refer to the Life Sciences Reporting Summary for additional experimental details.
Mice
Mice were housed in AAALAC-accredited animal facilities at UC Davis under SPF conditions. Protocols were approved by UC Davis IACUC and studies complied with ethical regulations and humane endpoints. Male and female C57BL/6NTac, female BALB/cAnNTac and male B6.129S6-Rag2tm1Fwa N12 (Rag2−/−) mice were purchased from Taconic Farms at 4 weeks of age. Diet induced obese and control mice were generated by feeding mice with an open-source purified diet consisting of either 60% fat or 10% fat (D12492 & D12450J, Research Diets, Inc) respectively, starting when the mice were 6 weeks old. 4–8-week-old male B6.Cg-Lepob/J (ob/ob) mice and female B6.BKS(D)-Leprdb/J (B6 db/db) and WT C57BL/6 mice were purchased from Jackson Laboratory. ob/ob mice were used when they were 3–5 months old and equivalent in weight to 9-month-old DIO mice. 6–9-month-old BKS.Cg-Dock7m +/+ Leprdb/J (BSK db/db) were kind gifts from Dr. R. Rivkah Isseroff. The female NSG mice (4–5-month-old) were kind gifts from Dr. Jan Nolta. 19-month-old ad libitum (AL) and calorie restricted (CR) C57BL/6 mice were obtained from the National Institute on Aging and were fed NIH31 regular chow with a 40% calorie restriction regimen previously published40.
Leptin and glucose measurements
Leptin concentrations of human plasma were measured using a sandwich ELISA kit from MilliporeSigma. Mouse serum leptin was measured using an electrochemiluminescent assay from Meso Scale Discovery. Mouse glucose was measured using an enzymatic assay from Fisher Diagnostics.
Tumor cell line and treatment
The murine melanoma cell lines B16-F0 (CRL6322™) and B16-F10 (CRL-6475™), Lewis lung carcinoma (3LL, CRL-1642™), and breast cancer 4T1 (CRL-2539™) were obtained from the American Type Culture Collection. C57BL/6 mice were injected subcutaneously in the right flank with 1×106 B16-F0 or 3LL tumor cells in 100 μL PBS. BALB/c mice were injected subcutaneously in the right mammary pad with 2×105 4T1 tumor cells in 100 μL PBS. Tumor growth was monitored daily and measured every 2–3 days. Tumor volume was determined as length (mm) × width2 (mm) × 0.5. C57BL/6 tumor-bearing mice were treated intraperitoneally with 500 μg αPD-1 mAb (B16: clone 29F.1A12; 3LL: clone J43, BioXCell) in 200 μL PBS on day 6, and 250 μg αPD-1 mAb on days 8, 10, 12, 14 and 16 post tumor inoculation. Control mice received rat IgG (Jackson ImmunoResearch Laboratories, Inc.). For the B16-F10 studies, 1×105 tumor cells were intravenously injected into C57BL/6 mice. Mice were treated intraperitoneally with 500 μg αPD-1 mAb (B16: clone 29F.1A12) in 200 μL PBS on day −1, and 250 μg αPD-1 mAb on days 1, 3, 5, 7 and 9 post tumor inoculation (d.p.i.). ob/ob mice were subcutaneously injected with 1×106 B16-F0 in 100 μL PBS in the right flank. 1 μg/g recombinant mouse leptin (rmLeptin, R&D Systems, Minneapolis, MN) was intraperitoneally twice a day from d.p.i. −3 to 14.
Adoptive transfer of cells into mice
2×107 cells from either C57BL/6 WT or BSK db/db mice isolated from spleens and lymph nodes were adoptively transferred into NSG mice. Immune parameters of B6 WT or BSK db/db T cells in the recipient of NSG mice were analyzed on day 13 post-transfer. 1×106 B16-F0 cells were subcutaneously inoculated in the right flank of 5–month-old male DIO and control Rag2−/− mice. MagniSort™ Mouse T-cell Enrichment Kit (ThermoFisher) was used to enrich T cells from spleens and lymph nodes of B6 WT or BSK db/db mice. 2×106 enriched T cells from either B6 WT or db/db mice were adoptively transferred into B16-F0-bearing control and DIO Rag2−/− mice at d.p.i. 6. Immune parameters of B6 WT or db/db T cells in the recipient of B16-F0-bearing Control and DIO Rag2−/− mice were analyzed on day 16 post-transfer.
Anti-CD40/IL-2 Immunotherapy
The agonistic anti–mouse CD40 antibody (αCD40, clone FGK115B3) was generated via ascites production in our laboratory and 6–8-month-old male DIO and Control B16-F0-bearing mice were treated with αCD40 and recombinant human IL-2 (rhIL-2, TECIN Teceleukin) as previously described 40,50. In brief, αCD40 (40 μg/dose in 200 μL PBS) was administered daily for a total of 5 consecutive days (d.p.i. 6–10) and rhIL-2 (105 IU/dose) was administered twice on d.p.i. 7 and 9. B16F0-bearing mice treated with either αCD40/rhIL-2 or anti-PD-1 were bled on day 8. Control mice received rat IgG (Jackson ImmunoResearch Laboratories, Inc.) and PBS.
Mouse Flow cytometry
Single cell suspensions were prepared from livers, tumors, spleens, lung and draining lymph nodes. Cells were incubated with Fc block (anti-CD16/32 clone 93, BioLegend) and stained with the fluorochrome-conjugated mAbs listed below. FMOs were used as negative staining controls to set gates. For Intracellular staining of TNFα and IFNγ, the Cytofix/CytopermTM kit (BD Biosciences) was used. 1×106 single cells from spleen, liver, and tumors were stimulated with 1 μg/ml anti-mouse CD3 antibody coated wells (clone 145–2C11) in RF10 Complete Medium for 6 hrs total at 37°C with 4 μL/6mL GolgiStop (BD Biosciences) and 1 μL/mL GolgiPlug (BD Biosciences) for the last 4 hrs prior to surface staining. For intracellular staining of Ki67, FoxP3, T-bet and Eomes, the FoxP3/Transcription Factor Staining kit (ThermoFisher) was used. 2×105 cells from liver and spleen were stimulated with 1 μg/ml Concanavalin A (ConA, MilliporeSigma) for 48 hrs at 37°C prior to surface staining. For pSTAT3 staining, 2–5×105 single cells from spleen were stimulated by 100 ng/ml anti-mouse CD3 antibody coated wells (clone 145–2C11) with or without 12 μM STAT3 inhibitor cryptotanshinone (EMD Millipore, CAS# 35825–57-1) for 1 hour at 37°C prior to addition of 100 ng/ml or 1000 ng/ml recombinant mouse leptin (rmleptin, Gibco LifeTechnologies). Intracellular pSTAT3 was stained based on the manufacturer’s two-step Protocol for Fixation/Methanol (IC Fixation Buffer, cat# 00–8222, ThermoFisher Scientific). Zombie Red viability dye and the following fluorochrome-conjugated mAbs were purchased from BioLegend:PB-anti-CD45 (30-F11), PB-anti-CD44 (IM7), APC-Cy7-anti-CD45 (30-F11), BV711-anti-CD4 (RM4–5), BV785-anti-CD3 (17A2), BV605-anti-CD8a (53–6.7), PE-Cy7-anti-TNFα (MP6-XT22), Alexa Fluor 700-anti-Ki67 (16A8), Alexa Fluor 647-anti-T-bet (4B10); From ThermoFisher: FITC-anti-PD-1 (RMP1–30), FITC-anti-FoxP3 (FJK-16s), PE-eFluor 610-anti-Eomes (Dan11mag), PE-anti-Tim3 (RMT3–23), PerCP-eFluor 710-anti-Lag3 (eBioC9B7W), PE-anti-PD-L1 (MIH5), PE-Cy7-anti-CD62L (MEL-14), APC-anti-pSTAT3 (Tyr705) (LUVNKLA); From BD Biosciences: APC-Cy7-anti-IFNγ (XMG1.2). Details of antibodies including dilutions used and validation can be found in Supplementary Table 3. Flow cytometry data were acquired on an LSR Fortessa flow cytometer (BD Biosciences) and analyzed using FlowJo software (Tree Star).
Mouse tumor staining
For histological staining, B16-F0 tumors from DIO or lean control mice were fixed in 10% paraformaldehyde and embedded in paraffin. Multiple 4 μm sections were cut for Hematoxylin and eosin (H&E) staining Slides were prepared and stained at Histology Consultation Services, Inc. (Everson, WA). H&E slides of tumor samples were reviewed by a dermatologist/pathologist (Dr. R. R. Isseroff). Images were captured by a BZ-9000 BioRevo Fluorescence Microscope equipped with 10× numerical aperture objective lens (KEYENCE, Canada).
Mouse lung whole mount preparation
Lungs from B16-F10-bearing mice were collected, separated into five lobes and fixed in 10% neutral buffered formalin. Lung tissue was transferred to 70% alcohol for 2 hrs then 100% alcohol for another 2 hrs. Lungs were then dehydrated using three changes of xylene (30 min, 1 hr, 1 hr), followed by processing through a graded series of alcohol. After rinsing in running tap water for 30 min, the tissues were stained with hematoxylin for 2 min. Lungs were destained in a 1% HCl solution for 15 min, then were placed under running tap water for 30 min, 70% alcohol for 1 hr, 100% alcohol for 1 hr, and finally xylene for 1 hr. Whole mounts were then submerged in methyl salicylate for storage.
18F-FDG-PET scanning image analysis
6-month-old control and DIO mice bearing B16-F0 tumors were analyzed for uptake of FDG by PET-CT image. Mice were anesthetized using vaporized isoflurane and oxygene. The radiotracer 18F-FDG (7.92±0.45MBq) was administered via lateral tail vein injection. At 30
min post-radiotracer injection, mice were placed prone on the animal bed of a small-animal PET imaging system (Inveon DPET, Siemens Preclinical Solutions), and scanned. PET list mode data were acquired for 30
min. PET images were reconstructed using the 3D MAP reconstruction with a single hyperbolic prior (SP-MAP) provided by the vendor. After the PET scan, the animal bed was moved to an adjacent CT scanner (Inveon CT, Siemens Preclinical Solutions, Siemens Healthcare Molecular Imaging) for anatomical image acquisition. Animal respiration was visually monitored throughout both scans. Heat lamps were used to maintain consistent temperature. The PET and CT images were co-registered and analyzed using PMOD v3.8 software (PMOD Technologies). To determine tumor activity based on 18F-FDG uptake in individual lesions, volumes of interest (VOIs) were drawn at sites of the tumors on overlay images and the maximum standardized uptake value (SUVmax) was determined for each VOI.
MRI data acquisition and image analysis
Mice were anesthetized with isofluorane and oxygen. They were scanned on the Biospec 70/30 7.0 Tesla small-animal MRI system (Bruker Biospin Inc) using a 60mm quadrature transmitter/receiver coil for whole body imaging. The scanning protocol consisted of the multi-slice with multi-echo (MSME) spin-echo sequence with a single echo and with respiratory gating to minimize breathing artifacts. Scan parameters were: TE 7.062, TR 775, conducted with and without chemical-selective fat suppression. Slice images were obtained in the coronal direction to improve spatial resolution while keeping scan time and TR at minimums. The in-plane matrix was 200×267 with a resolution of 0.3×0.3 mm. 44 slices were acquired with a slice thickness of 0.6mm. Field of view was 6×8×2.64 cm. Difference images were generated by subtracting the fat-suppressed images from the non-fat suppressed images, to identify the 3D distribution of fat deposits. Physiological monitoring (temperature and respiration) was used during the entire scan to ensure consistency and animal physiological stability.
RNA Extraction, Sequencing, Analysis and PCR
Total RNA was extracted from sorted CD44+CD8+ T Cells from spleen and lymph nodes using RNeasy plus mini kit (Qiagen). RNA concentrations were quantified using a Qubit fluorometer and RNA integrity was assessed by TapeStation 2200 (Agilent). Samples with RIN (RNA integrity number) ≥ 9 were used for this study. After QC procedures, mRNA was submitted to Novogene for strand-specific library preparation and sequenced on a HiSeq 2500 instrument (150 pair-end sequencing). Data analysis was performed by the UC Davis Bioinformatics core using expHTS to trim low quality sequences and adapter contamination, and to remove PCR duplicates from raw reads. Trimmed reads for each sample were aligned to the GRCm38 mouse genome with GENCODE vM10 annotation, using STAR v. 2.5.2b aligner 51, which also generated raw counts per gene, which were the input to the statistical analysis.. The Bioconductor package edgeR was used for read normalization 52. The most significant pathways by functional interpretation of candidate gene lists were input and analyzed using G:Profiler by the g:GOST comprehensive method (p<0.05). Network analysis was completed on up- and down-regulated genes in both CD4+ and CD8+ T cells from enriched gene sets using GeneMANIA 53.
RNA was extracted from tumor and liver tissue using Qiagen RNeasy Lipid Tissue Mini kit (QIAGEN, Valencia, CA) according to manufacturer’s protocol with optional on column DNase (QIAGEN, Valencia, CA). RNA was quantified using Qubit 4 (ThermoFisher Scientific, Grand Island, NY) RNA Broad range and 300ng RNA was converted to cDNA using Bio-Rad iScrpt Reverse Transcription Supermix (Bio-Rad, Hercules, CA) following standard protocols. qPCR was performed on a Bio-Rad CFX384 (Bio-Rad, Hercules, CA) with Bio-Rad SsoAdvanced Universal SYBR Green Supermix (Bio-Rad, Hercules, CA) in 10ul using 5ng cDNA. Primers for PD-1 (NM_008798.2) F-GCAGTTGAGCTGGCAATCAG, R-GGTGAAGGTGGCATTTGCTC, CPT1A (NM_013495.2) F-CACTGCAGCTCGCACATTAC, R-CCAGCACAAAGTTGCAGGAC, and HAVCR2 (NM_134250.2) F-CCTTGGATTTCCCCTGCCAA, R- CCCAGCACATAGGCACAAGT) designed using Primer-BLAST on https://www.ncbi.nlm.nih.gov/tools/primer-blast/, and EOMES (Qiagen, PPM32970F-200) were compared to GAPDH (PPM02946E) (QIAGEN, Valencia, CA) and β-actin (PPM02945B) (QIAGEN, Valencia, CA) as reference genes.
Non-human primate studies
This study was performed at the California National Primate Research Center (CNPRC) under strict compliance with the NIH Guide for the Care and Use of Laboratory Animals. The CNPRC, which is one of 7 centers supported by the National Institutes of Health, Office of the Director (NIH/OD), is accredited by AAALAC. The animal protocol was approved by UC Davis IACUC. Peripheral blood samples were obtained from adult (6–10-year old) male lean (7–9 kg) and obese (14.5–20 kg) rhesus macaques (Macaca mulatta). PBMCs were isolated by density centrifugation. Staining for flow cytometry was done with aqua viability dye (Invitrogen) and fluorochrome-conjugated antibodies against the following markers purchased from Biolegend: PE-anti-PD-1 (EH12.2H7) and BD Biosciences: PB-anti-CD3 (SP34–2), BV605-anti-CD4 (L200), AF700-anti-CD8 (RPA-T8), APC-anti-CD95 (DX2), and APC-H7-anti-CD28 (CD28.2)). Details of antibodies including dilutions used and validation can be found in Supplementary Table 3. For the proliferation assay, 1×106 PBMCs were cultured for four days in an anti-CD3ε-coated 96-well round bottom plate (10 μg/mL/50 μL/well 2 hr incubation at 37°C, followed by two washes with PBS), clone SP34, BD Biosciences). Cells were stained with the same surface marker antibody panel listed above and intracellular staining was performed with fixation/permeabilization solutions (BD Biosciences) to stain for Ki67 (clone B56 on AF488, BD Biosciences).
Human blood samples
Human blood collection study was approved by the IRB at the University of California at Davis. Human studies complied with all ethical regulations. Study participants were healthy female donors aged 27–56 years with no history of cancer, hypersensitivity, hyperglycemia, and/or hyperinsulinemia. Body mass index (BMI) for each participant was calculated as mass (kg)/height (m) ^2. Non-obese BMI was defined as <30 and obesity was defined as BMI of ≥ 30. PBMCs were isolated from peripheral blood samples of each donor using Ficoll-Paque density centrifugation. Cells were stained with fluorochrome-conjugated mAbs listed below in staining buffer with 1% human serum. Isotype-matched rat or mouse IgG mAbs were used as negative staining controls Zombie Red viability dye and the following fluorochrome-conjugated mAbs were purchased from BioLegend BV605-anti-CD8a (RPA-T8), BV711-anti-CD4 (OKT4), PE-anti-PD-1 (EH12.2H7), AF700-anti-CD45RA (HI100); From BD: FITC-anti-CD62L (SK11), BV421-anti-CD3 (HIT3a), BV421-anti-CD45RO (UCHL1); From ThermoFisher: APC-anti-Ki67 (20Raj1).
Naïve (CD3+CD45RA+CD45RO−) versus effector/memory (CD3+CD45RA−CD45RO+) T cells were isolated by a Beckman Coulter Astrios flow cytometric sorter. 2×105 cells were plated in each well and stimulated with 2.5 μg/mL ConA with or without the addition of 10 nM recombinant human leptin (rhleptin, Gibco LifeTechnologies) for 48 hours at 37°C in a 96-well flat bottom plate. Cell numbers were counted by using Automated Cell Counter (Bio-rad, TC20™).
Human CD3 tissue staining
A serially collected cohort of 152 colorectal cancer patients seen in the Yale Surgical Pathology suite was previously arranged into a tissue microarray (TMA) called YTMA 226 with annotated clinical data. This TMA was stained using validated / standardized multiplexed QIF panels as previously described54. We measured the levels of PD-1 (clone EH33, CST), CD3 (polyclonal, DAKO), and nuclear DAPI staining. Two TMA histospots were evaluated for each case and the average was obtained. Cases that have less than 5% tumor tissue in a histospot have been excluded from the analysis. Quantitative measurement of the fluorescence signal was performed using the AQUA method of QIF. Differences in QIF between samples from non-obese (BMI<30) and obese (BMI≥30) patients were compared using a two-tailed Student’s t-test.
TCGA analysis
TCGA-SKCM melanoma tumor mRNA expression data from 251 cases with clinical data including BMI were downloaded with the TCGAbiolinks55 R package from the GDC legacy archive (https://portal.gdc.cancer.gov/legacy-archive/), figures represent 126 patients (>60 years old). Differential expression analysis was carried out by using DESeq256 bioconductor R package (https://www.R-project.org/).
Human PD-(L)1 therapy clinical data
Using an IRB approved protocol, we reviewed all cancer patients, age 18 years or older, treated with anti PD-1 and PD-L1 therapy at the University of Oklahoma Health Sciences Center between Jan/2014 and Jan/2018 (n=250). Simple descriptive statistics were created for all covariates [mean, SD for continuous covariates and n (%) for categorical variables] overall and by BMI group. Kaplan-Meier Analysis was performed to assess how BMI category (< 30 versus ≥30) related to overall survival (OS) and progression free survival (PFS). A Cox proportional hazards model was used to assess the association of BMI category with OS and PFS adjusted for ECOG performance status, line of treatment, age, sex, and cancer type, checking for interactions between BMI category and other covariates.
Statistics
Graphs were made and statistical analyses were performed using Prism software (GraphPad Software Inc.). Data were expressed as mean ± SEM. For analysis of three or more groups, a ANOVA tests were performed with a Bonferroni or Tukey post- test, when appropriate. Analysis of differences between two normally distributed test groups was performed using the Student’s t-test. p-values were considered statistically significant when p < 0.05. Statistical outliers were identified using Grubb’s test. Statistical differences in survival were determined by log rank (Mantel–Cox) analysis.
Acknowledgements
We would like to thank W. Ma, and M. Metcalf from the Murphy lab, and D. Rowland, A. Chaudhari and Z. Harmany from the UC Davis CMGI, and J. Chen in the UC Davis Pathology Core for their technical expertise and help. We would also like to thank the other members in the Murphy lab for providing feedback and suggestions during preparation of the manuscript. This was work funded by NIH grant R01 CA095572, R01 CA195904, R01 CA214048, P01 CA065493, R01 HL085794, the California National Primate Research Center base operating grant (OD011107), the UC Davis Comprehensive Cancer Center Support Grant (CCSG) (P30 CA093373), and the UC Davis Mouse Metabolic Phenotyping Center (MMPC) grant (DK092993).
The content of this publication does not necessarily reflect the view or policies of the Department of Health and Human Services, nor does mention of trade names, commercial products, or organizations imply endorsement by the U.S. Government. This research was supported in part by the Intramural Research Program of the NIH, NCI, NHLBI and Center for Cancer Research.
Footnotes
Competing Interests Statement
The authors have no competing interests to declare.
Data Availability Statement
The datasets generated during and/or analyzed during the current study are available from the corresponding author on reasonable request. The datasets generated during and/or analyzed during the current study are available in the NCBI BioSample repository under accession # SAMN09873568, SAMN09873569, https://www.ncbi.nlm.nih.gov/biosample/9873568, https://www.ncbi.nlm.nih.gov/biosample/9873569.
Accession Codes
NCBI BioSample repository accession # SAMN09873568 and SAMN09873569
References (Main Text)
Full text links
Read article at publisher's site: https://doi.org/10.1038/s41591-018-0221-5
Read article for free, from open access legal sources, via Unpaywall:
https://europepmc.org/articles/pmc6324991?pdf=render
Citations & impact
Impact metrics
Citations of article over time
Alternative metrics
Article citations
A multidimensional analysis of the impact of obesity on immune checkpoint inhibitor therapy efficacy.
Cancer Cell Int, 24(1):358, 29 Oct 2024
Cited by: 0 articles | PMID: 39472922 | PMCID: PMC11523605
Impact of race, ethnicity, and social determinants on outcomes following immune checkpoint therapy.
J Immunother Cancer, 12(10):e010116, 26 Oct 2024
Cited by: 0 articles | PMID: 39461882 | PMCID: PMC11529590
Review Free full text in Europe PMC
Unraveling the Obesity Paradox: Exploring the Impact of Body Weight on Cutaneous Melanoma Prognosis in Asian Population.
Diabetes Metab Syndr Obes, 17:3863-3873, 19 Oct 2024
Cited by: 0 articles | PMID: 39449863 | PMCID: PMC11499613
Exploring risk factors for endocrine-related immune-related adverse events: Insights from meta-analysis and Mendelian randomization.
Hum Vaccin Immunother, 20(1):2410557, 08 Oct 2024
Cited by: 0 articles | PMID: 39377304 | PMCID: PMC11469449
Regulation of CD8+ T cells by lipid metabolism in cancer progression.
Cell Mol Immunol, 21(11):1215-1230, 14 Oct 2024
Cited by: 0 articles | PMID: 39402302 | PMCID: PMC11527989
Review Free full text in Europe PMC
Go to all (428) article citations
Data
Data behind the article
This data has been text mined from the article, or deposited into data resources.
BioStudies: supplemental material and supporting data
BioSamples (2)
- (2 citations) BioSamples - SAMN09873569
- (2 citations) BioSamples - SAMN09873568
Similar Articles
To arrive at the top five similar articles we use a word-weighted algorithm to compare words from the Title and Abstract of each citation.
Immune checkpoint blockade reprograms systemic immune landscape and tumor microenvironment in obesity-associated breast cancer.
Cell Rep, 35(12):109285, 01 Jun 2021
Cited by: 41 articles | PMID: 34161764 | PMCID: PMC8574993
Response to immune checkpoint blockade improved in pre-clinical model of breast cancer after bariatric surgery.
Elife, 11:e79143, 01 Jul 2022
Cited by: 14 articles | PMID: 35775614 | PMCID: PMC9342954
PD-1 Blockade Reverses Obesity-Mediated T Cell Priming Impairment.
Front Immunol, 11:590568, 29 Oct 2020
Cited by: 7 articles | PMID: 33193426 | PMCID: PMC7658608
Recent advances in the clinical development of immune checkpoint blockade therapy.
Cell Oncol (Dordr), 42(5):609-626, 14 Jun 2019
Cited by: 48 articles | PMID: 31201647
Review
Funding
Funders who supported this work.
NCI NIH HHS (7)
Grant ID: R01 CA214048
Grant ID: R01 CA195904
Grant ID: P30 CA225520
Grant ID: P01 CA065493
Grant ID: P30 CA093373
Grant ID: R01 CA095572
Grant ID: T32 CA009138
NHLBI NIH HHS (3)
Grant ID: R01 HL056067
Grant ID: R24 HL085794
Grant ID: R01 HL118979
NIAID NIH HHS (1)
Grant ID: R37 AI034495
NIDDK NIH HHS (1)
Grant ID: U24 DK092993
NIH HHS (2)
Grant ID: S10 OD018223
Grant ID: P51 OD011107