Abstract
Motivation
A key component in many RNA-Seq-based studies is contrasting multiple replicates from different experimental conditions. In this setup, replicates play a key role as they allow to capture underlying biological variability inherent to the compared conditions, as well as experimental variability. However, what constitutes a 'bad' replicate is not necessarily well defined. Consequently, researchers might discard valuable data or downstream analysis may be hampered by failed experiments.Results
Here we develop a probability model to weigh a given RNA-Seq sample as a representative of an experimental condition when performing alternative splicing analysis. We demonstrate that this model detects outlier samples which are consistently and significantly different compared with other samples from the same condition. Moreover, we show that instead of discarding such samples the proposed weighting scheme can be used to downweight samples and specific splicing variations suspected as outliers, gaining statistical power. These weights can then be used for differential splicing (DS) analysis, where the resulting algorithm offers a generalization of the MAJIQ algorithm. Using both synthetic and real-life data, we perform an extensive evaluation of the improved MAJIQ algorithm in different scenarios involving perturbed samples, mislabeled samples, same condition groups, and different levels of coverage, showing it compares favorably to other tools. Overall, this work offers an outlier detection algorithm that can be combined with any splicing pipeline, a generalized and improved version of MAJIQ for DS detection, and evaluation metrics with matching code and data for DS algorithms.Availability and implementation
Software and data are accessible via majiq.biociphers.org/norton_et_al_2017/.Contact
[email protected].Supplementary information
Supplementary data are available at Bioinformatics online.Free full text

Outlier detection for improved differential splicing quantification from RNA-Seq experiments with replicates
Abstract
Motivation
A key component in many RNA-Seq-based studies is contrasting multiple replicates from different experimental conditions. In this setup, replicates play a key role as they allow to capture underlying biological variability inherent to the compared conditions, as well as experimental variability. However, what constitutes a ‘bad’ replicate is not necessarily well defined. Consequently, researchers might discard valuable data or downstream analysis may be hampered by failed experiments.
Results
Here we develop a probability model to weigh a given RNA-Seq sample as a representative of an experimental condition when performing alternative splicing analysis. We demonstrate that this model detects outlier samples which are consistently and significantly different compared with other samples from the same condition. Moreover, we show that instead of discarding such samples the proposed weighting scheme can be used to downweight samples and specific splicing variations suspected as outliers, gaining statistical power. These weights can then be used for differential splicing (DS) analysis, where the resulting algorithm offers a generalization of the MAJIQ algorithm. Using both synthetic and real-life data, we perform an extensive evaluation of the improved MAJIQ algorithm in different scenarios involving perturbed samples, mislabeled samples, same condition groups, and different levels of coverage, showing it compares favorably to other tools. Overall, this work offers an outlier detection algorithm that can be combined with any splicing pipeline, a generalized and improved version of MAJIQ for DS detection, and evaluation metrics with matching code and data for DS algorithms.
Availability and implementation
Software and data are accessible via majiq.biociphers.org/norton_et_al_2017/.
Supplementary information
Supplementary data are available at Bioinformatics online.
1 Introduction
Alternative splicing (AS), the process by which segments of pre-mRNA can be arranged in different subsets to yield distinct mature transcripts, is a major contributor to transcriptome complexity. In humans, over 90% of multi-exon genes are alternatively spliced, and most of these exhibit splicing variations which are tissue- or condition-dependent (Pan et al., 2008). This key role of AS in transcriptome complexity, combined with the fact that aberrant splicing is commonly associated with disease state (Wang and Cooper, 2007), has led to various research efforts. These include efforts to accurately map transcriptome complexity and identify splicing variations between different cellular conditions, across developmental stages, or between cohorts of patients and controls.
Detection of splicing variations and the mapping of transcriptome complexity has been greatly facilitated by the development of high-throughput technologies to sequence transcripts, collectively known as RNA-Seq. Briefly, RNA from the cells of interest, typically poly-A selected or ribo-depleted, are sheared to a specific size range, amplified, and sequenced. In most technologies used today, the resulting sequence reads are typically around 100 bp with read number varying greatly, from around 20 to 200 M reads. The shortness of the reads, their sparsity, and various experimental biases make inference about changes in RNA splicing a challenging computational problem (Alamancos et al., 2014). To aid RNA-Seq based analysis, many studies include samples which are biological replicates of the conditions being studied. Replicates are a key component in helping researchers distinguish between the biological variability of interest (e.g. differences between two tissues) and variability associated with experimental or technical factors. However, what constitutes a ‘good’ replicate or an outlier experiment is not always clear. Intuitively, in the context discussed here, an outlier is a sample which exhibits disproportionately large deviations in exon inclusion levels compared with other samples from the same experimental condition (biological replicates). An outlier could be the result of a failed experiment or of some hidden deviation in sample identity (i.e. different tissue source). Remarkably, despite the obvious importance of the question of what constitutes an outlier, this question has been mostly ignored in the literature. Instead, researchers are left to define outliers based on heuristics which may not be accurate or carry unconscious biases. For example, in a recent work focused on good practices for RNA-Seq data analysis, the authors recommend performing PCA analysis to see if samples from the same condition ‘cluster together’ but admit ‘no clear standard exists for biological replicates’ (Conesa et al., 2016). As we demonstrate here, the presence of outliers can have deleterious effects on algorithms that aim to detect differential splicing (DS) between groups of replicates from different conditions. Thus an important contribution of this work is to formalize what constitutes an outlier replicate and suggest a method which researchers could use to detect outliers before applying any DS algorithm.
A second contribution of this work is in assessing the effect of outliers on DS algorithms and, more broadly, producing a general framework with matching datasets for assessing the performance of DS algorithms. Generally, DS algorithms can be divided into two classes. The first, which includes tools such as RSEM (Li and Dewey, 2011) and Cuffdiff (Trapnell et al., 2012), aims to quantify full gene isoforms, typically by assuming a known transcriptome and assigning the observed reads to the various gene isoforms in the given transcriptome database. The second class of algorithms, which includes rMATS (Shen et al., 2014) and DEXSeq (Anders et al., 2012), works at the exon level, detecting differential inclusion of exons or exonic fragments. Some algorithms, such as SUPPA (Entizne et al., 2017), can be considered a hybrid as they collapse isoform abundance estimates from other algorithms such as Salmon (Patro et al., 2017) to compute relative exon inclusion levels. Previous works showed that for the task of DS, quantification algorithms that work at the exon level generally perform better since they solve a simpler task and are less sensitive to isoform definitions or RNA-Seq biases within samples or along full isoforms (Liu et al., 2014). Thus, for the comparative analysis section of this paper, we focus on the second class of algorithms, and specifically the commonly used DEXSeq, rMATS, as well as the recently updated SUPPA, all of which support replicates. Using these algorithms and the one we propose (MAJIQ-lw) we detail here several metrics to assess DS algorithm performance based on biological or technical replicates. We also develop a synthetic dataset where each sample is generated so as to model a matching real sample.
A third contribution of this work is in developing MAJIQ-lw, an adaption of our recently published MAJIQ algorithm (Vaquero-Garcia et al., 2016) to automatically detect and downweight samples and splicing variations suspected to be outliers. MAJIQ offers a combined solution to detect, quantify, and visualize DS between experimental conditions with or without replicates. Besides the details of its statistical model and its visualization capabilities, two key features distinguish MAJIQ from the DS algorithms mentioned above. First, MAJIQ allows users to supplement previous transcriptome annotation with reliably detected de novo junctions, which results in over 30% increase in detection of differential variations and robustness to transcriptome definitions (Vaquero-Garcia et al., 2016). Second, MAJIQ uses a novel formulation for transcript variations. It does not quantify whole gene isoforms as the first class of algorithms described, nor does it use only the previously defined AS ‘types’ (e.g. cassette exons) as the second class of algorithms. Instead, MAJIQ defines a more general concept of ‘local splicing variations’, or LSVs. Briefly, LSVs are defined as splits in a gene splice graph where a reference exon is spliced together with other segments downstream or upstream of it (see Fig. 1a). Importantly, the formulation of LSVs enables MAJIQ to capture all previously defined types of AS but also many other variations which are more complex (see Fig. 1b and c). Similar to classical AS events, LSVs are quantified by the fraction of inclusion of each of the junctions, termed percent spliced in (PSI,
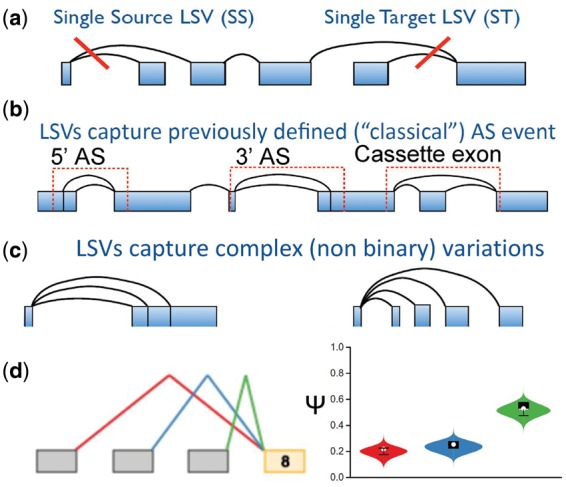
Illustration of LSVs. (a) LSVs are splits in a gene splice graph where multiple edges spawn out from (single-source, 5’ split) or merge into (single-target, 3’ join) a reference exon. (b) The LSV definition captures classical binary splicing event types. (c) The LSV definition can capture complex variations that involve more than two junctions. (d) A sample from MAJIQ’s output for a single-target LSV involving Exon 8 of Clta gene in mouse cerebellum. MAJIQ quantifies the LSV shown on the left by the marginal inclusion level (
2 Materials and methods
2.1 Outlier weight model
Let
In order to make the above definition formal we first need to specify a way to estimate
This allows us to define the deviation of sample t for LSV i as
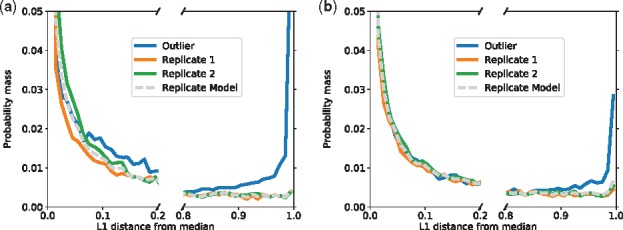
Per-experiment empirical PDF over LSVs L1 divergences from group median. The L1 divergence (
This formulation has the desired characteristic that ρ = 1 for ‘well behaved’ experiments for which Kt is less than the expected
In addition, the above global weight per-experiment ρt can be plugged into MAJIQ to produce a generalized version of the previous MAJIQ algorithm:
where
2.2 Local weights
The previous section described MAJIQ-gw as a way to globally weigh experiments using ρt so that outliers can be effectively detected and suppressed. However, by doing this we run the risk of discarding valuable information: Even if a sample is a bone-fide outlier, it is quite likely that this is a result of a subset of LSVs, while the majority of the LSVs are good representatives of the condition of interest
First, we estimate the null distribution of ‘well-behaved’ replicates by weighting the per-experiment densities of
where ε is a reasonable neighborhood parameter (default 0.05),
2.3 Performance evaluation metrics
When using synthetic RNA-Seq samples one can use standard measures of performance such as false discovery rate (FDR) or false negative rate (FNR). For real data, there is an inherent challenge in assessing the accuracy of DS methods since the underlying true values are rarely known. This problem is corroborated by the fact that different DS methods define transcriptome variations differently (Exonic segments, classical AS events, LSVs) and use vastly different criteria to detect those. DEXSeq, e.g. analyzes expression of exonic segments and assigns a P-value to expression changes of those. Other methods discussed here instead quantify the relative inclusion of RNA segments (PSI) yet still vastly differ in the underlying model and statistical criteria. One issue regarding comparison of different AS units is possible redundancy or overlap. For example, two overlapping single-source and single-target LSVs can capture the same differentially spliced cassette exon reported by other methods (see illustration in Fig. 1b). To avoid such cases of ‘double counting’ we impose an additional constraint on MAJIQ’s output where LSVs are not allowed to overlap. However, this constraint does not resolve the issue that a single LSV detected by MAJIQ may contain several ‘classic’ binary AS events reported separately by other methods. Here we suggest to circumvent the above challenges of different AS definitions and statistical criteria by focusing instead on ranked-based statistics derived using multiple biological and technical replicates. These statistics are applied to each method’s own units of variations, assessing how reproducible are the algorithm’s significantly changing events and how likely they are to be related to changes between the conditions of interest.
We start by describing the reproducibility ratio (RR) measure, following (Vaquero-Garcia et al., 2016). The RR measure is similar in spirit to the ireproducible discovery rate, which has been used extensively to evaluate ChIP-Seq peak calling methods (Li et al., 2011) and, more recently, for methods detecting cancer driver mutations (Tokheim et al., 2016). Conceptually, RR measures the proportion of high-ranked events (e.g. ChIP-Seq peaks or, in our case, differentially spliced events) that are also observed in a second, independent iteration of the same experimental procedure using biological or technical replicates. To compute RR, an algorithm A is run on a set of samples S1 comprised of two groups of replicates from two conditions (e.g. liver and cerebellum tissues). Algorithm A reports a set of AS events
Although useful, the RRA statistic may not be sufficient to assess an algorithm’s performance. First, both RRA and NA are not inherent characteristics of an algorithm but rather a combination of an algorithm and a specific dataset. Second, reproducibility alone is not a guarantee for accuracy as algorithms can be highly reproducible yet maintain a strong bias. Thus, in order to better assess accuracy of DS methods we combine RR with two additional tests. First, we assess how many DS events are reported by each algorithm when comparing groups of samples that are either biological or technical replicates from the same experimental condition. Biological replicates were obtained from the original studies we used, detailed below. To obtain technical replicates, we randomly partition the original paired-end FASTQ files and remap each read subset. This procedure results in a very strict definition of technical replicates which are experiments by the same lab, protocol, biological sample, machine and lane. Presumably, true variation in any specific sample would be canceled out when comparing two groups of replicates. However, since we can not completely rule out true variations between two groups of technical or biological replicates, we denote the reported DS events as putative FP, or PFP. Finally, we compute for each algorithm the intra-to-inter ratio (IIR) statistic, which is the ratio between the number of PFP and the number of DS events reported when comparing equivalent groups but from different conditions, i.e.
Finally, to further assess accuracy, we used RT-PCR triplicate experiments from previous studies (Vaquero-Garcia et al., 2016). We note this measure also carries limitations as it is constrained by the total number of events quantified, possible selection biases, and limitations of the experimental procedure. We discuss some of those limitations in Section 4. Nonetheless, carefully executed RT-PCR provide valuable independent experimental validation and is considered the gold standard in the RNA field.
2.4 Data and software versions
In order to perform some of the evaluations described below, datasets with at least six replicates are required. Most of the results described in the next section were derived using RNA-seq experiments from a recent study by Zhang et al. (2014), which includes samples from twelve mouse tissues (heart, hypothalamus, adrenal, brown and white fat, cerebellum (Cer), liver (Liv), muscle (Mus), lung, aorta, brainstem and kidney) across four circadian time points, repeated twice, with 60–120 million reads per experiment. When comparing groups of samples in the analysis described below time points were matched between the groups to avoid time as a confounding factor. The high coverage allowed us to also compare groups of technical replicates by randomly partitioning files to subsets of 50 and 25% of the original reads. To show our comparative results are not specific to the data of Zhang et al. (2014), we also repeated much of the analysis in a different dataset from the Mouse Genome Project (Keane et al., 2011), which includes six tissues (heart, hippocampus, liver, thymus, spleen, and lung) with six biological replicates each, at 18–30 million reads per experiment (see Supplementary Material).
For the comparative analysis we used DEXSeq version 1.18.4, rMATS-turbo version 0.1, SUPPA from public GitHub source pulled on 21 June 2017, and MAJIQ version 1.0.6.
2.5 Synthetically perturbed and mislabeled samples
To observe the impact of an outlier sample in a controlled fashion, we used a real biological replicate and perturbed it to create a synthetic new pseudo-replicate outlier. Three parameters were used to control the sample’s perturbation:
We also explore the effects of mislabeling a sample as an extreme case of an outlier. We simulate this by swapping out one replicate with a sample from a different condition within the same dataset. Specifically, in the analysis described below we swapped a Cerebellum sample for a Muscle sample.
2.6 Synthetic dataset
Synthetic datasets are commonly used to evaluate algorithms, including those for DS analysis, under controlled settings. Some works use actual sequencing of synthetically generated samples with specific transcripts spiked at different concentrations. Using spiked transcripts helps avoid making simplifying assumptions on factors such as read distribution but the sequenced samples may be very different from real life samples. This limitation along with the associated technical complexity and costs, lead most works that employ synthetic datasets to resort to in silico generated datasets. However, these datasets typically involve strong simplifying assumptions about expression levels, distribution of variation in and between the groups compared, read errors, coverage levels, underlying known transcriptome, and variants that involve only predefined ‘classical’ AS event types such as cassette exons.
In order to create more realistic synthetic data for DS analysis, we again employed real data from biological replicates, and developed an LSV-based approach for in silico RNA-Seq generation. Specifically, given a set of real RNA-Seq samples, we create a matching synthetic dataset using the following procedure: First, we estimate each gene’s coverage in each sample using standard FPKM. Next, we monitor for each gene g the most complex LSV (
Several key points are important to make regarding the above synthetic data generation scheme. First, expression levels and transcriptome variations are not based on a synthetic model but on real data. Second, the LSV formulation used here offers a way to define a lower bound on transcriptome complexity in each sample without resorting to assumptions about specific variations such as cassette exons. Third, we stress that the LSV detection and quantification is based directly on STAR junction-spanning reads and raw read ratios to avoid biasing estimations towards any specific algorithm. Finally, since each sample is modeled after a real experiment, no algorithm-specific assumptions are made about group-specific variations.
3 Results
To demonstrate the robustness of both the global (MAJIQ-gw) and local (MAJIQ-lw) weighting algorithms, we applied the synthetic perturbation procedure in Section 2.5 to one experiment in a group of three cerebellum samples from (Zhang et al., 2014), varying each of the three hyperparameters
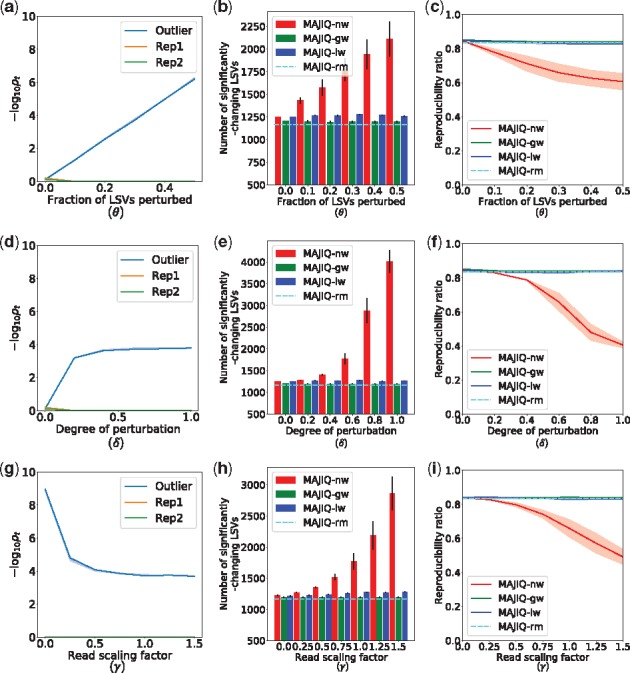
Synthetic perturbation of tissue replicates. In each test, three cerebella were compared against three livers from Zhang et al. (2014), but one of the cerebella was perturbed. Perturbation procedure detailed in Section 2.5. MAJIQ-nw is the previous algorithm equivalent to fixed weights (
Given the above results, we decided to focus subsequent comparative analysis on MAJIQ with local-weights (MAJIQ-lw) and the original algorithm (MAJIQ-nw). Using the procedure described in Section 2.6 we created a synthetic dataset based on real RNA-Seq samples from (Keane et al., 2011), involving mouse liver and hippocampus with 5-6 biological replicates per tissue. Following a common criteria in the RNA splicing field we used
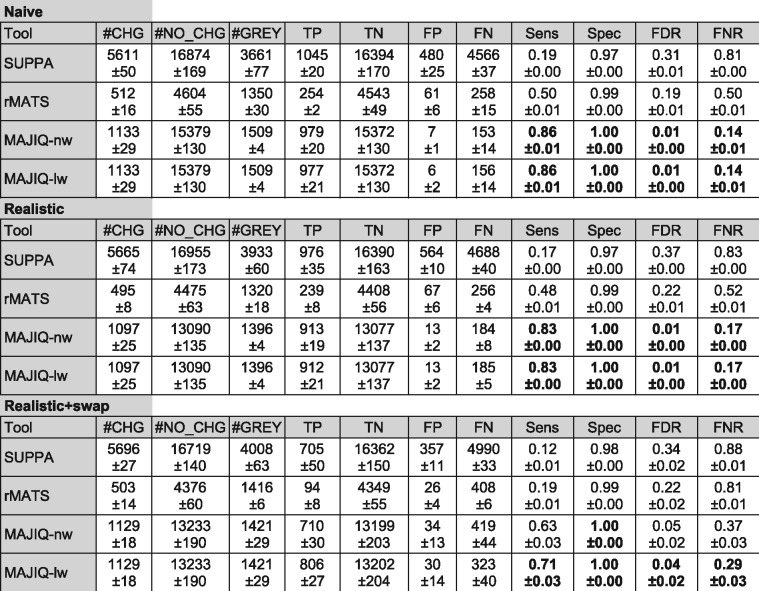
Evaluation using ‘realistic’ synthetic datasets. Each synthetic sample is created to match a real sample in terms of gene expression and a lower bound on transcriptome complexity. Three datasets were created: ‘Naive’ with naive read simulation (uniform coverage, no errors, biases or indels); ‘Realistic’ with more realistic read generation; ‘Realistic + swap’ where one sample in an outlier. All datasets involve three biological replicates per group. Each method was evaluated using its own definition of AS events, which means the number of events is not comparable between methods. CHG are changing events (
Next, we turned to evaluating the algorithms’ performance on real data with and without a sample swap. In all cases, we used three cerebellum versus three liver for the validation Set 2. Set 1 included either 3 cerebellum compared with three liver samples (control, no swap) or two cerebellum and one muscle compared with three livers (swap case). We chose to evaluate these tissues specifically because of the large number of reproducibly changing LSVs between them (Vaquero-Garcia et al., 2016), though similar results were observed for other tissues as well as for a different dataset from (Keane et al., 2011) (see Supplementary Figures). To produce the reproducibility curves discussed below, we used the following procedure. For MAJIQ, NA was defined as the set of events for which
Figure 5a and matching Supplementary Figures summarize the RR results for the control (no swap) and outlier (swap) cases. As noted in Section 2.3, one immediate observation is the huge difference in the number of events reported as significantly changing (NA), a consequence of the vastly different statistical criteria and event definition used by the various algorithms. Specifically, MAJIQ, which captures de-novo junctions and may combine multiple classical AS events into a single complex LSV, detects 22 766 events and 15 336 non overlapping ones while rMATS reports 18 732 events and SUPPA, which was run with RefSeq according to authors’ recommendation, only detects 7770 events. rMATS and MAJIQ report roughly 9% of the events as differentially spliced compared with 27% by SUPPA. DEXSeq which measures expression of exonic segments, naturally reports many more units detected (75 871) and finds about 40% of those to be differentially included (see Supplementary Fig. S2e for details).
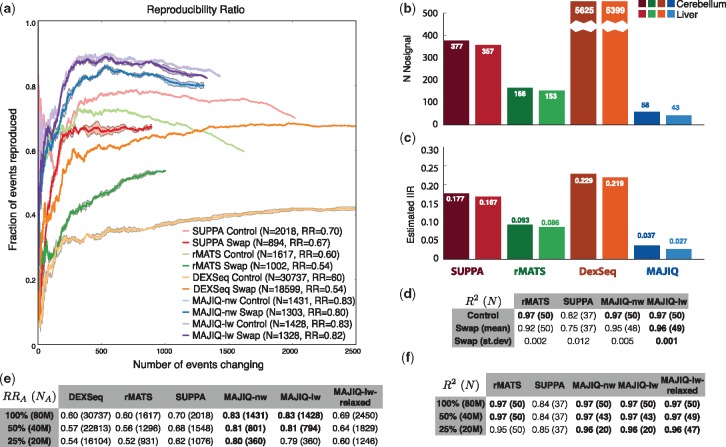
Evaluation using real data. (a)RR plots for detection of DS events between cerebellum and liver, with (Swap, dark line) or without (Control, faded line) a mislabeled muscle. The end of the line marks the point in the graph matching the number of events reported as significantly changing (RR(NA), see main text). Because DEXSeq report more than 3000 significantly changing exonic segments, we present its extended RR curve in Supplementary Figures. (b) Number of events reported by each methods as significantly changing between two groups of biological replicates from the same condition (light color—liver, dark color—cerebellum). See Supplementary Material for equivalent plots when comparing groups of technical replicates. (c) The IIR, representing the ratio between the number of DS events reported when comparing biological or technical replicates (NPFP) and the number of events reported when comparing similarly sized groups but from different conditions (NA, see Section 2.3 for details). See Supplementary Figures for equivalent plots when NPFP is derived using technical replicates. (d) The same control and swap experimental setup as in (a) with accuracy assessed using 50 RT-PCR experiments from (Vaquero-Garcia et al., 2016). Values represent fraction of variations explained (R2) and the number of events detected in parentheses. DEXSeq is not included here since it does not output
As shown in Figure 5a, the RR(n) was typically noisy for low n, which is to be expected, but varied greatly between algorithms and between runs with or without an outlier. MAJIQ consistently exhibited higher reproducibility compared with other algorithms regardless of n, with MAJIQ-lw and MAJIQ-nw practically tied in the control case with no outlier (
Next, we assessed the number of events reported by each method as DS between groups of biological or technical replicates from the same two conditions (liver, cerebellum) used in Figure 5a. Figure 5b and c show the resulting putative false positives (NPFP) and IIR (IIR =
Using 50 DS events quantified by Vaquero-Garcia et al. (2016) for the same two tissues from Zhang et al. (2014), we quantified the correlation between RNASeq based
Finally, we tested the effect of read coverage levels on the various algorithms. In general, all methods take into account the number of reads that hit an AS event when assessing its statistical significance. Consequently, we do not expect to see a dramatic effect on the reproducibility of events deemed significantly changing (RR) but rather a significant reduction in the number of events detected as differentially spliced (NA). Unlike other methods, MAJIQ relies solely on junction-spanning reads and is therefore likely to suffer more in detection power if its default parameters are not adjusted accordingly. We therefore tested two different MAJIQ execution configurations. The first is MAJIQ’s default settings, which are particularly conservative. These settings were designed for high reproducibility assuming multiple replicates with an average coverage of ~60 M and read length of 100 bases as in (Zhang et al., 2014). The second configuration, denoted ‘relaxed’, drops the quantifiable filter restrictions to allow the detection of junctions in a single experiment with as few as two reads mapping to two or more unique positions across the junction. We note that the other algorithms we analyzed do not have such coverage specific user adjustable parameters as these do no rely solely on junction spanning reads and as we show are less sensitive to changes in coverage.
By randomly sub-sampling the FASTQ files from (Zhang et al., 2014), read coverage was varied from the original average of 80 million reads (denoted 100%) through 40 million (50%) to 20 million (25%). The RR and detection power of each method evaluated is reported in Figure 5e, and correlations with RT-PCR quantifications in Figure 5f. In general, all methods lose power to detect differentially spliced events as coverage drops. As expected, MAJIQ run with default parameters is the most sensitive to sparse coverage, with detected events dropping up to 75%. When run with the relaxed parameters, MAJIQ-lw loses 50%, while rMATS, SUPPA, and DEXSeq lose 37, 32 and 48% respectively. However, MAJIQ’s conservative settings pay off in consistent high reproducibility of 79–83% compared with 61–70% for MAJIQ-lw with relaxed settings, and generally lower values for other algorithms. Similarly, when evaluating IIR we found MAJIQ-lw relaxed settings slightly increased the IIR by about 4% while detecting many more events overall (see Supplementary Figure). When evaluating the effect of sparse read coverage using the 50 RT-PCR experiments (Fig. 5f), we find a similar picture. It is worth noting that these 50 events were designed by (Vaquero-Garcia et al., 2016) to cover the wide range of read coverage levels across splicing events. Here, SUPPA and rMATS maintain the same number of detected events for all coverage levels and similar R2 values. For MAJIQ, the default and the relaxed settings maintain high R2 values which compare favorably to the other methods, but the default settings loses 62% of the events (19 versus 50).
4 Discussion
In this article, we developed a new model to automatically detect and downweight outliers in RNA-Seq datasets with replicates for DS analysis. The problem of detecting outliers in batches of biological replicates has not received much attention in the literature. As a result, researchers may include outliers that might affect their results, or discard samples before publication based on some heuristic such as PCA (Conesa et al., 2016). Using such a heuristic to discard samples may also reflect unconscious bias or cause good samples to be lost. Instead, we proposed a model for what constitutes an outlier sample in splicing analysis. This model is supported by our software, MAJIQ, so users can evaluate possible outliers regardless of which pipeline they use.
In addition, we developed an outlier weighing scheme and incorporated it into MAJIQ’s model either at the global, per-sample, level (MAJIQ-gw) or locally at the LSV level (MAJIQ-lw). Using both synthetic data perturbation and real data, we demonstrated that both MAJIQ-gw and MAJIQ-lw can maintain high levels of reproducibility when confronted with outliers. Furthermore, even when a sample is an outlier, using the local weight model (MAJIQ-lw) can help gain detection power by retaining LSVs which are ‘well-behaved’. In addition to sensitivity to outliers, we showed that low read coverage can also affect MAJIQ’s detection power as it relies on junction spanning reads. However, by adjusting MAJIQ’s default parameters, the new MAJIQ-lw was able to maintain high detection power with a moderate price in terms of reproducibility and correlation to RT-PCR, comparing favorably to other algorithms. Given that there is a natural trade-off between detection power and reproducibility, we recommend users decide between default and relaxed parameters based on their needs and dataset specifics.
Another contribution of this work is in developing a framework to tackle the challenge of evaluating DS algorithms based on both real and synthetic data. This work is definitely not the first to tackle this challenge, but most previous work focused on synthetic data using simplifying assumptions about binary AS events or transcript expression levels (Drewe et al., 2013; Entizne et al., 2017; Shen et al., 2014). Instead, we utilize biological replicates and create technical replicates by dividing the original FASTQ files. Using replicates we assess algorithms by using RR plots for DS events between different conditions, and by computing the IIR. The latter allows us to assess putative false positives with respect to DS between conditions and was in good qualitative agreement with FDR values we obtained from the synthetic dataset. We also use an extensive set of RT-PCR experiments from Vaquero-Garcia et al. (2016) to compute R2 correlation to the algorithms’
Although the new version of MAJIQ compared favorably to other algorithms in the experiments surveyed here, users should keep in mind that different datasets may suffer from other types of noise or biases than those tested here. It is therefore advisable for potential users to always test DS algorithms using the evaluation criteria introduced here, including reproducibility plots, same condition group comparisons, and RT-PCR. To aid such evaluations, we made all the scripts used for this work available at majiq.biociphers.org. Similarly, we also made all datasets, including the synthetic data, available for developers to test against.
Another important point to consider is that the methods tested here differ greatly in the set of features they offer. MAJIQ is the only one that offers the ability to detect complex splicing variations involving more than two alternative junctions, coupled with interactive visualization and genome browser connectivity. It is also capable of supplementing a given transcriptome annotation with reliable de novo junctions detected in the RNA-Seq data, making it less dependent on the exact annotation. Although useful for even normal tissues (Vaquero-Garcia et al., 2016), this feature is particularly relevant for disease studies, cases where uncharacteristic splicing is expected, and for species with poorly annotated transcriptomes. Although some software offers detection of de novo junctions between known splice sites, MAJIQ is able to detect completely novel exons and junctions.
Notably, MAJIQ’s detection of novel exons and junctions comes with a price of algorithmic complexity. MAJIQ first runs a builder which constructs de novo splice graphs per gene, then quantifies the detected LSVs in a second step. Importantly, the builder can be executed once and then used in multiple comparisons. This construction complicates comparison of time and memory to the other algorithms, making it highly context specific. For example, the analysis presented here involved 15 RNA-Seq samples, divided to groups three samples each, and a total of five pairwise comparisons. For this, MAJIQ used ~200 M of memory per node, making it suitable for running on anything from a laptop to an HPC. Running MAJIQ with 16 processes on a 32 Intel Xeon 2.4 GHz cores machine with 64GB of memory took about 3 h for the builder and another 40 m for the pairwise comparisons (each of which can be run in parallel). SUPPA and rMATS-turbo are significantly faster, taking a total of ~35 m (SUPPA) and 1 h (rMATS-turbo) with a similar amount of memory consumption. As for DEXSeq, we found it to be significantly slower and memory heavy, taking up to 1 GB of memory and over 6 h to finish. In addition, the fact DEXSeq does not report PSI or dPSI makes it less suitable for quantitative splicing focused research.
We note the algorithms surveyed here are by no means a complete list of DS methods and other algorithms may offer specific desirable features. For example, VAST-TOOLS (Irimia et al., 2014) offers sensitive detection of micro-exons, and DiffSplice (Hu et al., 2013) offers detection of AS modules in each gene’s splice graph.
There are several important directions in which this work can be extended. First, MAJIQ can be further improved both in terms of memory consumption and running time. More importantly, all the algorithms compared here were designed for datasets with small sets of biological replicates. Although we saw that outlier detection can work even for non biological replicates (see analysis of ρt using GTEx samples in Supplementary Figures) we note that large heterogeneous datasets, such as those created in cancer studies or GTEx (GTEx Consortium, 2013), are likely to benefit from different statistical models. Finally, MAJIQ’s improved quantifications can be used to subsequently derive new models for splicing codes and splicing predictions given genetic variations (Barash et al., 2010, 2013; Xiong et al., 2015). Such improvements form a promising path for future algorithm development.
Acknowledgements
We would like to thank an anonymous ISMB 2017 reviewer for helpful suggestions, as well as Matthew R. Gazzara and Anupama Jha from the BioCiphers Lab for helpful comments and suggestions.
Funding
This work has been supported in part by the Penn Institute for Biomedical Informatics Pilot [Grant R01 AG046544 to Y.B.].
Conflict of Interest: none declared.
References
- Alamancos G.P. et al. (2014). Methods to Study Splicing from High-Throughput RNA Sequencing Data, pp. 357–397. Humana Press, Totowa, NJ. [Abstract] [Google Scholar]
- Anders S. et al. (2012) Detecting differential usage of exons from rna-seq data. Genome Res., 22, 2008–2017. [Europe PMC free article] [Abstract] [Google Scholar]
- Barash Y. et al. (2010) Deciphering the splicing code. Nature, 465, 53–59. [Abstract] [Google Scholar]
- Barash Y. et al. (2013) AVISPA: a web tool for the prediction and analysis of alternative splicing. Genome Biol., 14, 1–8. [Europe PMC free article] [Abstract] [Google Scholar]
- Conesa A. et al. (2016) A survey of best practices for RNA-seq data analysis. Genome Biol., 17, 13.. [Europe PMC free article] [Abstract] [Google Scholar]
- Drewe P. et al. (2013) Accurate detection of differential RNA processing. Nucleic Acids Res., 41, 5189–5198. [Europe PMC free article] [Abstract] [Google Scholar]
- Entizne J.C. et al. (2017) Fast and accurate differential splicing analysis across multiple conditions with replicates. bioRxiv. 10.1101/086876. [Europe PMC free article] [Abstract] [Google Scholar]
- Grant G.R. et al. (2011) Comparative analysis of RNA-Seq alignment algorithms and the RNA-Seq unified mapper (RUM). Bioinformatics, 27, 2518–2528. [Europe PMC free article] [Abstract] [Google Scholar]
- GTEx Consortium (2013) The Genotype-Tissue Expression (GTEx) Project. Nat. Genet., 45, 580–585. 10.1038/ng.2653. [Europe PMC free article] [Abstract] [Google Scholar]
- Hu Y. et al. (2013) DiffSplice: the genome-wide detection of differential splicing events with RNA-seq. Nucleic Acids Res., 41, e39–e39. [Europe PMC free article] [Abstract] [Google Scholar]
- Irimia M. et al. (2014) A highly conserved program of neuronal microexons is misregulated in autistic brains. Cell, 159, 1511–1523. [Europe PMC free article] [Abstract] [Google Scholar]
- Keane T.M. et al. (2011) Mouse genomic variation and its effect on phenotypes and gene regulation. Nature, 477, 289–294. [Europe PMC free article] [Abstract] [Google Scholar]
- Li B., Dewey C.N. (2011) Rsem: accurate transcript quantification from rna-seq data with or without a reference genome. BMC Bioinformatics, 12, 323.. [Europe PMC free article] [Abstract] [Google Scholar]
- Li Q. et al. (2011) Measuring reproducibility of high-throughput experiments. Ann. Appl. Stat., 5, 1752–1779. [Google Scholar]
- Liu R. et al. (2014) Comparisons of computational methods for differential alternative splicing detection using rna-seq in plant systems. BMC Bioinform., 15, 364. [Europe PMC free article] [Abstract] [Google Scholar]
- Lonsdale J. et al. (2013) The genotype-tissue expression (GTEx) project. Nat. Genet., 45, 580–585. [Europe PMC free article] [Abstract] [Google Scholar]
- Pan Q. et al. (2008) Deep surveying of alternative splicing complexity in the human transcriptome by high-throughput sequencing. Nat. Genet., 40, 1413–1415. [Abstract] [Google Scholar]
- Patro R. et al. (2017) Salmon provides fast and bias-aware quantification of transcript expression. Nat. Methods, 14, 417–419. [Europe PMC free article] [Abstract] [Google Scholar]
- Shen S. et al. (2014) rmats: Robust and flexible detection of differential alternative splicing from replicate rna-seq data. Proceedings of the National Academy of Sciences, 111, E5593–E5601. [Europe PMC free article] [Abstract] [Google Scholar]
- Tokheim C.J. et al. (2016) Evaluating the evaluation of cancer driver genes. Proc. Natl. Acad. Sci. USA, 113, 14330–14335. [Europe PMC free article] [Abstract] [Google Scholar]
- Trapnell C. et al. (2012) Differential analysis of gene regulation at transcript resolution with RNA-seq. Nat. Biotech., 31, 46–53. [Europe PMC free article] [Abstract] [Google Scholar]
- Vaquero-Garcia J. et al. (2016) A new view of transcriptome complexity and regulation through the lens of local splicing variations. eLife, 5, e11752.. [Europe PMC free article] [Abstract] [Google Scholar]
- Wang E.T., Cooper A.T. (2007) Splicing in disease: disruption of the splicing code and the decoding machinery. Nature, 8, 749–761. [Abstract] [Google Scholar]
- Xiong H.Y. et al. (2015) The human splicing code reveals new insights into the genetic determinants of disease. Science, 347, 1254806.. [Europe PMC free article] [Abstract] [Google Scholar]
- Zhang R. et al. (2014) A circadian gene expression atlas in mammals: Implications for biology and medicine. Proc. Natl. Acad. Sci. USA, 111, 16219–16224. [Europe PMC free article] [Abstract] [Google Scholar]
Articles from Bioinformatics are provided here courtesy of Oxford University Press
Full text links
Read article at publisher's site: https://doi.org/10.1093/bioinformatics/btx790
Read article for free, from open access legal sources, via Unpaywall:
https://academic.oup.com/bioinformatics/article-pdf/34/9/1488/25417002/btx790.pdf
Citations & impact
Impact metrics
Article citations
Dysregulation of RNA splicing in early non-alcoholic fatty liver disease through hepatocellular carcinoma.
Sci Rep, 14(1):2500, 30 Jan 2024
Cited by: 4 articles | PMID: 38291075 | PMCID: PMC10828381
MAJIQlopedia: an encyclopedia of RNA splicing variations in human tissues and cancer.
Nucleic Acids Res, 52(d1):D213-D221, 01 Jan 2024
Cited by: 2 articles | PMID: 37953365 | PMCID: PMC10767883
LIS1 RNA-binding orchestrates the mechanosensitive properties of embryonic stem cells in AGO2-dependent and independent ways.
Nat Commun, 14(1):3293, 06 Jun 2023
Cited by: 0 articles | PMID: 37280197 | PMCID: PMC10244377
The problem of selection bias in studies of pre-mRNA splicing.
Nat Commun, 14(1):1966, 08 Apr 2023
Cited by: 2 articles | PMID: 37031238 | PMCID: PMC10082818
RNA splicing analysis using heterogeneous and large RNA-seq datasets.
Nat Commun, 14(1):1230, 03 Mar 2023
Cited by: 24 articles | PMID: 36869033 | PMCID: PMC9984406
Go to all (21) article citations
Data
Data behind the article
This data has been text mined from the article, or deposited into data resources.
BioStudies: supplemental material and supporting data
Similar Articles
To arrive at the top five similar articles we use a word-weighted algorithm to compare words from the Title and Abstract of each citation.
Integrative deep models for alternative splicing.
Bioinformatics, 33(14):i274-i282, 01 Jul 2017
Cited by: 37 articles | PMID: 28882000 | PMCID: PMC5870723
MAJIQ-SPEL: web-tool to interrogate classical and complex splicing variations from RNA-Seq data.
Bioinformatics, 34(2):300-302, 01 Jan 2018
Cited by: 12 articles | PMID: 28968636 | PMCID: PMC7263396
Inference of alternative splicing from RNA-Seq data with probabilistic splice graphs.
Bioinformatics, 29(18):2300-2310, 11 Jul 2013
Cited by: 13 articles | PMID: 23846746 | PMCID: PMC3753571
FDM: a graph-based statistical method to detect differential transcription using RNA-seq data.
Bioinformatics, 27(19):2633-2640, 08 Aug 2011
Cited by: 29 articles | PMID: 21824971 | PMCID: PMC3179659
Funding
Funders who supported this work.
NIA NIH HHS (1)
Grant ID: R01 AG046544
NIGMS NIH HHS (1)
Grant ID: R01 GM128096
Penn Institute for Biomedical Informatics Pilot (1)
Grant ID: R01 AG046544