Abstract
Free full text

Single-amino acid variants reveal evolutionary processes that shape the biogeography of a global SAR11 subclade
Abstract
Members of the SAR11 order Pelagibacterales dominate the surface oceans. Their extensive diversity challenges emerging operational boundaries defined for microbial 'species' and complicates efforts of population genetics to study their evolution. Here, we employed single-amino acid variants (SAAVs) to investigate ecological and evolutionary forces that maintain the genomic heterogeneity within ubiquitous SAR11 populations we accessed through metagenomic read recruitment using a single isolate genome. Integrating amino acid and protein biochemistry with metagenomics revealed that systematic purifying selection against deleterious variants governs non-synonymous variation among very closely related populations of SAR11. SAAVs partitioned metagenomes into two main groups matching large-scale oceanic current temperatures, and six finer proteotypes that connect distant oceanic regions. These findings suggest that environmentally-mediated selection plays a critical role in the journey of cosmopolitan surface ocean microbial populations, and the idea ‘everything is everywhere but the environment selects’ has credence even at the finest resolutions.
Introduction
The SAR11 order Pelagibacterales (Thrash et al., 2011; Ferla et al., 2013) is one of the most ubiquitous free-living lineages of heterotrophic bacteria in the world’s oceans (Giovannoni et al., 1990; Morris et al., 2002; Carlson et al., 2009; Eiler et al., 2009; Schattenhofer et al., 2009; Treusch et al., 2009). Successful cultivation efforts and single amplified genomes from the environment have led to studies revealing their critical role in marine carbon cycling (Rappé et al., 2002; Giovannoni et al., 2005; Stingl et al., 2007; Oh et al., 2011; Tsementzi et al., 2016; White et al., 2019), and environmental sequencing surveys have offered detailed insights into the ecology of this ancient branch of life in aquatic environments across the globe (Zinger et al., 2011; Brown et al., 2012).
The evolution of SAR11 is an active area of research (Giovannoni, 2017) that is critically important to understanding the determinants of its remarkable ability to maintain abundant populations in the global ocean. The evolutionary origins of SAR11 and thus its precise placement in the Tree of Life is debated (Thrash et al., 2011; Rodríguez-Ezpeleta and Embley, 2012; Ferla et al., 2013; Viklund et al., 2013), and our understanding of the evolutionary processes that define the biogeography of SAR11 cells is not complete. At the level of major SAR11 clades, previous studies have attributed markedly distinct patterns of distribution in the global ocean to both niche-based (Brown et al., 2012; Eren et al., 2013a) and neutral processes (Manrique and Jones, 2017). At the level of individual populations, a key simulation by Hellweger et al. (2014) showed that the intra-population sequence divergence that reflects the geographic patterns of distribution for SAR11 cells could emerge solely as a function of ocean currents, without selection (Hellweger et al., 2014). Between the extremes of inter-clade and intra-population diversity lies a wealth of variation that potentially can yield insights into the ecological and genetic forces that determine genomic diversity and fitness between closely-related, naturally occurring SAR11 populations.
High-throughput sequencing of metagenomes provides access to genome-wide heterogeneity within environmental populations (Simmons et al., 2008), and current computational strategies can reveal associations between ecological parameters and microdiversity patterns at various levels of resolution (Eren et al., 2015; Scholz et al., 2016; Nayfach et al., 2016; Costea et al., 2017; Truong et al., 2017). However, SAR11 poses multiple challenges for such investigations, including their remarkable intra-population genomic diversity and the limited success of reconstructing SAR11 genomes from metagenomic data. Comprehensive investigations of the genetic contents of naturally occurring microbial populations (see Denef, 2018) for a review) often rely on population genomes directly reconstructed from metagenomes (Simmons et al., 2008; Bendall et al., 2016; Anderson et al., 2017; Garcia et al., 2018). While advances in genome-resolved metagenomics have made microbial clades more accessible without cultivation (Spang et al., 2015; Brown et al., 2015; Anantharaman et al., 2016), reconstructing SAR11 genomes from the surface ocean remains a difficult endeavor, as evident in recent comprehensive surveys of metagenome-assembled genomes (MAGs) from seawater samples from around the globe (Tully et al., 2018; Delmont et al., 2018). In the absence of population genomes recovered directly from the environment, genomes from isolates can also offer insights into environmental populations through genome-wide recruitment analyses in which short metagenomic reads are aligned to a reference (Denef, 2018).
Using metagenomic read recruitment to investigate the structure of environmental populations is confounded by the challenge of defining the boundaries of microbial populations. Without an established species concept in microbiology, defining units of microbial diversity and their boundaries is a significant challenge (see Shapiro, 2018 and Cohan, 2019 for discussions). Nevertheless, from analyses of isolated microbial strains with formal taxonomic descriptions, a genome-wide average nucleotide identity (gANI) cutoff of 95% emerged as an operational delineation of species (Konstantinidis and Tiedje, 2005; Varghese et al., 2015) and was confirmed in a recent analysis of eight billion pairwise comparisons of whole genomes (Jain et al., 2018). Both gANI calculations using complete genomes, as well as the average nucleotide identity of metagenomic short reads (ANIr) recruited from environmental metagenomes using reference genomes, show an interesting discontinuity among sequence‐discrete populations at sequence identity levels between 80% and 90–95% (Konstantinidis and DeLong, 2008; Caro-Quintero and Konstantinidis, 2012; Jain et al., 2018). Regardless of their theoretical significance, these cutoffs are essential for multiple practical purposes, such as the identification and subsequent exclusion of metagenomic reads that originate from non-target environmental populations, to avoid inflating variants arising from contaminating non-specific reads in microbial population genetics studies.
Interestingly, the boundaries of environmental SAR11 populations appear to not comply with the 95% ANIr cutoff. For instance, Tsementzi et al. (2016) observed substantial sequence diversity within sequence-discrete SAR11 subclades in the environment, and suggested that an ANIr as low as 92% would be required to adequately define the boundaries of the SAR11 populations recovered in their study (Tsementzi et al., 2016). These findings are consistent with a comprehensive study of isolate genomes and marine metagenomes by Nayfach et al. (2016), which suggested that SAR11 is one of the most genetically heterogeneous marine microbial clades (Nayfach et al., 2016). The substantial sequence diversity within environmental SAR11 populations not only explains the absence of SAR11 population genomes in genome-resolved metagenomics studies, but also challenges conventional approaches to the study of population genetics in microorganisms. For instance, the multiple occurrence of single-nucleotide variants in individual codon positions would render commonly used computational strategies that classify synonymous and non-synonymous variations based on independent nucleotide sites (such as in Schloissnig et al., 2013; Bendall et al., 2016) unfeasible. Despite these challenges, SAR11, with its ubiquity in surface seawater samples, extensive diversity in sequence space, and unique evolutionary history, remains one of the exciting puzzles of contemporary microbiology.
Here we investigated the evolutionary processes that maintain genetic diversity within a natural SAR11 lineage accessible through a single isolate genome that recruited more than 1% of surface ocean metagenomic reads from a global dataset. Using single-amino acid variants, we were able to (1) delineate multiple proteotypes whose distributions were more closely linked to large-scale oceanic current temperatures than they were to geographic proximity, and (2) resolve positive and negative selection mediated by temperature and its co-variables. Our findings suggest that environmentally mediated selection, rather than neutral processes, dominate the biogeographic partitioning of SAR11 at fine scales of taxonomic resolution. Our study also offers new computational approaches to characterize variation within complex microbial populations, including additional means to integrate amino acid and protein biochemistry into microbial population genetics.
Results and discussion
To find the most appropriate SAR11 isolate genome to study the population genetics of naturally occurring SAR11, we used the complete genomes of 21 SAR11 isolates in a competitive recruitment of short reads from 103 metagenomes. Most of these metagenomes were from the TARA Oceans Project (Sunagawa et al., 2015), and correspond to 93 stations across four oceans and two seas. We also included an additional 10 metagenomes from the Ocean Sampling Day Project (Kopf et al., 2015) to cover high-latitude areas of the Northern hemisphere. All metagenomes correspond to small planktonic cells (0.2–3 μm in size) from the surface (0–15 meters depth; n = 71) and deep chlorophyll maximum (17–95 meters depth; n = 32) layers of the water column (Supplementary file 1a). The isolates we used belonged to SAR11 subclades Ia.1 (n = 6), Ia.3 (n = 11), II (n = 1), IIIa (n = 2) and the related alphaproteobacterium Va (n = 1) (Supplementary file 1b), which collectively recruited 1,029,716,339 reads from all metagenomes, or 3.3% of the dataset (Supplementary file 1c).
The metapangenome of SAR11
To investigate associations between ecology and gene content of SAR11 lineages, we first performed a pangenomic analysis in conjunction with read recruitment from the metagenomic data. The pangenome of SAR11 genomes consisted of all 29,719 genes grouped into 6175 gene clusters (Supplementary file 1d). The clustering of genomes based on shared gene clusters (Supplementary file 1e) matched that of the previously described phylogenetic clades (Grote et al., 2012) (Figure 1A; an interactive version of which is available at http://anvi-server.org/p/4Q2TNo). The SAR11 pangenome across metagenomes (i.e., the SAR11 metapangenome) revealed distinct distribution patterns for each clade within SAR11 (Figure 1A). Clade Ia recruited the most reads compared to other clades (Supplementary file 1b), consistent with previous studies that found this clade to be highly abundant in surface seawater (Field et al., 1997; Brown et al., 2012; Eren et al., 2013a; Manrique and Jones, 2017). Gene clusters divided clade Ia into two main clusters corresponding to the high-latitude subclade Ia.1 and the low-latitude subclade Ia.3 (Figure 1A). While all high-latitude genomes displayed a bi-polar geographic distribution in the metagenomic dataset, gene clusters in low-latitude genomes revealed multiple sub-groups that also showed different patterns of geographic distribution (Figure 1A). This emphasized the need to further refine subclade 1a.3, in which each genome pair had over 98.6% sequence identity at the 16S rRNA gene level (Supplementary file 1f). Our consideration of geographical co-occurrence patterns, phylogenomic characteristics, and pangenomic properties in this metapangenome revealed six subclades within 1a.3 with cultured representatives (Figure 1A, also see Supplementary file 1g for gANI estimates between SAR11 genomes). We tentatively name them SAR11 subclade 1a.3.I (HTCC7211, HTCC7214 and HTCC7217; gANI of >93% and 16S rRNA gene identity of >99.4%), 1a.3.II (HIMB5), 1a.3.III (HIMB4 and HIMB1321; gANI of 94.8% and 16S rRNA gene identity of 100%), 1a.3.IV (HTCC8051 and HTCC9022; gANI of 86.9% and 16S rRNA gene identity of 100%), 1a.3.V (HIMB83) and 1a.3.VI (HIMB122 and HIMB140; gANI of 94.6% and 16S rRNA gene identity of 99.7%). Overall, the refinement of SAR11 subclades reveals a striking agreement between phylogeny, pangenome, and the ecology of the members of the SAR11 clade Ia.
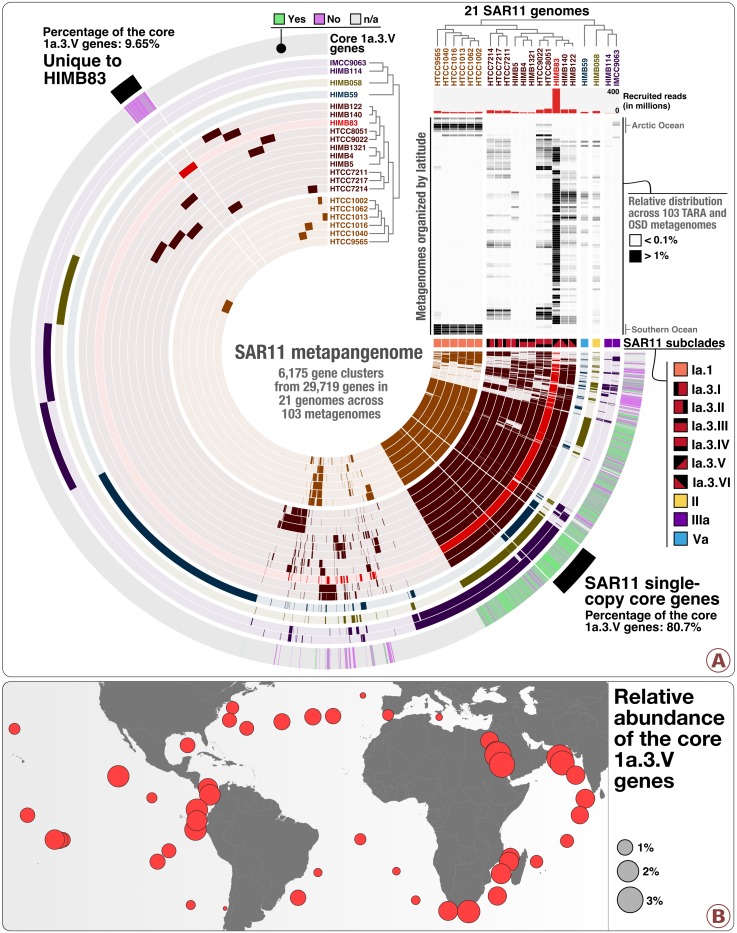
Panel A describes the pangenome of 21 SAR11 isolate genomes based on the occurrence of 6175 gene clusters, in conjunction with their phylogeny (clade level) and relative distribution of recruited reads in 103 metagenomes ordered by latitude from the North Pole to the South Pole (top right heat map). The relative distributions were displayed for a minimum value of 0.1% and a maximum value of 1%. The layer named ‘Core 1a.3.V genes’ displays the occurrence of the 799 core 1a.3.V genes (in green) and those found in HIMB83 but not in the 1a.3.V lineage (in purple). Panel B describes the relative distribution of reads the 799 core 1a.3.V genes recruited across surface metagenomes from TARA Oceans.
Figure 1—figure supplement 1.
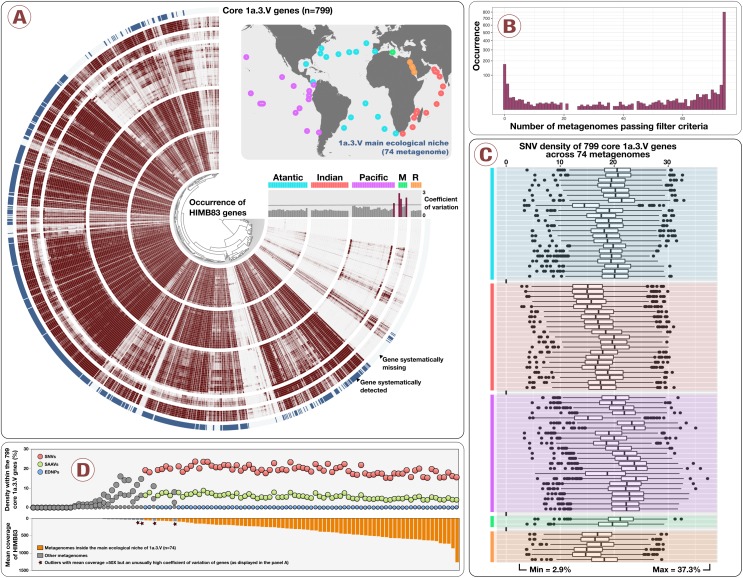
V genes. Panel A displays the relative distribution of HIMB83 genes across 78 metagenomes, along with their coefficient of variation and the selection of 799 core 1a.3.V genes (blue outer circle). A world map provides the location of 74 metagenomes corresponding to the main ecological niche of 1a.3.V metagenomes (four metagenomes were disregarded due to high coefficients of variation). Panel B shows the number of metagenomes in which genes were consistently present. Genes were considered to be present in a metagenome consistently only if their coverage was within a factor of 5 of the average coverage of all genes for that metagenome. Those that passed the filter criteria in all 74 metagenomes (far right) were defined as the core 1a.3.V genes. Panel C displays the SNV density of core 1a.3.V genes across these 74 metagenomes. SNV density varied between 2.9% and 37.3% across genes and metagenomes. Panel D summarizes the heterogeneity extent of the core 1a.3.V genes within the population main ecological niche. Specifically, the panel displays the density of single nucleotide variants (>1% from consensus), environmentally disconnected nucleotide position (i.e., positions stable in the environment but differing from the reference,<1% from consensus) and single amino acid variants (>10% from consensus) within the core genes of 1a.3.V across 103 metagenomes as a function of the mean coverage of HIMB83.
A remarkably abundant and widespread SAR11 lineage at low latitudes
While Ia.3 was the most abundant SAR11 subclade in our dataset, the new subclades we defined in this group differed remarkably in their competitive recruitment of short reads from metagenomes (Figure 1A, Supplementary file 1b). For example, while the least abundant subclade (1a.3.II; represented by HIMB5) recruited 22.6 million reads, the most abundant one (1a.3.V; represented by HIMB83), recruited 390.9 million reads, or 1.18% of the entire metagenomic dataset (Supplementary file 1b). For perspective, this is roughly two times more reads than the most abundant Prochlorococcus isolate genome recruited from the same dataset (Delmont and Eren, 2018) (Supplementary file 1h). Strain HIMB83 contains a 1.4 Mbp genome with 1470 genes, and was isolated from coastal seawaters off Hawai’i, USA. But it also recruited large numbers of reads from locations that were distant to the source of isolation (Supplementary file 1c). The gANI between HIMB83 and the most similar genome in our dataset, HIMB122 (1a.3.VI) was 82.6%, and the remarkable abundance of HIMB83 has also been recognized by others (Brucks, 2014; Nayfach et al., 2016). To the best of our knowledge, 1a.3.V is the most abundant and widespread SAR11 subclade in the euphotic zone of low-latitude oceans and seas.
Although it is a member of the subclade 1a.3.V, the genomic context HIMB83 provides does not exhaustively describe the gene content of all members of 1a.3.V. Nevertheless, it gives access to the core 1a.3.V genes through read recruitment. To identify core 1a.3.V genes, we used a conservative two-step filtering approach. First, we defined a subset of the 103 metagenomes within the main ecological niche of 1a.3.V using genomic mean coverage values (Supplementary file 1c). Our selection of 74 metagenomes in which the mean coverage of HIMB83 was >50X encompassed three oceans and two seas between −35.2° and +43.7° latitude, and water temperatures at the time of sampling between 14.1°C and 30.5°C (Figure 1—figure supplement 1, Supplementary file 1i). We then defined a subset of HIMB83 genes as the core 1a.3.V genes if they occurred in all 74 metagenomes and their mean coverage in each metagenome remained within a factor of 5 of the mean coverage of all HIMB83 genes in the same metagenome. This criterion accounted for biological characteristics influencing coverage values in metagenomic surveys of the surface ocean such as cell division rates and variations in coverage as a function of changes in GC-content throughout the genomic context. Figure 1—figure supplement 1 displays the coverage of all HIMB83 genes across all metagenomes, and Supplementary file 1j reports underlying coverage statistics. While the 799 genes that met these criteria systematically occurred within the niche boundaries of 1a.3.V, 40% of the remaining 671 HIMB83 genes that were filtered out were present in five or fewer metagenomes and coincided with hypervariable genomic loci (Figure 1—figure supplement 1). Hypervariable genome regions are common features of surface ocean microbes (Coleman et al., 2006; Zaremba-Niedzwiedzka et al., 2013; Kashtan et al., 2014; Delmont and Eren, 2018) that are not readily addressed through metagenomic read recruitment but do influence pangenomic trends. Here, less than 10% of gene clusters unique to HIMB83 were among core 1a.3.V genes (Figure 1A), indicating HIMB83’s unique genes are mostly accessory to the members of 1a.3.V. In contrast, more than 80% of gene clusters that were core to the 21 SAR11 genomes matched to the core 1a.3.V genes. The overlap between environmental core genes of 1a.3.V revealed by the metagenomic read recruitment and the genomic core of SAR11 revealed by the pangenomic analysis of isolate genomes suggests that these genes represent a large fraction of the 1a.3.V genomic backbone (Figure 1A). Core 1a.3.V genes recruited on average 1.25% of reads in the 74 metagenomes (Figure 1B, Supplementary file 1j). The broad geographic prevalence of core 1a.3.V genes represents a unique opportunity to study the population genetics of an abundant marine microbial subclade across distant geographies.
SAR11 subclade 1a.3.V maintains a substantial amount of genomic heterogeneity
To investigate the amount of genomic heterogeneity within 1a.3.V, we first studied individual short reads that the HIMB83 genome recruited from metagenomes. The percent identity of reads that matched to the 799 core 1a.3.V genes ranged from 88% to 100% (Figure 2), which is considerably more diverse than those observed in similar reference-based metagenomic studies (Konstantinidis and DeLong, 2008; Tsementzi et al., 2016; Meziti et al., 2019). Notably, we also observed similar trends for the other SAR11 genomes included in this study (Figure 2—figure supplement 1), suggesting that the relatively high sequence diversity observed among core 1a.3.V genes may be a characteristic shared with other SAR11 lineages in the surface ocean.
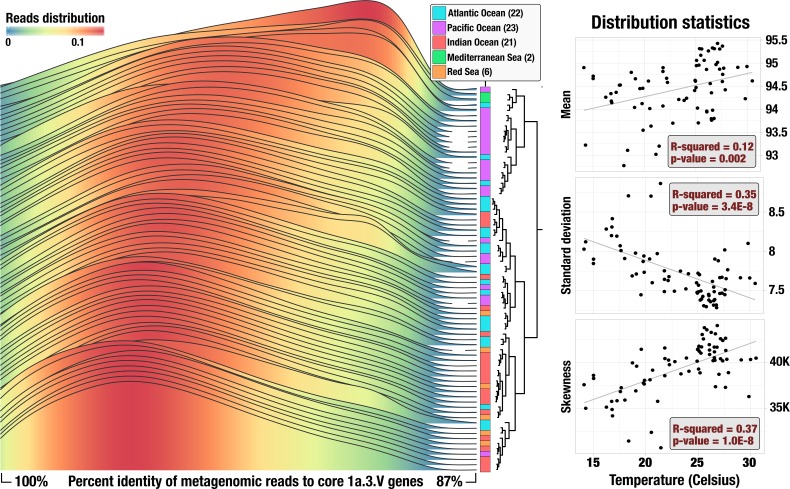
Left panel shows percent identity distributions in each of the 74 metagenomes. Curves are colored based on height. Metagenomes are ordered according to how the percent identity distributions hierarchically cluster based on Euclidean distance (dendrogram). Right panels display a summary of distribution statistics for each percent identity distribution compared against in situ temperature in a linear regression (correlations to all other available parameters are summarized in Figure 2—figure supplement 2). Each point is a metagenome and black lines are lines of best fit. For visual clarity, the data in left panel considers only the median read length and interpolates between data points, whereas the data in right panels consider all read lengths with no interpolation.
Figure 2—figure supplement 1.
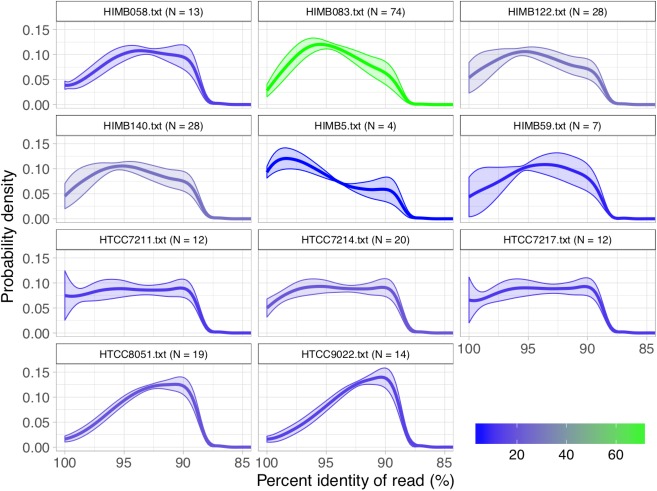
For each reference genome, metagenomes were only included if they recruited at least 50X coverage. 10 references failed to recruit 50X coverage in any metagenome and were excluded from the plot. Curves were colored according to
Figure 2—figure supplement 2.

For example, cells in the temperature column of this matrix quantify the linear correlation coefficients of the scatterplots shown in Figure 2B. Cell colors correspond to their linear correlation coefficient and sizes are proportional to R-squared values. We did not correct for multiple testing due to the potential for strong inter-dependence of the parameters. Supplementary file 1k reports full numerical statistics including p-values.
Overall, our data confirm that ANIr values of >95% used previously to delineate sequence-discrete populations does not apply to SAR11. One immediate implication of this substantial amount of sequence diversity that defies previous empirical observations is our inability to explicitly define what we are accessing in the environment. This challenge is partially because a precise and exhaustive description of what constitutes a ‘population’ remains elusive (Cohan and Perry, 2007; Shapiro and Polz, 2015; Cohan, 2019), which creates significant practical challenges (Rocha, 2018), such as the accurate determination of the boundaries of naturally occurring microbial populations especially in metagenomic read recruitment results. Nevertheless, the term ‘population’ is frequently used in literature (Simmons et al., 2008; Kashtan et al., 2014; Bendall et al., 2016), which implies that Charles Darwin’s observation in his historical work ‘On the Origin of Species’ continues to summarize our struggle in life sciences to describe theoretical boundaries of fundamental units of life even though contemporary enviornmental microbiology has gone beyond the term species in this pursuit: 'no one definition of species has yet satisfied all naturalists; yet every naturalist knows vaguely what [they mean] when [they speak] of a species’ (Darwin, 1859). Our study is not well-positioned to offer a precise theoretical definition for the term 'population', either. Instead, similar to previous studies, we resort to an operational definition that suggests a population is 'an agglomerate of naturally occurring microbial cells, genomes of which are similar enough to align to the same genomic reference with high sequence identity’ (Delmont and Eren, 2018; also see Denef, 2018 and references therein for a comprehensive discussion of what constitutes a population from a metagenomic perspective). By outsourcing the hypothetical radius of a population in sequence space to the minimum sequence identity of short reads recruited from metagenomes, this approach offers a practical means to study very closely related environmental sequences without invoking theoretical considerations. The broad heterogeneity continuum that possesses no discernible sequence-discrete components we observed within the narrow sequence set defined this way, i.e., the metagenomic reads that match competitively to conserved HIMB83 genes (Figure 2), supports the assumption that this set originates within a population boundary (Figure 1—figure supplement 1). However, due to the incomplete theoretical foundation and limitations associated with the use of short metagenomic reads, in discussions here we more conservatively assume that our reads originate from multiple closely related yet intertwined SAR11 populations within subclade 1a.3.V.
Both high recombination rates between cells displaying low gANI values and frequent transfer of adaptive genes between ecologically distinct clades could explain the high-level of cohesion between SAR11 populations in the surface ocean (Cohan, 2019; Vergin et al., 2007). The high density of closely related 1a.3.V cells in the surface ocean suggests the strength of these two forces could be high within populations as well. At least two hypotheses reconcile extensive SAR11 sequence diversity and aide in understanding its implications. One hypothesis is that the members of 1a.3.V we access are in the process of evolving into multiple sequence-discrete populations and we are simply observing an emerging fork in the evolutionary journey of SAR11. Alternatively, the observed diversity may represent a cloud of random sequence variants akin to a quasispecies (Domingo et al., 2012). To examine these hypotheses, we tested the correlation between basic statistical properties of these curves (i.e., mean, standard deviation, and skewness) and environmental parameters via linear regression (Figure 2—figure supplement 2, Supplementary file 1k). This analysis revealed a significant correlation between in situ temperature and distribution shape (mean p-value:
SAAVs: Accurate characterization of non-synonymous variation
Percent identity distributions are useful to assess overall alignment statistics of short reads to a reference; however, they do not convey information regarding allele frequencies, their functional significance, or association with biogeography. To bridge this gap, we implemented a framework to characterize amino acid substitutions in metagenomic data and to study genomic variation that impacts amino acid sequences (see Materials and methods). Briefly, our approach employs only metagenomic short reads that cover all three nucleotides in a given codon to determine the frequency of single-amino acid variants (SAAVs) in translated protein sequences. While synonymity is a codon characteristic, in practice it is often determined from a single-nucleotide variant (SNV) with the assumption that the two remaining nucleotides are invariant. However, populations with extensive nucleotide variation can violate this assumption. Indeed, in the case of the core 1a.3.V genes, on average 22.5% of SNVs per metagenome co-occurred with other SNVs in the same codon. Thus, quantifying frequencies of full codon sequences as implemented in the SAAV workflow is a requirement to correctly assess synonymity.
Among the 799 core 1a.3.V genes and 74 metagenomes, we identified 1,074,096 SAAVs in which >10% of amino acids diverged from the consensus (i.e., the most frequent amino acid for a given codon position and metagenome). The SAAV density (the percentage of codon positions that harbor a SAAV) of core 1a.3.V genes averaged 5.76% and correlated with SNV density (19.3% on average) across the 74 metagenomes (linear regression, p-value
Hydrophobicity influences the strength of purifying selection acting on amino acids
To understand how commonly each amino acid was found in variant sites, we compared the amino acid composition of SAAVs to the amino acid composition of the core 1a.3.V genes (see Materials and methods). In a scenario in which amino acids are as common in SAAVs as they are across all 799 core genes, the frequency that an amino acid occurred in SAAVs (variant sites) would share one-to-one correspondence with its frequency within the core genes (all sites). While these variables were correlated (linear regression, p-value:
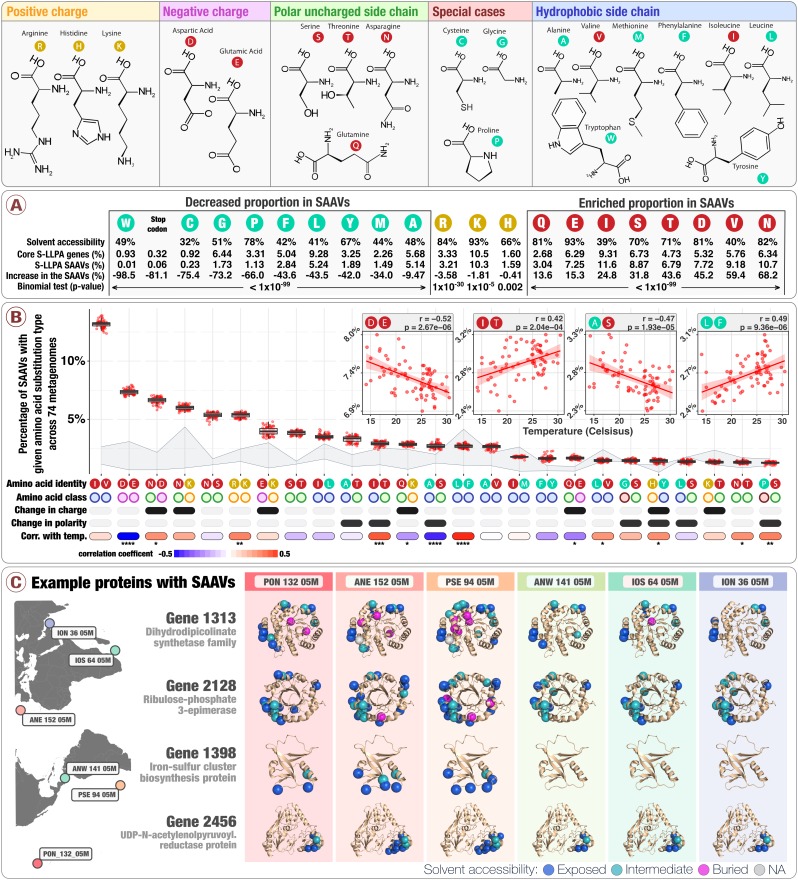
The top panel describes the structure of 20 amino acids grouped by their main chemical properties. Panel A describes the solvent accessibility of amino acids, their relative distribution in both the core 1a.3.V genes and SAAVs, and their percentage increase in SAAVs as compared to the core 1a.3.V genes. The solvent accessibility of amino acids derives from the analysis of 55 proteins (Bordo and Argos, 1991). Panel B describes the relative abundance of the top 25 most prevalent amino acid substitution types (AASTs) across 74 metagenomes (boxplots), along with the classes their amino acids belong to and the correlation coefficient between AAST prevalence and in situ temperature calculated via linear regression (see Figure 3—figure supplement 2 for p-values). The area shaded in light gray shows bounds for the expected frequency distribution given strictly neutral processes. The upper bound is Model one and the lower bound is Model 2 (see Materials and methods). The four insets example the relationship between AAST prevalence and in situ temperature for the AASTs 'aspartic/glutamic acid', 'isoleucine/threonine', 'alanine/serine', and 'leucine/phenylalanine' (Figure 3—figure supplement 2 illustrate similar plots for all 25 of the most prevalent AASTs). The 25 AASTs included in the analysis cover 87.1% of all SAAVs. Panel C displays SAAVs on the predicted protein structures of four core 1a.3.V genes across six metagenomes from distant locations.
Figure 3—figure supplement 1.
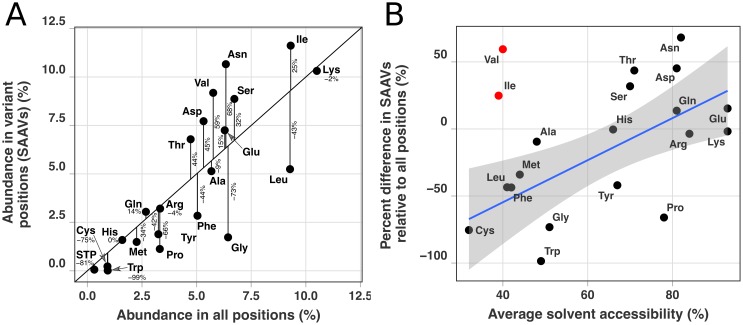
The amino acid composition of all positions (y-axis) was quantified by calculating the frequency that amino acids appeared within the core 1a.3.V genes of the reference sequence, HIMB83, whereas amino acid composition in SAAVs (x-axis) was quantified by calculating the frequency that an amino acid was one of the two dominant alleles across the 738,324 SAAVs. The black diagonal line signifies a one-to-one correspondence between these two variables, and black vertical lines illustrate the deviation of amino acids from this null expectation. Vertical lines are labeled with percent differences between frequencies of amino acids in SAAVs relative to all positions. The linear correlation coefficient of panel A is 0.81, with an R-squared of 0.65, and a probability that no correlation exists of
Figure 3—figure supplement 2.
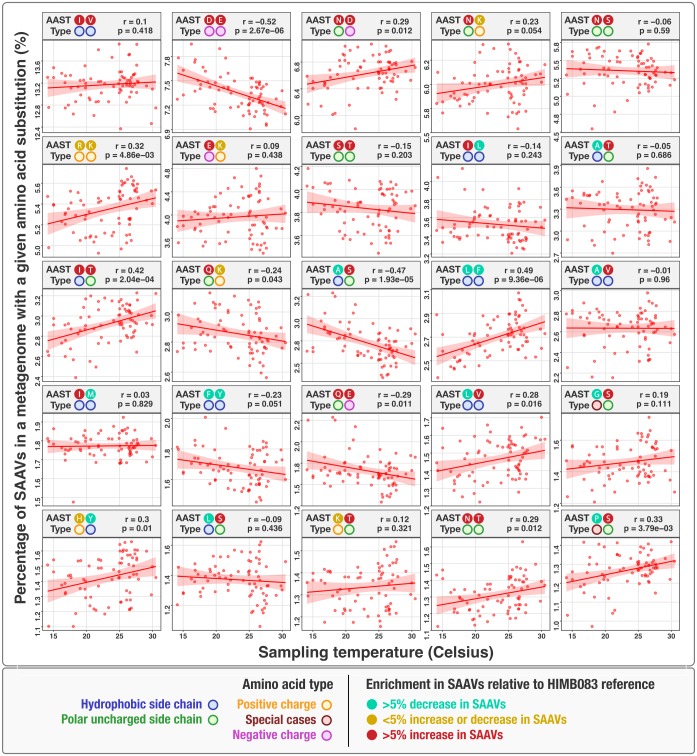
Each dot represents a metagenome, the x-axis is in situ temperature, and the y-axis is the percentage of SAAVs that were a given AAST. The red line is the line of best fit and the shaded-in region illustrates the 95% confidence interval. The linear correlation coefficient is given as
Figure 3—figure supplement 3.
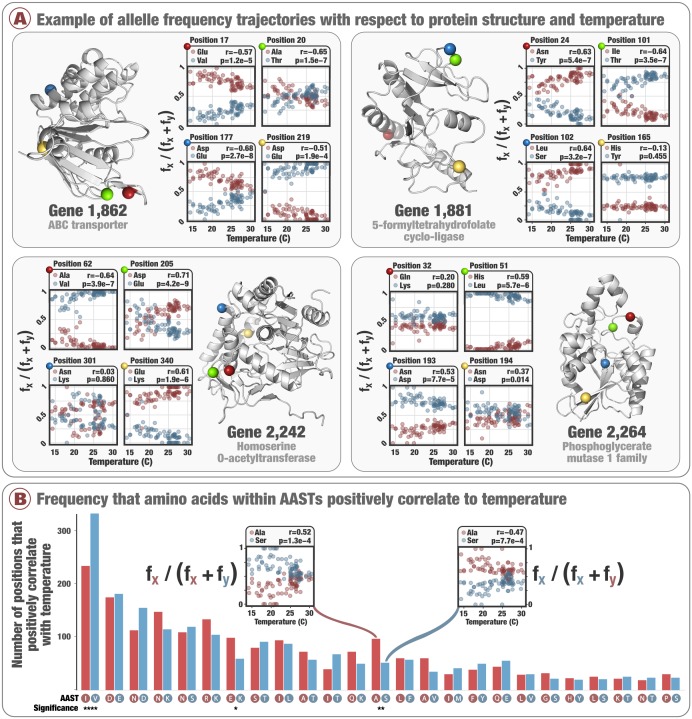
Panel A illustrates allele frequency trajectories across 74 metagenomes with respect to temperature for 16 manually chosen SAAV positions in the context of protein structures predicted from the core 1a.3.V genes. Only the two most abundant amino acids were considered for each SAAV. The linear correlation coefficient is denoted as
Figure 3—figure supplement 4.

Panel A illustrates the membrane topology predicted by Phobius (See Materials and methods), which associates to each position a probability it is periplasmic, cytosolic, transmembrane, or within the signaling peptide (colored lines). We categorized each position according to the maximum probability (shaded regions). Temperature-correlated and uncorrelated variant positions are denoted by solid red and dashed red vertical lines, respectively. Panel B illustrates the frequency that variant positions are found in the membrane, cytosol, and periplasm, based on whether or not they correlate with temperature. The y-axis is the fraction of variant positions observed (excluding positions observed in the signaling peptide), and numbers within each bar denote the number of variant positions observed within each class. The probability that positions are distributed independent of temperature correlation was 0.034. Formally, this is the probability that temperature-correlated positions were distributed according to a trinomial distribution with a probability vector equal to the empirical distribution observed in temperature-uncorrelated positions.
Hydrophobic interactions within the solvent inaccessible core of proteins are known to be critical for maintaining the stability required for folding and activity, which enforces a strong purifying selection placed on mutations occurring in buried (solvent inaccessible) positions (Bustamante et al., 2000; Chen and Zhou, 2005; Worth et al., 2009). Since hydrophobic amino acids form the majority of buried positions, they are on average under stronger purifying selection, which is the likely explanation for the underrepresentation of hydrophobic amino acids within SAAVs. On the other hand, mutations in exposed (solvent accessible) positions on the surface of proteins are tolerated more, as they are less likely to disrupt protein architecture. Overall, our compositional analysis revealed that the occurrence of amino acids in SAAVs is roughly correlate with the occurrence of amino acids within the core 1a.3.V genes, and that deviations from this expectation are driven in part by levels of purifying selection that depend upon the suitability of an amino acid’s hydrophobicity for a given physicochemical environment (Figure 3—figure supplement 1).
Amino acid exchange rates reveal hallmarks of neutral, purifying, and adaptive evolution
Next, we sought to investigate amino acids that co-occur in variable sites. SAAVs were often dominated by a few amino acids; hence, the frequency vector for a given SAAV contained many zero values. To reduce sparsity, we first simplified our data by associating each SAAV with an amino acid substitution type (AAST), defined as the two most frequent amino acids in a given SAAV. In 738,324 SAAVs, we observed 182 of 210 theoretically possible unique AASTs and a highly skewed AAST frequency distribution (Supplementary file 2g, Figure 3B boxplots). For example, the two most frequent AASTs, ‘isoleucine/valine’ and ‘aspartic/glutamic acid’, together comprised 20% of all SAAVs (Figure 3B). This is not surprising, since the amino acids in both of these AASTs (1) are common in the genome, (2) share very similar chemical structure (both differing by only a single methylene bridge), and (3) can be substituted through a single nucleotide substitution. On the other hand, the ‘glycine/tryptophan’ pair represents an opposite example: these amino acids (1) are uncommon in the genome, (2) share no chemical or structural similarity to one another, and (3) can only be substituted through a triple nucleotide substitution. Expectedly, ‘glycine/tryptophan’ was exceedingly rare in our data and occurred only once in 738,324 SAAVs (Supplementary file 2h).
While such a skewed AAST frequency distribution cannot be explained by strictly random mutational process (Figure 3B light-gray shaded area), it is compatible with standard theories of neutral or nearly-neutral evolution, since such theories consider the role of purifying selection (Ohta and Gillespie, 1996). Within subclade 1a.3.V the distribution of AAST frequencies was notably constrained across geographies (Figure 3B). For example, the relative standard deviation of ‘aspartic/glutamic acid’ frequencies across the 74 metagenomes was just 3.0%, and the statistical spread of other AASTs was comparable (Figure 3B). The overall consistency of AAST frequency distributions across geographies supports the hypothesis that purifying selection controls the permissibility of amino acid exchangeability within 1a.3.V and enables an interpretation of these data through a neutral model: SAAVs composing the AAST frequency distribution represent primarily neutral mutations that have drifted to measurable levels, and the lack of SAAVs in AASTs of dissimilar amino acids that likely represent deleterious mutations reflect the influence of purifying selection. However, a closer inspection reveals a subtle divergence of amino acid exchangeabilities that correlates with water temperature and/or its co-variables (Figure 3B insets, Figure 3—figure supplement 2). Note that this divergence is AAST specific; for example, positions with mixed proportions of glutamic and aspartic acid are less commonly found in warm waters (linear regression, uncorrected p-value:
To address this shortcoming, we next sought to link SAAVs to predicted protein structures of the core 1a.3.V genes, 436 of which had significant matches in Protein Data Bank for template-based structure modeling (see Materials and methods). Placing SAAVs on predicted protein structures revealed that their occurrence was not randomly distributed but was instead strongly dependent on the local physicochemical environment of the structure (Figure 3C, Supplementary file 3a and http://data.merenlab.org/sar11-saavs). Within the subset of the 1a.3.V proteome accessible to us, we found that buried amino acids (0-10% relative solvent accessibility) were approximately 4.4 times less likely to be variant than those that were exposed (41-100% relative solvent accessibility) (ANOVA, p-value:
Temperature correlates with amino acid allele frequency trajectories
In addition to considering patterns of variability that emerged when we pooled data across 37,416 codon positions exhibiting variation within the core 1a.3.V genes, we also investigated the allele frequency trajectories of individual positions (i.e., the relative frequency between the two most prevalent amino acids across the 74 metagenomes) and sought to identify those that correlate with in situ temperature and/or its co-variables. Amino acid allele frequencies in 4592 of the 37,416 positions were correlated with temperature (Supplementary file 3b; Benjamini–Hochberg multiple testing correction on linear regression p-values, false discovery rate 5%). Figure 3—figure supplement 3 illustrates example cases and correlation statistics per AAST. It is statistically implausible that such correlations with temperature could have arisen from neutral evolution, given that distant oceans share similar temperatures (Supplementary file 1a). It is therefore most plausible to conclude that these allele frequency trajectories are the result of environmentally mediated selection. Although we note that, considering the pervasive effect of genetic hitchhiking in microbial evolution (Good et al., 2017), variation in a considerable fraction of positions may be neutral despite their association with temperature.
We then sought to investigate which positions are under selection, and whether the variation at these positions can be explained by differing levels of purifying selection, or diversifying selection that could be evidence of adaptive evolution. Scrutinizing all 4592 positions to address these critical questions is an intractable problem, so we narrowed our focus to genes possessing disproportionately high ratios of temperature-correlated to temperature-uncorrelated SAAV positions, since we expected this to be a reasonable criterion for identifying likely candidates of adaptive evolution (Supplementary file 3c). Of the 10 genes fitting this criterion (see Materials and methods), the permease subunit of a glycine betaine ATP-binding cassette (ABC) transporter stood out due to its appreciated relevance to SAR11 biology: glycine betaine transporters of SAR11 are highly translated proteins in the environment and transport osmolyte compounds into cells for energy production (Noell and Giovannoni, 2019). To investigate the positioning of amino acids in the tertiary structure of the permease relative to the cellular membrane, we first categorized the location of each residue as transmembrane, cytosolic (inside the inner membrane), or periplasmic (outside the inner membrane) (Figure 3—figure supplement 4). Positions that were not correlated with temperature were commonly transmembrane, and infrequently periplasmic. In contrast, most positions that correlated with temperature were periplasmic (Figure 3—figure supplement 4). The probability of observing a similar distribution between temperature-correlated and temperature-uncorrelated positions across transmembrane, periplasmic, and cytosolic regions was only 0.034 (analytic trinomial test, temperature-uncorrelated distribution as prior), which indicates temperature-correlated positions are subjected to unique evolutionary forces. A previous study suggested that periplasmic residues of transmembrane proteins undergo higher rates of adaptive evolution due to their increased exposure to changing environmental conditions (Sojo et al., 2016). This observation lends additional support to the hypothesis that periplasmic SAAV positions within this gene that correlate with temperature are more likely shaped by adaptive processes.
Allele frequency trajectories also provide an opportunity to study the directionality of exchange rates of AASTs. For example, of the 1066 positions dominated by 'alanine/serine' SAAVs, 158 positions correlated with temperature (Figure 3—figure supplement 3). If there was no temperature-driven preference for either amino acid in this subset of positions, the frequency of alanine should positively correlate with temperature as often as the frequency of serine does. Yet this expectation is grossly violated: in 103 of 158 positions alanine frequencies positively correlated with temperature (binomial test, Bonferroni-corrected p-value: 0.004). Overall, this result indicates temperature-dependent amino acid substitution preferences that are independent of site (Figure 3—figure supplement 3).
SAAV partitioning between warm and cold currents
We finally sought to extend the concept of allele frequency tracking at individual SAAV positions to investigate large-scale geographic partitioning of metagenomes. For this, we simplified the 738,324 SAAVs into a presence-absence matrix for codon position-specific AASTs across 74 metagenomes (Supplementary file 2i, also see ‘Recovering codon position-specific AASTs from SAAVs’ in Materials and methods). Of 57,277 codon position-specific AASTs affiliated with 37,415 unique codon positions, we detected 1.94% in all 74 metagenomes, while 33.3% were found in single metagenomes (Supplementary file 2i). To estimate distances between metagenomes based on these data, we used a Deep Learning approach. Briefly, this approach relies on a graph-based activity regularization technique for competitive learning from hyper-dimensional data, modified to reveal latent groups of variants in a fully unsupervised manner through frequent random sampling of variants (Kilinc and Uysal, 2017). Hierarchical clustering of samples based on Deep Learning-estimated distances (Supplementary file 4a) resulted in two main groups: the Western (warm) and Eastern (cold) boundary currents (Figure 4A). High latitude, relatively cold, and relatively nutrient rich waters are the source of Eastern boundary currents, which warm up and typically decline in nutrients as they transit in an equatorial direction. The opposite is true of Western boundary currents, which move poleward. The first group of 41 metagenomes, which matched cold currents (Benguela, Canary, California and Peru), encompassed most metagenomes from the Eastern Pacific Ocean, as well as the East side of the Atlantic Ocean (except near the southern tip of Africa) and the Mediterranean Sea (Figure 4B). The second group of 33 metagenomes, which matched warm currents (Agulhas, Somali, Mozambique, Brazil and Gulf stream), encompassed all metagenomes from the Red Sea and Indian Ocean, as well as metagenomes from the West side of the Atlantic Ocean (Figure 4B). Samples collected from the deep chlorophyll maximum layer of the water column mirrored trends observed in the surface samples (Figure 4—figure supplement 1). The association between SAAVs and ocean current type revealed a strong, global signal at the amino acid-level for 1a.3.V and suggested the presence of two main ecological niches for this lineage. Warm and cold currents are dynamic environments that differ in a host of factors in addition to the latitude and temperature of source waters. Factors that could drive adaptive changes in amino acid sequences between warm and cold currents include major differences in phytoplankton communities, altered composition of dissolved organic carbon pools, and the water temperature itself. Interestingly, the niche defined by cold currents exhibited significantly more SAAVs (ANOVA, p-value:
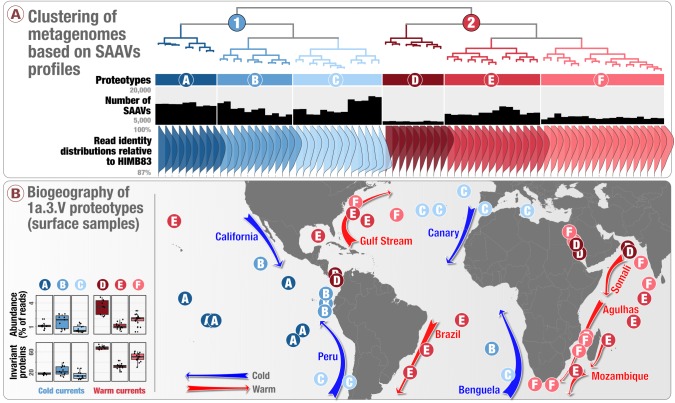
Panel A describes the organization of 74 metagenomes based on 57,277 codon position-specific AASTs affiliated with 37,415 unique codon positions and summarizes the number of detected SAAVs and percent identity of reads HIMB83 recruited for each metagenome. The world map in panel B displays the geographic partitioning of the two main metagenomic groups and six proteotypes. Panel B also describes the relative abundance of 1a.3.V and the number of invariant proteins across the six proteotypes.
Figure 4—figure supplement 1.
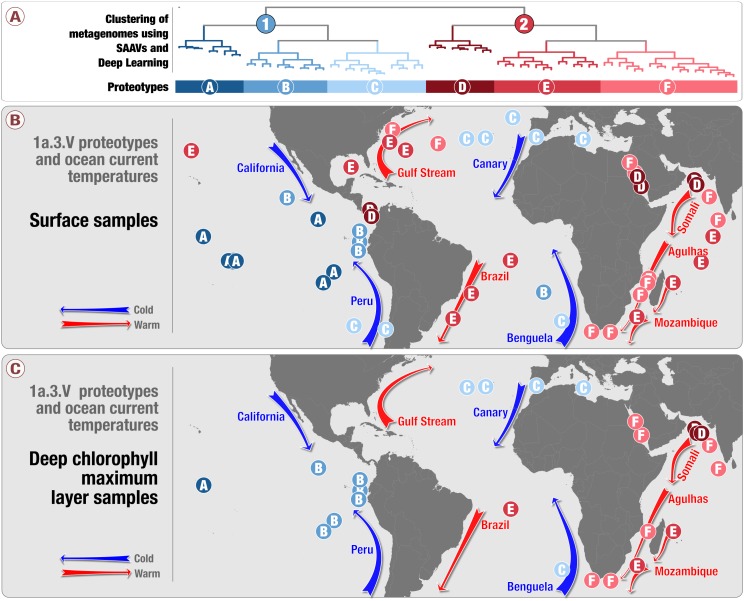
V groups and proteotypes between metagenomes sampled from the surface versus those sampled from the deep maximal chlorophyll layer. Panels A and B contain the same information as shown in Figure 4, and panel C shows the locations of deep maximal chlorophyll layer metagenomes and the proteotypes to which they belong.
Figure 4—figure supplement 2.

The elbow of this curve is k = 6, which informed our decision to define six proteotypes.
Figure 4—figure supplement 3.

V genes. Above, the metagenomes are organized by applying a novel graph-based activity regularization technique for competitive learning from hyper-dimensional data to the 738,324 SAAVs (see Materials and methods). Below, the metagenomes are organized by a less comprehensive approach in which the percent identity distributions are hierarchically clustered via Euclidean distance. The first method therefore is sensitive to the identity and positions of amino acid variability, whereas the second method is based solely on a summary statistic that quantifies the degree of sequence divergence at the DNA level and is agnostic to position and identity. Each metagenome is connected to itself through a straight line and is colored according to the proteotype to which it belongs.
Figure 4—figure supplement 4.
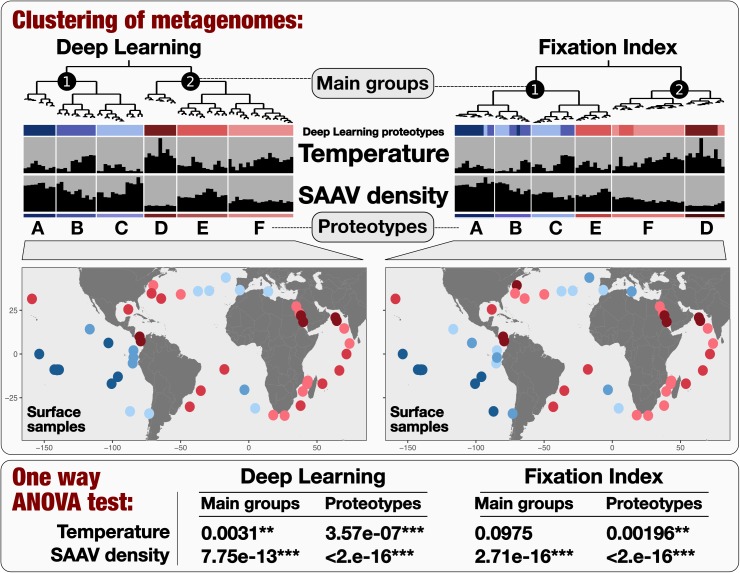
V based on single amino acid variants using Deep Learning (left) and Fixation Index (right) (see Material and methods). The world maps display the geographic partitioning of six proteotypes based on the two methodologies. Finally, ANOVA tests determine whether there are statistically significant differences between the means of either in situ temperature or SAAV density across (1) the two main groups and (2) the six proteotypes as inferred from the two methodologies.
Figure 4—figure supplement 5.

The figure emphasizes biogeographic differences between this simulation focused on neutral evolution and our large-scale empirical analysis.
To explore more detailed trends of the relationships between metagenomes, we further divided our dendrogram into six sub-clusters based on the elbow of the intra-cluster sum-of-squares curve of k-means clusters (Figure 4—figure supplement 2). These 1a.3.V ‘proteotypes’ grouped samples with similar amino acid variations (Figure 4A) and could not have been predicted from the clustering of samples based on percent identity distributions of short reads alone Figure 4—figure supplement 3). Among the environmental measurements for each metagenome (Supplementary file 4b, and 4c), latitude and temperature at the time of sampling were the most significant predictors of the proteotypes (ANOVA, p-values: 8.56 × 1013 and 3.57 × 10-7, respectively).These two variables were followed by the concentrations of nitrate, phosphate, oxygen, and to a lesser extent, silicate and latitude (Supplementary file 4d). The number of SAAVs and the number of invariant proteins, however, were more significant predictors of these groups compared to all environmental parameters (ANOVA, p-values:
The striking connection between geographically distant regions of the oceans through SAAVs suggests a likely role for adaptive processes to maintain the genomic heterogeneity of closely related SAR11 populations within 1a.3.V (Figure 4—figure supplement 5). In fact, both the main ecological niches and more refined proteotypes indicate that SAAVs are not primarily structured by the global dispersal of water masses but instead tend to link distant geographic regions with similar environmental conditions (Figure 4B). Overall, these results indicate that environmentally-mediated selection is a strong determinant of SAR11 evolution and biogeography.
One question remains: what is the proportion of distinct evolutionary processes acting upon closely related SAR11 populations within 1a.3.V? Offering a precise answer to this critical question is compounded by multiple theoretical and technical factors. These factors include, but are not limited to, (1) the phenomenon of genetic hitchhiking that prevents accurate determination of amino acid positions that likely confer fitness, (2) the metagenomic short-read recruitment strategy that prevents absolute confidence regarding the origin of each fragment, (3) heavy reliance on temperature as the sole environmental stressor to predict associations between environmental parameters and variation due to limited insights into in situ physiochemistry, (4) the lack of a complete understanding of syntrophic relationships between taxa in the environment, and (5) computational bottlenecks to gain rapid and accurate insights into the role of variable amino acid residues even when protein structures are available. With these significant limitations in mind, we could nevertheless speculate that among the 252,333 total codon positions, 37,416 were variable, suggesting purifying selection maintains the conservancy of 85% of the positions within 799 core 1a.3.V genes. Of those 37,416 positions that were within the scope of permissible mutations, 4592 had amino acid frequency trajectories that significantly correlated with temperature, suggesting an upper-bound of 12% for the variable positions that are likely under the influence of temperature-driven adaptive processes, while neutral processes explain at least 88% of the variation. In summary, this global view of the data suggests that among the remarkable amount of variation within some of the most abundant and prevalent microbial populations in the ocean, adaptive evolutionary processes operating on core genes are responsible for variation in about 2% of all codon positions.
Conclusions
We took advantage of billions of metagenomic reads to investigate single-amino acid variants (SAAVs) within the environmental core genes of the remarkably abundant and closely related SAR11 populations within subclade Ia.3.V, which we defined from a SAR11 metapangenome. The results elicit a highly-resolved quantitative description of purifying selection constraining the scope of permissible mutations to those that are not detrimental to protein stability requirements. Of permissible variation, thousands of codon positions harbored allele frequencies that systematically correlated with in situ temperatures and, overall, patterns of amino acid diversity reflected the temperature trends of large-scale ocean currents. This was especially apparent regarding the clear SAAV partitioning between Western and Eastern boundary currents. Previous studies have subdivided SAR11 clade Ia into cold-water (Ia.1) and warm-water (Ia.3) subclades with distinct latitudinal distributions (Brown et al., 2012), and reported sinusoidal oscillations between their abundances as a function of seawater temperature at a single temperate ocean site (Eren et al., 2015). At a much finer evolutionary scale (i.e., closely related populations within Ia.3.V), we observed significantly more protein variants in cold currents and more invariant proteins in warm currents, revealing a global pattern of alternating diversity for SAR11 in surface ocean currents in temperate and tropical latitudes. We were able to track this variation to changes in amino acid sequence preserved by selection.
Trends that emerged from our culture-independent survey of SAR11 were consistent with a recent study that also suggested an important role for environmental and ecological selective processes defining the spatial and temporal distribution of a widespread diatom species (Whittaker and Rynearson, 2017). Overall, these findings suggest that environmentally-mediated selection plays a critical role in the journey of cosmopolitan microbial populations in the surface ocean, lending credence to the idea for marine systems that ‘everything is everywhere but the environment selects’ (Baas-Becking, 1934). However, identifying environmental variables and their contributions to genomic heterogeneity within microbial populations is shrouded by both the dynamism and complexity of natural habitats, as well as the rich evolutionary dynamics that arise even in the simplest of conceivable environments (Good et al., 2017). These formidable challenges stress the importance of designing appropriate experiments to uncover variables that underpin the evolutionary divergence of closely related lineages, and drive transitions between them through space and time.
Materials and methods
The URL http://merenlab.org/data/sar11-saavs contains a reproducible bioinformatics workflow that extends the descriptions and parameters of programs used here for (1) the metapangenome of SAR11 using cultivar genomes, (2) the profiling of metagenomic reads that the cultivar genomes recruited, (3) the analysis of single nucleotide variants using Deep Learning, and (4) the visualization of single nucleotide variants in the context of protein structures.
SAR11 cultivar genomes
We acquired the genomic content of 21 SAR11 isolates from NCBI and simplified the deflines using anvi’o (Eren et al., 2015). We then concatenated all contigs into a single FASTA file, and generated an anvi’o contigs database, during which Prodigal (Hyatt et al., 2010) v2.6.3 identified open reading frames in contigs, and we annotated them with InterProScan (Zdobnov and Apweiler, 2001) v1.17. Supplementary file 1b reports the main genomic features.
Metagenomic datasets
We acquired 103 metagenomes from the European Bioinformatics Institute (EBI) repository under the project IDs ERP001736 (n = 93; TARA Oceans project) and ERP009703 (n = 10; Ocean Sampling Day project), and removed noisy reads with the illumina-utils library (Eren et al., 2013b) v1.4.1 (available from https://github.com/meren/illumina-utils using the program ‘iu-filter-quality-minoche’ with default parameters, which implements the method previously described by Minoche et al. (2011). Supplementary file 1a reports accession numbers and additional information (including the number of reads and environmental metadata) for each metagenome.
Pangenomic analysis
We used the anvi’o pangenomic workflow (Delmont and Eren, 2018) to organize translated gene sequences from SAR11 genomes into gene clusters. Briefly, anvi’o uses BLAST (Altschul et al., 1990) to assess the similarity between each pair of amino acid sequences among all genomes, and then resolves this graph into gene clusters using the Markov Cluster algorithm (Enright et al., 2002). We built the gene clustering metric using a minimum percent identity of 30%, an inflation value of 2, and a maxbit score of 0.5 for high sensitivity. Anvi’o used the occurrence of gene clusters across genomes data, which are also reported in Supplementary file 1e, to compute clustering dendrograms both for SAR11 genomes and gene clusters using Euclidian distance and Ward linkage algorithm.
Estimating distances between isolate genomes based on full-length 16S ribosomal RNA gene sequences
We used the program 'anvi-get-sequences-for-hmm-hits' (with parameters ‘--hmm-source Ribosomal_RNAs’ and ‘--gene-name Bacterial_16S_rRNA’) to recover full-length 16S ribosomal RNA gene sequences from the anvi’o contigs database for the 21 isolate genomes. We then used PyANI (Pritchard et al., 2016) through the program 'anvi-compute-ani' to estimate pairwise distances between each sequence.
Competitive recruitment and profiling of metagenomic reads
We mapped reads competitively from each metagenome against a single FASTA file containing all SAR11 genomes using Bowtie2 (Langmead and Salzberg, 2012) v.2.0.5 with default parameters, and converted the resulting SAM files into BAM files using samtools (Li et al., 2009a) v1.3.1. Competitive read recruitment ensures that short reads that match to more than one genome are assigned uniquely and randomly to one of the matching genomes. This minimizes computational biases at the mapping level and avoid inflated coverage statistics. To confirm our observations, we also used BWA (Li and Durbin, 2009b) to recruit reads (with the option n = 0.05). We used anvi’o to generate profile databases from the BAM files and combine these mapping profiles into a merged profile database, which stored coverage and variability statistics as outlined in Eren et al. (2015). Supplementary file 1c reports the mapping results (number of recruited reads, as well as mean coverage and detection statistics) per genome across the 103 metagenomes.
Determining the coverage of HIMB83 genes across metagenomes
The anvi’o merged profile database contains the coverage of individual genes across metagenomes. We normalized the coverage of HIMB83 genes in each metagenome (summarized in Supplementary file 1j) and calculated their coefficient of gene variation. We used the coefficient of gene variation estimates to identify metagenomes in which HIMB83 was well detected, yet the coverage values of its genes were highly unstable, which is an indicator of non-specific read recruitment from other lineages.
Determining the main ecological niche and core genes of 1a.3.V
We considered metagenomes in which HIMB83 was sufficiently abundant (mean genomic coverage >50X) with a stable detection of its genes (coefficient of gene variation <1.25) to represent the main ecological niche of 1a.3.V. To determine the core 1a.3.V genes, we first disregarded metagenomes that displayed an unusually high coefficient of gene coverage variation (Figure 1—figure supplement 1), which can indicate non-specific read recruitments from other abundant populations. The 74 metagenomes fitting these criteria are summarized in Supplementary file 1i. We defined the subset of HIMB83 genes as the core 1a.3.V genes if in each of the 74 metagenomes, the mean coverage of a gene remained within a factor of 5 of the mean coverage across all genes. The 799 genes fitting this criterion are summarized in Supplementary file 1j.
Calculation of percent identity distributions of recruited metagenomic short reads
We used percent identity distributions to broadly characterize how well short reads within a metagenome matched to the reference sequences by which they were recruited. We determined the percent identity for each read as
Generating single-nucleotide variants (SNV) data
We used the program 'anvi-gen-variability-profile' to report variability tables describing the nucleotide frequency (i.e., ratio of the four nucleotides) in recruited metagenomic reads per SNV position. To study the extent of variation of the core 1a.3.V genes across all metagenomes, we instructed anvi’o to report positions with more than 1% variation at the nucleotide level (i.e., at least 1% of recruited reads differ from the consensus nucleotide). To compare the densities of SAAVs to SNVs, we instructed anvi’o to report only positions with more than 10% variation at the nucleotide level. Supplementary file 1c reports the density of SNVs for all SAR11 genomes across all metagenomes. We also used anvi’o to report SNVs for a subset of genes and metagenomes, and by considering only nucleotide positions with a minimum coverage cut-off across metagenomes under consideration. Controlling the minimum coverage of single nucleotide positions across metagenomes improves confidence in variability analyses. Supplementary file 1L reports the SNV density values for all core 1a.3.V genes.
Definitions of ‘SAAV’, ‘allele frequency’ and ‘AAST’
A single amino acid variant (SAAV) is a codon position that exhibits variation in a metagenome, and the unique identifier of a SAAV is a single codon position and a metagenome. The position of a SAAV in the reference sequence, and a vector of 21 elements that contain the allele frequencies of each amino acid as well as the stop codon fully characterize a SAAV. The allele frequency of an amino acid is equal to the number of short reads that fully cover the codon that resolves to the amino acid, divided by the total number of reads that fully cover the same position (the sum of all 21 allele frequencies is therefore 1). We also attributed to each SAAV an amino acid substitution type (AAST), which corresponds to the two amino acids with the largest and second largest allele frequencies.
Generating single-amino acid variants (SAAVs) data
The program `anvi-gen-variability-profile` (with an additional ‘--engine AA’ flag) reported variability tables describing the allele frequencies for each SAAV. Anvi’o only considers short reads that cover the entire codon to determine amino acid frequencies at a given codon position in a metagenome. We instructed anvi’o to report only positions with more than 10% variation at the amino acid-level (i.e., at least 10% of recruited reads differ from the consensus amino acid). Supplementary file 1L reports the density of SAAVs for all SAR11 genome across all metagenomes. We also used anvi’o to report SAAVs for a subset of genes and metagenomes, and by considering only gene codons with a minimum coverage cut-off of 20X across all metagenomes of interest. Controlling the minimum coverage of gene codons across metagenomes improves confidence in variability analyses.
Differential occurrence of amino acids in SAAVs and in the core 1a.3.V genes
We determined the amino acid composition in the 799 core 1a.3.V genes as well as in SAAVs maintained in each metagenome using anvi’o programs `anvi-get-aa-counts` and `anvi-get-codon-frequencies` (with the flag `--return-AA-frequencies-instead`). We quantified the amino acid composition of all core 1a.3.V genes of in HIMB83 using the program `anvi-get-aa-counts`. In contrast, we quantified the amino acid composition of SAAVs by calculating the frequency of a given amino acid being one of the two dominant alleles. We then calculated p-values via a binomial test that represents the probability of observing the difference between amino acid frequencies computed over all core 1a.3.V genes versus only 1a.3.V SAAVs, given the null hypothesis that amino acids in 1a.3.V SAAVs are distributed according to the same distribution as the amino acids in the core 1a.3.V genes.
Estimating a neutral AAST frequency distribution
This calculation provides an estimate for the AAST frequency distribution given strictly neutral mutations. Unlike the neutral theory of evolution, it excludes the influential effects of purifying selection (negative selection coefficients). Since all mutations are equally likely to drift to detectable frequencies under a neutral model, the expected number of variant positions that have
Where
In general,
In Model 1,
In Model 2,
where
Predicting 3D structure of proteins using template-based modeling
We used a template-based structure modeling tool, RaptorX Structure Prediction (Källberg et al., 2012), to predict structures of 1a.3.V amino acid sequences based on available data from the Protein Data Bank (PDB) (Bernstein et al., 1977). We used the program blastp in NCBI’s BLAST distribution to identify core 1a.3.V genes that matched to an entry with at least 30% similarity over the length of the given core gene. We then programmatically mapped SAAVs from metagenomes onto the predicted tertiary structures, and used PyMOL (DeLano, 2002; Schrödinger LLC, 2015) to visualize these data. We colored SAAVs based on RaptorX-predicted structural properties, including solvent accessibility and secondary structure.
Identifying genes with disproportionately high number of temperature-correlated positions
First, we calculated the number of temperature-correlated and temperature-uncorrelated positions for each of the 1a.3.V core genes. Then, we performed a one-sided binomial test that these numbers are biased towards higher proportion of temperature-correlated positions compared to a model distribution defined from the total number of temperature-correlated positions in 1a.3.V. Since there were 4,592 such positions out of 37,416, the model probability of success was defined as
Predicting transmembrane, periplasmic, and cytosolic regions in the glycine betaine permease
To categorize amino acid positions as transmembrane, periplasmic, and cytosolic, we used Phobius (Käll et al., 2004; Käll et al., 2007), a membrane topology and prediction software through the webserver at http://phobius.sbc.su.se. The output is a probability of the four classes for each residue, and to simplify the data we categorized each residue into the class found to be most probable. We removed residues with signaling peptide association from downstream analyses.
Recovering codon position-specific AASTs from SAAVs
We simplified the hyper-dimensional SAAV data into a simpler presence-absence matrix for downstream analyses. For this, we defined codon position-specific AASTs (cAASTs) and summarized their occurrence across metagenomes. In such a table the value of ‘1’ indicates that a given metagenome had a SAAV at a given codon position that resolved to a given AAST. In contrast, the value ‘0’ indicates that the metagenome did not have a SAAV that resolved to this AAST. In the latter case a given metagenome may have another AAST in this particular codon position (in which case this information would appear in another row in the same table that is affiliated with the same AAST with the same codon position). Hence, each AAST listed in the first column of the table will be unique to a single codon position, yet a given codon position may have different AASTs in different metagenomes, resulting in multiple AASTs in the resulting table that belong to the same codon position. Combining AAST with the codon position would then result in a unique cAAST.
Application of deep learning to codon-position-specific AASTs data
To estimate an unbiased distance between our metagenomes based on SAAVs, we used a novel deep neural network modification called the auto-clustering output layer (ACOL). Briefly, ACOL relies on a recently introduced graph-based activity regularization (GAR) technique for competitive learning from hyper-dimensional data to demarcate fine clusters within user-defined ‘parent’ classes (Kilinc and Uysal, 2017). In this application of ACOL, however, we modified the algorithm so it can reveal latent groups in our SAAVs in a fully unsupervised manner through frequent random sampling of SAAVs to create pseudo-parent class labels instead of user-defined classes (Kilinc and Uysal, 2018). See the URL http://merenlab.org/data/sar11-saavs for the details of the pseudo parent-class generation algorithm, and the reproducible distance estimation workflow in Python.
Other statistical tests and visualization
We used the aov function in R to perform one way ANOVA tests, used the ggplot2 (Ginestet, 2011) package for R to visualize the relative distribution of 1a.3.V genes and geographic distribution of proteotypes, and finalized all figures using an open-source vector graphics editor, Inkscape (available from http://inkscape.org/).
Code and data availability
The vast majority of analyses relied on the open-source software platform anvi’o v2.4.0 (available from http://merenlab.org/software/anvio). The URL http://merenlab.org/data/sar11-saavs serves the remaining custom code used in our analyses. We made available (1) SAR11 isolate genomes (10.6084/m9.figshare.5248945), (2) the anvi’o contigs database and merged profile for SAR11 genomes across metagenomes (10.5281/zenodo.835218) and the static HTML summary for the mapping results (10.6084/m9.figshare.5248453), (3) the SAR11 metapangenome (10.6084/m9.figshare.5248459), single-nucleotide and single-amino acid variant reports for 1a.3.V across 74 TARA Oceans metagenomes (10.6084/m9.figshare.5248447), and (4) SAAVs overlaid on predicted tertiary structures of 58 core 1a.3.V genes (10.6084/m9.figshare.5248432). The URL http://anvi-server.org/p/4Q2TNo serves an interactive version of the SAR11 metapangenome, and the URL http://data.merenlab.org/sar11-saavs serves an interactive web page to investigate the link between SAAVs and predicted protein structures.
Acknowledgements
We thank the TARA Oceans consortium for generating metagenomic datasets of great legacy, as well as all researchers involved in the characterization of SAR11 isolates. We thank Kostas Konstantinidis, Edward Delong, Lois Maignien, Mike Lee, and the members of the Meren Lab for helpful discussions. We acknowledge the support of the Natural Sciences and Engineering Research Council of Canada (NSERC) to EK, as well as the Frank R Lillie Research Innovation Award and the start-up funds from the University of Chicago to AME.
Funding Statement
The funders had no role in study design, data collection and interpretation, or the decision to submit the work for publication.
Contributor Information
Paul B Rainey, Max Planck Institute for Evolutionary Biology, Germany.
Detlef Weigel, Max Planck Institute for Developmental Biology, Germany.
Funding Information
This paper was supported by the following grants:
University of Chicago to A Murat Eren.
Marine Biological Laboratory to A Murat Eren.
Additional information
Competing interests
No competing interests declared.
Author contributions
Conceptualization, Data curation, Formal analysis, Validation, Investigation, Visualization, Methodology, Writing—original draft.
Conceptualization, Data curation, Software, Formal analysis, Validation, Investigation, Visualization, Methodology, Writing—review and editing.
Software, Writing—review and editing.
Software, Visualization, Methodology.
Software, Supervision.
Supervision, Validation, Writing—review and editing.
Supervision, Validation, Project administration, Writing—review and editing.
Conceptualization, Resources, Data curation, Software, Formal analysis, Supervision, Funding acquisition, Validation, Investigation, Visualization, Methodology, Writing—original draft, Project administration, Writing—review and editing.
Additional files
Supplementary file 1.
Details of SAR11 genomes, marine metagenomes, and metagenomic read recruitment results.(a) Summary of 103 Tara Oceans and Ocean Sampling Day metagenomes. (b) Features of 21 SAR11 isolate genomes, clades to which they belong, and their genome-level metagenomic read recruitment summaries. (c) Comprehensive summary of metagenomic read recruitment results per SAR11 genome, including the number of recruited reads, mean coverage, relative distribution, as well as the detection of 21 SAR11 genomes across 103 metagenomes, along with the total number of single nucleotide variants (SNVs) and single amino acid variants (SAAVs) identified in each metagenome. (d) Summary of the SAR11 metapangenome and gene cluster membership statistics. (e) Presence/absence summary of gene clusters across SAR11 genomes. Distances between SAR11 genomes based on (f) their full-length 16S ribosomal RNA genes and (g) whole genome average nucleotide identity. (h) Relative distribution and abundance of 21 SAR11 genomes, 31 Prochlorococcus genomes, and 957 additional marine population genomes across from three studies across 103 metagenomes. (i) SAR11 genomes with more than 50X coverage across Tara Oceans Project and Ocean Sampling Day metagenomes. (j) Detection of individual HIMB83 genes across metagenomes and whether they belong to 1a.3.V core or not. (k) Summary of the degree of correlation between percent identity histograms of metagenomics reads recruited by HIMB83 and environmental data reported per metagenome. (l) SNV and SAAV density of HIMB83 across metagenomes. This table also reports the predicted functions of individual HIMB83 genes and their DNA sequences, and functional summary of HIMB83 genes that represent core 1a.3.V genes and those that belong to environmental accessory genes of HIMB83.
Supplementary file 2.
Details and raw data for single-nucleotide variants, single-amino acid variants, and amino acid substitution types.(a) SNV density of core 1a.3.V genes, (departure from consensus of >1%). (b) SNV density of core 1a.3.V genes (departure from consensus of >10%) (c) SAAV density of core 1a.3.V genes (departure from consensus of >10%). (d) Number of SAAVs among core 1a.3.V genes. (e) Distribution of invariant core 1a.3.V proteins across metagenomes. (f) Frequency and proportion of amino acids in SAAVs. (g) Comprehensive summary statistics and ratios for amino acid substitution types (AASTs) across metagenomes. (h) Unique coordinates per AAST in 738,324 core 1a.3.V genes (cAASTs) (1) that were covered more than 20X across 74 metagenomes, (2) and in which a divergence >10% from consensus was observed in the frequency of amino acids. (i) cAASTs across metagenomes. This table also reports BLOSUM estimates per AAST, and BLOSUM AAST summary statistics across core 1a.3.V genes.
Supplementary file 3.
Relationships between SAAVs and protein structures and temperature.(a) SAAV characteristics, including solvent accessibility for each SAAV belonging to a core 1a.3.V gene with a successfully predicted protein structure. (b) The correlation of allele frequencies to temperature for each position containing at least one SAAV. (c) The summary of the proportion of temperature-correlated and temperature-uncorrelated SAAV positions per core 1a.3.V gene.
Supplementary file 4.
Details of proteotypes within 1a.3.V.(a) Deep Learning-estimated distances between 74 metagenomes based on cAASTs reconstructed from 738,324 SAAVs. (b) Six 1a.3.V proteotypes. (c) Summary of all data per metagenome to estimate significant determinants of proteotype organization. (d) ANOVA test statistic per sample data category given the six proteotypes.
Transparent reporting form
Data availability
The vast majority of analyses relied on the open-source software platform anvi'o v2.4.0 (available from http://merenlab.org/software/anvio). The URL http://merenlab.org/data/sar11-saavs serves the remaining custom code used in our analyses. We made available (1) SAR11 isolate genomes (10.6084/m9.figshare.5248945), (2) the anvi'o contigs database and merged profile for SAR11 genomes across metagenomes (10.5281/zenodo.835218) and the static HTML summary for the mapping results (10.6084/m9.figshare.5248453), (3) the SAR11 metapangenome (10.6084/m9.figshare.5248459), single-nucleotide and single-amino acid variant reports for 1a.3.V across 74 TARA Oceans metagenomes (10.6084/m9.figshare.5248447), and (4) SAAVs overlaid on predicted tertiary structures of 58 core 1a.3.V genes (10.6084/m9.figshare.5248432). The URL http://anvi-server.org/p/4Q2TNo serves an interactive version of the SAR11 metapangenome, and the URL http://data.merenlab.org/sar11-saavs serves an interactive web page to investigate the link between SAAVs and predicted protein structures.
The following datasets were generated:
A Murat Eren. 2017. Anvi'o split profile for HIMB83 across metagenomes. figshare. [CrossRef]
A Murat Eren. 2017. The SAR11 Metapangenome. figshare. [CrossRef]
A Murat Eren. 2017. Anvi'o summary of SAR11 genomes across metagenomes. figshare. [CrossRef]
A Murat Eren. 2017. Raw SNV and SAAV data for SAR11 1a.3.V. figshare. [CrossRef]
A Murat Eren. 2017. S-LLPA SAAVs. figshare. [CrossRef]
A Murat Eren. 2017. Anvi'o merged profile database for 21 SAR11 isolates across metagenomes. Zenodo. [CrossRef]
The following previously published datasets were used:
Shinichi Sunagawa et al. 2015. Ocean plankton. Structure and function of the global ocean microbiome. NCBI SRA. PRJEB1787
Anna Kopf et al. 2015. The ocean sampling day consortium. EMBL-EBI. PRJEB5129
References
- Altschul SF, Gish W, Miller W, Myers EW, Lipman DJ. Basic local alignment search tool. Journal of Molecular Biology. 1990;215:403–410. 10.1016/S0022-2836(05)80360-2. [Abstract] [CrossRef] [Google Scholar]
- Anantharaman K, Brown CT, Hug LA, Sharon I, Castelle CJ, Probst AJ, Thomas BC, Singh A, Wilkins MJ, Karaoz U, Brodie EL, Williams KH, Hubbard SS, Banfield JF. Thousands of microbial genomes shed light on interconnected biogeochemical processes in an aquifer system. Nature Communications. 2016;7:13219. 10.1038/ncomms13219. [Europe PMC free article] [Abstract] [CrossRef] [Google Scholar]
- Anderson RE, Reveillaud J, Reddington E, Delmont TO, Eren AM, McDermott JM, Seewald JS, Huber JA. Genomic variation in microbial populations inhabiting the marine subseafloor at deep-sea hydrothermal vents. Nature Communications. 2017;8:1114. 10.1038/s41467-017-01228-6. [Europe PMC free article] [Abstract] [CrossRef] [Google Scholar]
- Baas-Becking LGM. Geobiologie of Inleiding Tot De Milieukunde. Den Haag: W.P. Van Stockum & Zoon; 1934. [Google Scholar]
- Bendall ML, Stevens SL, Chan LK, Malfatti S, Schwientek P, Tremblay J, Schackwitz W, Martin J, Pati A, Bushnell B, Froula J, Kang D, Tringe SG, Bertilsson S, Moran MA, Shade A, Newton RJ, McMahon KD, Malmstrom RR. Genome-wide selective sweeps and gene-specific sweeps in natural bacterial populations. The ISME Journal. 2016;10:1589–1601. 10.1038/ismej.2015.241. [Europe PMC free article] [Abstract] [CrossRef] [Google Scholar]
- Benjamini Y, Hochberg Y. Controlling the false discovery rate: a practical and powerful approach to multiple testing. Journal of the Royal Statistical Society: Series B. 1995;57:289–300. 10.1111/j.2517-6161.1995.tb02031.x. [CrossRef] [Google Scholar]
- Bernstein FC, Koetzle TF, Williams GJ, Meyer EF, Brice MD, Rodgers JR, Kennard O, Shimanouchi T, Tasumi M. The protein data bank. European Journal of Biochemistry. 1977;80:319–324. [Abstract] [Google Scholar]
- Bordo D, Argos P. Suggestions for "safe" residue substitutions in site-directed mutagenesis. Journal of Molecular Biology. 1991;217:721–729. 10.1016/0022-2836(91)90528-E. [Abstract] [CrossRef] [Google Scholar]
- Brown MV, Lauro FM, DeMaere MZ, Muir L, Wilkins D, Thomas T, Riddle MJ, Fuhrman JA, Andrews-Pfannkoch C, Hoffman JM, McQuaid JB, Allen A, Rintoul SR, Cavicchioli R. Global biogeography of SAR11 marine Bacteria. Molecular Systems Biology. 2012;8:595. 10.1038/msb.2012.28. [Europe PMC free article] [Abstract] [CrossRef] [Google Scholar]
- Brown CT, Hug LA, Thomas BC, Sharon I, Castelle CJ, Singh A, Wilkins MJ, Wrighton KC, Williams KH, Banfield JF. Unusual biology across a group comprising more than 15% of domain Bacteria. Nature. 2015;523:208–211. 10.1038/nature14486. [Abstract] [CrossRef] [Google Scholar]
- Brucks ES. University of Hawaii; 2014. Metagenome recruitment of the global ocean survey dataset to four closely-related SAR11 genomes.https://pdfs.semanticscholar.org/3cd6/8dec1657d2d4e7b1577f321f1aae62bd73e7.pdf [Google Scholar]
- Bustamante CD, Townsend JP, Hartl DL. Solvent accessibility and purifying selection within proteins of Escherichia coli and Salmonella enterica. Molecular Biology and Evolution. 2000;17:301–308. 10.1093/oxfordjournals.molbev.a026310. [Abstract] [CrossRef] [Google Scholar]
- Carlson CA, Morris R, Parsons R, Treusch AH, Giovannoni SJ, Vergin K. Seasonal dynamics of SAR11 populations in the euphotic and mesopelagic zones of the northwestern sargasso sea. The ISME Journal. 2009;3:283–295. 10.1038/ismej.2008.117. [Abstract] [CrossRef] [Google Scholar]
- Caro-Quintero A, Konstantinidis KT. Bacterial species may exist, metagenomics reveal. Environmental Microbiology. 2012;14:347–355. 10.1111/j.1462-2920.2011.02668.x. [Abstract] [CrossRef] [Google Scholar]
- Chen H, Zhou HX. Prediction of solvent accessibility and sites of deleterious mutations from protein sequence. Nucleic Acids Research. 2005;33:3193–3199. 10.1093/nar/gki633. [Europe PMC free article] [Abstract] [CrossRef] [Google Scholar]
- Cohan FM. Systematics: the cohesive nature of bacterial species Taxa. Current Biology. 2019;29:R169–R172. 10.1016/j.cub.2019.01.033. [Abstract] [CrossRef] [Google Scholar]
- Cohan FM, Perry EB. A systematics for discovering the fundamental units of bacterial diversity. Current Biology. 2007;17:R373–R386. 10.1016/j.cub.2007.03.032. [Abstract] [CrossRef] [Google Scholar]
- Coleman ML, Sullivan MB, Martiny AC, Steglich C, Barry K, Delong EF, Chisholm SW. Genomic islands and the ecology and evolution of Prochlorococcus. Science. 2006;311:1768–1770. 10.1126/science.1122050. [Abstract] [CrossRef] [Google Scholar]
- Costea PI, Munch R, Coelho LP, Paoli L, Sunagawa S, Bork P. metaSNV: a tool for metagenomic strain level analysis. PLOS ONE. 2017;12:e0182392. 10.1371/journal.pone.0182392. [Europe PMC free article] [Abstract] [CrossRef] [Google Scholar]
- Darwin C. On the Origin of Species. Routledge; 1859. [Google Scholar]
- DeLano W. Schrödinger LLC; 2002. http://pymol.org [Google Scholar]
- Delmont TO, Quince C, Shaiber A, Esen ÖC, Lee ST, Rappé MS, McLellan SL, Lücker S, Eren AM. Nitrogen-fixing populations of planctomycetes and proteobacteria are abundant in surface ocean metagenomes. Nature Microbiology. 2018;3:804–813. 10.1038/s41564-018-0176-9. [Europe PMC free article] [Abstract] [CrossRef] [Google Scholar]
- Delmont TO, Eren AM. Linking pangenomes and metagenomes: the Prochlorococcus metapangenome. PeerJ. 2018;6:e4320. 10.7717/peerj.4320. [Europe PMC free article] [Abstract] [CrossRef] [Google Scholar]
- Denef VJ. Peering into the genetic makeup of natural microbial populations using metagenomics. In: Polz M, Rajora O, editors. Population Genomics: Microorganisms. Cham: Springer; 2018. [CrossRef] [Google Scholar]
- Domingo E, Sheldon J, Perales C. Viral quasispecies evolution. Microbiology and Molecular Biology Reviews. 2012;76:159–216. 10.1128/MMBR.05023-11. [Europe PMC free article] [Abstract] [CrossRef] [Google Scholar]
- Eiler A, Hayakawa DH, Church MJ, Karl DM, Rappé MS. Dynamics of the SAR11 bacterioplankton lineage in relation to environmental conditions in the oligotrophic north pacific subtropical gyre. Environmental Microbiology. 2009;11:2291–2300. 10.1111/j.1462-2920.2009.01954.x. [Abstract] [CrossRef] [Google Scholar]
- Enright AJ, Van Dongen S, Ouzounis CA. An efficient algorithm for large-scale detection of protein families. Nucleic Acids Research. 2002;30:1575–1584. 10.1093/nar/30.7.1575. [Europe PMC free article] [Abstract] [CrossRef] [Google Scholar]
- Eren AM, Maignien L, Sul WJ, Murphy LG, Grim SL, Morrison HG, Sogin ML. Oligotyping: differentiating between closely related microbial taxa using 16S rRNA gene data. Methods in Ecology and Evolution. 2013a;4:1111–1119. 10.1111/2041-210X.12114. [Europe PMC free article] [Abstract] [CrossRef] [Google Scholar]
- Eren AM, Vineis JH, Morrison HG, Sogin ML. A filtering method to generate high quality short reads using Illumina paired-end technology. PLOS ONE. 2013b;8:e66643. 10.1371/journal.pone.0066643. [Europe PMC free article] [Abstract] [CrossRef] [Google Scholar]
- Eren AM, Esen ÖC, Quince C, Vineis JH, Morrison HG, Sogin ML, Delmont TO. Anvi'o: an advanced analysis and visualization platform for 'omics data. PeerJ. 2015;3:e1319. 10.7717/peerj.1319. [Europe PMC free article] [Abstract] [CrossRef] [Google Scholar]
- Ferla MP, Thrash JC, Giovannoni SJ, Patrick WM. New rRNA gene-based phylogenies of the alphaproteobacteria provide perspective on major groups, mitochondrial ancestry and phylogenetic instability. PLOS ONE. 2013;8:e83383. 10.1371/journal.pone.0083383. [Europe PMC free article] [Abstract] [CrossRef] [Google Scholar]
- Field KG, Gordon D, Wright T, Rappé M, Urback E, Vergin K, Giovannoni SJ. Diversity and depth-specific distribution of SAR11 cluster rRNA genes from marine planktonic Bacteria. Applied and Environmental Microbiology. 1997;63:63–70. [Europe PMC free article] [Abstract] [Google Scholar]
- Garcia SL, Stevens SLR, Crary B, Martinez-Garcia M, Stepanauskas R, Woyke T, Tringe SG, Andersson SGE, Bertilsson S, Malmstrom RR, McMahon KD. Contrasting patterns of genome-level diversity across distinct co-occurring bacterial populations. The ISME Journal. 2018;12:742–755. 10.1038/s41396-017-0001-0. [Europe PMC free article] [Abstract] [CrossRef] [Google Scholar]
- Ginestet C. ggplot2: elegant graphics for data analysis. Journal of the Royal Statistical Society: Series A. 2011;174:245–246. 10.1111/j.1467-985X.2010.00676_9.x. [CrossRef] [Google Scholar]
- Giovannoni SJ, Britschgi TB, Moyer CL, Field KG. Genetic diversity in sargasso sea bacterioplankton. Nature. 1990;345:60–63. 10.1038/345060a0. [Abstract] [CrossRef] [Google Scholar]
- Giovannoni SJ, Bibbs L, Cho JC, Stapels MD, Desiderio R, Vergin KL, Rappé MS, Laney S, Wilhelm LJ, Tripp HJ, Mathur EJ, Barofsky DF. Proteorhodopsin in the ubiquitous marine bacterium SAR11. Nature. 2005;438:82–85. 10.1038/nature04032. [Abstract] [CrossRef] [Google Scholar]
- Giovannoni SJ. SAR11 Bacteria: the most abundant plankton in the oceans. Annual Review of Marine Science. 2017;9:231–255. 10.1146/annurev-marine-010814-015934. [Abstract] [CrossRef] [Google Scholar]
- Good BH, McDonald MJ, Barrick JE, Lenski RE, Desai MM. The dynamics of molecular evolution over 60,000 generations. Nature. 2017;551:45–50. 10.1038/nature24287. [Europe PMC free article] [Abstract] [CrossRef] [Google Scholar]
- Grote J, Thrash JC, Huggett MJ, Landry ZC, Carini P, Giovannoni SJ, Rappé MS. Streamlining and core genome conservation among highly divergent members of the SAR11 clade. mBio. 2012;3:e00252-12. 10.1128/mBio.00252-12. [Europe PMC free article] [Abstract] [CrossRef] [Google Scholar]
- Hellweger FL, van Sebille E, Fredrick ND. Biogeographic patterns in ocean microbes emerge in a neutral agent-based model. Science. 2014;345:1346–1349. 10.1126/science.1254421. [Abstract] [CrossRef] [Google Scholar]
- Hyatt D, Chen GL, Locascio PF, Land ML, Larimer FW, Hauser LJ. Prodigal: prokaryotic gene recognition and translation initiation site identification. BMC Bioinformatics. 2010;11:119. 10.1186/1471-2105-11-119. [Europe PMC free article] [Abstract] [CrossRef] [Google Scholar]
- Jain C, Rodriguez-R LM, Phillippy AM, Konstantinidis KT, Aluru S. High throughput ANI analysis of 90K prokaryotic genomes reveals clear species boundaries. Nature Communications. 2018;9:5114. 10.1038/s41467-018-07641-9. [Europe PMC free article] [Abstract] [CrossRef] [Google Scholar]
- Jönsson BF, Watson JR. The timescales of global surface-ocean connectivity. Nature Communications. 2016;7:11239. 10.1038/ncomms11239. [Europe PMC free article] [Abstract] [CrossRef] [Google Scholar]
- Käll L, Krogh A, Sonnhammer EL. A combined transmembrane topology and signal peptide prediction method. Journal of Molecular Biology. 2004;338:1027–1036. 10.1016/j.jmb.2004.03.016. [Abstract] [CrossRef] [Google Scholar]
- Käll L, Krogh A, Sonnhammer EL. Advantages of combined transmembrane topology and signal peptide prediction--the phobius web server. Nucleic Acids Research. 2007;35:W429–W432. 10.1093/nar/gkm256. [Europe PMC free article] [Abstract] [CrossRef] [Google Scholar]
- Källberg M, Wang H, Wang S, Peng J, Wang Z, Lu H, Xu J. Template-based protein structure modeling using the RaptorX web server. Nature Protocols. 2012;7:1511–1522. 10.1038/nprot.2012.085. [Europe PMC free article] [Abstract] [CrossRef] [Google Scholar]
- Kashtan N, Roggensack SE, Rodrigue S, Thompson JW, Biller SJ, Coe A, Ding H, Marttinen P, Malmstrom RR, Stocker R, Follows MJ, Stepanauskas R, Chisholm SW. Single-cell genomics reveals hundreds of coexisting subpopulations in wild Prochlorococcus. Science. 2014;344:416–420. 10.1126/science.1248575. [Abstract] [CrossRef] [Google Scholar]
- Kilinc O, Uysal I. Auto-clustering output layer: automatic learning of latent annotations in neural networks. arXiv. 2017 https://arxiv.org/abs/1702.08648
- Kilinc O, Uysal I. Learning latent representations in neural networks for clusteringthrough pseudo supervision and Graph-Based activity regularization. International Conference on Learning Representations; 2018. [Google Scholar]
- Konstantinidis KT, DeLong EF. Genomic patterns of recombination, clonal divergence and environment in marine microbial populations. The ISME Journal. 2008;2:1052–1065. 10.1038/ismej.2008.62. [Abstract] [CrossRef] [Google Scholar]
- Konstantinidis KT, Tiedje JM. Genomic insights that advance the species definition for prokaryotes. PNAS. 2005;102:2567–2572. 10.1073/pnas.0409727102. [Europe PMC free article] [Abstract] [CrossRef] [Google Scholar]
- Kopf A, Bicak M, Kottmann R, Schnetzer J, Kostadinov I, Lehmann K, Fernandez-Guerra A, Jeanthon C, Rahav E, Ullrich M, Wichels A, Gerdts G, Polymenakou P, Kotoulas G, Siam R, Abdallah RZ, Sonnenschein EC, Cariou T, O'Gara F, Jackson S, Orlic S, Steinke M, Busch J, Duarte B, Caçador I, Canning-Clode J, Bobrova O, Marteinsson V, Reynisson E, Loureiro CM, Luna GM, Quero GM, Löscher CR, Kremp A, DeLorenzo ME, Øvreås L, Tolman J, LaRoche J, Penna A, Frischer M, Davis T, Katherine B, Meyer CP, Ramos S, Magalhães C, Jude-Lemeilleur F, Aguirre-Macedo ML, Wang S, Poulton N, Jones S, Collin R, Fuhrman JA, Conan P, Alonso C, Stambler N, Goodwin K, Yakimov MM, Baltar F, Bodrossy L, Van De Kamp J, Frampton DM, Ostrowski M, Van Ruth P, Malthouse P, Claus S, Deneudt K, Mortelmans J, Pitois S, Wallom D, Salter I, Costa R, Schroeder DC, Kandil MM, Amaral V, Biancalana F, Santana R, Pedrotti ML, Yoshida T, Ogata H, Ingleton T, Munnik K, Rodriguez-Ezpeleta N, Berteaux-Lecellier V, Wecker P, Cancio I, Vaulot D, Bienhold C, Ghazal H, Chaouni B, Essayeh S, Ettamimi S, Zaid elH, Boukhatem N, Bouali A, Chahboune R, Barrijal S, Timinouni M, El Otmani F, Bennani M, Mea M, Todorova N, Karamfilov V, Ten Hoopen P, Cochrane G, L'Haridon S, Bizsel KC, Vezzi A, Lauro FM, Martin P, Jensen RM, Hinks J, Gebbels S, Rosselli R, De Pascale F, Schiavon R, Dos Santos A, Villar E, Pesant S, Cataletto B, Malfatti F, Edirisinghe R, Silveira JA, Barbier M, Turk V, Tinta T, Fuller WJ, Salihoglu I, Serakinci N, Ergoren MC, Bresnan E, Iriberri J, Nyhus PA, Bente E, Karlsen HE, Golyshin PN, Gasol JM, Moncheva S, Dzhembekova N, Johnson Z, Sinigalliano CD, Gidley ML, Zingone A, Danovaro R, Tsiamis G, Clark MS, Costa AC, El Bour M, Martins AM, Collins RE, Ducluzeau AL, Martinez J, Costello MJ, Amaral-Zettler LA, Gilbert JA, Davies N, Field D, Glöckner FO. The ocean sampling day consortium. GigaScience. 2015;4:27. 10.1186/s13742-015-0066-5. [Europe PMC free article] [Abstract] [CrossRef] [Google Scholar]
- Langmead B, Salzberg SL. Fast gapped-read alignment with bowtie 2. Nature Methods. 2012;9:357–359. 10.1038/nmeth.1923. [Europe PMC free article] [Abstract] [CrossRef] [Google Scholar]
- Li H, Handsaker B, Wysoker A, Fennell T, Ruan J, Homer N, Marth G, Abecasis G, Durbin R, 1000 Genome Project Data Processing Subgroup The Sequence Alignment/Map format and SAMtools. Bioinformatics. 2009a;25:2078–2079. 10.1093/bioinformatics/btp352. [Europe PMC free article] [Abstract] [CrossRef] [Google Scholar]
- Li H, Durbin R. Fast and accurate short read alignment with Burrows-Wheeler transform. Bioinformatics. 2009b;25:1754–1760. 10.1093/bioinformatics/btp324. [Europe PMC free article] [Abstract] [CrossRef] [Google Scholar]
- Manrique JM, Jones LR. Are ocean currents too slow to counteract SAR11 evolution? A next-generation sequencing, phylogeographic analysis. Molecular Phylogenetics and Evolution. 2017;107:324–337. 10.1016/j.ympev.2016.11.015. [Abstract] [CrossRef] [Google Scholar]
- Meziti A, Tsementzi D, Rodriguez-R LM, Hatt JK, Karayanni H, Kormas KA, Konstantinidis KT. Quantifying the changes in genetic diversity within sequence-discrete bacterial populations across a spatial and temporal riverine gradient. The ISME Journal. 2019;13:767–779. 10.1038/s41396-018-0307-6. [Europe PMC free article] [Abstract] [CrossRef] [Google Scholar]
- Minoche AE, Dohm JC, Himmelbauer H. Evaluation of genomic high-throughput sequencing data generated on illumina HiSeq and genome analyzer systems. Genome Biology. 2011;12:R112. 10.1186/gb-2011-12-11-r112. [Europe PMC free article] [Abstract] [CrossRef] [Google Scholar]
- Morris RM, Rappé MS, Connon SA, Vergin KL, Siebold WA, Carlson CA, Giovannoni SJ. SAR11 clade dominates ocean surface bacterioplankton communities. Nature. 2002;420:806–810. 10.1038/nature01240. [Abstract] [CrossRef] [Google Scholar]
- Nayfach S, Rodriguez-Mueller B, Garud N, Pollard KS. An integrated metagenomics pipeline for strain profiling reveals novel patterns of bacterial transmission and biogeography. Genome Research. 2016;26:1612–1625. 10.1101/gr.201863.115. [Europe PMC free article] [Abstract] [CrossRef] [Google Scholar]
- Noell SE, Giovannoni SJ. SAR11 Bacteria have a high affinity and multifunctional glycine betaine transporter. Environmental Microbiology. 2019;21:2559–2575. 10.1111/1462-2920.14649. [Abstract] [CrossRef] [Google Scholar]
- Oh HM, Kang I, Lee K, Jang Y, Lim SI, Cho JC. Complete genome sequence of strain IMCC9063, belonging to SAR11 subgroup 3, isolated from the arctic ocean. Journal of Bacteriology. 2011;193:3379–3380. 10.1128/JB.05033-11. [Europe PMC free article] [Abstract] [CrossRef] [Google Scholar]
- Ohta T, Gillespie JH. Development of neutral and nearly neutral theories. Theoretical Population Biology. 1996;49:128–142. 10.1006/tpbi.1996.0007. [Abstract] [CrossRef] [Google Scholar]
- Pritchard L, Glover RH, Humphris S, Elphinstone JG, Toth IK. Genomics and taxonomy in diagnostics for food security: soft-rotting enterobacterial plant pathogens. Analytical Methods. 2016;8:12–24. 10.1039/C5AY02550H. [CrossRef] [Google Scholar]
- Rappé MS, Connon SA, Vergin KL, Giovannoni SJ. Cultivation of the ubiquitous SAR11 marine bacterioplankton clade. Nature. 2002;418:630–633. 10.1038/nature00917. [Abstract] [CrossRef] [Google Scholar]
- Rocha EPC. Neutral theory, microbial practice: challenges in bacterial population genetics. Molecular Biology and Evolution. 2018;35:1338–1347. 10.1093/molbev/msy078. [Abstract] [CrossRef] [Google Scholar]
- Rodríguez-Ezpeleta N, Embley TM. The SAR11 group of alpha-proteobacteria is not related to the origin of mitochondria. PLOS ONE. 2012;7:e30520. 10.1371/journal.pone.0030520. [Europe PMC free article] [Abstract] [CrossRef] [Google Scholar]
- Schattenhofer M, Fuchs BM, Amann R, Zubkov MV, Tarran GA, Pernthaler J. Latitudinal distribution of prokaryotic picoplankton populations in the Atlantic ocean. Environmental Microbiology. 2009;11:2078–2093. 10.1111/j.1462-2920.2009.01929.x. [Abstract] [CrossRef] [Google Scholar]
- Schloissnig S, Arumugam M, Sunagawa S, Mitreva M, Tap J, Zhu A, Waller A, Mende DR, Kultima JR, Martin J, Kota K, Sunyaev SR, Weinstock GM, Bork P. Genomic variation landscape of the human gut microbiome. Nature. 2013;493:45–50. 10.1038/nature11711. [Europe PMC free article] [Abstract] [CrossRef] [Google Scholar]
- Scholz M, Ward DV, Pasolli E, Tolio T, Zolfo M, Asnicar F, Truong DT, Tett A, Morrow AL, Segata N. Strain-level microbial epidemiology and population genomics from shotgun metagenomics. Nature Methods. 2016;13:435–438. 10.1038/nmeth.3802. [Abstract] [CrossRef] [Google Scholar]
- Schrider DR, Hourmozdi JN, Hahn MW. Pervasive multinucleotide mutational events in eukaryotes. Current Biology. 2011;21:1051–1054. 10.1016/j.cub.2011.05.013. [Europe PMC free article] [Abstract] [CrossRef] [Google Scholar]
- Schrödinger LLC The {PyMOL} Molecular Graphics System. ~1.82015
- Shapiro BJ. What microbial population genomics has taught Us about speciation. In: Polz M, Rajora O, editors. Population Genomics: Microorganisms. Chem: Springer; 2018. [CrossRef] [Google Scholar]
- Shapiro BJ, Polz MF. Microbial speciation. Cold Spring Harbor Perspectives in Biology. 2015;7:a018143. 10.1101/cshperspect.a018143. [Europe PMC free article] [Abstract] [CrossRef] [Google Scholar]
- Simmons SL, Dibartolo G, Denef VJ, Goltsman DS, Thelen MP, Banfield JF. Population genomic analysis of strain variation in Leptospirillum group II Bacteria involved in acid mine drainage formation. PLOS Biology. 2008;6:e177. 10.1371/journal.pbio.0060177. [Europe PMC free article] [Abstract] [CrossRef] [Google Scholar]
- Smith NG, Webster MT, Ellegren H. A low rate of simultaneous double-nucleotide mutations in primates. Molecular Biology and Evolution. 2003;20:47–53. 10.1093/molbev/msg003. [Abstract] [CrossRef] [Google Scholar]
- Sojo V, Dessimoz C, Pomiankowski A, Lane N. Membrane proteins are dramatically less conserved than Water-Soluble proteins across the tree of life. Molecular Biology and Evolution. 2016;33:2874–2884. 10.1093/molbev/msw164. [Europe PMC free article] [Abstract] [CrossRef] [Google Scholar]
- Spang A, Saw JH, Jørgensen SL, Zaremba-Niedzwiedzka K, Martijn J, Lind AE, van Eijk R, Schleper C, Guy L, Ettema TJG. Complex archaea that bridge the gap between prokaryotes and eukaryotes. Nature. 2015;521:173–179. 10.1038/nature14447. [Europe PMC free article] [Abstract] [CrossRef] [Google Scholar]
- Stingl U, Tripp HJ, Giovannoni SJ. Improvements of high-throughput culturing yielded novel SAR11 strains and other abundant marine Bacteria from the Oregon coast and the Bermuda Atlantic time series study site. The ISME Journal. 2007;1:361–371. 10.1038/ismej.2007.49. [Abstract] [CrossRef] [Google Scholar]
- Sunagawa S, Coelho LP, Chaffron S, Kultima JR, Labadie K, Salazar G, Djahanschiri B, Zeller G, Mende DR, Alberti A, Cornejo-Castillo FM, Costea PI, Cruaud C, d'Ovidio F, Engelen S, Ferrera I, Gasol JM, Guidi L, Hildebrand F, Kokoszka F, Lepoivre C, Lima-Mendez G, Poulain J, Poulos BT, Royo-Llonch M, Sarmento H, Vieira-Silva S, Dimier C, Picheral M, Searson S, Kandels-Lewis S, Bowler C, de Vargas C, Gorsky G, Grimsley N, Hingamp P, Iudicone D, Jaillon O, Not F, Ogata H, Pesant S, Speich S, Stemmann L, Sullivan MB, Weissenbach J, Wincker P, Karsenti E, Raes J, Acinas SG, Bork P, Tara Oceans coordinators Ocean plankton. Structure and function of the global ocean microbiome. Science. 2015;348:1261359. 10.1126/science.1261359. [Abstract] [CrossRef] [Google Scholar]
- Thrash JC, Boyd A, Huggett MJ, Grote J, Carini P, Yoder RJ, Robbertse B, Spatafora JW, Rappé MS, Giovannoni SJ. Phylogenomic evidence for a common ancestor of mitochondria and the SAR11 clade. Scientific Reports. 2011;1:1–9. 10.1038/srep00013. [Europe PMC free article] [Abstract] [CrossRef] [Google Scholar]
- Treusch AH, Vergin KL, Finlay LA, Donatz MG, Burton RM, Carlson CA, Giovannoni SJ. Seasonality and vertical structure of microbial communities in an ocean gyre. The ISME Journal. 2009;3:1148–1163. 10.1038/ismej.2009.60. [Abstract] [CrossRef] [Google Scholar]
- Truong DT, Tett A, Pasolli E, Huttenhower C, Segata N. Microbial strain-level population structure and genetic diversity from metagenomes. Genome Research. 2017;27:626–638. 10.1101/gr.216242.116. [Europe PMC free article] [Abstract] [CrossRef] [Google Scholar]
- Tsementzi D, Wu J, Deutsch S, Nath S, Rodriguez-R LM, Burns AS, Ranjan P, Sarode N, Malmstrom RR, Padilla CC, Stone BK, Bristow LA, Larsen M, Glass JB, Thamdrup B, Woyke T, Konstantinidis KT, Stewart FJ. SAR11 Bacteria linked to ocean Anoxia and nitrogen loss. Nature. 2016;536:179–183. 10.1038/nature19068. [Europe PMC free article] [Abstract] [CrossRef] [Google Scholar]
- Tully BJ, Graham ED, Heidelberg JF. The reconstruction of 2,631 draft metagenome-assembled genomes from the global oceans. Scientific Data. 2018;5:1–8. 10.1038/sdata.2017.203. [Europe PMC free article] [Abstract] [CrossRef] [Google Scholar]
- Varghese NJ, Mukherjee S, Ivanova N, Konstantinidis KT, Mavrommatis K, Kyrpides NC, Pati A. Microbial species delineation using whole genome sequences. Nucleic Acids Research. 2015;43:6761–6771. 10.1093/nar/gkv657. [Europe PMC free article] [Abstract] [CrossRef] [Google Scholar]
- Vergin KL, Tripp HJ, Wilhelm LJ, Denver DR, Rappé MS, Giovannoni SJ. High intraspecific recombination rate in a native population of candidatus pelagibacter ubique (SAR11) Environmental Microbiology. 2007;9:2430–2440. 10.1111/j.1462-2920.2007.01361.x. [Abstract] [CrossRef] [Google Scholar]
- Viklund J, Martijn J, Ettema TJ, Andersson SG. Comparative and phylogenomic evidence that the alphaproteobacterium HIMB59 is not a member of the oceanic SAR11 clade. PLOS ONE. 2013;8:e78858. 10.1371/journal.pone.0078858. [Europe PMC free article] [Abstract] [CrossRef] [Google Scholar]
- Weir BS. Estimating F-statistics: a historical view. Philosophy of Science. 2012;79:637–643. 10.1086/667904. [Europe PMC free article] [Abstract] [CrossRef] [Google Scholar]
- White AE, Giovannoni SJ, Zhao Y, Vergin K, Carlson CA. Elemental content and stoichiometry of SAR11 chemoheterotrophic marine Bacteria. Limnology and Oceanography Letters. 2019;4:44–51. 10.1002/lol2.10103. [CrossRef] [Google Scholar]
- Whittaker KA, Rynearson TA. Evidence for environmental and ecological selection in a microbe with no geographic limits to gene flow. PNAS. 2017;114:2651–2656. 10.1073/pnas.1612346114. [Europe PMC free article] [Abstract] [CrossRef] [Google Scholar]
- Worth CL, Gong S, Blundell TL. Structural and functional constraints in the evolution of protein families. Nature Reviews Molecular Cell Biology. 2009;10:709–720. 10.1038/nrm2762. [Abstract] [CrossRef] [Google Scholar]
- Zaremba-Niedzwiedzka K, Viklund J, Zhao W, Ast J, Sczyrba A, Woyke T, McMahon K, Bertilsson S, Stepanauskas R, Andersson SG. Single-cell genomics reveal low recombination frequencies in freshwater Bacteria of the SAR11 clade. Genome Biology. 2013;14:R130. 10.1186/gb-2013-14-11-r130. [Europe PMC free article] [Abstract] [CrossRef] [Google Scholar]
- Zdobnov EM, Apweiler R. InterProScan--an integration platform for the signature-recognition methods in InterPro. Bioinformatics. 2001;17:847–848. 10.1093/bioinformatics/17.9.847. [Abstract] [CrossRef] [Google Scholar]
- Zinger L, Amaral-Zettler LA, Fuhrman JA, Horner-Devine MC, Huse SM, Welch DB, Martiny JB, Sogin M, Boetius A, Ramette A. Global patterns of bacterial beta-diversity in seafloor and seawater ecosystems. PLOS ONE. 2011;6:e24570. 10.1371/journal.pone.0024570. [Europe PMC free article] [Abstract] [CrossRef] [Google Scholar]
Decision letter
Thank you for submitting your work entitled "The global biogeography of a single SAR11 population is governed by natural selection" for consideration by eLife. Your article has been reviewed by three peer reviewers, including Paul Rainey as the Reviewing Editor, and the evaluation has been overseen by Detlef Weigel as the Senior Editor. The outside reviewers have opted to remain anonymous.
Our decision has been reached after extensive consultation between the reviewers. Based on these discussions and the individual reviews below, we regret to inform you that your work will not be considered further for publication at this time. However, we do see the potential for worthy eLife publication, provided all the issues raised by the reviewers can be addressed. Any resubmission would need to be a very substantial revision and would be treated as a new submission.
All reviewers agree that the topic is of considerable interest. They also indicate that your methods appear to have many strengths, but at the same time all are in agreement that much more needs to be done to justify your approaches. For example, Reviewer 3 requests calibration against standard population genetic approaches. Additional problems concern the main claims of the paper such as selective sweeps, the effect of selection, and so forth, which are stated, but not evidently backed by data. Further issues surround use and understanding of terms in population biology/ecology: Reviewer 2 points out the problems with refereeing to diversification within a population when the "population" of interest is really a substantial portion of a genus.
Reviewer #1:
This deals with the causes of patterns of diversity in a widespread marine microbe. The data come from a set of contigs assembled from shot gun sequencing of 21 genomes onto which are read-mapped metagenomic data from 103 samples. The focus is a particular clone that is particularly abundant in southern samples. A core genome is assembled from the metagenomic data and this is interrogated for signatures of selection using a curious approach based on physico-chemical properties of amino acids. A further step looks for correlations with water currents.
At first glance I found the paper reasonably clear, but the closer I read, the more murky things became. In fact I am not too sure what the authors have really done and I am not persuaded that their main claim, that selection is responsible for biogeography in S-LLPA, is supported by their data. Overall the level of explanation is insufficient to allow the reader to understand what has been done.
Among the difficulties:
Title/Abstract: The title states that biogeography is governed by selection, but in the Abstract the authors use more temperate language. So I guess they are not so sure. The Abstract claims that systematic purifying selection and adaptive mechanisms governing non-synonymous variation have been identified, but at best what they have are signatures consistent with expectations. I disagree that analysis of sequence data reveals different niches (it may reveal the existence of ecotypes that may be indicative of distinct niches). What is a proteotype?
Place of isolation of the focal 21 SAR11 genomes is important in a study of biogeography, but no attention has been given to this.
There are many challenges in drawing conclusions from metagenomic data via read-mapping to a reference. Particularly problematic is the fact that diversity of closely related organisms within a sample is not known. Thus when many SNPs are detected is this indicative of a diverse population, or is it a signature that comes from a single over-represented clone that is rather divergent? Similarly, the authors take high similarity as signature of a recent sweep, but this might reflect a single over-represented genome from a closely related organism. I'm not sure how the authors dealt with these and similar problems.
With regard to selective sweeps, the results of the analysis appear not to be given (subsection “SNVs to SAAVs: Accurate characterization of non-synonymous variation”, gives the conditions that need to be fulfilled in order for a protein to have undergone a sweep, but not the results).
Patterns of amino acid substitution types seems an interesting way to go, but this needs to be unpacked, explained and justified.
Partitioning of SAAV between warm and cold currents: there seems to be a correlation with clustering based on SAAVs, but as above, I need to have more explanation. Much relies on the robustness of the SAAV profile clustering (Figure 3A) and there is no indication of its statistical likelihood.
The crunch of the paper is rejection of the neutral model, but this is done by simply saying that neutral models could not explain the observed patters of amino acid substitutions, but no evidence is provided. Also, Figure 4, which I hoped would enlighten me, does not exist.
In general I found the figures overly dense and very difficult to understand given the paucity of explanation in the captions. Take Figure 1: latitudinal gradient, I presume north is at the top? Colouring seems inconsistent and so on.
Reviewer #2:
It's hard to tell who this paper is written for. The bioinformatics applications are the most interesting: identifying the core genome of a lineage of interest from metagenomics data, using a novel algorithm for (somehow) identifying significant subclades within the lineage, and finding a surprisingly high frequency of certain amino acid substitutions that are not predicted by Blosum. There are also interesting implications for ecological diversification, but this aspect of the paper is written without enough explanation or interpretation of data, as I will detail. Also, I think most microbial ecologists would be amazed to see a paper on diversification within "a single population" that is actually not a population at all, but rather a fair chunk of a genus.
It is so inappropriate to call the S-LLPA lineage a "single population," since it has nearly 20% sequence divergence within it, and because there is clearly profound ecological diversification within this group. (Given that the Eren group has made tremendous progress toward discovering newly divergent, ecologically distinct populations of bacteria with their MED algorithm, it seems ironic that the group is now calling this phylogenetically huge group a single population.) Calling this group a population seems to have led to the conclusion that "their broad geographic prevalence suggest<s> dispersion is not a limiting factor for SAR11 in surface seawater…." But this conclusion is based on a false reification of all the various true populations within what the authors are calling a population.
The authors talk about S-LLPA offers "a unique opportunity to study the genomic diversity and evolutionary genomics of a single marine microbial population…." Not so unique when the "population" is a huge chunk of a genus.
The authors do justify their calling this clade a population because it constitutes what is recruited by a single isolate from various metagenomes. But their own work clearly shows, to my mind, that this is not a good way to discover populations.
The authors have used their Deep Learning algorithm for demarcating six phylogenetic groups, which they call proteotypes, from their S-LLPA lineage. It is frustrating that the authors neglected to give any rationale for their approach. Is it based on sequence clustering of shared genes? Is it based on genome content sharing? Or both? (Even though the algorithm has been published, they should say something briefly about what the inputs are and how it works.)
On two occasions the authors write about protein sweeps. They state that the sweeps are "rare." I think most evolutionary ecologists of bacteria would want to know how rare, and in which genes. Also, it would be important to indicate whether the sweeps traveled across ecologically distinct groups. In the first paragraph of the Discussion, the authors mention that there are more sweeps in warm currents, but this should be fleshed out and made clearer.
Subsection “Purifying selection governs the identity of amino acid substitution types”, first paragraph. It wasn't clear to me whether the authors were claiming that their focus group was unique in having a stable proportion of amino acids on a global scale, or whether other groups are showing the same pattern.
In the subsection “Purifying selection governs the identity of amino acid substitution types”, I don't see how the authors are finding evidence of purifying selection. Rather, it seems they are finding the constraints on adaptive substitutions of amino acids. That's different from purifying selection, which is usually the evolutionary stability of a particular sequence.
It's interesting that there are two clades within S-LLPA that are associated with different temperature regimes. And it's interesting that the subclades within these clades are different in the geographic regions where they are most abundantwith similar temperatures. The interpretation of this result depends on whether different cold-adapted subclades are never found together (meaning that they may have no adaptation differences) or whether they sympatric to some extent (meaning that they are coexisting based on ecological differences other than temperature partitioning). It seems that the latter is the case, but the authors should have made this clearer. For example, pie charts showing the relative abundances of the subclades in each region would be useful.
Reviewer #3:
The work by Delmont et al. describes a large-scale and thorough analysis of an important marine bacterium population, SAR111. They use multiple approaches to identify signals of selection to understand the evolution and ecology of these microbes. The authors deserve credit for their very clear presentation of data and methods. However, I have multiple concerns regarding the analysis and interpretation of the data:
1) The nature, and strength of, selection pressures acting on this bacterial population are not clearly defined.
a) The description of the substitution analysis "suggests a powerful influence of purifying selection", while also "suggesting a role for adaptive processes for functional diversification of S-LLPA proteins". Which of these dominates? Could this just be noisy neutral evolution – what is the expected variation of the S statistic, and the SAAV patterns, under a neutral scenario?
b) The neutral model of Hellweger et al. still predicts geographic separation of strains, which should be made clear in the Introduction. A falsification of this would be a repeated association of bacterial strains with ecological niche. This appears to be the case at the highest level of the hierarachy inferred with "deep learning", but at lower levels, predictors are genetic, not ecological, and therefore likely represent characteristics that were identified by the clustering algorithm itself. This might be expected under a neutral model.
2) The authors have opted against traditional population genetics methods. It is possible that the new methods are a substantial improvement, but they need to be justified. Some examples:
a) The authors justify using the "S" statistic rather than d(N)/d(S) based on there being many instances of multiple substitutions within the same codon. But this is exactly what d(N)/d(S) is meant to correct for, and S does not capture – there may be many synonymous substitutions on an evolutionary pathway, but if only one of them is non-synonymous, then S will only count this only as a non-silent change, underestimating purifying selection. What is the neutral expectation for S?
b) The BLOSUM matrices are used as "as a proxy to assess the functional difference between the pairs of amino acids", which are then compared to the substitution frequencies – but this is circular reasoning, as these matrices are calculated from substitution frequencies. The versions used in the paper are also inappropriate for this dataset, as they were calculated using more closely-related sequences than are compared in this paper.
c) "Deep learning" is applied to cluster populations together through a method designed without genetic data in mind. This does not mean it is wrong, but as it is not an intuitive approach, it would be helpful to see validation against a more standard method, such as the fixation index, F(ST). This would help understand the distinct properties of the six subclusters – for instances, proteotype D looks to be a single successful "strain" – is this expected to be picked up as a signal by the "deep learning"?
3) Quality of read mapping. Many of the sequences being compared are highly divergent, but most mapping software is intended to align reads to highly similar reference sequences. While there is a promising correlation between the SNP densities identified by BWA and BOWTIE2, there is a greater than two-fold difference in the absolute densities. This suggests a very high false positive or false negative rate, depending on the method. The authors need to validate the performance of these algorithms when using highly divergent reads. Additionally, what is the justification for using the "the base-5 logarithm of the mean coverage of a gene remained within {plus minus}1 of that for the mean coverage across all genes" for assessing consistency of coverage?
[Editors’ note: what now follows is the decision letter after the authors submitted for further consideration.]
Thank you for submitting your article "Single-amino acid variants reveal evolutionary processes that shape the biogeography of a global SAR11 lineage" for consideration by eLife. Your article has been further reviewed by three peer reviewers, including Paul B Rainey as the Reviewing Editor and Reviewer #1, and the evaluation has been overseen by Detlef Weigel as the Senior Editor. The following individual involved in review of your submission has agreed to reveal his identity: Fred Cohan (Reviewer #2).
The reviewers have discussed the reviews with one another and the Reviewing Editor has drafted this decision to help you prepare a further revised submission.
All reviewers appreciate and acknowledge the significant work that has gone into the paper during the course of the revision, the reviewers are all equally enthusiastic about the paper. However, there remain issues that need attention in order for the paper achieve the impact that it deserves. The reviewers' comments are pasted below, with the collective comments boiling down to a request to supplement the deep learning analysis with a more standard evolutionary approach in order for the reader to know whether the results are consistent with what most people in the field know. Here it is important to clearly distinguish between neutral evolution, purifying selection, and adaptive divergence. It is the latter that is particularly relevant to addressing the question of the possible ecological distinctions among populations.
Collected reviewer comments:
The paper is vastly improved since the original version. It is now much clearer and more compelling, and the paper deals with the issue of populations better.
The paper is fascinating for showing that whatever cohesion occurs at >95% ANI in pretty much every other bacterial group does not occur here in SAR11. The paper is also really interesting for giving a robust and sensitive way to identify ecologically distinct metagenome groups from sequence data.
My concerns are mostly focused on what the authors have found out about ecological diversity within the 1a.3.V lineage.
First, I appreciate that the authors are trying to be more careful about using the concept of population, but there are still places where they are still unclear about what they mean. The authors write of "remarkable intra-population genomic diversity…." It's not clear exactly what the authors mean by "population" here, but in this context what they mean is very important. If there isn't a good definition of population being used, then how can one talk about one population's intra-population genomic diversity being higher than another? It all depends on what you mean by population, and that's not clear here.
Figure 2 purports to show that there are not sequence-discrete populations within 1a.3.v, at least not in the sense that Kostas Konstantinidis demonstrates them in his studies, most recently in Jain et al., 2018. It is really interesting that all the SAR11 clades (with just this one clade shown in Figure 2) do not show the drop-off in recruitment of metagenome sequences below about 95% ANI. The authors argue that part of the great sequence diversity of what is recruited into this lineage (that is with much lower ANI) emerges from there being ecological diversity within the lineage (adaptations to different temperatures among different sublineages). I don't think this is a reasonable explanation, as almost every species taxon, nearly all obeying the 95% ANI drop-off rule, is also ecologically heterogeneous. I have suggested, in an interpretation of the recent Jain et al. paper, that the cohesion holding a species taxon together stems from an ability of recombination to act frequently at >95% ANI, plus the possibility of adaptive genes passing between ecologically distinct populations that are >95% ANI (Cohan, 2019) I'd argue that there is more potential for either recurrent recombination to limit divergence among ecologically distinct populations in SAR11, or greater opportunities for a single gene to be adaptive across different populations.
I'd like to add that whatever cohesion occurs at >95% ANI in most species taxa does not address the ecological diversity within species taxa, or whether there are sequence-discrete populations within each taxon. In fact, most species taxa have sequence-discrete and sequence-discoverable ecologically distinct populations. (This is the point of the second half of my Current Biology dispatch I mentioned.) So, I don't think that Figure 2 makes an argument that there are not sequence-discrete, ecologically distinct populations within the focus lineage.
The authors have noted that there is a small minority of genes that are invariant at the amino acid level across a given metagenome. In the earlier version, they interpreted these observations as evidence of sweeps, but here they have chosen not to address the dynamics that can be inferred by these invariant genes. I think that such single-gene sweeps are very interesting, and have been the topic of discussion of some very interesting papers, including papers by Jesse Shapiro and by Rex Malmstrom. I'd encourage the authors to bring this discussion back. I'll mention that these instances provide evidence that a generally adaptive gene has passed by recombination across all the ecologically distinct populations within a lineage, but I wouldn't expect the authors to necessarily buy in to that interpretation.
I found the authors' partitioning of metagenomes into ecologically distinct groups fascinating. And it's particularly interesting that these groups could not be revealed (at least entirely) by analyzing single reads (as shown in Figure 4—figure supplement 3). I'll encourage the authors to venture from their interpretation regarding whole metagenomes to inferring that there appear to be multiple ecologically distinct populations that cause these metagenomes to hold different niches. What might be the ecological distinctions of the populations? Clearly they are different for their temperature adaptation, as revealed by Figure 4 (mislabeled Figure 5). Perhaps the authors could glean something about what is different about the constituent populations from their Figure 4—figure supplement 3, that is by comparing the clusters that they do find with known environmental parameters.
Re the concluding statement about "everything is everywhere," I'd temper that by saying 'at least for marine bacteria'. This is because the statement does talk about "everything," so I think there needs to be some limitation to the conclusion.
Concerns about evolutionary analyses:
In response to point (1), the authors state:
"Simply, we observed that certain amino acids (mostly hydrophilic), and most notably, few AASTs (e.g., alanine/isoleucine) predominated in our SAAV table."
It has been well-established for decades that hydrophobic amino acids in solvent-inaccessible positions are more conserved (e.g. see reviews cited in the Introduction to Ramsey et al. 2011 Genetics 188(2):478-88). Reproducing this observation in the SAR11 dataset simply confirms that purifying selection can be observed over long timescales. It does not help identify what the changes are that enable ecological adaptation.
Delmont et al: "These points are interesting, but they do not favor natural selection over neutral evolution. Instead they provide information regarding permissible versus non-permissible diversification"
I assume "permissible" refers to purifying selection? Which is an example of natural selection – rather than random, neutral diversification. It is distinct from the adaptive/Darwinian evolution that allows the ecological differentiation of the bacteria. Not separating neutrality, purifying selection and adaptive evolution plagues the whole manuscript. The authors have a strain distribution that appears to reflect ecological differentiation, rather than neutral diffusion. They have a mutation distribution that mainly seems to reflect purifying selection, rather than neutral evolution. Some analyses (e.g. below) suggest adaptive evolution, which is potentially very interesting, but the overall trend is consistent with purifying selection. The authors need to clearly distinguish their different conclusions.
Delmont et al: "we determined that thousands of 1a.3.V allele frequency trajectories correlated with in situ temperature, in line with the biogeography of proteotypes"
It is not surprising that many of the variable sites correlate with temperature, because the proteotypes are defined using the variable sites, and the proteotypes correlate with temperature. The authors should seek to synthesize these data – do the variable sites have properties that suggest selection between niches? Do they concentrate in particular genes that might drive adaptation to different niches? Or do they simply correlate with the population structure?
Overall, I return to my original point from the first round of reviews: is the diversification mainly neutral; dominated by purifying selection; or dominated by adaptive evolution? The most interesting signals may not be from the dominant evolutionary process, of course.
In response to point 2, the authors state:
Delmont et al: "As far as we know, there is no published study that effectively takes into account multiple SNVs per codon without calculating the exact codon frequencies to estimate synonymity accurately…SNV density was high for 1a.3.V and many SNVs co-occurred in the same codon, rendering classical d(N)/d(S) analyses limited, which had never been challenged with extremely complex environments, if not completely irrelevant."
Yang (2007) MBE 24(8):1586-91 – with over 6,000 citations – describes how baseml can be used to calculate d(N)/d(S) using base substitution, rather than codon substitution, models. This accounts for multiple substitutions per codon, and provides a statistical test for evidence of selection versus neutrality, unlike the methods presented in the current version. It does not matter how complex the environments are – indeed, d(N)/d(S) has been applied to the question of SAR11 adaptation to different ecologies by Brown et al., 2012 and Luo and Hughes, 2012 Mol. Syst. Biol. 8:625. The authors should at least apply standard methods to the assembled genomes, based on the ecological separation they have determined. Methods also exist for calculation of this statistic from metagenomic data, and might be more informative.
Delmont et al: "The significant shift in SAAVs/SNVs ratio between cold currents and warm currents is of relevance to our study, especially in light of other insights (especially, the linkage between allele frequency trajectories and in situ temperature, and the biogeography of proteotypes), favors the predominant role of natural selection acting on the permissible amino acid diversification traits of this global SAR11 lineage."
The correlation between allele frequencies and temperature, and proteotype and temperature, is confounded by the correlation between proteotypes and allele frequencies. This should be explained (if this is wrong, please also make that clear in the text). The authors need to make clear whether the natural selection they are referring to is stronger/weaker purifying selection changing the SAAV/SNV ratio; or positive selection driving adaptive diversification increasing the SAAV/SNV in some populations.
Delmont et al: "Regarding the fixation index, we agree with the reviewer that this would have been a valuable addition to our work…We believe the significant amount of methodological development in this work that offers descriptions of the biogeography of the most abundant lineage in surface oceans conveys a story that will not benefit from the inclusion of additional analyses. "
I don't understand how this statistic can be "a valuable addition", yet the story "will not benefit from the inclusion of additional analyses.". The deep learning analysis is still not intuitively explained in the new version (not the authors' fault, as these methods are designed to be complex). It should be complemented by fixation index calculations, which are simple, and would allow the readers a much greater insight into the basis of the results.
Contributor Information
Paul B Rainey, Max Planck Institute for Evolutionary Biology, Germany.
Paul B Rainey, Max Planck Institute for Evolutionary Biology, Germany.
Fred Cohan, Wesleyan.
Author response
[Editors’ note: the author responses to the first round of peer review follow.]
Reviewer #1:
This deals with the causes of patterns of diversity in a widespread marine microbe. The data come from a set of contigs assembled from shot gun sequencing of 21 genomes onto which are read-mapped metagenomic data from 103 samples. The focus is a particular clone that is particularly abundant in southern samples. A core genome is assembled from the metagenomic data and this is interrogated for signatures of selection using a curious approach based on physico-chemical properties of amino acids. A further step looks for correlations with water currents.
At first glance I found the paper reasonably clear, but the closer I read, the more murky things became. In fact I am not too sure what the authors have really done and I am not persuaded that their main claim, that selection is responsible for biogeography in S-LLPA, is supported by their data. Overall the level of explanation is insufficient to allow the reader to understand what has been done.
We thank the reviewer for their interest in our study. We agree with the reviewer’s criticism regarding the lack of clarity of our initial submission, which made it difficult for readers to follow our analyses and findings. These concerns prompted us to go back to the drawing board and improve the clarity of our manuscript as well as substantiate our claims with new analyses.
Our revised manuscript includes a simplified terminology, an entirely re-written Introduction, and a vastly modified Results section that clarifies both the question we set out to investigate, and the novelty of the approaches we developed to address it. It also includes new analyses to support our main claim. These analyses include (1) the percent identity distribution of recruited short metagenomic reads for each SAR11 genome, (2) the investigation of the relative frequency of amino acids involved in SAAVs, to demonstrate the link between purifying selection and hydrophobicity, and (3) the identification of thousands of SAAV allele frequency trajectories correlating with in situ temperature. One critical point is that we now follow more common naming conventions for SAR11 clades and sub-clades. Thus, in our revision we now define six sublineages within 1a.3 by linking ecology, phylogeny, and gene content of available SAR11 genomes, and replace the term S-LLPA with 1a.3.V.
Overall, these new analyses support our main claim that environmentally-mediated selection is a strong determinant of SAR11 evolution and biogeography by providing more evidence for the linkage between SAAVs and in situ temperature at the highly resolved level of allele frequency trajectories for individual codon positions across metagenomes.
Among the difficulties:
Title / Abstract: The title states that biogeography is governed by selection, but in the Abstract the authors use more temperate language. So I guess they are not so sure. The Abstract claims that systematic purifying selection and adaptive mechanisms governing non-synonymous variation have been identified, but at best what they have are signatures consistent with expectations. I disagree that analysis of sequence data reveals different niches (it may reveal the existence of ecotypes that may be indicative of distinct niches). What is a proteotype?
We have replaced “natural selection” by “environmentally-mediated selection” throughout the manuscript to clarify our findings, and incorporated new analyses corroborating the role of selection in the global biogeography of 1a.3.V. We have also changed our title to better reflect our findings.
We define a proteotype as homogeneous configuration of environmental single-amino acid variants in the context of a microbial lineage we access through a reference genome and metagenomic read recruitment. We have defined the six ‘proteotypes’ in our revised manuscript from sets of metagenomes that are clustered together based on environmental single-amino acid variant profiles. Thus, these proteotypes correspond to oceanic regions that shared similar amino acid variations for 1a.3.V. Respecting the controversy surrounding the term, we adopt a simple definition of ‘niche’ as in “the fit of a [population] living under specific environmental conditions” (10.1007/978-94-017-9014-7_26). If proteotypes reflect environmental conditions, which is the main premise of our study, as they reflect oceanic current temperatures, we do not see why the sequence data analyses would not be insufficient to distinguish niches. Hence, we prefer to keep the term in our manuscript, and hope the reviewer agrees with our resolution.
Place of isolation of the focal 21 SAR11 genomes is important in a study of biogeography, but no attention has been given to this.
We agree with the reviewer that it is an intuitive consideration that we should have been more careful in addressing. Our analyses show that the location of isolation (coastal Hawai’i) has very little relevance to the biogeography and population genetics of the genome. 1a.3.V is not particularly more prevalent, or less diverse in samples closer to the location of isolation. We now clarify this point in two sections of the text:
“Strain HIMB83 contains a 1.4 Mbp genome with 1,470 genes, and was isolated from coastal seawaters off Hawai’i, USA. Despite this, it recruited more reads from distant locations than from samples closest to the source of isolation (Supplementary file 1C).”
“The SAAV density (the percentage of codon positions that harbor a SAAV) of core 1a.3.V genes averaged 5.76% and correlated with SNV density (19.3% on average) across the 74 metagenomes (R2=0.89; Figure 1—figure supplement 1). SNV and SAAV density metrics did not decrease in metagenomes sampled closest to the source of isolation, suggesting that the location of isolation for strain HIMB83 does not predict the biogeography and population genetics of 1a.3.V.”
Our revision also includes a new analysis that summarizes percent identity statistics of all metagenomic reads recruited by HIMB83 to substantiate this observation.
There are many challenges in drawing conclusions from metagenomic data via read-mapping to a reference. Particularly problematic is the fact that diversity of closely related organisms within a sample is not known. Thus when many SNPs are detected is this indicative of a diverse population, or is it a signature that comes from a single over-represented clone that is rather divergent? Similarly, the authors take high similarity as signature of a recent sweep, but this might reflect a single over-represented genome from a closely related organism. I'm not sure how the authors dealt with these and similar problems.
We thank the reviewer for their insightful question and an opportunity to clarify this point, which in fact is an important aspect of our study. While we rely on reference genomes for metagenomic read recruitment, our analyses of genetic heterogeneity in the environment through singlenucleotide variants (SNVs) and single-amino acid variants (SAAVs) rely on the consensus of the environment rather than divergence from the reference sequence. In other words, we established our SNVs and SAAVs based on the information content within a metagenome, rather than by calculating distance between a metagenome and a reference genome. Thus, if there was a single over-represented clone in the environment as the reviewer suggests, there would have been no SNVs or SAAVs identified from that metagenome: despite the disagreement between that clone and HIMB83, the environment would reveal subtle variation within the clone. In contrast, we found many variants and remarkable diversity that differed across metagenomes, which allowed us to to place this variation in the context of geography. The new Figure 2 in our revised manuscript substantiates this point.
Sweeps could occur at the genome-level causing over-representation of a single genome (which we did not observe for SAR11 in any of the metagenomes analyzed here) or at the gene-level when all closely related members of a diverse lineage share the same gene (which we also rarely observed). Thanks to SAAVs, we also had the opportunity to study conservancy of genes at the level of amino acid sequences. These analyses also suggested a lack of sweeps, and instead revealed the systematic occurrence of different variants in sets of metagenomes that originated from distant geographic locations. These two observations support the hypothesis that there was not a single over-represented clone in any of the samples analyzed here.
With regard to selective sweeps, the results of the analysis appear not to be given (subsection “SNVs to SAAVs: Accurate characterization of non-synonymous variation”, gives the conditions that need to be fulfilled in order for a protein to have undergone a sweep, but not the results).
Supplementary file 2D describes SAAV density for each gene across the 74 metagenomes; values of zero indicate no variation in the environment. The reviewer’s comment prompted us to be more careful with the terminology we use to describe this particular observation, which is the lack of variation rather than an event of gene or protein sweep, which requires more evidence than what our data can afford. Our Figure 5 now summarizes the percentage of invariant proteins across proteotypes and, to address this particular reviewer concern, we extended the section where invariant proteins are described with the following addition:
“We considered a protein to be ‘invariant’ (i.e., absence of variation due to intensive purifying selection acting on introduced mutations) in a given metagenome if it lacked SAAVs; such invariant proteins were rare in our data. In total, we detected 2,548 invariant proteins (only 4.3% of all possibilities across the 74 metagenomes) that encompassed only 113 genes. In addition, all genes, except one 679 nucleotide long ABC transporter (gene id 1469), contained at least one SAAV in at least one metagenome, revealing a wide range of amino acid sequence diversification among core 1a.3.V proteins (Supplementary file 2D).”
Patterns of amino acid substitution types seems an interesting way to go, but this needs to be unpacked, explained and justified.
We thank the reviewer for their guidance. AASTs indeed effectively summarize the multidimensional information the complex SAAV table carries. We now have significantly edited the related section for clarity:
“Next, we sought to investigate amino acids that co-occur in variable sites. SAAVs were often dominated by a few amino acids; hence, the frequency vector for a given SAAV contained many zero values. […] Expectedly, ‘glycine/tryptophan’ was exceedingly rare in our data and occurred only once in 738,324 SAAVs (Supplementary file 2H).”
In addition, where we investigated the correlation between AASTs and temperature, we described our results the following way, further justifying its relevance:
“Within subclade 1a.3.V the distribution of AAST frequencies was notably constrained across geographies (Figure 3B). […] Yet, close inspection of AAST frequencies revealed that amino acid exchangeabilities subtly diverge in a pattern that correlates with water temperature and/or its co-variables (Figure 3B insets, Figure 3—figure supplement 2).”
Finally, we relied on AASTs to clarify temperature-associated exchange rates between amino acids in AASTs, which further explains their use:
“The results of this analysis also provide an opportunity to study the directionality of exchange rates of AASTs. […] The most statistically significant asymmetry was between the most common AAST, isoleucine and valine, in which valine was highly preferred in warmer waters (binomial test p-value: 5.7×10-6) despite chemical structure similarities.”
Partitioning of SAAV between warm and cold currents: there seems to be a correlation with clustering based on SAAVs, but as above, I need to have more explanation. Much relies on the robustness of the SAAV profile clustering (Figure 3A) and there is no indication of its statistical likelihood.
Here we reanalyzed our data using also a more conventional approach rather than Deep Learning analysis to demonstrate that our findings are preserved even with a different clustering strategy. Deep Learning provided us with a distance matrix that estimates the dissimilarity between 74 metagenomes based on their SAAV profiles, and a hierarchical clustering of these data enabled us to identify 6 clusters. To confirm this outcome was not a by-product of our bioinformatics approach, we also performed an analysis of the SAAV table using Euclidian distance and Ward’s linkage. The figures in Author response images 1 and 2 shows the two main clusters, which are identical to the two main clusters Deep Learning revealed.
Asking for 6 clusters also provided us with near identical organization of metagenomes:
We favored the Deep Learning approach since its unbiased distance estimation resulted in more highly-resolved branches in the resulting dendrogram.
To further link the clustering of metagenomes based on SAAV profiles, and the underlying sequence composition, in our revised Figure 4A we added the percent identity curves of recruited reads at the bottom of the dendrogram. The strong association between percent identity curves and clusters indicate that our SAAV clustering approach was able to infer the signal that underpins the differentiation among these metagenomes in the context of a single lineage:
We hope these additional insights give the reviewer more confidence in the patterns we observe, and adequately demonstrate that these results are not due to an algorithmic anomaly.
The crunch of the paper is rejection of the neutral model, but this is done by simply saying that neutral models could not explain the observed patters of amino acid substitutions, but no evidence is provided. Also, Figure 4, which I hoped would enlighten me, does not exist.
We regret that Figure 4 was not available to the reviewer:
We hope the remarkable differences between the neutral model and the clusters identified through our analysis (most strikingly the group D, C, and E that connect distant geographies) offers the reviewer better insights.
Our new analysis of allele frequency trajectories adds further statistical support to our claim, corroborating the biogeography of proteotypes. We wrote:
“In addition to considering patterns of variability that emerged when we pooled data across 37,416 codon positions exhibiting variation within the core 1a.3.V genes, we also investigated the allele frequency trajectories of individual positions (i.e., the relative frequency between the two most prevalent amino acids across the 74 metagenomes) and sought to identify those that correlate with in situ temperature and/or its co-variables. […] It is therefore most plausible to conclude that allele frequencies at these positions are predominantly shaped by environmentally mediated selection.”
And we now end the Results and Discussion section with:
“The striking connection between geographically distant regions of the oceans through SAAVs render neutral processes an unlikely candidate for evolutionary processes that maintain the genomic heterogeneity within 1a.3.V (Figure 4—figure supplement 5). […] Overall, these results indicate that environmentally-mediated selection is a strong determinant of SAR11 evolution and biogeography.”
In general I found the figures overly dense and very difficult to understand given the paucity of explanation in the captions. Take Figure 1: latitudinal gradient, I presume north is at the top? Colouring seems inconsistent and so on.
We thank the reviewer for their attention. We now have clarified the latitudinal gradient in Figure 1 (the reviewer was correct in their guess: the organization of metagenomes top to bottom followed North to South). We have also tried to strategically use color to enhance the clarity of new figures incorporated into the resubmission.
We agree with the reviewer that some of the figures are dense with information. We have dedicated considerable time to create them so that they could convey relevant information from our holistic analyses (e.g., the SAR11 metapangenome in Figure 1A) and the new concepts introduced in this study (e.g., SAAVs in the context of inferred protein structures in Figures 3C and 4A). Carefully selected main figures cover significant aspects of the study, and altogether support our main claim that environmentally-mediated selection plays a role in governing the global biogeography of SAR11.
We thank the reviewer for their efforts to evaluate our study and for their helpful insights.
Reviewer #2:
It's hard to tell who this paper is written for. The bioinformatics applications are the most interesting: identifying the core genome of a lineage of interest from metagenomics data, using a novel algorithm for (somehow) identifying significant subclades within the lineage, and finding a surprisingly high frequency of certain amino acid substitutions that are not predicted by Blosum. There are also interesting implications for ecological diversification, but this aspect of the paper is written without enough explanation or interpretation of data, as I will detail. Also, I think most microbial ecologists would be amazed to see a paper on diversification within "a single population" that is actually not a population at all, but rather a fair chunk of a genus.
It is so inappropriate to call the S-LLPA lineage a "single population," since it has nearly 20% sequence divergence within it, and because there is clearly profound ecological diversification within this group. (Given that the Eren group has made tremendous progress toward discovering newly divergent, ecologically distinct populations of bacteria with their MED algorithm, it seems ironic that the group is now calling this phylogenetically huge group a single population.) Calling this group a population seems to have led to the conclusion that "their broad geographic prevalence suggest<s> dispersion is not a limiting factor for SAR11 in surface seawater…." But this conclusion is based on a false reification of all the various true populations within what the authors are calling a population.
We are very thankful for the reviewer’s criticism. In fact, their comments and concerns motivated us to perform new set of comprehensive analyses that helped us improve our intellectual and technical approaches and led to significant improvements in our manuscript. We agree with the reviewer the occurrence of multiple sequence-discrete populations would have influenced our conclusions dramatically, although our new analyses suggest that it is not the case. The new Figure 2 provides new insights into the percent identity of reads core 1a.3.V genes recruited across the 74 metagenomes. These results show that the data suggest the absence of various true populations within the niche of 1a.3.V, and much more clearly reveal the complex nature of this lineage, which was also observed in other studies that investigated sequence-discrete SAR11 populations (we cited multiple of these studies in our new introduction).
We agree with the reviewer that the use of the term “population” to describe 1a.3.V without extensive justification was an oversight on our part. However, we respectfully disagree with the reviewer’s suggestion that the metagenomic reads we competitively recruit through the HIMB83 genome represent a fair chunk of a genus. There is no consensus among microbiologists on what the appropriate theoretical definition of a population should be. While there are considerably wellsupported computational attempts to offer operational definitions for practical conveniences, SAR11 is a well-known and previously documented outlier of these operational definitions (our new introduction makes a more appropriate attempt to cover the literature). Where does a genus start and end? In the absence of an appropriate theoretical definition for a population, the boundaries between different levels of taxonomy are subject to interpretation. We believe, however, that most microbiologists and microbial ecologists would agree that a genus describing multiple ‘species’ must contain multiple sequence-discrete populations (SDPs). In other words, it is conceivable to expect that if metagenomic reads we recruit from the environment using HIMB83 represent a fair chunk of a genus, then there should be multiple SDPs that can be inferred from their levels of identity. However, what we found in our data was a lack of any evidence for SDPs. In fact, the recruited reads showed a much broader identity distribution pattern, similar to what is proposed for SAR11 by leading scientists in the field. Thus, our new short-read-level analyses do not support the hypothesis of the presence of multiple SDPs in our dataset. But ultimately, after much consideration we elected to not use the controversial term ‘population’. Our data gives access to a large number of environmental cells that differ from each other rather dramatically, but not randomly: in a cloud of sequences that can’t be distilled into multiple SDPs, disagreements across the genomes that make up this cloud emerge from very specific locations of genes without the presence of individually abundant clones. Our study distills that information to show that amino acid sequence-level diversification connects geographically distinct stations, and we accomplish this by focusing on a prevalent and abundant subclade of SAR11 that has a single cultivated representative.
We have extensively modified our text to better introduce and describe our work. We thank the reviewer for their insights, and hope that our new framework satisfies the reviewer.
The authors talk about S-LLPA offers "a unique opportunity to study the genomic diversity and evolutionary genomics of a single marine microbial population…." Not so unique when the "population" is a huge chunk of a genus.
We have removed this sentence as a part of the changes described above.
The authors do justify their calling this clade a population because it constitutes what is recruited by a single isolate from various metagenomes. But their own work clearly shows, to my mind, that this is not a good way to discover populations.
Based on the reviewer’s comments we have modified the wording accordingly. HIMB83 provided the opportunity to study an abundant and widespread SAR11 lineage (1a.3.V) that genomeresolved metagenomics has thus far failed to provide access. We do agree that read recruitment to isolate genomes can in principle lead to mistakes due to non-specific recruitment; however, we have taken care to guard against this error and show that our approach offers valuable insights when sufficient samples of a population’s genomes are unavailable. Our gene-level recruitment results and the reliance on the core genes of this lineage minimize shortcomings of the use of isolate genomes to support our claims.
The authors have used their Deep Learning algorithm for demarcating six phylogenetic groups, which they call proteotypes, from their S-LLPA lineage. It is frustrating that the authors neglected to give any rationale for their approach. Is it based on sequence clustering of shared genes? Is it based on genome content sharing? Or both? (Even though the algorithm has been published, they should say something briefly about what the inputs are and how it works.)
This important point requires clarification. We first identified 738,324 SAAVs across the 799 core 1a.3.V genes and 74 metagenomes. We then simplified our data by taking into account two most frequent amino acids in each SAAV. To explain our reasoning we have added the following description to the text:
“SAAVs were often dominated by a few amino acids; hence, the frequency vector for a given SAAV contained many zero values. To reduce sparsity, we first simplified our data by associating each SAAV with an amino acid substitution type (AAST), defined as the two most frequent amino acids in a given SAAV.”
We have also clarified the data used by the Deep Learning algorithm (we regret the poor description in our initial submission) and further explained the Deep Learning approach. The relevant section now reads:
“We then sought to extend the concept of allele frequency tracking at individual SAAV positions to investigate the potential for large-scale geographic partitioning within the metagenome dataset. […] Hierarchical clustering of samples based on Deep Learning-estimated distances (Supplementary file 4A) resulted in two main groups: the Western (warm) and Eastern (cold) boundary currents (Figure 4A).”
On two occasions the authors write about protein sweeps. They state that the sweeps are "rare." I think most evolutionary ecologists of bacteria would want to know how rare, and in which genes. Also, it would be important to indicate whether the sweeps traveled across ecologically distinct groups. In the first paragraph of the Discussion, the authors mention that there are more sweeps in warm currents, but this should be fleshed out and made clearer.
We agree with the reviewer that protein sweeps and their linkage to ecologically distinct groups and functions are of particular interest. We have modified the relevant section for clarity and included in our revision a new supplementary table that describes additional statistics:
“We considered a protein to be ‘invariant’ (i.e., absence of variation due to intensive purifying selection acting on introduced mutations) in a given metagenome if it lacked SAAVs; such invariant proteins were rare in our data. In total, we detected 2,548 invariant proteins (only 4.3% of all possibilities across the 74 metagenomes) that encompassed only 113 genes. In addition, all genes, except one 679 nucleotide long ABC transporter (gene id 1469), contained at least one SAAV in at least one metagenome, revealing a wide range of amino acid sequence diversification among core 1a.3.V proteins (Supplementary file 2D).”
While we are happy to see the reviewer finds this of interest, we believe protein sweeps do not represent significant aspects of our study. Yet the data we have made available provide access to the occurrence of invariant proteins in the context of metagenomes (Supplementary file 2D) along with extensive information on metagenomes (Supplementary file 1A) as well as each gene (Supplementary file 1J). We hope the reviewer agrees with our allocation of the limited manuscript space.
Subsection “Purifying selection governs the identity of amino acid substitution types”, first paragraph. It wasn't clear to me whether the authors were claiming that their focus group was unique in having a stable proportion of amino acids on a global scale, or whether other groups are showing the same pattern.
We have revised the section in question to improve its clarity using our new analyses:
“Within subclade 1a.3.V the distribution of AAST frequencies was notably constrained across geographies (Figure 3B). For example, the relative standard deviation of ‘aspartic/glutamic acid’ frequencies across the 74 metagenomes was just 3.0%, and the statistical spread of other AASTs was comparable (Figure 3B). This suggests that there exists some evolutionary mechanism(s) maintaining amino acid exchangeability within subclade 1a.3.V throughout the global surface ocean.”
Our study does not intend to make a claim regarding how unique observed patterns are to microbial taxa. However, we find the question posed by the reviewer an interesting one.
In the subsection “Purifying selection governs the identity of amino acid substitution types”, I don't see how the authors are finding evidence of purifying selection. Rather, it seems they are finding the constraints on adaptive substitutions of amino acids. That's different from purifying selection, which is usually the evolutionary stability of a particular sequence.
We thank the reviewer for bringing up this point. Our revised study includes significant changes to clarify our observations.
Purifying selection, the selective removal of alleles that are deleterious, indeed does not impact permissible amino acid substitutions, but we found evidence that purifying selection shapes the relative frequency of amino acids involved in SAAVs as a function of hydrophobicity, which is supported by the existing literature:
“Hydrophobic interactions within the solvent inaccessible core of proteins are known to be critical for maintaining the stability required for folding and activity, which enforces a strong purifying selection placed on mutations occurring in buried (solvent inaccessible) positions (Bustamante, Townsend and Hartl, 2000; Chen and Zhou, 2005; Worth, Gong and Blundell, 2009). […]Overall, our compositional analysis revealed that the occurrence of amino acids in SAAVs is roughly correlated with the occurrence of amino acids within the core 1a.3.V genes, and that deviations from this expectation are driven in part by levels of purifying selection that depend upon the suitability of an amino acid’s hydrophobicity for a given physicochemical environment (Figure 3—figure supplement 1).”
Besides the relative proportion of amino acids involved in SAAVs, our analysis of inferred 3D structures for hundreds of core 1a.3.V genes provides additional insights into the apparent effect of purifying selection in the occurrence of SAAVs as a function of solvent accessibility:
“Within the subset of the 1a.3.V proteome accessible to us, we found that buried amino acids (0-10% relative solvent accessibility) were approximately 4.4 times less likely to be variant than those that were exposed (41-100% relative solvent accessibility) (ANOVA, pvalue: <2×10-16). […] The local physicochemical environment therefore shapes variation, and visual inspection of Figure 3C indicates that this is conserved across distant geographies; i.e. positions that vary in one metagenome are likely to vary in others, as well.”
Strong biases associated with hydrophobicity and solvent accessibility both expose a predominant role of purifying selection in observed amino acid frequencies in SAAVs within the members of SAR11 lineage 1a.3.V. We hope the reviewer appreciates our clarifications.
It's interesting that there are two clades within S-LLPA that are associated with different temperature regimes. And it's interesting that the subclades within these clades are different in the geographic regions where they are most abundantwith similar temperatures. The interpretation of this result depends on whether different cold-adapted subclades are never found together (meaning that they may have no adaptation differences) or whether they sympatric to some extent (meaning that they are coexisting based on ecological differences other than temperature partitioning). It seems that the latter is the case, but the authors should have made this clearer. For example, pie charts showing the relative abundances of the subclades in each region would be useful.
The reviewer brings up a very important point, which was of significant interest to us as well. However, our short-read level analyses suggested that an increased fitness to a given temperature regime does not yield sequence-discrete populations that are distinguishable, which prevents us from making quantitative estimates of their differential abundance across geography. Deconvoluting haplotypes found in metagenomic read recruitment results is possible thanks to the increasing availability of tools (i.e., Lineage, DESMAN, or ConStrains), however, none of them are suitable to resolve the extensive diversity we observe in SAR11 subclade 1a.3.V. We are looking forward to new contributions from single-cell genomics and cultivation to address this significant question our study could not contribute.
Reviewer #3:
The work by Delmont et al. describes a large-scale and thorough analysis of an important marine bacterium population, SAR111. They use multiple approaches to identify signals of selection to understand the evolution and ecology of these microbes. The authors deserve credit for their very clear presentation of data and methods. However, I have multiple concerns regarding the analysis and interpretation of the data:
1) The nature, and strength of, selection pressures acting on this bacterial population are not clearly defined.
We agree with the reviewer. The manuscript now better describes the nature and strength of selection pressures acting on 1a.3.V. Especially, we show that (1) hydrophobicity and solvent accessibility influences the strength of purifying selection acting on amino acids, and (2) few AASTs along with thousands of allele frequency trajectories significantly correlate with in situ temperatures, in line with the biogeography of proteotypes. Our response below clarifies these points further.
a) The description of the substitution analysis "suggests a powerful influence of purifying selection", while also "suggesting a role for adaptive processes for functional diversification of S-LLPA proteins". Which of these dominates? Could this just be noisy neutral evolution – what is the expected variation of the S statistic, and the SAAV patterns, under a neutral scenario?
This is an important consideration and requires a comprehensive clarification. Our revision no longer contains the sentences the reviewer cited, and better clarifies that our investigation of purifying selection acting on 1a.3.V is independent of the debate regarding neutral evolution versus natural selection. Simply, we observed that certain amino acids (mostly hydrophilic), and most notably, few AASTs (e.g., alanine/isoleucine) predominated in our SAAV table.
Regarding the amino acids, we now write:
“Hydrophobic interactions within the solvent inaccessible core of proteins are known to be critical for maintaining the stability required for folding and activity, which enforces a strong purifying selection placed on mutations occurring in buried (solvent inaccessible) positions (Bustamante, Townsend and Hartl, 2000; Chen and Zhou, 2005; Worth, Gong and Blundell, 2009). […] Overall, our compositional analysis revealed that the occurrence of amino acids in SAAVs is roughly correlated with the occurrence of amino acids within the core 1a.3.V genes, and that deviations from this expectation are driven in part by levels of purifying selection that depend upon the suitability of an amino acid’s hydrophobicity for a given physicochemical environment (Figure 3—figure supplement 1).”
Besides the relative proportion of amino acids involved in SAAVs, the analysis of inferred 3D structures for hundreds of core 1a.3.V genes provides additional insights into the apparent effect of purifying selection on the occurrence of SAAVs (role of solvent accessibility this time):
“Within the subset of the 1a.3.V proteome accessible to us, we found that buried amino acids (0-10% relative solvent accessibility) were approximately 4.4 times less likely to be variant than those that were exposed (41-100% relative solvent accessibility) (ANOVA, pvalue: <2×〖10〗^(-16)). […] The local physicochemical environment therefore shapes variation, and visual inspection of Figure 3C indicates that this is conserved across distant geographies; i.e. positions that vary in one metagenome are likely to vary in others, as well.”
Regarding the AASTs, we found that predominant ones corresponded to competing amino acids with similar properties, shedding a different light on purifying selection. We described our findings in our revision:
“In 738,324 SAAVs, we observed 182 of 210 theoretically possible unique AASTs at varying frequencies (Supplementary file 2H). […] Expectedly, ‘glycine/tryptophan’ was exceedingly rare in our data and occurred only once in 738,324 SAAVs (Supplementary file 2H).”
These points are interesting, but they do not favor natural selection over neutral evolution. Instead they provide information regarding permissible versus non-permissible diversification. On the other hand, analysis of the permissible SAAVs can shed light in the natural selection versus neutral evolution debate. This is now covered in subsequent sections of our revision to help the reader. For instance, we found that some AASTs, and thousands of allele frequency trajectories, significantly correlated with in situ temperature, suggesting an important role for natural selection. We first wrote:
“Within subclade 1a.3.V the distribution of AAST frequencies was notably constrained across geographies (Figure 3B). […] Yet, close inspection of AAST frequencies revealed that amino acid exchangeabilities subtly diverge in a pattern that correlates with water temperature and/or its co-variables (Figure 3B insets, Figure 3—figure supplement 2).”
We then found that for some AASTs, one amino acid tended to be avoured by temperature increases compared to its competing amino acid genome-wide. We added:
“The results of this analysis also provide an opportunity to study the directionality of exchange rates of AASTs. […] The most statistically significant asymmetry was between the most common AAST, isoleucine and valine, in which valine was highly preferred in warmer waters (binomial test p-value: 5.7×10-6) despite chemical structure similarities.”
Finally, we determined that thousands of 1a.3.V allele frequency trajectories correlated with in situ temperature, in line with the biogeography of proteotypes. We concluded:
“In addition to considering patterns of variability that emerged when we pooled data across 37,416 codon positions exhibiting variation within the core 1a.3.V genes, we also investigated the allele frequency trajectories of individual positions (i.e., the relative frequency between the two most prevalent amino acids across the 74 metagenomes) and sought to identify those that correlate with in situ temperature and/or its co-variables. In 2,740 of the 37,416 positions, the null hypothesis that no correlation with temperature exists was rejected (Supplementary file 3B; p-value < 0.01). Figure 3—figure supplement 3 illustrates example cases and correlation statistics per AAST. It is statistically implausible that such correlations with temperature could have arisen from neutral evolution, given that distant oceans share similar temperatures (Supplementary file 1A). It is therefore most plausible to conclude that allele frequencies at these positions are predominantly shaped by environmentally mediated selection.”
Regarding the last question of the reviewer: under a neutral scenario, SAAV patterns should have reproduced trends depicted in Panel C of this supplemental figure (patterns we observed are different, and favor natural selection due to the distant metagenomes sharing similar SAAV profiles, for which Proteotype D offers a remarkable demonstration), see Figure 4—figure supplement 5.
b) The neutral model of Hellweger et al. still predicts geographic separation of strains, which should be made clear in the Introduction. A falsification of this would be a repeated association of bacterial strains with ecological niche. This appears to be the case at the highest level of the hierarachy inferred with "deep learning", but at lower levels, predictors are genetic, not ecological, and therefore likely represent characteristics that were identified by the clustering algorithm itself. This might be expected under a neutral model.
In principle, the reviewer is correct. But we did not observe variation at the level of SAAVs that fit the neutral model, even at finer scales of evolutionary distance. At the level of two main groups (Western versus Eastern boundary currents) as well as at the level of individual proteotypes, the clustering of metagenomes based on differential occurrence of SAAVs favors natural selection (now introduced as environmentally-mediated selection). Proteotype D is a particularly good example connecting the Red Sea and two sides of the Panama despite considerable distances.
Proteotype C connects remarkably distant, yet polar regions of the ocean. Similarly, Proteotype E also connects distant locations. Neutral evolution alone could lead to such patterns, as indicated by the simulation (Figure 4—figure supplement 3, panel C).
To cover this point, the Introduction now reads:
“At the level of individual populations, a key simulation by Hellweger et al., 2014, showed that the intra-population sequence divergence that reflects the geographic patterns of distribution for SAR11 cells could emerge solely as a function of ocean currents, without selection (Hellweger et al., 2014).
And the Results and Discussion reads:
“The striking connection between geographically distant regions of the oceans through SAAVs renders neutral processes an unlikely candidate for evolutionary processes that maintain the genomic heterogeneity within 1a.3.V (Figure 4—figure supplement 5). In fact, both the main ecological niches and more refined proteotypes indicate that SAAVs are not primarily structured by the global dispersal of water masses but instead tend to link distant geographic regions with similar environmental conditions (Figure 4B). This is corroborated by thousands of allele frequency trajectories correlated with in situ temperatures. Overall, these results indicate that environmentally-mediated selection is a strong determinant of SAR11 evolution and biogeography.”
2) The authors have opted against traditional population genetics methods. It is possible that the new methods are a substantial improvement, but they need to be justified. Some examples:
a) The authors justify using the "S" statistic rather than d(N)/d(S) based on there being many instances of multiple substitutions within the same codon. But this is exactly what d(N)/d(S) is meant to correct for, and S does not capture – there may be many synonymous substitutions on an evolutionary pathway, but if only one of them is non-synonymous, then S will only count this only as a non-silent change, underestimating purifying selection. What is the neutral expectation for S?
The “S” statistic (introduced as SAAVs/SNVs ratio) is not central to our study but provides an opportunity to link SNV and SAAV densities at the level of individual genes or entire metagenomes. We observed interesting trends, such as the increase of this ratio in cold waters (proteotypes A, B and C).
The accuracy of d(N)/d(S) deteriorates if a given codon contains more than a single SNV (the URL http://merenlab.org/2015/07/20/analyzing-variability/#single-codon-variants also contains some visual support for this statement with a mock example). A substantial fraction of codons analyzed in our study contained more than one SNV, a critical aspect of our study that is now better introduced in our revised manuscript. In those situations, SNVs need to be analyzed simultaneously, since the effect of one SNV (whether it is synonymous or not) will depend on the occurrence of others. As far as we know, there is no published study that effectively takes into account multiple SNVs per codon without calculating the exact codon frequencies to estimate synonymity accurately. Since they rely on actual codon frequencies and not pileups of nucleotide positions that are independent of each other, we hope the reviewer agrees that SAAVs will provide a better metric of synonymity compared to d(N)/d(S) in the case of lineages with extremely high SNV densities.
Under the neutral model, changes in the SAAVs/SNVs ratio must tend to associate with geographical adjacency as a function of the movement of water mases, rather than environmental parameters. The significant shift in SAAVs/SNVs ratio between cold currents and warm currents is of relevance to our study, especially in light of other insights (especially, the linkage between allele frequency trajectories and in situ temperature, and the biogeography of proteotypes), favors the predominant role of natural selection acting on the permissible amino acid diversification traits of this global SAR11 lineage.
Overall, we do not suggest that the SAAVs/SNVs ratio should replace d(N)/d(S). Simply, SNV density was high for 1a.3.V and many SNVs co-occurred in the same codon, rendering classical d(N)/d(S) analyses limited, which had never been challenged with extremely complex environments, if not completely irrelevant.
We appreciate reviewer’s input as it helped us better clarify the relevance of SAAVs regarding synonymy in our revision in multiple places. Specifically, the Introduction now reads:
“The substantial sequence diversity within environmental SAR11 populations not only explains the absence of SAR11 population genomes in genome-resolved metagenomics studies, but also challenges conventional approaches to the study of population genetics in microorganisms. For instance, the multiple occurrence of single-nucleotide variants in individual codon positions would render commonly used computational strategies that classify synonymous and non-synonymous variations based on independent nucleotide sites (such as in (Schloissnig et al., 2012; Bendall et al., 2016)) unfeasible.”
And the revised Results and Discussion reads:
“Percent identity distributions are useful to assess overall alignment statistics of short reads to a reference; however, they do not convey information regarding allele frequencies, their functional significance, or association with biogeography. […] Thus, quantifying frequencies of full codon sequences is a requirement to correctly assess synonymity, and it is this principle we employed in our workflow to extract SAAVs.”
And finally, regarding the specific case of the SAAVs/SNVs ratio, we added the following section:
“Interestingly, the niche defined by cold currents exhibited significantly more SAAVs (ANOVA, p-value: 1.66×〖10〗^(-12)) and a significantly higher SAAVs/SNVs ratio (ANOVA, p-value: 1.07×〖10〗^(-10)). This observation could be explained either by (1) extinction/re-emergence events that operate continually on specific codon positions (adaptive evolution), or (2) changes in abundances within a large seed bank of variants due to positive and negative selection as the lineage transits.”
We hope the reviewer agrees with our changes.
b) The BLOSUM matrices are used as "as a proxy to assess the functional difference between the pairs of amino acids", which are then compared to the substitution frequencies – but this is circular reasoning, as these matrices are calculated from substitution frequencies. The versions used in the paper are also inappropriate for this dataset, as they were calculated using more closely-related sequences than are compared in this paper.
We agree with the reviewer. We have removed BLOSUM matrices from our revision.
c) "Deep learning" is applied to cluster populations together through a method designed without genetic data in mind. This does not mean it is wrong, but as it is not an intuitive approach, it would be helpful to see validation against a more standard method, such as the fixation index, F(ST). This would help understand the distinct properties of the six subclusters – for instances, proteotype D looks to be a single successful "strain" – is this expected to be picked up as a signal by the "deep learning"?
First, we would like to address reviewer’s suggestion regarding the nature of Proteotype D. Our analysis of SAAVs using Deep Learning estimated distances between 74 metagenomes based on single amino acid variants we observed in them in the context of a single genome. This analysis linked 74 metagenomes to six proteotypes. Proteotype D emerged as a tight cluster despite its geographical spread, as the metagenomes it represents displayed similar SAAVs. If this was a single successful strain, we would have expected to find zero divergence from the environmental consensus for SAAVs. However, this was not the case: although much less when compared to other proteotypes, proteotype D did contain >5,000 SAAVs per metagenome (Figure 5, panel A). In addition, our new analysis of the percent identity of recruited reads also showed that proteotype D still maintains a large degree of variability and is far from a state that can be considered nearclonal.
Regarding the fixation index, we agree with the reviewer that this would have been a valuable addition to our work. However, we respectfully disagree with the reviewer regarding the level of intuitiveness of our approach, and we hope that our revision did a better job at introducing its relevance to study this lineage. Besides, the application of classical population genetics methods to these data are challenging due to technical reasons we outlined in our response regarding the remarkable complexity of these populations. We are hoping that our study will cultivate interest from the experts of population genetics to develop new methods that can deal with intra-population diversity of complex environmental agglomerates through ‘omics data. Our study formally introduces strategies and analytical tools for (1) the concept of SAAVs and AASTs, (2) the linkage between environmental variants and predicted protein structures, and (3) the linkage between allele frequency trajectories and environmental variables. We believe the significant amount of methodological development in this work that offers descriptions of the biogeography of the most abundant lineage in surface oceans conveys a story that will not benefit from the inclusion of additional analyses.
On the reviewer concern regarding the validation of Deep Learning: as we mentioned in our response to the Reviewer #1, we did use a more standard approach to cluster metagenomes based on the same SAAV frequency table as well. Here we would like to share parts of our previous response with the Reviewer #3 in case they do not have access to other responses: To make sure our findings with Deep Learning were not a by-product of our bioinformatics approach, we also performed an analysis of the SAAV table using Euclidian distance and Ward’s linkage. The figure in Author response images 1 and 2 shows the two main clusters, which are identical to the two main clusters Deep Learning revealed
Despite these results we elected to use the Deep Learning approach since the unbiased distance estimation afforded by this approach resulted in more highly-resolved branches in the resulting dendrogram.
3) Quality of read mapping. Many of the sequences being compared are highly divergent, but most mapping software is intended to align reads to highly similar reference sequences. While there is a promising correlation between the SNP densities identified by BWA and BOWTIE2, there is a greater than two-fold difference in the absolute densities. This suggests a very high false positive or false negative rate, depending on the method. The authors need to validate the performance of these algorithms when using highly divergent reads. Additionally, what is the justification for using the "the base-5 logarithm of the mean coverage of a gene remained within {plus minus}1 of that for the mean coverage across all genes" for assessing consistency of coverage?
We agree with the reviewer that mapping stringency is indeed critical to study population genetics, especially when one relies on reference genomes and recruitment of metagenomic reads. In most microbial lineages, the extent of diversity within a population is rather low and stringent mapping stringencies (e.g., >95% sequence identity) can be applied. This, however, is not the case for SAR11. To better clarify this point, we have significantly modified the Introduction. The relevant section now reads:
“Interestingly, the boundaries of environmental SAR11 populations appear to not comply with the 95% ANIr cutoff. For instance, Tsementzi et al., 2016, observed substantial sequence diversity within sequence-discrete SAR11 subclades in the environment, and suggested that an ANIr as low as 92% would be required to adequately define the boundaries of the SAR11 populations recovered in their study (Tsementzi et al., 2016). These findings are consistent with a comprehensive study of isolate genomes and marine metagenomes by Nayfach et al., 2016, which suggested that SAR11 is one of the most genetically heterogeneous marine microbial clades (Nayfach et al., 2016). The substantial sequence diversity within environmental SAR11 populations not only explains the absence of SAR11 population genomes in genome-resolved metagenomics studies, but also challenges conventional approaches to the study of population genetics in microorganisms.”
In line with observations from Tsementzi et al., 2016, our new analyses of short reads recruited by HIMB83 revealed extensive diversity, suggesting lower mapping stringencies would benefit deeper insights into SAR11 population genetics. During our in-house tests we found that using a stringency cut-off of 95% resulted in drastic changes in coverage values for Ia.3.V, and led decreased stability of coverage across metagenomes which resulted in much less number of core genes and metagenomes to work with. After exploring different software mapping algorithms and parameters, we concluded that the decreased SNV density with BWA was most likely due to false negatives (gene sections with high degree of SNVs were entirely overlooked). Using Bowtie2 allowed us to study the heterogeneity within this lineage at the amino acid variants level using DNA sequences that were up to 12% divergent from the reference (Figure 2—figure supplement 1 displays similar trends for all other isolate genomes).
Regarding the “base-5 logarithm”: we thank the reviewer for pointing it out. We have now better clarified our strategy:
“We then defined a subset of HIMB83 genes as the core 1a.3.V genes if they occurred in all 74 metagenomes and their mean coverage in each metagenome remained within a factor of 5 of the mean coverage of all HIMB83 genes in the same metagenome. This criterion accounted for biological characteristics influencing coverage values in metagenomic surveys of the surface ocean such as cell division rates and variations in coverage as a function of changes in GC-content throughout the genomic context. Figure 1—figure supplement 1 displays the coverage of all HIMB83 genes across all metagenomes, and Supplementary file 1J reports the coverage statistics.”
We would also like to acknowledge that given the complexity of both SAR11 lineages and metagenomics, it is indeed difficult to defend any heuristic to identify core genes. We fully appreciate this limitation, and to address further concerns we made an attempt to better explain the relevance of the 799 core 1a.3.V genes we identified:
“While the 799 genes that met these criteria systematically occurred within the niche boundaries of 1a.3.V, 40% of the remaining 671 HIMB83 genes that were filtered out were present in five or less metagenomes and coincided with hypervariable genomic loci (Figure 1—figure supplement 1). Hypervariable genome regions are common features of surface ocean microbes (Coleman et al., 2006; Zaremba-Niedzwiedzka et al., 2013; Kashtan et al., 2014; Delmont and Eren, 2018) that are not readily addressed through metagenomic read recruitment but do influence pangenomic trends. Here, less than 10% of gene clusters unique to HIMB83 were among core 1a.3.V genes (Figure 1A), indicating HIMB83’s unique genes are mostly accessory to the 1a.3.V lineage. In contrast, more than 80% of gene clusters that were core to the 21 SAR11 genomes matched to the core 1a.3.V genes. The overlap between environmental core genes of 1a.3.V revealed by the metagenomic read recruitment and the genomic core of SAR11 revealed by the pangenomic analysis of isolate genomes suggests that these genes represent a large fraction of the 1a.3.V genomic backbone (Figure 1A).”
We hope the reviewer agrees with our resolution.
[Editors' note: the author responses to the re-review follow.]
Collected reviewer comments:
The paper is vastly improved since the original version. It is now much clearer and more compelling, and the paper deals with the issue of populations better.
The paper is fascinating for showing that whatever cohesion occurs at >95% ANI in pretty much every other bacterial group does not occur here in SAR11. The paper is also really interesting for giving a robust and sensitive way to identify ecologically distinct metagenome groups from sequence data.
My concerns are mostly focused on what the authors have found out about ecological diversity within the 1a.3.V lineage.
First, I appreciate that the authors are trying to be more careful about using the concept of population, but there are still places where they are still unclear about what they mean. The authors write of "remarkable intra-population genomic diversity…." It's not clear exactly what the authors mean by "population" here, but in this context what they mean is very important. If there isn't a good definition of population being used, then how can one talk about one population's intra-population genomic diversity being higher than another? It all depends on what you mean by population, and that's not clear here.
The reviewer comment made us realize that our attempt to tip-toe around this issue by trying to avoid the use of the term ‘population’ did not improve the clarity of our study. Instead, it became more confusing due to uncommon and imprecise use of other terms (such as ‘lineage’) to describe what we are studying. We also came to the realization that most studies that use the term ‘population’ do not offer an explicit description (i.e., 10.1126/science.1248575), adding more to the confusion. We have now updated the manuscript with the following paragraph, which includes an explicit operational definition to clarify what we mean by a ‘population’ from a practical perspective with its shortcomings and cites insightful studies that offer deeper theoretical and practical discussions for this historical challenge:
“Overall, our data confirm that ANIr values of >95% used previously to delineate sequence-discrete populations does not apply to SAR11. […] However, due to the incomplete theoretical foundation and limitations associated with the use of short metagenomic reads, in discussions here we more conservatively assume that our reads originate from multiple closely related yet intertwined SAR11 populations within subclade 1a.3.V.”
We thank the reviewer for their persistence on this matter.
Figure 2 purports to show that there are not sequence-discrete populations within 1a.3.v, at least not in the sense that Kostas Konstantinidis demonstrates them in his studies, most recently in Jain et al., 2018. It is really interesting that all the SAR11 clades (with just this one clade shown in Figure 2) do not show the drop-off in recruitment of metagenome sequences below about 95% ANI. The authors argue that part of the great sequence diversity of what is recruited into this lineage (that is with much lower ANI) emerges from there being ecological diversity within the lineage (adaptations to different temperatures among different sublineages). I don't think this is a reasonable explanation, as almost every species taxon, nearly all obeying the 95% ANI drop-off rule, is also ecologically heterogeneous. I have suggested, in an interpretation of the recent Jain et al. paper, that the cohesion holding a species taxon together stems from an ability of recombination to act frequently at >95% ANI, plus the possibility of adaptive genes passing between ecologically distinct populations that are >95% ANI (Cohan, 2019) I'd argue that there is more potential for either recurrent recombination to limit divergence among ecologically distinct populations in SAR11, or greater opportunities for a single gene to be adaptive across different populations.
Very helpful insights. We believe the reviewer will find in our revision additional analyses that more clearly demonstrate that the temperature-driven changes in variable positions constitute a very small fraction of variable amino acids. We also offer a clearer discussion of populations in general and alternative interpretations of sequence heterogeneity within 1a.3.V. As a reminder, we also reworded a related paragraph to highlight possible forces driving cohesiveness within a species:
“Both high recombination rates between cells displaying low gANI values and frequent transfer of adaptive genes between ecologically distinct clades could explain the high-level of cohesion between SAR11 populations in the surface ocean (Vergin et al., 2007; Cohan, 2019). […] This analysis revealed a significant correlation between in situ temperature and distribution shape (mean p-value: 2.0×10^(-3); standard deviation p-value: 3.4×10^(-8); skewness p-value: 1.0×10^(-8)), which suggests a strong influence of temperature and its co-variables on the sequence heterogeneity within 1a.3.V (Figure 2) and is incompatible with the hypothesis of random sequence variants.”
I'd like to add that whatever cohesion occurs at >95% ANI in most species taxa does not address the ecological diversity within species taxa, or whether there are sequence-discrete populations within each taxon. In fact, most species taxa have sequence-discrete and sequence-discoverable ecologically distinct populations. (This is the point of the second half of my Current Biology dispatch I mentioned.) So, I don't think that Figure 2 makes an argument that there are not sequence-discrete, ecologically distinct populations within the focus lineage.
We agree with the reviewer. ANI >95% is nothing but an empirical observation that delineates species boundaries and does not suggest anything regarding the within-species population structures. But various values of ANI that are above 95% are often used by others as a practical expedient to define sequence-discrete populations in metagenomic read-recruitment studies (i.e., 10.1038/ismej.2015.241, 10.1371/journal.pbio.0060177, etc), and these practical cutoffs slowly become de facto expectations. The data in Figure 2 shows that if we were to follow a similar strategy for SAR11 by selecting a sequence similarity cutoff of ANI >95%, we would have been arbitrarily cutting a biologically relevant continuum. We are (and the entirety of our study is) in agreement with the reviewer on this point; the purpose of the sentence the reviewer quotes from our study is to clarify the inappropriateness to apply the commonly used cutoffs to our data.
The authors have noted that there is a small minority of genes that are invariant at the amino acid level across a given metagenome. In the earlier version, they interpreted these observations as evidence of sweeps, but here they have chosen not to address the dynamics that can be inferred by these invariant genes. I think that such single-gene sweeps are very interesting, and have been the topic of discussion of some very interesting papers, including papers by Jesse Shapiro and by Rex Malmstrom. I'd encourage the authors to bring this discussion back. I'll mention that these instances provide evidence that a generally adaptive gene has passed by recombination across all the ecologically distinct populations within a lineage, but I wouldn't expect the authors to necessarily buy in to that interpretation.
We removed the section on ‘protein sweeps’ because we realized that in the absence of timeseries data it was not straightforward to suggest invariant proteins were evidence of protein sweeps or intensive purifying selection. We changed our text to remind the reader both alternatives by adding this sentence: “We considered a protein to be ‘invariant’ (i.e., absence of variation due to intensive purifying or positive selection) in a given metagenome if it lacked SAAVs”. That said, we have further explored their dynamic across metagenomes to contribute to the exchange with the reviewer. For this, we have clustered metagenomes in a similar fashion to their clustering in the manuscript, but this time only using the differential occurrence of invariant genes at the amino acid level (see Supplementary File 2h and 2i). This analysis recapitulated Proteotype D, a set of distantly related metagenomes central to our study.
As one interpretation, it is possible that the same adaptive genes have passed by recombination across sub lineages in distant geographies (Proteotype D encompasses two sides of Panama as well as Red Sea and north of Indian Ocean). Yet, predominance of the same sub lineage in these regions due to natural selection is also a valid interpretation of the results. As mentioned above, while such analyses may seem to contribute to our study, we believe it would be misleading to suggest invariant proteins used for this figure to have resulted from events of protein sweeps. We hope the reviewer agrees with our resolution.
I found the authors' partitioning of metagenomes into ecologically distinct groups fascinating. And it's particularly interesting that these groups could not be revealed (at least entirely) by analyzing single reads (as shown in Figure 4—figure supplement 3). I'll encourage the authors to venture from their interpretation regarding whole metagenomes to inferring that there appear to be multiple ecologically distinct populations that cause these metagenomes to hold different niches. What might be the ecological distinctions of the populations? Clearly they are different for their temperature adaptation, as revealed by Figure 4 (mislabeled Figure 5). Perhaps the authors could glean something about what is different about the constituent populations from their Figure 4—figure supplement 3, that is by comparing the clusters that they do find with known environmental parameters.
We thank the reviewer very much for their suggestion (and catching the mislabeled Figure 5, which is now fixed). Taking their advice, we have now created a new table (Supplementary File 4d) to investigate and make available to others the ANOVA statistic for each known environmental parameter released by the Tara Oceans Project along with data our study revealed.
As shown in Figure 4, the strong association with latitude can easily be explained by the geographic partitioning of oceanic currents. Besides longitude, temperature best explains clusters of proteotypes as a function of SAAVs. We have updated the results in our revision to cite this table.
Re the concluding statement about "everything is everywhere," I'd temper that by saying 'at least for marine bacteria'. This is because the statement does talk about "everything," so I think there needs to be some limitation to the conclusion.
Fair. The sentence now reads:
“Overall, these findings suggest that environmentally-mediated selection plays a critical role in the journey of cosmopolitan microbial populations in the surface ocean, lending credence to the idea for marine systems that “everything is everywhere but the environment selects” (Baas-Becking, 1934).”
Concerns about evolutionary analyses:
In response to point (1), the authors state:
"Simply, we observed that certain amino acids (mostly hydrophilic), and most notably, few AASTs (e.g., alanine/isoleucine) predominated in our SAAV table."
It has been well-established for decades that hydrophobic amino acids in solvent-inaccessible positions are more conserved (e.g. see reviews cited in the Introduction to Ramsey et al. 2011 Genetics 188(2):478-88). Reproducing this observation in the SAR11 dataset simply confirms that purifying selection can be observed over long timescales. It does not help identify what the changes are that enable ecological adaptation.
Indeed, this section does not contribute to our investigation of ecological adaptation within the SAR11 lineage. It sheds light into purifying selection.
Delmont et al: "These points are interesting, but they do not favor natural selection over neutral evolution. Instead they provide information regarding permissible versus non-permissible diversification"
I assume "permissible" refers to purifying selection? Which is an example of natural selection – rather than random, neutral diversification. It is distinct from the adaptive/Darwinian evolution that allows the ecological differentiation of the bacteria. Not separating neutrality, purifying selection and adaptive evolution plagues the whole manuscript. The authors have a strain distribution that appears to reflect ecological differentiation, rather than neutral diffusion. They have a mutation distribution that mainly seems to reflect purifying selection, rather than neutral evolution. Some analyses (e.g. below) suggest adaptive evolution, which is potentially very interesting, but the overall trend is consistent with purifying selection. The authors need to clearly distinguish their different conclusions.
We are extremely thankful for the reviewer for bringing up this point, which made us revise our manuscript carefully to elucidate which aspects of our data are consistent with neutral evolution, purifying selection, and adaptive evolution. We hope that our revisions summarized below will satisfy the reviewer.
First, to convey that a skewed AAST frequency distribution is incompatible with a purely neutral process, but is indicative of purifying selection that is consistent with modern neutral theories of molecular evolution, we have added the following text at the introduction of the AAST frequency distribution:
"While such a skewed AAST frequency distribution cannot be explained by strictly random mutational process (Figure 3B light-gray shaded area), it is compatible with standard theories of neutral or nearly-neutral evolution, since such theories consider the role of purifying selection (Ohta and Gillespie, 1996)."
To support this point and to illustrate how neutral events alone are unable to recapitulate the observed AAST distribution, we have calculated the expected AAST frequency distribution under strictly neutral mutational events by considering the codon frequencies observed in the HIMB83 reference sequence, the edit distance between codons, and the transition/transversion ratio in a probabilistic model (we added a new Materials and methods section "Estimating a neutral AAST frequency distribution" to detail our approach, and updated our reproducible workflow with the details of the procedure). Briefly, we used extremes for the transition probabilities between codons to calculate upper and lower bounds for a strictly neutral AAST frequency distribution, and updated panel B in our Figure 3 to illustrate the expected AAST frequency distribution in the absence of both purifying and adaptive selection (light gray shaded area).
Next, we revised the text to explicitly state and offer interpretation to the apparent role that purifying selection has in maintaining the AAST frequency distribution across distant geographies. We replaced the following text in our previous submission with the following one:
"The overall consistency of AAST frequency distributions across geographies supports the hypothesis that purifying selection maintains amino acid exchangeability within 1a.3.V and enables an interpretation of these data through a neutral model: SAAVs composing the AAST frequency distribution represent primarily neutral mutations that have drifted to measurable levels, and the lack of SAAVs in uncommon AASTs arises due to a strong purifying selection acting against deleterious mutations that occur frequently between dissimilar amino acids."
Moreover, in our previous manuscript we had stated: “Yet, close inspection of AAST frequencies revealed that amino acid exchange abilities subtly diverge in a pattern that correlates with water temperature and/or its co-variables (Figure 3B insets, Figure 3—figure supplement 2).”
But after careful consideration of the reviewer’s point, we found that this statement does not do justice in describing the subtlety of this signal in comparison to the predominant signal of purifying selection. To address this, we have amended the text so that it now reads:
"However, a closer inspection reveals a subtle divergence of amino acid exchangeabilities that correlates with water temperature and/or its co-variables (Figure 3B insets, Figure 3—figure supplement 2). Note that this divergence is AAST specific; for example, positions with mixed proportions of glutamic and aspartic acid are less commonly found in warm waters (linear regression, uncorrected p-value: 2.7×10^(-6)), yet for isoleucine and valine such a correlation is nonexistent (linear regression, uncorrected p-value: 0.418). These findings suggest that amidst a signal that is predominantly indicative of purifying selection, there appears to be a fingerprint of adaptive/divergent processes caused by temperature and/or its co-variables that subtly shift the mutational profile within 1a.3.V."
In addition, in the section entitled “Temperature correlates with amino acid allele frequency trajectories”, we have made more substantial changes that are detailed in our response to the next reviewer concern. Finally, we added a final paragraph that summarizes the ratio between these processes as suggested by the data, which is also described later in this document.
Delmont et al: "we determined that thousands of 1a.3.V allele frequency trajectories correlated with in situ temperature, in line with the biogeography of proteotypes"
It is not surprising that many of the variable sites correlate with temperature, because the proteotypes are defined using the variable sites, and the proteotypes correlate with temperature. The authors should seek to synthesize these data – do the variable sites have properties that suggest selection between niches? Do they concentrate in particular genes that might drive adaptation to different niches? Or do they simply correlate with the population structure?
We are again very thankful for this question, which helped us to make a remarkable observation that not only supports our findings but also will be helpful for those who study SAR11 biology. Unfortunately, as of today, scrutinizing all 4,592 positions where amino acid frequencies correlated with temperature with their structural context in protein structures is a computationally intractable problem. However, to tackle this important point we surveyed all genes to quantify the ratio of temperature-correlated and temperature-uncorrelated SAAV positions in each, and then focused on a single gene, which encoded glycine betaine ATP-binding cassette transporter, for an in-depth analysis using its predicted structure. The reason we chose this gene is two-fold. First, betaine transporters of SAR11 are highly translated proteins in the environment that transport osmolyte compounds into cells for energy production, thus playing an essential role in SAR11 biology. Second, this gene exhibited a high ratio of temperature-correlated and temperature-uncorrelated SAAV positions, enabling us to tease apart the distribution of these SAAVs in the structure of the gene while being able to rely on the large number of positions to test whether our observations are statistically meaningful. We laid out our findings in a new paragraph, with this critical section:
“(…) To investigate the positioning of amino acids in the tertiary structure of the permease structure relative to the cellular membrane, we first categorized the location of each residue as transmembrane, cytosolic (inside the inner membrane), or periplasmic (outside the inner membrane) (Figure 3—figure supplement 4). Positions that were not correlated with temperature were commonly transmembrane, and infrequently periplasmic. In contrast, most positions that correlated with temperature were periplasmic (Figure 3—figure supplement 4). The probability of observing a similar distribution between temperature-correlated and temperature-uncorrelated positions across transmembrane, periplasmic, and cytosolic regions was only 0.034 (analytic trinomial test, temperature-uncorrelated distribution as prior), which indicates temperaturecorrelated positions are subjected to unique evolutionary forces. A previous study suggested that periplasmic residues of transmembrane proteins undergo higher rates of adaptive evolution due to their increased exposure to changing environmental conditions (Sojo et al., 2016). This observation lends additional support to the hypothesis that periplasmic SAAV positions within this gene that correlate with temperature are more likely shaped by adaptive processes.”
We also added a new supplementary figure (Figure 3—figure supplement 4) to put this observation into a visual context.
We hope the reviewer appreciates our effort to show that correlation with temperature is not evenly or randomly distributed in the context of this gene. In contrast, there is a significant coupling between the kind of variation and the structural context. We are striving to improve upon current computational limitations to ensure that in the future investigations of such phenomena will be able to explore a wider range of structural diversity.
Overall, I return to my original point from the first round of reviews: is the diversification mainly neutral; dominated by purifying selection; or dominated by adaptive evolution? The most interesting signals may not be from the dominant evolutionary process, of course.
We are certain that the reviewer agrees that quantifying these differential forces accurately is a challenging task. However, we also acknowledge that the reviewer is raising a valid question which will be asked by many readers, and a gross summary is necessary. We addressed this need by including the following paragraph in our revised manuscript to solidify our consideration of the primary evolutionary forces, and to offer a broad summary of our data that shows their ratios. We also used this opportunity to include a limitations statement to warn careful readers with the hope that they will take these suggestions with a grain of salt:
“One question remains: what is the proportion of distinct evolutionary processes acting upon closely related SAR11 populations within 1a.3.V? Offering a precise answer to this critical question is compounded by multiple theoretical and technical factors. […] In summary, this gross summary of the data suggests that among the remarkable amount of variation within some of the most abundant and prevalent microbial populations in the ocean, adaptive evolutionary processes operate on core genes are responsible for variation in about 2% of all codon positions.”
We thank the reviewer for pushing us to better communicate our findings.
In response to point 2, the authors state:
Delmont et al: "As far as we know, there is no published study that effectively takes into account multiple SNVs per codon without calculating the exact codon frequencies to estimate synonymity accurately…SNV density was high for 1a.3.V and many SNVs co-occurred in the same codon, rendering classical d(N)/d(S) analyses limited, which had never been challenged with extremely complex environments, if not completely irrelevant."
Yang (2007) MBE 24(8):1586-91 – with over 6,000 citations – describes how baseml can be used to calculate d(N)/d(S) using base substitution, rather than codon substitution, models. This accounts for multiple substitutions per codon, and provides a statistical test for evidence of selection versus neutrality, unlike the methods presented in the current version. It does not matter how complex the environments are – indeed, d(N)/d(S) has been applied to the question of SAR11 adaptation to different ecologies by Brown et al., 2012 and Luo and Hughes, 2012, Mol. Syst. Biol. 8:625. The authors should at least apply standard methods to the assembled genomes, based on the ecological separation they have determined. Methods also exist for calculation of this statistic from metagenomic data, and might be more informative.
We apologize for the vague statement. Indeed, when performing comparative genomics, it is possible to calculate d(N)/d(S) even in the case of multiple substitutions per codon. The critical point here is that both Yang as well as Brown et al. relied on comparative genomics to study genes under positive selection, i.e., they did have genomes to compare with each other (for instance, Brown et al. wrote in their manuscript: “Signatures of positive selection were detected in the two polar genomes compared with their most closely related tropical counterparts”). Our study differs from these studies and other previous attempts as it deals with novel challenges that arise when working with metagenomes. To address these additional challenges that none of the previous studies addressed, we developed a new workflow to determine SAAVs regardless of the number of SNVs per codon in a given metagenome without relying on comparative genomics. We regret that our previous responses may have compounded this misunderstanding; however, the manuscript clearly states that our contribution is from the perspective of metagenomics rather than comparative genomics:
“SAAVs: Accurate characterization of non-synonymous variation
Percent identity distributions are useful to assess overall alignment statistics of short reads to a reference; however, they do not convey information regarding allele frequencies, their functional significance, or association with biogeography. […] Thus, quantifying frequencies of full codon sequences is a requirement to correctly assess synonymity, and it is this principle we employed in our workflow to extract SAAVs.”
We hope this clarification will satisfy the reviewer.
Delmont et al: "The significant shift in SAAVs/SNVs ratio between cold currents and warm currents is of relevance to our study, especially in light of other insights (especially, the linkage between allele frequency trajectories and in situ temperature, and the biogeography of proteotypes), favors the predominant role of natural selection acting on the permissible amino acid diversification traits of this global SAR11 lineage."
The correlation between allele frequencies and temperature, and proteotype and temperature, is confounded by the correlation between proteotypes and allele frequencies. This should be explained (if this is wrong, please also make that clear in the text).
We agree with the reviewer that allele frequency trajectories confound proteotype classification, and so we have removed the sentence, “This is corroborated by thousands of allele frequency trajectories correlated with in situ temperatures” from the text.
The authors need to make clear whether the natural selection they are referring to is stronger/weaker purifying selection changing the SAAV/SNV ratio; or positive selection driving adaptive diversification increasing the SAAV/SNV in some populations.
We agree that the manuscript needed to clearly distinguish between varying levels of purifying selection versus adaptive evolution, and we hope the reviewer is satisfied with our consistency in delineating the evolutionary forces that can be gleaned through our data in the latest version of our manuscript. In the specific case of the SAAV/SNV ratio, we have opted to remove our SAAV/SNV ratio analysis from our revision altogether. Our rationale stems from planned future efforts to create a more robust and meaningful metric, in contrast to the current one that has weaknesses as the reviewer pointed. Nevertheless SAAV/SNV ratio was only ever briefly mentioned in our study, and the exclusion of it does not influence our findings. We thank the reviewer for pointing this.
Delmont et al: "Regarding the fixation index, we agree with the reviewer that this would have been a valuable addition to our work…We believe the significant amount of methodological development in this work that offers descriptions of the biogeography of the most abundant lineage in surface oceans conveys a story that will not benefit from the inclusion of additional analyses. "
I don't understand how this statistic can be "a valuable addition", yet the story "will not benefit from the inclusion of additional analyses.". The deep learning analysis is still not intuitively explained in the new version (not the authors' fault, as these methods are designed to be complex). It should be complemented by fixation index calculations, which are simple, and would allow the readers a much greater insight into the basis of the results.
We thank the reviewer for their persistence in benchmarking the Deep Learning method against classically used and well-understood methods. We have now calculated fixation indices for the SAAV data, and created a new computational tool to make sure this approach is accessible to other researchers in a more straightforward manner, as well. The results recapitulate our primary observations of distant geographies being linked, and we have added a new supplementary figure (Figure 4—figure supplement 4) comparing the dendrograms and placement of the clusters onto the world map.
Articles from eLife are provided here courtesy of eLife Sciences Publications, Ltd
Citations & impact
Impact metrics
Article citations
Modern microbiology: Embracing complexity through integration across scales.
Cell, 187(19):5151-5170, 01 Sep 2024
Cited by: 0 articles | PMID: 39303684
Review
Genomic representativeness and chimerism in large collections of SAGs and MAGs of marine prokaryoplankton.
Microbiome, 12(1):126, 15 Jul 2024
Cited by: 0 articles | PMID: 39010229 | PMCID: PMC11247762
Decoding populations in the ocean microbiome.
Microbiome, 12(1):67, 01 Apr 2024
Cited by: 1 article | PMID: 38561814 | PMCID: PMC10983722
Gradients of bacteria in the oceanic water column reveal finely-resolved vertical distributions.
PLoS One, 19(4):e0298139, 02 Apr 2024
Cited by: 0 articles | PMID: 38564528 | PMCID: PMC10986988
A cryptic plasmid is among the most numerous genetic elements in the human gut.
Cell, 187(5):1206-1222.e16, 01 Feb 2024
Cited by: 8 articles | PMID: 38428395
Go to all (57) article citations
Data
Data behind the article
This data has been text mined from the article, or deposited into data resources.
BioStudies: supplemental material and supporting data
Data Citations (Showing 6 of 6)
- (1 citation) DOI - 10.6084/m9.figshare.5248432
- (1 citation) DOI - 10.6084/m9.figshare.5248453
- (1 citation) DOI - 10.6084/m9.figshare.5248945
- (1 citation) DOI - 10.5281/zenodo.835218
- (1 citation) DOI - 10.6084/m9.figshare.5248459
- (1 citation) DOI - 10.6084/m9.figshare.5248447
Show less
Similar Articles
To arrive at the top five similar articles we use a word-weighted algorithm to compare words from the Title and Abstract of each citation.
Red Sea SAR11 and Prochlorococcus Single-Cell Genomes Reflect Globally Distributed Pangenomes.
Appl Environ Microbiol, 85(13):e00369-19, 17 Jun 2019
Cited by: 5 articles | PMID: 31028022 | PMCID: PMC6581160
Natural variation in SAR11 marine bacterioplankton genomes inferred from metagenomic data.
Biol Direct, 2:27, 07 Nov 2007
Cited by: 67 articles | PMID: 17988398 | PMCID: PMC2217521
Are ocean currents too slow to counteract SAR11 evolution? A next-generation sequencing, phylogeographic analysis.
Mol Phylogenet Evol, 107:324-337, 25 Nov 2016
Cited by: 3 articles | PMID: 27894996
The unique metabolism of SAR11 aquatic bacteria.
J Microbiol, 51(2):147-153, 27 Apr 2013
Cited by: 23 articles | PMID: 23625213
Review
Funding
Funders who supported this work.
Marine Biological Laboratory
NIBIB NIH HHS (1)
Grant ID: T32 EB009412