Abstract
Motivation
Data-independent acquisition mass spectrometry allows for comprehensive peptide detection and relative quantification than standard data-dependent approaches. While less prone to missing values, these still exist. Current approaches for handling the so-called missingness have challenges. We hypothesized that non-random missingness is a useful biological measure and demonstrate the importance of analysing missingness for proteomic discovery within a longitudinal study of disease activity.Results
The magnitude of missingness did not correlate with mean peptide concentration. The magnitude of missingness for each protein strongly correlated between collection time points (baseline, 3 months, 6 months; R = 0.95-0.97, confidence interval = 0.94-0.97) indicating little time-dependent effect. This allowed for the identification of proteins with outlier levels of missingness that differentiate between the patient groups characterized by different patterns of disease activity. The association of these proteins with disease activity was confirmed by machine learning techniques. Our novel approach complements analyses on complete observations and other missing value strategies in biomarker prediction of disease activity.Supplementary information
Supplementary data are available at Bioinformatics online.Free full text

The use of missing values in proteomic data-independent acquisition mass spectrometry to enable disease activity discrimination
Abstract
Motivation
Data-independent acquisition mass spectrometry allows for comprehensive peptide detection and relative quantification than standard data-dependent approaches. While less prone to missing values, these still exist. Current approaches for handling the so-called missingness have challenges. We hypothesized that non-random missingness is a useful biological measure and demonstrate the importance of analysing missingness for proteomic discovery within a longitudinal study of disease activity.
Results
The magnitude of missingness did not correlate with mean peptide concentration. The magnitude of missingness for each protein strongly correlated between collection time points (baseline, 3months, 6
months; R
=
0.95–0.97, confidence interval = 0.94–0.97) indicating little time-dependent effect. This allowed for the identification of proteins with outlier levels of missingness that differentiate between the patient groups characterized by different patterns of disease activity. The association of these proteins with disease activity was confirmed by machine learning techniques. Our novel approach complements analyses on complete observations and other missing value strategies in biomarker prediction of disease activity.
Supplementary information
Supplementary data are available at Bioinformatics online.
1 Introduction
Proteomic biomarkers have a range of uses in respect to prediction of disease risk, response to therapy, prognosis and diagnosis. The discovery of proteomic biomarkers for these purposes can enable better patient stratification and disease management. Large biobank studies have been created, including UK Biobank (Sudlow et al., 2015) and 100 000 Genomes Project (Caulfield et al., 2017), which emphasize the interrogation of large-scale cohorts to uncover the cause of disease progression. Such biobanks create a fertile ground for discovery of new biomarkers using the many samples collected. In clinical research, over 200 specific proteins are measured to guide clinical decisions, and new interdisciplinary omics analyses will enable development of novel approaches to patient stratification and health care. As an example, in the study of cancers, measurement of circulating tumour DNA and proteins has enabled risk detection algorithms to be developed (Cohen et al., 2018). Thus, to achieve this high demand, there is a necessity for high sample throughput, specificity, quantitative reproducibility and deep coverage proteomics techniques to profile molecular signatures that report on the state of the patient at a given time and relate this to a genomic propensity to a condition; risk of ill health or potential (adverse) response to treatment.
Sequential window acquisition of all theoretical mass spectra (SWATH) is a data-independent acquisition mass spectrometry technique created to be a reproducible, label-free method of proteomic analyses, with the sensitivity of targeted methods but with increased proteome depth (Gillet et al., 2012). The use of cyclical acquisition covering the entire m/z range allows for retrospective interrogation of all peptides in a sample with the use of peptide reference libraries. SWATH is more reproducible in peptide identification and identifies more peptides than data-dependent methods (Krasny et al., 2018), therefore, the resulting data has fewer missing values although it offers informatic challenges.
The handling of data containing missing values in biomarker discovery and proteomics remains a challenge and is not yet standardized (Lazar et al., 2016; Webb-Robertson et al., 2015; Wei et al., 2018). Missing values in a mass spectrometry setting can be due to variation at a number of sources in the analysis pipeline from sample storage, extraction, losses in protein digestion and separation, measurement failure during mass spectrometry acquisition, relative quantification and depends on the quality control thresholds used and the signal-to-noise ratio. Missingness has been generalized into three types (Rubin, 1976), missing completely at random, missing at random and missing not at random, which are usually handled by imputation approaches specific to the type of missingness (Lazar et al., 2016). For the handling of values missing not at random, the limit of detection (LOD) of a mass spectrometer is usually the focus of missingness approaches. Data are produced with missing values of this sort are ‘left-censored’ data where the distribution of the proteins’ abundance is truncated on the left side, due to the lower abundances not detected by the mass spectrometer at the LOD (Lazar et al., 2016).
Missing values cannot be analysed by standard statistical tests, which has led to three principle methods of approaching missing data:
1. Devoting laboratory time and money to reprocessing and rerunning samples.
2. Removing the participants, samples or analytes with most missing values from statistical analyses, and therefore, diminishing the scope of the study due to the loss of data and study power.
3. Imputation via the replacement of missing values found in the raw data with one number, zero, a small value, the mean (Gromski et al., 2014) or variations of calculations around the value of the LOD (Hornung and Reed, 1990); any of these approaches may introduce selection biases in the distributions of the proteins. Alternatively, imputation techniques to create a varying value for each missing value present through correlations or other statistical estimations may be used (Oba et al., 2003).
There are many imputation approaches and software tools now available for mass spectrometry (Choi et al., 2019; Karpievitch et al., 2012; Wei et al., 2018), however, there are many cases where imputation is not accurate enough in metrics of variance and sample classification (Webb-Robertson et al., 2015); for example, if there is a high level of missing data, if the sample size is small, if a large subset of analytes have at least one missing value at random and the training set, therefore, contains a small number of complete observations, or when the ratio between the number of values used for the training set is small compared to the number requiring imputation from that training set. Therefore, there is a need to analyse missing values as they are found in the raw data, to complement the current analyses of observed data in biomarker discovery.
In metabolomics and subsequent biomarker studies, the handling of missing data is often by the ‘80% rule’ (Yang et al., 2015), which is a threshold for complete observations, keeping only those metabolites identified in at least 80% of samples. This threshold is arbitrary and may not be appropriate for studies including longitudinal data such as drug treatment response studies, where such a threshold likely leads to the loss of potentially interesting data. There is no agreed set threshold specifically for proteomics studies.
The coverage of the proteome achieved by undertaking SWATH allows for the analysis of a novel subgroup of missing not at random values: missing with interest or biologically missing. As SWATH technology allows for more consistency and reproducibility in the analyses of the proteome, we present a novel approach of analysing missing values in a longitudinal study for the discovery of biomarkers associated with disease activity; an example of a study setting where missing values can be informative and such analyses complement current measurement protocols. We highlight the value of missing values in deep proteomic biomarker studies.
2 Materials and methods
2.1 Participants and samples
Rheumatoid arthritis patients were recruited at baseline and were assessed at follow-up at 3 and 6months. About 64 participants were selected for SWATH proteomic analyses according to their disease activity and used for the informatics study paradigm described below. Serum samples were collected from the 64 participants over the three time points; 58 participant samples were measured at baseline, 47 at 3 months and 44 at 6
months. Disease activity at 6 months was scored using an algorithm derived to combine data on standard markers of the disease, and this outcome was categorized into low- and high disease activity using established criteria (Prevoo et al., 1995), which was used as the outcome for all analyses (Supplementary Methods). From the 64 participants selected for proteomic analyses, 32 participants had low disease activity, 12 had high disease activity and 20 had low disease activity at 3
months but progressive worsening by 6
months (secondary high disease activity group). The study complies with the declaration of Helsinki. Participants provided written informed consent, and the study is ethically approved (COREC 04/Q1403/37). The proteomics methodology can be found in the Supplementary Methods.
2.2 Post-acquisition bioinformatics and relative quantification
The SWATH map from the mass spectrometer was converted from proprietary SCIEX format (.wiff and accompanying scan files) to .mzML open-format for the OpenSWATH engine using the SCIEX MS Data Converter (Beta 1.3) under profile mode. The resulting .mzML files were time-normalized against an iRT library and interrogated with a serum-focused spectral library (Röst et al., 2014) using the OpenSWATH single executable (OpenSWATHWorkflow version 2.0.1). Parameters were set as previously described (Navarro et al., 2016). The OpenSWATH output files were subjected to pyProphet algorithm (Teleman et al., 2015) for false discovery assessment of the inferred transition groups. The outputs were aligned using the TRIC algorithm (Röst et al., 2016), to retain only consistent inferred transition groups. Proteotypic peptides were quantotypic (criterion of one peptide per protein) with a 15% consensus filter, or appear in both duplicate injections. Quality control filtering included removal of sample data if the coefficient of variation across duplicates was above a median of 20%. MSstats from Bioconductor in R (Choi et al., 2014) was used for normalization and protein quantification. The equalize medians and Top 3 features per peptide options parameters were used.
2.3 Statistical analyses
2.3.1 Missing value threshold
Proteins that were not detected in >10% of total samples were removed from the analyses (required > 15 observations of the total 149). This threshold was used after assessment of the distribution of total missingness, which showed an inflated distribution of proteins with <15 observations (see Supplementary Figs S1 and S2). The removal of the proteins with <10% total observations ensures that proteomic outliers in specific discrete samples were excluded from analyses, which may arise from individual heterogeneity in this small cohort or underlying mass spectrometry noise, which is not biologically informative. Therefore, acceptance of proteins that are present in at least 10% of measurements confirms the mass spectrometry reproducibility in multiple disease activity groups at different time points. Thus, the 565 proteins that remained from the initially identified 742 that were used for further analyses are unlikely to be outliers and their presence has been reproducibly confirmed by the mass spectrometer and further quality control assessments. R software (version 3.5.2) was used for all analyses and graphic plotting.
2.3.2 Assessment of proteomic outliers via magnitude of missingness
We hypothesized that the most informative proteins would be reproducibly missing in a similar pattern for all patients with the same disease activity, regardless of the time point of collection (of which there are three); such proteins would be consistently missing/present over the three time points. To assess if each protein’s presence or missingness was similar over time, regardless of disease status, the strength of relationship between time points was calculated via Pearson’s correlation coefficient by counting the missing values for each protein, at each of the three time points separately, and adjusting by the number of samples collected at that time point (Equation 1). To identify proteins whose presence or absence altered with disease activity, the strength of relationship was assessed by counting missing values for each protein, in each of the three disease activity statuses, regardless of time point, and adjusting for the number of samples collected for certain disease activity (Equation 1). The relationship of a protein's missingness was plotted and outliers were visually identified for further assessment. The magnitude of missing values (
2.3.3 Machine learning protocol
Feature selection and machine learning were undertaken using the framework proposed by Perez-Riverol et al. (2017), to identify proteins at baseline and at 3 months that predict the 6-month disease activity outcome. Feature selection removed proteins by univariate correlation of R<
0.3 with disease activity, and by multivariate correlation of R
>
0.75 with each other. Recursive feature elimination and importance evaluation identified the best protein predictors of the disease outcome via Random Forest. The missing values for the comparative machine learning analyses were set to zero, as the exploited approach does not handle missing values.
2.3.4 Assessment of batch effects
Assessment of batch effects on the magnitude of missing values was completed in four ways:
1.
2.
3.
4.
3. Results
3.1 Participant characteristics
Serum samples of 64 participants from three time points were analysed. Table 1 highlights the description of the participants included. There was little correlation between age [R = −0.18, confidence interval (CI) = −0.41 to 0.07] or gender (R = −0.08, CI = −0.32 to 0.17) and disease activity group (distributions depicted in Supplementary Fig. S3).
Table 1.
Summary statistics of the study participants
Trait | Mean (range) | ||
---|---|---|---|
DA | High | 2° High | Low |
N | 12 | 20 | 32 |
Age (years) | 63 (21–82) | 66 (37–81) | 59 (28–81) |
Gender | 8% male | 20% male | 19% male |
BMIa | 32.29 (22.27–50.32) | 26.61 (17.04–35.80) | 29.43 (19.71–43.70) |
Note: The mean and range for each trait that describes the 64 participants included in the proteomic analyses are shown.
BMI, body mass index; DA, disease activity; n, sample size.
3.2 SWATH missingness is not due to LOD
A total of 565 proteins were included in the analysis; 99 proteins had complete observations in all serum samples, the remainder of proteins contained at least one missing value (Supplementary Table S1). We addressed the hypothesis that missing values found in SWATH analyses are increased in low abundance proteins where concentrations are near or below the LOD of the mass spectrometer. We found a weak relationship between the mean log abundance of the proteins and the count of missing values in the 149 samples (R = −0.37, CI = −0.44 to −0.30, Supplementary Fig. S1), as well as when the samples are separated by disease activity group and collection time point (R = −0.33 (CI = −0.40 to −0.25) to R = −0.09 (CI = −0.17 to −0.01), Supplementary Fig. S2). We concluded that when using the SWATH mass spectrometry methodology, including the standard bioinformatic approaches, missingness is not strictly left-censored and this suggests that missingness might be reproducible and informative at the biological level. Such missing values could, therefore, be treated as measures in themselves, rather than due to methodological issues in measuring them (e.g. mass spectrometry matrix effects).
3.3 Proteomic missingness is similar over time
Since it is possible that some protein levels alter markedly over the time course of the study, we examined how levels of missingness change over time. There was higher magnitude of missingness (see Equation 1) in proteins measured at the baseline collection of samples (58 samples, 39% missing protein values) than the samples collected at 3months (47 samples, 31% protein missing values) or 6
months (44 samples, 30% protein missing values). We assessed the strength of the relationship of magnitudes of missingness for each protein between the time points (Fig. 1). This relationship was strong (R
=
0.95–0.97, CI = 0.94–0.97), showing that the magnitude of missingness for each protein was similar regardless of time point of collection. This similarity in missingness is likely due to the reproducibility of the technique; identifying proteins in the same way in each sample, or further, from the fidelity of the bioinformatics workflow; assessing the certainty of the presence of the peptide with appropriate quality control filters. This reproducibility is a firm foundation for the analyses of missing data, as any deviations in missingness are more likely due to a biological change.
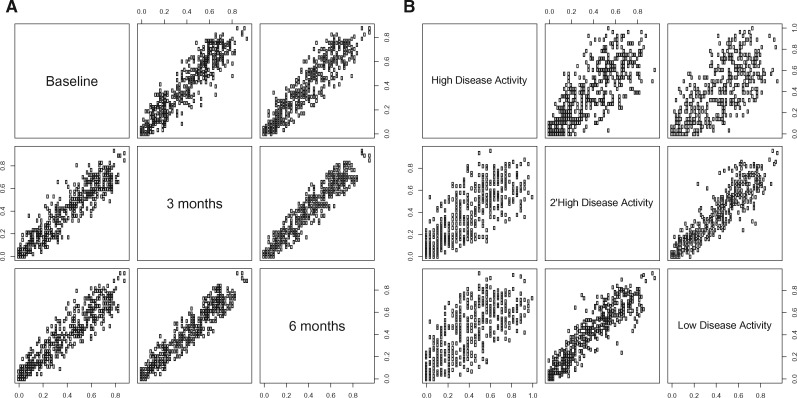
The assessment of missingness for each protein over collection time points and by disease activity status. (A) The correlation between protein missingness over three time points showed a strong relationship; the magnitude of missingness for each protein measured at baseline correlated with those measured at 3 months and with those at 6 months to R=
0.95 (CI = 0.94–0.96). The protein missingness measured at 3 months correlated with those measured at 6 months to R
=
0.97 (CI = 0.96–0.97). (B) The correlation between protein missingness separated by response status to treatment. The magnitude of missingness for each protein identified in the high disease activity group correlated with those measured in secondary high disease activity group to R
=
0.84 (CI = 0.82–0.86), and to those measured in low disease activity group to R
=
0.82 (CI = 0.79–0.84). The protein missingness measured in secondary high disease activity group correlated with that of low disease activity group to R
=
0.94 (CI = 0.93–0.95)
3.4 Proteomic missingness differs by disease activity
The relationship strength and similarity found previously in the analysis of the magnitude of missingness between time points, decreased when comparing between disease activity groups. Figure 1 also depicts the correlation between the disease activity groups for the magnitude of missingness of each protein. Missingness in the high disease activity group correlated with secondary high disease activity to R=
0.84 (CI = 0.82–0.86), and with low disease activity to R
=
0.82 (CI = 0.79–0.84). Such strength of relationship was stronger between the missingness of peptides between secondary high disease activity and low disease activity, at R
=
0.94 (CI = 0.93–0.95), which is likely due to the secondary high disease activity participants having a favourable disease activity until the 3-month time point. As the strength of relationship between missingness at the different time points was R
>
0.95, and the strength of relationship when analysing the magnitude of protein missingness by the disease activity of the participant was lower (R
<
0.94), outliers of missingness can be assessed from correlation with disease activity status, and such outliers may be informative of a biological change due to the response to treatment.
In this analysis outliers of missingness were identified; one of these is highlighted in Figure 2. An outlier when comparing the magnitude of missingness between low disease activity and both types of high disease groups was found, demonstrating a higher than expected degree of missingness in the low disease activity group. This suggests that the protein may predict the underlying biology when comparing low disease activity with any high disease activity category and offers encouragement that this approach can identify biomarkers to be validated further. The protein did not demonstrate outlier behaviour in the correlation of magnitude of missingness between high disease activity and secondary high disease activity, and therefore, does not differentiate between these two groups. Further, it was not found to be an outlier of missingness when comparing the different time points (Supplementary Fig. S4). This example demonstrates how correlation analysis assessing the relationship of total missingness in all measurements, identified a protein that can potentially discriminate in respect of disease activity status.
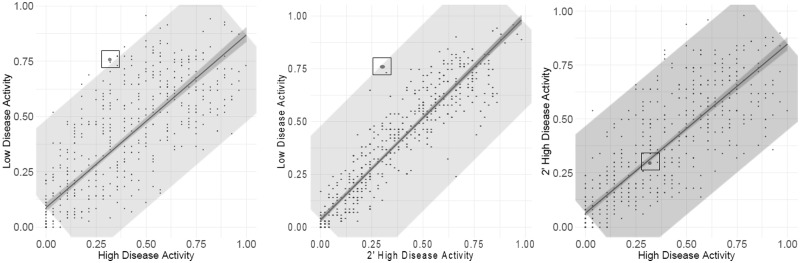
Identification of an example outlier protein (in the square) as a predictor of disease activity from the assessment of missing values. The outlier protein is identified as an outlier due to increased missingness count in low disease activity participants when compared to both types of high disease activity groups. The protein’s missingness does not separate those at particularly high disease activity participants from secondary high disease activity participants. The shaded area is a line parallel to the linear regression line, expanded in size
3.5 Missingness outliers may associate with disease activity
Further descriptions of the protein identified as an outlier of missingness between response groups are shown in Table 2. The table shows the raised level of missing values identified for the measurement of this protein in low disease activity participants at each time point. The protein is a candidate biomarker of high disease activity due to its raised number of observations. To assess how abundant this protein is usually in serum, we used the Protein Abundance Database, PaxDb (Wang et al., 2015), a comprehensive absolute protein abundance database. In humans, the protein is very abundant in multiple cells and tissues around the body, while there is no data on serum expression; it is found at lowest identifiable abundance in plasma (0.04–0.11ppm range). This may suggests that an abnormal increased concentration can be indicative of disease pathology. This, and other identified outlier proteins were not found to be enriched with any specific protein domains or functional categories, as assessed by enrichment analysis (Supplementary Methods).
Table 2.
Missingness summary statistics of an outlier protein
Time | N | HD | 2°HD | LD | n (miss) | % (miss) in LD |
---|---|---|---|---|---|---|
Baseline | 58 | 5 (42%) | 9 (47%) | 21 (77%) | 35 | 60% |
3 months | 47 | 0 (0%) | 4 (27%) | 18 (78%) | 22 | 82% |
6 months | 44 | 4 (57%) | 2 (13%) | 15 (71%) | 21 | 71% |
Total | 149 | 9 (32%) | 15 (30%) | 54 (76%) | 78 | 69% |
Note: High disease participants (HD), secondary high disease participants (2°HD), and low disease participants (LD) are described. HD and 2°HD had low levels of missingness at each time point of collection, while LD showed >2-fold levels of missingness at all time points. n = count of participants at each time point; % indicates missing values of the total participants with that outcome at that time point; n(miss) = the count of total missing values at each time point; and %(miss) in LD is the % of total missingness for the outlier protein found in LD at each collection time point. The total contains a sum of the counts and the mean % in brackets.
3.6 Confirmation of a missingness outlier protein as a predictor of disease activity by machine learning techniques
Clearly, our data also contain relative quantitation measurements of the outlier protein in the samples where it was detected. Machine learning was used as previously published (Perez-Riverol et al., 2017) to identify proteomic biomarkers that predict the disease activity at 6months based on the measured levels within the same analysis.
Using the observed values for the proteomic biomarkers measured at baseline (as opposed to magnitude of missingness), Random Forest identified the exemplar outlier protein alongside 21 other proteins in the prediction of disease activity (Supplementary Fig. S5). Using the proteomic biomarkers measured at the 3-month time point, the outlier protein was identified with only three other proteins as predictors of disease activity (Supplementary Fig. S6), supporting our identification of this protein from our analysis of missingness.
3.7 Batch does not affect the missingness results
The samples were run together in the proteomic analyses, i.e. each participant’s samples (baseline, 3months, 6
months) were run sequentially. We, therefore, assessed the effect of batch on the missingness outcomes of disease activity status. There were 12 batches used to run the samples, Supplementary Figure S7 depicts the distributions of the samples at each time point and disease activity status over the batches. Samples of all time points were distributed over all 12 batches. High disease activity samples were distributed over only two batches, secondary high disease activity samples over eight batches and low disease activity samples over 10 batches. All four adjustments to assess batch effects (see Section 2.3.4 ) did not affect the results, including the relationships of missingness or on the outlier protein (Supplementary Fig. S8).
3.8 Reproducible missingness is created by standard SWATH bioinformatic approaches
The bioinformatics tools align the samples to retain only consistently inferred transition groups. At this point, the presence of any peptide for the exemplar protein was investigated in the samples to explore where the missing values were created along the bioinformatic pipeline (Supplementary Fig. S9). As expected, there was an increase in the magnitude of missing values for the protein after bioinformatic quality control thresholds were applied (post pyProphet, TRIC and thresholds applied). However, the trend of missing values between collection time points was comparable before and after bioinformatic quality control. The missing values were assessed similarly against disease activity, which showed that the low disease activity participants had an increased magnitude of missing values both before and after bioinformatic quality control when compared with high disease activity participants. This highlights the biological importance of this protein, which was more likely missing in low disease activity participants and more identifiable in high disease activity participants from the mass spectrometry data. The missingness is increased by the bioinformatic quality control in the identification of high-fidelity assignment of protein identity using standard proteomic approaches.
3.9 Further analyses of current missingness thresholds
The effect of specific thresholds in our biomarker discovery process was assessed; three based on the percentage of missing values (<90%, <50% and <20%), and one using a discrete value based on the distribution of missingness, which showed a dip in the frequency of proteins with <50 missing values (see Supplementary Fig. S10). The same machine learning technique was used to identify proteomic biomarkers that predict disease activity using each of the different missingness thresholds. The best performance was achieved for proteins at baseline and 3 months when using a threshold of >10% observations (<90% missing values) (Supplementary Fig. S11). This accuracy is less due to the differing number of proteins included at each threshold, as the correlation between the number of proteins and the accuracy of the machine learning was R=
0.48 (CI = −0.21 to 0.85; n
=
10), but more likely the removal of noise and the underlying assumption by the machine learning software that the proteins with the most missing values are lowest due to the zero imputation. Further correlation plots and Principal component analyses using the different missing values thresholds are shown in Supplementary Figure S12, where the strength of relationship between the missingness and time point or disease activity status decreases with the missing values lost at each observation threshold.
4 Discussion and conclusions
We present an alternative approach for the analysis of missing data in a SWATH mass spectrometry setting for an exemplar set of 149 samples. This complements the analyses of complete observations, imputation, and other missing value replacement statistical techniques. We show that such analyses allow for the identification of proteins that differ in missingness by disease activity. We confirmed the predictive importance of an exemplar protein by machine learning techniques. Similarly, we show that this protein was observed more in high disease activity participants, with more missingness found in patients with low disease activity.
The 90% missingness threshold (10% observations), which includes any biomarker found with at most 90% missing values, allowed for better accuracy in the prediction of proteomic biomarkers of disease activity and therefore showed that more stringent thresholds decrease prediction accuracy and remove potentially important discoveries. As there was a weak relationship between the number of proteins included in the analysis and the accuracy of the analysis, this high accuracy found when using the 90% missing threshold is likely due to the importance of missing values on prediction of disease activity. Had a missing value threshold of 80% been used (maximum 20% missing values), as is usual of mass spectrometry studies, the outlier protein we identified as a marker of low activity would have been removed from analyses and left undiscovered.
Missingness as an informative tool is not a novel concept; missingness is informative when a value is missing due to a test not being carried out because of a reason that is not missing at random. For example, this can be due to the test being left unanswered; where personal information is involved, where it was answered as ‘Prefer not to answer’, where it is thought that scoring highly is unlikely, or where a test is only provided to subgroups of participants (e.g. medical screening specific for certain genders, ages, risk populations, or disease activity). For example, a portion of the UK female population do not have cervical smear test results, as many do not attend their free testing appointment (77% attendance rate). Some of the reasons provided for non-attendance include embarrassment, negative perceptions of health professionals, worry and trust in results, concerns about the procedure, idiosyncratic beliefs, extreme negative experiences and not receiving prompts to be screened (Marlow et al., 2019). In some cases, the absence of data can be informative by its own accord, as in electronic health records (EHRs). Missing values in this setting may be due to biased screening, tests only conducted if clinically relevant to specific ailments, or information being fragmented across multiple healthcare systems (Beaulieu-Jones et al., 2018). Thus, in some scenarios, no test result can potentially be informative. While it may be possible that missingness is not informative in such a way in a mass spectrometry setting, informative missingness can be exampled by blood antibody rheumatoid factor, where the concentration correlates with severity of rheumatoid arthritis. This test is typically only performed for patients with some indication of rheumatoid arthritis. Thus, patients with high rheumatoid factor levels are more likely to have rheumatoid factor measures, while the missing values could not be imputed (Mason et al., 2010).
The need for novel biomarkers of disease has led to an increase in the collection of large-scale cohorts and consortiums, in the aim of identifying biological predictors that stratify patients with polymorphic phenotypes, differing response to treatment, or those at risk of the occurrence of a disease event. Many cohorts have limited samples and resources; it is of great importance that the full benefit of the effort dedicated to the cohort collection and funding, is achieved in the resulting discoveries. SWATH-MS is a particularly useful tool for large-scale proteomics due to its reproducibility, analysis depth and the ability to recursive data mine for proteomic biomarkers of interest via differential peptide/protein reference libraries. The proteomic coverage obtained from SWATH results in the production of fewer missing values and we show here that they are not fully due to the LOD. The proteins that are of an average log intensity but identified in few samples are likely due to the quality control thresholds of the retrospective data interrogation used for SWATH proteomics (Fu et al., 2018). As the assumption of missing-at-random (MAR) cannot be verified from observed data, sensitivity analyses have been used to assess the impact on results of departures from the assumption of MAR (Leacy et al., 2017). Here, we provide an additional method of such assessment for SWATH-MS data.
The generalisability of this approach to other datasets, which contain their own missing data patterns and processes, is currently unclear. However, the strength of the dataset used here is the availability of protein measurements at multiple time points, allowing for confirmation of reproducible missingness. Similarly, other application would also require similar data that allow the consistency of missingness to be tested. While not tested here, it is likely that this method can be applied to other analysis platforms, sample types and diseases, as it is the dataset of multiple time points and disease statuses that is of importance to the methodology. This is demonstrated by a similar study assessing mortality outcomes and ICD-9 criteria (Che et al., 2018). As far as we are aware, there is no reason wider application could not be pursued. A limitation of this study is the relatively small sample size, and large cohort studies will aid this effort. In order to take forward any protein as a biomarker, the results will need to be validated in an independent cohort. Nevertheless, here, we demonstrate the proposed technique has potential and can contribute to presently employed informatics techniques. In conclusion, we have shown that the peculiar features of SWATH-MS allow for the inclusion of samples with missing values in statistical analysis for biomarker discovery. Furthermore, we have shown the usefulness of analysing missingness as it is found in raw data, complementing and expanding on the findings achievable with current missing value strategies.
Funding
This work was supported by the Medical Research Council, UK through an MRC Doctoral Award and an MRC Flexible Training Supplement [MR/K501311/1 to K.M.]; and a University of Manchester President’s Doctoral Scholarship [to K.M.]. This work was further supported by the Medical Research Council and the Engineering and Physical Sciences Research Council [MR/N00583X/1 ‘Manchester Molecular Pathology Innovation Centre (MMPathIC): bridging the gap between biomarker discovery and health and wealth’ to A.D.W, N.G. and A.B.]; National Institute for Health Research Manchester Biomedical Research Centre [to D.P., A.B. and A.D.W.], Versus Arthritis [21754] and Bloodwise [13005]. Equipment at the Stoller Biomarker Discovery Centre is funded by the Medical Research Council [MR/M008959/1]. This work was also supported by the Cancer Research UK Manchester Centre award [C5759/A25254 to D.C. and A.D.W.]. B.D.K. is supported by a British Heart Foundation Personal Chair.
Conflict of Interest: none declared.
References
- Beaulieu-Jones B.K. et al. (2018) Characterizing and managing missing structured data in electronic health records: data analysis. JMIR Med. Inform., 6, e11. [Europe PMC free article] [Abstract] [Google Scholar]
- Caulfield M. et al. (2017) The 100,000 genomes project protocol. Genomics Engl.P, 3, 1–112. [Google Scholar]
- Che Z. et al. (2018) Recurrent neural networks for multivariate time series with missing values. Sci. Rep., 8, 6085. [Europe PMC free article] [Abstract] [Google Scholar]
- Choi J. et al. (2019) A comparison of different methods to handle missing data in the context of propensity score analysis. Eur. J. Epidemiol., 34, 23–36. [Europe PMC free article] [Abstract] [Google Scholar]
- Choi M. et al. (2014) MSstats: an R package for statistical analysis of quantitative mass spectrometry-based proteomic experiments. Bioinformatics, 30, 2524–2526. [Abstract] [Google Scholar]
- Cohen J.D. et al. (2018) Detection and localization of surgically resectable cancers with a multi-analyte blood test. Science, 359, 926–930. [Europe PMC free article] [Abstract] [Google Scholar]
- Fu J. et al. (2018) Discovery of the consistently well-performed analysis chain for SWATH-MS based pharmacoproteomic quantification. Front. Pharmacol., 9, 1–11. [Europe PMC free article] [Abstract] [Google Scholar]
- Gillet L. et al. (2012) Targeted data extraction of the MS/MS spectra generated by data independent acquisition: a new concept for consistent and accurate proteome analysis. Mol. Cell. Proteomics, 11, O111.016717. [Europe PMC free article] [Abstract] [Google Scholar]
- Gromski P. et al. (2014) Influence of missing values substitutes on multivariate analysis of metabolomics data. Metabolites, 4, 433–452. [Europe PMC free article] [Abstract] [Google Scholar]
- Hornung R., Reed L. (1990) Estimation of average concentration in the presence of nondetectable values. Appl. Occup. Environ. Hyg., 5, 46–51. [Google Scholar]
- Karpievitch Y.V. et al. (2012) Normalization and missing value imputation for label-free LC-MS analysis. BMC Bioinformatics, 13 (Suppl. 1), S5. [Europe PMC free article] [Abstract] [Google Scholar]
- Krasny L. et al. (2018) SWATH mass spectrometry as a tool for quantitative profiling of the matrisome. J. Proteomics, 189, 11–22. [Europe PMC free article] [Abstract] [Google Scholar]
- Lazar C. et al. (2016) Accounting for the multiple natures of missing values in label-free quantitative proteomics data sets to compare imputation strategies. J. Proteome Res., 15, 1116–1125. [Abstract] [Google Scholar]
- Leacy F.P. et al. (2017) Analyses of sensitivity to the missing-at-random assumption using multiple imputation with delta adjustment: application to a tuberculosis/HIV prevalence survey with incomplete HIV-status data. Am. J. Epidemiol., 185, 304–315. [Europe PMC free article] [Abstract] [Google Scholar]
- Marlow L. et al. (2019) Barriers to cervical screening among older women from hard-to-reach groups: a qualitative study in England. BMC Womens Health, 19, 1–10. [Europe PMC free article] [Abstract] [Google Scholar]
- Mason A. et al. (2010) Insights into the use of Bayesian models for informative missing data.Technical Report. Imperial College London. 1–38. [Google Scholar]
- Navarro P. et al. (2016) A multicenter study benchmarks software tools for label-free proteome quantification. Nat. Biotechnol., 34, 1130–1136. [Europe PMC free article] [Abstract] [Google Scholar]
- Oba S. et al. (2003) A Bayesian missing value estimation method for gene expression profile data. Bioinformatics, 19, 2088–2096. [Abstract] [Google Scholar]
- Perez-Riverol Y. et al. (2017) Accurate and fast feature selection workflow for high-dimensional omics data. PLoS One, 12, e0189875–14. [Europe PMC free article] [Abstract] [Google Scholar]
- Prevoo M.L.L. et al. (1995) Modified disease activity scores that include twenty‐eight‐joint counts development and validation in a prospective longitudinal study of patients with rheumatoid arthritis. Arthritis Rheum., 38, 44–48. [Abstract] [Google Scholar]
- Röst H.L. et al. (2014) OpenSWATH enables automated, targeted analysis of data-independent acquisition MS data. Nat. Biotechnol., 32, 219–223. [Abstract] [Google Scholar]
- Röst H.L. et al. (2016) TRIC: an automated alignment strategy for reproducible protein quantification in targeted proteomics. Nat. Methods, 13, 777–783. [Europe PMC free article] [Abstract] [Google Scholar]
- Rubin D.B. (1976) Inference and missing data. Biometrika, 63, 581–592. [Google Scholar]
- Sudlow C. et al. (2015) UK Biobank: an open access resource for identifying the causes of a wide range of complex diseases of middle and old age. PLoS Med., 12, e1001779. [Europe PMC free article] [Abstract] [Google Scholar]
- Teleman J. et al. (2015) DIANA-algorithmic improvements for analysis of data-independent acquisition MS data. Bioinformatics, 31, 555–562. [Abstract] [Google Scholar]
- Wang M. et al. (2015) Version 4.0 of PaxDb: protein abundance data, integrated across model organisms, tissues, and cell-lines. Proteomics, 15, 3163–3168. [Europe PMC free article] [Abstract] [Google Scholar]
- Webb-Robertson B.-J.M. et al. (2015) Review, evaluation, and discussion of the challenges of missing value imputation for mass spectrometry-based label-free global proteomics. J. Proteome Res., 14, 1993–2001. [Europe PMC free article] [Abstract] [Google Scholar]
- Wei R. et al. (2018) Missing value imputation approach for mass spectrometry-based metabolomics data. Sci. Rep., 8, 1–10. [Europe PMC free article] [Abstract] [Google Scholar]
- Yang J. et al. (2015) A data preprocessing strategy for metabolomics to reduce the mask effect in data analysis. Front. Mol. Biosci., 2, 4. [Europe PMC free article] [Abstract] [Google Scholar]
Articles from Bioinformatics are provided here courtesy of Oxford University Press
Full text links
Read article at publisher's site: https://doi.org/10.1093/bioinformatics/btz898
Read article for free, from open access legal sources, via Unpaywall:
https://academic.oup.com/bioinformatics/article-pdf/36/7/2217/33027393/btz898.pdf
Citations & impact
Impact metrics
Citations of article over time
Alternative metrics
Smart citations by scite.ai
Explore citation contexts and check if this article has been
supported or disputed.
https://scite.ai/reports/10.1093/bioinformatics/btz898
Article citations
Identification of a Proteomic Signature for Predicting Immunotherapy Response in Patients With Metastatic Non-Small Cell Lung Cancer.
Mol Cell Proteomics, 23(10):100834, 29 Aug 2024
Cited by: 0 articles | PMID: 39216661 | PMCID: PMC11474190
Proteomic Characterization of Plasma in Lemierre's Syndrome.
Thromb Haemost, 124(5):432-440, 19 Oct 2023
Cited by: 0 articles | PMID: 37857346 | PMCID: PMC11038868
Pan-Cancer Proteomics Analysis to Identify Tumor-Enriched and Highly Expressed Cell Surface Antigens as Potential Targets for Cancer Therapeutics.
Mol Cell Proteomics, 22(9):100626, 28 Jul 2023
Cited by: 3 articles | PMID: 37517589 | PMCID: PMC10494184
Proteomic changes induced by longevity-promoting interventions in mice.
Geroscience, 46(2):1543-1560, 31 Aug 2023
Cited by: 1 article | PMID: 37653270 | PMCID: PMC10828338
Proteomic signature associated with chronic kidney disease (CKD) progression identified by data-independent acquisition mass spectrometry.
Clin Proteomics, 20(1):19, 20 Apr 2023
Cited by: 4 articles | PMID: 37076799 | PMCID: PMC10116780
Go to all (14) article citations
Data
Data behind the article
This data has been text mined from the article, or deposited into data resources.
BioStudies: supplemental material and supporting data
Similar Articles
To arrive at the top five similar articles we use a word-weighted algorithm to compare words from the Title and Abstract of each citation.
OptiMissP: A dashboard to assess missingness in proteomic data-independent acquisition mass spectrometry.
PLoS One, 16(4):e0249771, 15 Apr 2021
Cited by: 2 articles | PMID: 33857200 | PMCID: PMC8049317
GMSimpute: a generalized two-step Lasso approach to impute missing values in label-free mass spectrum analysis.
Bioinformatics, 36(1):257-263, 01 Jan 2020
Cited by: 11 articles | PMID: 31199438 | PMCID: PMC6956786
Folic acid supplementation and malaria susceptibility and severity among people taking antifolate antimalarial drugs in endemic areas.
Cochrane Database Syst Rev, 2(2022), 01 Feb 2022
Cited by: 12 articles | PMID: 36321557 | PMCID: PMC8805585
Review Free full text in Europe PMC
Missing data is poorly handled and reported in prediction model studies using machine learning: a literature review.
J Clin Epidemiol, 142:218-229, 16 Nov 2021
Cited by: 43 articles | PMID: 34798287
Review
Funding
Funders who supported this work.
Blood Cancer UK (1)
Application of advanced protein technologies to identify common treatment targets for myeloid leukaemias
Professor Anthony Whetton, University of Manchester
Grant ID: 13005
British Heart Foundation (2)
Investigation of genetic mechanisms underlying susceptibility to complex congenital and arrhythmic cardiovascular disease (renewal)
Professor Bernard Keavney, University of Manchester
Grant ID: RG/15/12/31616
The BHF Chair of Cardiovascular Medicine
Professor Bernard Keavney, Manchester, University of
Grant ID: CH/13/2/30154
British Heart Foundation Personal Chair
Cancer Research UK (1)
Grant ID: C5759/A25254
Cancer Research UK Manchester Centre (1)
Grant ID: C5759/A25254
Engineering and Physical Sciences Research Council (1)
Grant ID: MR/N00583X/1
MRC Flexible Training Supplement (1)
Grant ID: MR/K501311/1
Manchester Molecular Pathology Innovation Centre
Medical Research Council (4)
Grant ID: 1637982
Manchester Molecular Pathology Innovation Centre (MMPathIC): bridging the gap between biomarker discovery and health and wealth
Professor Anthony Freemont, The University of Manchester
Grant ID: MR/N00583X/1
Manchester Academic Health Science Centre Technology Hub: Clinical Proteomics Centre for Stratified Medicine
Professor Anthony Whetton, The University of Manchester
Grant ID: MR/M008959/1
Grant ID: MR/K501311/1
National Institute for Health Research Manchester Biomedical Research Centre
University of Manchester President’s Doctoral Scholarship
Versus Arthritis (1)
Centre for Genetics & Genomics Versus Arthritis
Prof Jane Worthington, University of Manchester
Grant ID: 21754