Abstract
Free full text

The DisGeNET knowledge platform for disease genomics: 2019 update
Associated Data
Abstract
One of the most pressing challenges in genomic medicine is to understand the role played by genetic variation in health and disease. Thanks to the exploration of genomic variants at large scale, hundreds of thousands of disease-associated loci have been uncovered. However, the identification of variants of clinical relevance is a significant challenge that requires comprehensive interrogation of previous knowledge and linkage to new experimental results. To assist in this complex task, we created DisGeNET (http://www.disgenet.org/), a knowledge management platform integrating and standardizing data about disease associated genes and variants from multiple sources, including the scientific literature. DisGeNET covers the full spectrum of human diseases as well as normal and abnormal traits. The current release covers more than 24 000 diseases and traits, 17 000 genes and 117 000 genomic variants. The latest developments of DisGeNET include new sources of data, novel data attributes and prioritization metrics, a redesigned web interface and recently launched APIs. Thanks to the data standardization, the combination of expert curated information with data automatically mined from the scientific literature, and a suite of tools for accessing its publicly available data, DisGeNET is an interoperable resource supporting a variety of applications in genomic medicine and drug R&D.
INTRODUCTION
Modern genome sequencing technologies are fostering the integration of genomics into clinical practice. The exploration of human variation at large scale by genome sequencing or SNP array genotyping are enabling the identification of disease-associated variants for a wide range of diseases and conditions. Nevertheless, the interpretation of the results of genomic analysis and the identification of variant of clinical relevance remain a significant challenge (1). Variant assessment still involves manual exploration of multiple sources of data, which requires a significant amount of time and experts in the domain. In this context, new bioinformatic tools and resources that enable the automation of every possible step in this process are crucial. In this regard, resources such as ClinVar (2), ClinGen (3), the Genomics England PanelApp (https://panelapp.genomicsengland.co.uk/), Orphanet (4) and OMIM (5), among others, have demonstrated their utility to support variant interpretation. Here, we present a new release of DisGeNET, a knowledge management platform that houses one of the most exhaustive and publicly available catalogues of genes and genomic variants associated with human diseases. Originally implemented in 2010 as a Cytoscape plugin (6), during the last years DisGeNET has evolved into different formats and tools (7–10), and it now undergoes its sixth release as a knowledge management platform aimed at supporting different application scenarios and users.
The DisGeNET database contents
The core concepts in the DisGeNET database structure (Figure (Figure1A)1A) are the Gene–Disease Association (GDA) and the Variant–Disease Association (VDA), that are collated from different data sources (Figure (Figure2).2). The integration of these diverse sources of data is enabled by proper standardization of genes, variants, diseases (diseases, symptoms and traits) and associations using community-driven ontologies and controlled vocabularies, as well as ontologies developed ad hoc (e.g. the DisGeNET association type ontology). Of note, the provenance of the information is provided in several ways: (a) as the field ‘original database’ that indicates where the data was taken from (e.g. ClinVar or UniProt (11)), (b) with the number of articles that support the association and the NCBI PMIDs of these publications and (c) with a text excerpt from the article that expresses the evidence for the association. GDAs and VDAs are further annotated with in-house and external attributes easing data analysis, exploration and prioritization. For the attributes incorporated from external resources the provenance is also provided.
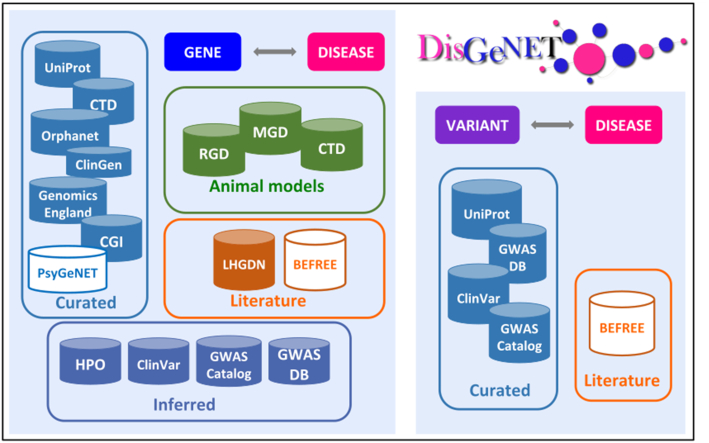
Data sources and data types in DisGeNET. For Gene–Disease Associations (GDAs) the data sources are classified as Curated, Animal models, Inferred and Literature. For Variant-Disease Associations (VDAs) the data sources are classified as Curated and Literature. The data sources in white are developed in-house, while the others are third-party resources.
New data sources and data types
The current release (v6.0) of the database contains 628 685 gene-disease associations (GDAs), involving 17 549 genes and 24 166 diseases, and 210 498 variant-disease associations (VDAs), including 117 337 variants and 10 358 diseases (see details in Table Table1).1). Note that the term ‘disease’ refers to a wide range of phenotypes relevant in human genomics: actual diseases, disease symptoms and abnormal phenotypes that are observed as disease manifestations, as well as normal traits and phenotypes that are currently explored in large scale Genome Wide Association studies (GWAs) (see section New data attributes and prioritization metrics for more details on disease standardization and annotation). The GDAs and VDAs integrated in the DisGeNET database originate from over a dozen repositories, each one with a different focus, for example, databases that annotate clinically relevant variants (ClinVar) or genes (ClinGen, Genomics England PanelApp, among others), or specialized in certain disease classes (e.g. Orphanet for rare diseases) or compiling information from animal models of disease (e.g. MGD (12) and RGD (13)) (Figure (Figure2).2). In addition to the original source of information for the VDAs and GDAs, DisGeNET provides a classification for the database sources: for the gene-disease associations (GDAs), the information is classified as Curated, Animal Models, Literature and a new category, Inferred (Figure (Figure22 and Table Table3).3). In the case of variant-disease associations (VDAs), the data is classified into Curated and Literature. For more details about the data content in DisGeNET 6.0, see Tables Tables11 and 2.
Table 1.
Distribution of genes, diseases and GDAs by source
Source | Genes | Diseases | Assocs |
---|---|---|---|
CGI | 341 | 200 | 1650 |
CLINGEN | 274 | 205 | 518 |
GEN. ENGLAND | 3326 | 114 | 7897 |
CTD_human | 7919 | 8251 | 62 794 |
ORPHANET | 3496 | 3520 | 6850 |
PSYGENET | 1530 | 109 | 3656 |
UNIPROT | 3730 | 4542 | 6798 |
CURATED | 9413 | 10 370 | 81 746 |
HPO | 3688 | 7502 | 134 890 |
CLINVAR | 3848 | 6307 | 10 695 |
GWASDB | 3948 | 321 | 8253 |
GWASCAT | 4767 | 653 | 14 182 |
INFERRED | 8700 | 13 176 | 163 626 |
CTD_mouse | 71 | 298 | 474 |
CTD_rat | 22 | 26 | 46 |
MGD | 1637 | 2111 | 4711 |
RGD | 1585 | 681 | 6364 |
ANIMAL MODELS | 2795 | 2789 | 11 517 |
LHGDN | 5938 | 1800 | 31431 |
BEFREE | 15 147 | 12 219 | 401 440 |
LITERATURE | 15 283 | 12 418 | 415 583 |
ALL | 17 549 | 24 166 | 628 685 |
Table 2.
Distribution of variants, diseases and VDAs by source
Source | Variants | Diseases | Assocs |
---|---|---|---|
CLINVAR | 50 141 | 6443 | 67 978 |
GWASDB | 32 162 | 386 | 46 468 |
GWASCAT | 20 486 | 725 | 32 950 |
UNIPROT | 20 148 | 4246 | 35 217 |
CURATED | 104 653 | 7954 | 165 354 |
BEFREE | 19 407 | 4228 | 48 998 |
LITERATURE | 19 407 | 4228 | 48 998 |
ALL | 117 337 | 10 358 | 210 498 |
Table 3.
Classification of the data sources in DisGeNET
Source type | GDAs | VDAs |
---|---|---|
Curated | UniProt | UniProt |
CTD | ClinVar | |
Orphanet | GWAS Catalog | |
ClinGen* | GWAS DB* | |
Genomics England* | ||
CGI* | ||
PsyGeNET | ||
Animal models | RGD | NA |
MGD | ||
CTD | ||
Inferred | HPO | NA |
ClinVar | ||
GWAS Catalog | ||
GWAS DB* | ||
Literature | BeFree | BeFree |
LHGDN |
*New source with respect to the previous release 5.0. NA: not available.
Mining disease-associated genes and variants from the literature
A distinctive feature of DisGeNET is its unique collection of GDAs and VDAs extracted by text mining the scientific literature (14,15). DisGeNET contains a corpus of 400K publications with information about GDAs and VDAs. Sixty percent of the GDAs included in DisGeNET have been extracted from the scientific literature by text mining and are not reported in any of the curated resources integrated in DisGeNET. Due to the current challenge to manually identify, curate and properly store phenotype–genotype information as structured data, it is important to have means to extract this information from the literature in an automatic and exhaustive manner to keep the pace of the most recent scientific findings. The importance of collecting this information is particularly evident in the clinical genomics area, where there is a pressing need to identify all the knowledge, including the most recent one, on disease association for sequence variants identified in the genome of patients. An example of the insights that this expanded information can potentially provide over current authoritative resources and gene panels databases is illustrated in section ‘Expanding information for rare diseases’. Our text mining tools leverage on controlled vocabularies and ontologies to properly identify and standardize the entities and relationships found in the literature, and they exploit linguistic and semantic textual features to identify genotype-phenotype relationships. On the other hand, it is noteworthy that 78% of the GDAs and 91% of the VDAs reported in DisGeNET are supported by at least one bibliographic reference. In addition, to help the user in navigating literature-derived data, for each publication we provide an exemplary sentence or text excerpt that expresses the association under study (see Figure Figure7C7C for an example).

Analysis of GWAs results with DisGeNET. (A) 61 out of 143 variants identified by a recent GWAs of Type 2 Diabetes Mellitus (T2D) (29) are reported in DisGeNET as associated to cardiometabolic diseases and traits, and 47 variants are annotated to T2D. (B) Top-scoring variants in DisGeNET from those found in the study (29). DisGeNET provides additional information such as the consequence type of the variant according to VEP, allele frequencies from the gnomAD database, DisGeNET score, number of supporting publications with linkouts to MEDLINE, to name a few attributes. (C) Network of diseases and phenotypes associated with variant rs7903146 annotated by curated databases, created with the DisGeNET Cytoscape App. Examples of text excerpts extracted by text mining from publications supporting the association are shown.
Disease–disease associations
In this DisGeNET release we present a new dataset, the DisGeNET disease-disease associations (DDAs), which can be used to explore similarities between pairs of diseases or between diseases and traits based on shared genes and variants. The analysis of DDAs can support a variety of applications, such as the study of disease comorbidities as well as finding genomic similarities among different disease diagnosis. The DDAs are obtained by connecting two diseases if they share at least one gene or one variant in a particular source database (Figure (Figure3A).3A). The fraction of shared genes (or variants) between two diseases is assessed by the Jaccard Index (JI), and a P-value obtained by permutation testing (for more details see http://www.disgenet.org/dbinfo). The DDAs dataset contains more than 11 × 106 pairs of diseases sharing at least one gene (P-value < 0.05) and over 200 000 pairs of diseases sharing at least one variant (P-value < 0.05). The DDAs dataset can be explored via the web interface, where the user can search by disease or database source and apply different filters such as JI value, minimum number of shared genes (or variants), P-value threshold, disease class, among others. This new dataset is also available via the DisGENET REST-API, the disgenet2r package and the Cytoscape App (Figure (Figure3B3B).
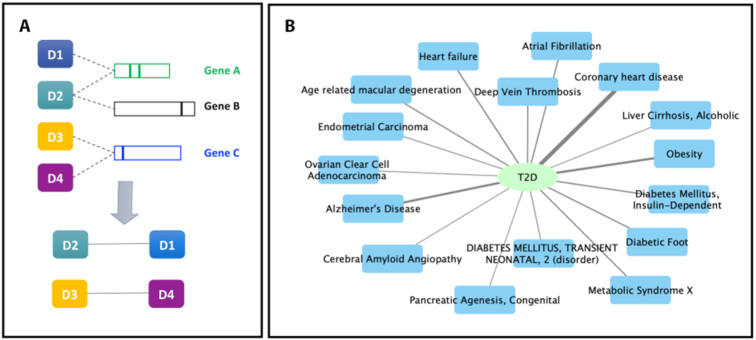
Disease-Disease associations in DisGeNET. (A) Two diseases are connected if they share at least one gene or one variant in the GDA or the VDA dataset, respectively. A Jaccard Index with its associated P-value are provided for each association to rank and filter the Disease-Disease association results. For more details see http://www.disgenet.org/dbinfo. (B) The Disease-Disease association network of Type 2 Diabetes Mellitus (T2D, CUI: C0011860). The network shows the diseases associated to T2D through common variants from DisGeNET curated databases with a P-value ≤ 0.05.
New data attributes and prioritization metrics
Diseases, genes and variants in DisGeNET are annotated with attributes that cover a wide variety of biomedical resources: diseases are coded using UMLS® (16) concept unique identifiers (CUIs), and annotated with the UMLS® semantic type, the MeSH class, and the top level concepts from the Human Disease Ontology (17) and the Human Phenotype Ontology (18). Genes are referred by their NCBI identifiers, and are annotated with the official gene symbol, the UniProt accession, the protein class, and with their value of pLI (probability of being loss-of-function intolerant), a gene constraint metric from the gnomAD consortium (19). The genomic variants are identified using the dbSNP identifier and annotated with their reference and alternative alleles, and their genomic coordinates (corresponding to NCBI dbSNP Human Build 151, and Assembly GRCh38). Additionally, the variants are classified according to their most severe consequence type assigned by the Variant Effect Predictor (20) based on canonical gene transcripts. Most DisGeNET variants are missense (28%), followed by intronic (26%), frameshift and intergenic (both 11%) (Figure (Figure4).4). In this new release of DisGeNET, variants are also annotated with the allelic frequency in genomes and exomes according to data from the gnomAD consortium.
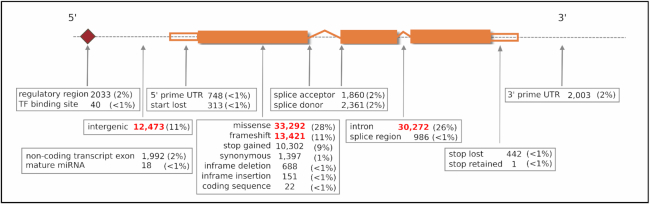
Distribution of most severe consequence types in DisGeNET variants. Consequence types are obtained from the Variant Effect Predictor (ENSEMBL).
Additionally, the platform includes a series of in-house developed metrics and attributes to facilitate ranking and filtering the information. Each phenotypic concept is classified according to a DisGeNET type as disease (such as Crohn disease, schizophrenia, Alzheimer's disease, etc.), phenotype (such as depressive symptoms, blood pressure, body mass index, neutrophil count, etc.) or group (such as cardiovascular diseases, neoplasms, etc.). This classification is based on the UMLS semantic types and expert curation. Sixty-six percent of DisGeNET CUIs are classified as diseases, 4% are classified as groups, and 30% as phenotypes. This release of DisGeNET includes a larger number of phenotypes because in this class are included traits, measurements and laboratory test results that are collected mainly by the GWAS catalog (21) and GWASdb (22).
While 20% of the genes in the Curated DisGeNET subset (12% in the whole DisGeNET database) are annotated to a single disease or phenotype concept, the remaining genes are annotated to more than one disease or phenotype, with exceptional cases of clinically relevant genes annotated to over hundreds or thousands of concepts, such as TP53, TNF, PTEN and MTHFR (Figure (Figure5A).5A). A similar behavior is observed for the variants, although the fraction of variants annotated to a single concept is higher than for genes (over 60%, Figure Figure5B).5B). In this regard, we define the Disease Specificity Index (DSI) and the Disease Pleiotropy Index (DPI) to reflect the different behaviour of genes and variants with respect to the number of associated diseases (see http://www.disgenet.org/dbinfo#DPI and http://www.disgenet.org/dbinfo#DSI for more details). Both metrics are aimed at indicating how specific is a gene or variant with respect to the associated diseases. A value of the DSI close to one means that the gene or variant is disease-specific, while a value close to zero indicates that the gene or variant is disease-promiscuous. The DPI considers if the diseases associated with the gene (or variant) are similar among them and belong to the same disease class (e.g. Cardiovascular Diseases) or belong to different disease classes. In this case, disease–promiscuous genes or variants generate values of DPI close to one.

Distribution of number of associated diseases per gene (panel A) and variant (panel B) in the DisGeNET Curated subset and in the whole database (ALL). Note that genes or variants associated to a single UMLS concept have a DSI equal to one, and a DPI close to zero, while genes or variants associated to several UMLS concepts have higher DPI, and lower DSI.
DisGeNET provides several attributes and metrics that allow the user to evaluate the relevance of the gene and variant associations, which is especially helpful in the case of diseases with a large number of associated genes or variants (for instance Schizophrenia has >1000 genes and variants in Curated sources, and over 1700 in the whole database). The DisGeNET association type provides a semantic classification of the biology of the association. The Evidence Level, only available for GDAs coming from ClinGen and Genomics England PanelApp, classifies the association according to the available evidence based on expert assessment in these databases (23). The number of supporting publications indicates how well studied is the association, along with its temporal span (year of first and last publication recorded in DisGeNET, see Figure Figure6A6A for an example). This last feature can also be used to distinguish novel associations from those well described associations having a large number of publications and to identify new trends in the field of disease genetics and genomics. The DisGeNET score is an in-house developed metric that reflects how well established is a particular association based on current knowledge. The DisGeNET score gives the highest value to associations that are reported by several databases, in particular to those reported by expert curated resources, and with a large number of supporting publications. More details on how the score is calculated are provided in the online documentation (http://www.disgenet.org/dbinfo#GDAScore). Finally, both GDAs and VDAs are annotated with the Evidence Index (EI), which indicates the existence of contradictory results in the publications supporting the associations. This index is computed for the associations coming from BeFree and PsyGeNET (24), which identify the publications reporting a negative finding on a particular VDA or GDA. Note that only in the case of PsyGeNET the information used to compute the EI has been validated by experts. An EI equal to one indicates that all the publications support the GDA or the VDA, while an EI smaller than one indicates that there are publications that assert that there is no association between the gene/variants and the disease.
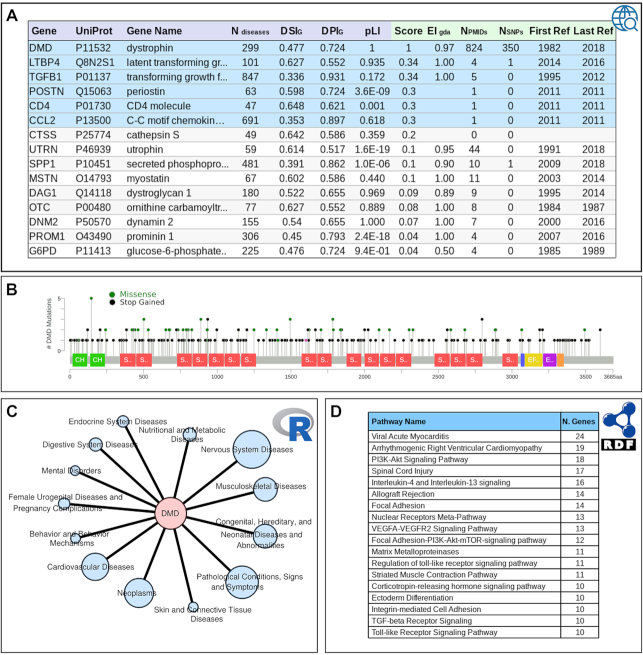
DisGeNET provides comprehensive information on genes and variants for rare diseases. (A) Highest scoring genes associated to Duchenne Muscular Dystrophy in DisGeNET. In blue background, the genes annotated by CURATED resources. (B) DisGeNET annotates 350 DMD variants to Duchenne muscular dystrophy, being most of them stop-gained variants. (C) The DMD gene is associated to a large number of diseases and phenotypes belonging to different disease classes. (D) Pathways associated with Duchenne Muscular Dystrophy obtained by a federated query interrogating DisGeNET and WikiPathways.
DisGeNET tools
DisGeNET is available via a suite of tools (Figure (Figure1B)1B) described in more detail in the next section.
The DisGeNET web interface
DisGeNET 6.0 benefits from a completely new web interface aimed at improving the user experience. The Search functionality of the web interface supports searches by single disease, gene, or variant, as well as lists of these entities. The Browse functionality allows exploring DisGeNET by the source databases, for example CURATED. The results of the searches are organized in tables providing different views of the information: summaries of GDAs and VDAs, evidence supporting the associations, or views focused on diseases, genes or variants. The results of the browsing or the initial searches can be furthered filtered and prioritized using a collection of flexible filters that can be used alone or in combination. For example, diseases might be filtered by UMLS semantic type, and/or by MeSH disease class. Genes might be filtered by protein class, or by values of the DPI, DSI or the pLI. The variants might be filtered by their consequence type, and their allelic frequency in exome and genome data. The results of the searches can be downloaded as tabulated or Microsoft Excel files, or shared by using a URL.
The DisGeNET REST API
DisGeNET 6.0 features a new REST application programming interface (API) that enables programmatic retrieval of the information contained in the platform. The base URL for the DisGeNET REST API is http://www.disgenet.org/api/. The services in the API allow to retrieve GDAs, VDAs, DDAs and attributes of genes, diseases, and variants in different formats (json, xml and tsv). Additionally, the API includes a service that provides mappings between different disease identifiers from a variety of sources such as UMLS, MeSH, OMIM, HPO, DO, MONDO, NCI and ICD-9.
The DisGeNET-RDF dataset
The DisGeNET-RDF Linked Dataset is an alternative way to access the DisGeNET data and enables the integration and joint querying of the DisGeNET data with other databases available as Linked Open Data (https://lod-cloud.net/). DisGeNET-RDF (8) encodes DisGeNET data using the Resource Description Framework (RDF) (http://www.w3.org/RDF/), which is a core part of Semantic Web standards. The main components of the DisGeNET-RDF dataset are the GDA and VDA datasets, metadata description of the RDF dataset (VoID description), and linkouts to other Linked Datasets. The RDF representation of DisGeNET (v6.0.0) contains 62,359,775 triples serialized in Turtle syntax that annotate the 628,685 GDAs and 210,498 VDAs contained in DisGeNET 6.0. Entities and properties are semantically defined using standard ontologies such as the National Cancer Institute thesaurus (NCIt), and resources identified by using de-referenceable IRIs. GDAs are integrated using the DisGeNET Association Type Ontology and they are semantically harmonized using SIO classes (25).
By relying on the web-based data representation and integration framework known as Linked Open Data, that constitutes the backbone of the Semantic Web, DisGeNET-RDF enables sharing and integration of the DisGeNET data with external resources such as databases on gene expression, drugs and chemicals, proteins, biological pathways and systems biology models. Through the SPARQL endpoint, query federation to interrogate DisGeNET in combination with these LOD resources is possible. Examples of research questions that can be addressed using DisGeNET-RDF are provided at disgenet.org/rdf. The DisGeNET-RDF API has been recently selected as one of the 10 interoperability resources recommended by ELIXIR (https://elixir-europe.org/platforms/interoperability/rirs), the European organisation that brings together bioinformatics resources in life sciences, to facilitate interoperability and reusability of life science data and support the principles of FAIR data management.
The DisGeNET Cytoscape App
The DisGeNET Cytoscape App contains a set of functions to query, analyze, and visualize DisGeNET data from a network biology perspective. The GDAs, VDAs and DDAs can be represented, queried and filtered as bipartite and monopartite networks. Note that VDAs are a new feature in this release of the DisGeNET App. The new version of the App includes functions to query DisGeNET data for specific diseases, genes, and variants, and their combinations, and for filtering the information by source, the DisGeNET score, DisGeNET association type, Evidence Index and disease class. Note that VDAs, the DisGeNET score and Evidence Index are a new feature in this release of the DisGeNET App. Another novelty is a function for the annotation of entities from foreign networks with DisGeNET data, such as protein, gene or variants generated by other Cytoscape Apps or networks uploaded by the user. Finally, the App features a new automation module that includes a set of functions to programmatically execute different functionalities using popular programming languages such as R and Python. In summary, with its newly implemented capabilities, the DisGeNET Cytoscape App provides the biomedical community a tool that enables a systems-level analysis of human diseases in an easy and automatic way.
The disgenet2r package
The disgenet2r package has been updated for the new database framework. The package allows to easily interact with both the REST API and the SPARQL API and provides a series of plots for the visualization of DisGeNET data which include networks, heatmaps and Venn diagrams. Additionally, a suite of functions has been incorporated to interrogate DisGeNET-RDF and perform federated queries such as the retrieval of all the pathways for a particular disease, or the search of the disease-associated proteins that are also drug targets.
Data downloads
The DisGeNET database is made available under the Attribution-NonCommercial-ShareAlike 4.0 International License. The complete database and different subsets of data are available for download as tabulated files (http://www.disgenet.org/downloads) as well as the results of specific searches carried out through the web interface.
Application examples of DisGeNET
Expanding information for rare diseases
DisGeNET integrates information from databases with a special focus on rare diseases (such as Orphanet) with data coming from other resources, significantly expanding the number of genes, variants and publications annotated to those diseases. An illustrative example of this expansion is presented here with Duchenne muscular dystrophy, a neuromuscular disease characterized by rapidly progressive muscle weakness and wasting. This severe, inherited X-linked recessive disease has no current treatment beyond symptoms management. Muscle damage is caused by absence of the sarcolemmal protein dystrophin as a result of DMD gene mutations.
Two genes are annotated to the disease in Orphanet (DMD, LTB4, https://www.orpha.net/consor/cgi-bin/Disease_Genes_Simple.php?lng=EN&LnkId=13913&Typ=Pat&diseaseType=Gen&from=rightMenu) and one in OMIM (DMD, https://www.omim.org/entry/310200?search=duchenne%20muscular%20dystrophy&highlight=duchenne%20dystrophy%20muscular). Contrastingly, DisGeNET provides 189 genes, 6 of them from CURATED resources (in blue in Figure Figure6A),6A), as well as 353 variants (most of them from ClinVar, an expert curated resource on clinical genomics). The top 15 genes ranked by DisGeNET score are shown in Figure Figure6A.6A. The DMD gene has the highest score, while the other genes have lower score mainly because they are reported in a lower number of databases and/or in fewer publications (column NPMIDS in the table shown in Figure Figure6A).6A). DisGeNET annotates 350 DMD variants to Duchenne muscular dystrophy, being most of them stop gained variants (Figure (Figure6B)6B) located throughout the protein coding sequence. An interesting example among the list of genes associated with Duchenne muscular dystrophy is the gene UTRN encoding the utrophin protein whose increased levels have previously been shown to compensate in part for the loss of dystrophin (26) and proposed to play a role as disease modifier. The role of utrophin in the disease has been studied since 1991 (44 publications listed in DisGeNET) and the effect of its expression is currently being investigated by genome editing technologies (27). DisGeNET also indicates that the DMD gene is associated to a large number of diseases and phenotypes (almost 300 UMLS CUIs), as reflected by its low DSI. It is associated with different types of muscular dystrophies (Duchenne and Becker Muscular Dystrophy), cardiovascular diseases (Dilated and Familial Cardiomyopathy), and mental diseases (Impaired Cognition and Intellectual Disability), among others (Figure (Figure6C).6C). By performing federated queries to jointly interrogate DisGeNET-RDF and WikiPathways (28), it is possible to identify the pathways associated with the disease (Figure (Figure6D).6D). Of note, pathways related to cardiomyopathy (Viral acute myocarditis, Arrhythmogenic Right Ventricular Cardiomyopathy, and Striated Muscle Contraction), spinal cord injury, and several signalling pathways, all processes related to the disease pathophysiology, concentrate the largest number of genes. In summary, DisGeNET significantly expands information on genes and variants associated to rare diseases, which can be exploited for development of clinical genomics pipelines in this area and supporting research and development of new therapies.
Analysis of NGS and GWAs data for complex diseases
DisGeNET can also be used for the analysis and interpretation of genomic data from studies on complex diseases and traits. A recent meta-analysis of GWAs identified 143 risk variants for type 2 diabetes (T2D) through the study of 62 000 T2D cases and 596 000 controls of European ancestry (29). DisGeNET, as a database that aggregates available knowledge on disease relevance of genomic variants, can aid in the analysis of the GWAs signals, in particular to identify those variants already reported to be associated with the disease or trait under study. From the list of 143 variants identified by Xue and colleagues, 61 are reported in DisGeNET as associated to cardiometabolic diseases and traits (Figure (Figure7A),7A), and from them, 47 variants are annotated to T2D. Notably, two of these variants (rs10401969, rs7674212) are reported as novel independent risk loci not previously associated to T2D by Xue and colleagues (see Table Table11 in ref. (29)), although there are publications that report their association to diabetes and metabolic traits dating from 2011 in DisGeNET (http://www.disgenet.org/browser/2/1/0/rs7674212::rs10401969/). For the disease associated variants, DisGeNET provides genomic information such as the consequence type of the variant according to VEP, allele frequencies from the gnomAD databases, along with detailed information on disease and phenotype annotation including the DisGeNET score, Evidence Index, number of supporting publications with linkouts to MEDLINE abstracts and the text excerpt asserting the VDA. In addition, for each VDA, the first and last year of reference publications are provided (Figure (Figure7B).7B). The DSI and DPI can be used to select variants according to their specificity for the disease (Figure (Figure7B).7B). Figure Figure7C7C shows the diseases associated with variant rs7903146, an intronic variant in the gene TCF7L2. The association with T2D has a high DisGeNET score (0.9) and is supported by 138 publications dating from 2006. Notably, the T allele of rs7903146 variants confers the strongest risk of T2D known to date in Caucasians (P < 10−347) (29), and is a common variant with AF of 0.26 in gnomAD. Moreover, the literature on VDAs captured in DisGeNET can provide insights on putative mechanisms by which the variant confer risk to the disease (by modification of the effect of incretins on insulin secretion (30–32)). In summary, DisGeNET, as a publicly available knowledge management tool and comprehensive database enables efficient analysis and interpretation of GWAs.
CONCLUSION
The DisGeNET platform is designed to allow easy exploration and analysis of the genetic underpinnings of the full spectrum of human diseases: Mendelian, rare and complex, as well as symptoms, signs and other phenotypic manifestations of diseases. The platform contains data from the most popular repositories in the field that have been enriched and expanded with information extracted from the scientific literature using state-of-the-art text mining tools and mostly not reported in any other repository.
The data in DisGeNET is harmonized and standardized by controlled vocabularies and ontologies following the FAIR principles, which enables an easy link with other biomedical resources. This interoperability is particularly facilitated by providing an RDF version of the DisGeNET database. In addition, the information is enriched with a series of in-house developed metrics and external attributes facilitating its interpretation and analysis, both manual and automatic. For a better assessment of the genotype-phenotype associations, DisGeNET provides information about their original source, links to the publications that support the associations, as well as a representative sentence of each publication. In addition to the possibility of downloading data in various formats, DisGeNET offers a series of bioinformatics tools to facilitate access and analysis of data: a web interface, a Cytoscape App, an R package and different APIs (SPARQL endpoint, Rest API, Cytoscape Automation).
Since its first release, DisGeNET has become an established and mature resource, broadly used by the biomedical community, enabling a wide variety of applications in the field of drug R&D, disease genomics and for the development of bioinformatic tools and databases.
DATA AVAILABILITY
DisGeNET is available at the following URLs:
Platform web site: http://www.disgenet.org/
Cytoscape App: https://apps.cytoscape.org/apps/disgenetapp
REST-API: http://www.disgenet.org/api/
RDF-API: http://rdf.disgenet.org/sparql/, http://rdf.disgenet.org/lodestar/sparql
R package: https://bitbucket.org/ibi_group/disgenet2r
FUNDING
ISCIII-FEDER [PI13/00082, PI17/00230, CPII16/00026]; IMI-JU resources of which are composed of financial contribution from the EU-FP7 [FP7/2007–2013] and EFPIA companies in kind contribution [116030 to TransQST, 777365 to eTRANSAFE], and the EU H2020 Programme 2014–2020 [676559 to Elixir-Excelerate]; Agència de Gestió d’Ajuts Universitaris i de Recerca Generalitat de Catalunya [2017SGR00519]. The Research Programme on Biomedical Informatics (GRIB) is a member of the Spanish National Bioinformatics Institute (INB), funded by ISCIII and FEDER (PRB2-ISCIII [PT13/0001/0023, of the PE I+D+i 2013–2016]). The DCEXS is a ‘Unidad de Excelencia María de Maeztu’, funded by the MINECO [MDM-2014-0370]. Funding for open access charge: Agència de Gestió d’Ajuts Universitaris i de Recerca Generalitat de Catalunya [2017SGR00519].
Conflict of interest statement. None declared.
REFERENCES
Articles from Nucleic Acids Research are provided here courtesy of Oxford University Press
Full text links
Read article at publisher's site: https://doi.org/10.1093/nar/gkz1021
Read article for free, from open access legal sources, via Unpaywall:
https://academic.oup.com/nar/article-pdf/48/D1/D845/31697865/gkz1021.pdf
Citations & impact
Impact metrics
Citations of article over time
Alternative metrics
Smart citations by scite.ai
Explore citation contexts and check if this article has been
supported or disputed.
https://scite.ai/reports/10.1093/nar/gkz1021
Article citations
A multi-omics Mendelian randomization study identifies new therapeutic targets for alcohol use disorder and problem drinking.
Nat Hum Behav, 11 Nov 2024
Cited by: 0 articles | PMID: 39528761
Network pharmacology to unveil the mechanism of Astragali Radix in the treatment of lupus nephritis via PI3K/AKT/mTOR pathway.
Sci Rep, 14(1):25983, 29 Oct 2024
Cited by: 0 articles | PMID: 39472740 | PMCID: PMC11522298
Study on the anti-atherosclerosis mechanisms of Tanyu Tongzhi formula based on network pharmacology, Mendelian randomization, and experimental verification.
Pharm Biol, 62(1):790-802, 25 Oct 2024
Cited by: 0 articles | PMID: 39450854 | PMCID: PMC11514399
Exploring the potential pharmacological mechanism of aripiprazole against hyperprolactinemia based on network pharmacology and molecular docking.
Schizophrenia (Heidelb), 10(1):105, 07 Nov 2024
Cited by: 0 articles | PMID: 39511179 | PMCID: PMC11544107
Mechanism of Yi-Qi-Bu-Shen Recipe for the Treatment of Diabetic Nephropathy Complicated with Cognitive Dysfunction Based on Network Pharmacology and Experimental Validation.
Diabetes Metab Syndr Obes, 17:3943-3963, 23 Oct 2024
Cited by: 0 articles | PMID: 39465123 | PMCID: PMC11512782
Go to all (1,108) article citations
Data
Data behind the article
This data has been text mined from the article, or deposited into data resources.
BioStudies: supplemental material and supporting data
Diseases
- (1 citation) OMIM - 310200
SNPs (3)
- (3 citations) dbSNP - rs7903146
- (1 citation) dbSNP - rs10401969
- (1 citation) dbSNP - rs7674212
Similar Articles
To arrive at the top five similar articles we use a word-weighted algorithm to compare words from the Title and Abstract of each citation.
DisGeNET: a discovery platform for the dynamical exploration of human diseases and their genes.
Database (Oxford), 2015:bav028, 15 Apr 2015
Cited by: 475 articles | PMID: 25877637 | PMCID: PMC4397996
DisGeNET: a comprehensive platform integrating information on human disease-associated genes and variants.
Nucleic Acids Res, 45(d1):D833-D839, 19 Oct 2016
Cited by: 1172 articles | PMID: 27924018 | PMCID: PMC5210640
The DisGeNET cytoscape app: Exploring and visualizing disease genomics data.
Comput Struct Biotechnol J, 19:2960-2967, 11 May 2021
Cited by: 173 articles | PMID: 34136095 | PMCID: PMC8163863
Development of bioinformatics resources for display and analysis of copy number and other structural variants in the human genome.
Cytogenet Genome Res, 115(3-4):205-214, 01 Jan 2006
Cited by: 140 articles | PMID: 17124402
Review
Funding
Funders who supported this work.
Agència de Gestió d’Ajuts Universitaris i de Recerca Generalitat de Catalunya
EU H2020 Programme (1)
Grant ID: 676559
EU-FP7 (1)
Grant ID: FP7/2007–2013
FEDER (1)
Grant ID: PT13/0001/0023
IMI-JU
ISCIII
ISCIII-FEDER (3)
Grant ID: PI13/00082
Grant ID: CPII16/00026
Grant ID: PI17/00230
MINECO (1)
Grant ID: MDM-2014-0370