Abstract
Free full text
Image‐guided mathematical modeling for pharmacological evaluation of nanomaterials and monoclonal antibodies
Abstract
While plasma concentration kinetics has traditionally been the predictor of drug pharmacological effects, it can occasionally fail to represent kinetics at the site of action, particularly for solid tumors. This is especially true in the case of delivery of therapeutic macromolecules (drug‐loaded nanomaterials or monoclonal antibodies), which can experience challenges to effective delivery due to particle size‐dependent diffusion barriers at the target site. As a result, disparity between therapeutic plasma kinetics and kinetics at the site of action may exist, highlighting the importance of target site concentration kinetics in determining the pharmacodynamic effects of macromolecular therapeutic agents. Assessment of concentration kinetics at the target site has been facilitated by non‐invasive in vivo imaging modalities. This allows for visualization and quantification of the whole‐body disposition behavior of therapeutics that is essential for a comprehensive understanding of their pharmacokinetics and pharmacodynamics. Quantitative non‐invasive imaging can also help guide the development and parameterization of mathematical models for descriptive and predictive purposes. Here, we present a review of the application of state‐of‐the‐art imaging modalities for quantitative pharmacological evaluation of therapeutic nanoparticles and monoclonal antibodies, with a focus on their integration with mathematical models, and identify challenges and opportunities.
This article is categorized under:
Therapeutic Approaches and Drug Discovery > Nanomedicine for Oncologic Disease
Diagnostic Tools > in vivo Nanodiagnostics and Imaging
Nanotechnology Approaches to Biology > Nanoscale Systems in Biology
Abstract
Pharmacological evaluation of nanoparticles and antibodies performed through quantitative imaging‐guided mathematical models.
1. INTRODUCTION
Following drug administration into an in vivo system, the interplay between the pharmacokinetic (PK) processes of absorption, distribution, metabolism, and excretion (ADME) governs the plasma (or systemic) concentration kinetics of the drug, which is traditionally regarded as a predictor of the therapeutic efficacy or toxicity of the given drug. Plasma kinetics has been used to evaluate the pharmacological exposure‐response relationships under the assumption that plasma concentration is a surrogate for drug concentration at the site of action (Gabrielsson & Weiner, 2001). However, due to challenges associated with drug chemistry, dosage form, inter‐individual variability, or biological differences between healthy and diseased tissues, drugs may not effectively reach the site of action to achieve a therapeutic concentration locally, despite achieving a therapeutically relevant concentration systemically (Nizzero, Ziemys, & Ferrari, 2018; Rizk, Zou, Savic, & Dooley, 2017). This can result in differential therapeutic responses across treatments, or across patients, and can often lead to therapeutic resistance (T. Brocato et al., 2014), suggesting that the plasma concentration does not always reflect the target site concentration of the drug and highlighting the importance of target site concentration as the true predictor of drug effects. Further, assessment of therapeutic concentration at the target site becomes increasingly relevant when nanomaterials such as nanoparticle (NP) drug carriers or nano‐sized therapeutic monoclonal antibodies (mAbs) are involved. This is primarily due to the additional physical constraints imposed by biological barriers that impede the transport of these nano‐sized entities to the site of action due to their large particle size compared to free drugs (Dreher et al., 2006; Nizzero, Shen, Ferrari, & Corradetti, 2019; Schmidt & Wittrup, 2009; Tang et al., 2014; Thurber, Schmidt, & Wittrup, 2008; Thurber, & Weissleder, 2011), thereby creating greater disparity between plasma concentration and target site concentration for these macromolecules (for the sake of brevity, wherever feasible, we will collectively refer to NPs and mAbs as macromolecules throughout this manuscript).
The plasma concentration kinetics of macromolecules provides an incomplete understanding of their whole‐body distribution kinetics, as macromolecules do not achieve uniform dispersion across tissues, primarily due to their predominant distribution and prolonged retention in the liver and spleen (Dogra et al., 2018; L. Liu, 2018; Tsoi et al., 2016). It thus becomes imperative to accurately assess the concentration kinetics of macromolecules discretely at the site of action, in addition to unravelling the whole‐body kinetics for a complete understanding of their in vivo disposition. To date, efforts towards this end have been hindered by challenges associated with the assessment of therapeutic concentrations in the tissues or biological fluids of interest (Rizk et al., 2017), and plasma concentration kinetics has remained a popular choice for pharmacological evaluation of macromolecules. Advancements in non‐invasive in vivo imaging (Ding & Wu, 2012; Smith & Gambhir, 2017) are helping to overcome these limitations, and it is now becoming increasingly common to conduct whole‐body imaging over time (Dogra et al., 2018) to investigate the diseased site kinetics of NPs (Goel et al., 2019; Meng, Wang, Ping, & Yeo, 2018) and mAbs (Cole et al., 2017; Niemeijer et al., 2018), and thus obtain a comprehensive understanding of the PK behavior of macromolecules, which can explain the therapeutic benefits or insufficiencies associated with these xenobiotics (Ng, Garlin, Weissleder, & Miller, 2020).
Several imaging modalities are available to quantify macromolecules at the diseased site or in healthy compartments of the body in both preclinical and clinical settings. This is typically achieved by labeling the macromolecules with imaging agents; these are generally small molecules that, upon stimulation with an input signal, or without, produce a reporter signal that is recognized by the receiver device of the imaging modality to produce a time‐varying image sequence across the body (Smith & Gambhir, 2017). The most commonly used quantitative imaging modalities for living systems that are discussed in detail in this article are magnetic imaging, nuclear imaging, and optical imaging. These modalities can vary considerably in their spatiotemporal resolution, penetration depth, and sensitivity (see Figure Figure1),1), thereby providing guidelines for the selection of the appropriate modality for a given macromolecule. The non‐invasive nature of these modalities improves patient compliance and allows for the investigation of macromolecules in an unperturbed in vivo environment. The series of images obtained through non‐invasive imaging can be quantified to guide the development of empirical or mechanistic mathematical models. The former modeling approach can be used to describe the in vivo disposition kinetics and pharmacodynamics of macromolecules, and the latter can be used as an in silico tool to investigate the role of physicochemical or pathophysiological factors in affecting the pharmacology of macromolecules, in addition to predicting their spatiotemporal concentration dynamics. The mathematical models thus developed can vary significantly in terms of the time‐ or length‐scales they represent (Dogra, Butner, et al., 2019), which are selected by the modeler based on the biological system being modeled and any limitations of the spatiotemporal resolution of the available imaging data for model parameterization and validation, among other factors.

Spatiotemporal resolution, penetration depth, and sensitivity of the key imaging modalities in (a) clinical and (b) preclinical settings. Temporal resolution is represented by solid (few seconds to minutes), dashed (few minutes), and dotted (few minutes to hour) boundaries of the cuboids whose color denotes the imaging modality‐type, and the x‐, y‐, and z‐axis represent spatial resolution, penetration depth, and sensitivity, respectively. Key: MRI, magnetic resonance imaging; PET, positron emission tomography; SPECT, single photon emission computed tomography; biolum., bioluminescence; fluor., fluorescence
Increased application of non‐invasive quantitative imaging will likely allow for the development of new therapeutics, improve diagnosis, and allow real‐time monitoring of treatment and its optimization. In the following, we will i) discuss the biophysical factors that govern the transport of macromolecules, thereby affecting their pharmacology, ii) present the key imaging modalities that are used in vivo or in the clinic for macromolecule visualization and estimation of model parameters, and iii) review key imaging‐driven mathematical models for pharmacological evaluation of macromolecules intended for therapeutic, diagnostic, or theranostic (e.g., hybridized therapeutic and diagnostic) applications in cancer.
2. TRANSPORT PHYSICS
Efficient delivery of drugs, or macromolecules, to the site of action is essential to produce the desired pharmacological effects. Delivery to the target site is dominated by the physical transport phenomena of perfusion, microvascular extravasation, and interstitial migration, which represent the key transport processes in the vascular, transvascular, and interstitial phases of delivery, respectively (see Figure Figure2)2) (R. K. Jain & Stylianopoulos, 2010). Perfusion refers to the passage of blood through the vascular network of an organ or tissue. It is quantified as the blood flow rate per unit mass of organ (units, ml/min/g), is a property of the biological system, and varies across tissues and across species (Shah & Betts, 2012). Blood flow rate Q can be expressed as the ratio of the pressure difference between arterial and venous ends (ΔP), and flow resistance (FR):
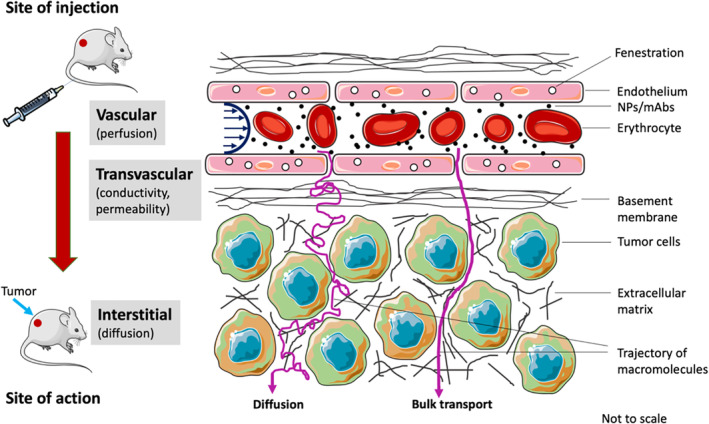
Transport of macromolecules from site of injection to target site. Delivery of nanoparticles and monoclonal antibodies to the site of action is characterized by perfusion, hydraulic conductivity, permeability, and diffusion. (Reprinted with permission from Dogra, Butner, et al. (2019). Copyright 2019 Springer)
(R. K. Jain & Stylianopoulos, 2010). Flow resistance is a product of apparent viscosity (due to high erythrocyte density) and geometrical resistance, which tends to be greater in tumors compared to healthy tissues, due to vessel deformation and abnormalities, thus lowering perfusion. As such, the perfusion‐dependent influx rate of macromolecules into the region of interest (ROI) is defined by the product of Q and macromolecule concentration in the systemic circulation.
The capillary microvasculature is a highly dynamic region of the vascular system, due to its involvement in the exchange of nutrients and waste materials between the blood and interstitial space. This exchange is feasible due to certain microanatomical features of the capillaries, such as interendothelial gaps and cellular pores or fenestrations within the single endothelial cell membrane that forms the capillary wall (Sarin, 2010). These properties allow fluid filtration through the capillary wall and make it semipermeable to solutes. There are two key mechanisms through which transvascular extravasation of substances occurs: diffusion and bulk transport. Small molecules can cross the capillary wall via diffusion due to the concentration gradient across the wall. However, due to size constraints, macromolecules do not diffuse as effectively across the capillary wall, but instead primarily rely on bulk transport to extravasate into the interstitium. Bulk transport occurs because of net filtration of fluid, generally out of the capillary into the interstitium due to the difference in hydrostatic and osmotic pressures on the two sides of the capillary wall, and is the dominant mechanism of transvascular flow of macromolecules in healthy tissues.
The combined effect of diffusion and bulk transport that governs the flux J of macromolecules through the capillary wall into the interstitium can be expressed as (R. K. Jain & Stylianopoulos, 2010):
where P represents vascular permeability, S is the vascular surface area, Cp is the macromolecule concentration in systemic circulation, Ci is the interstitial concentration of macromolecules, L is hydraulic conductivity, σ is the reflection coefficient, pv−
pi represents the difference between hydrostatic pressures between microvasculature and interstitial space, and πv
−
πi is the difference in osmotic pressure across the wall. The first part of Equation 2 accounts for diffusion‐mediated macromolecular extravasation, and the second part accounts for bulk transport mediated extravasation. P, L, and σ are critical transport parameters that govern the tendency of a substance to undergo transvascular extravasation. Diffusion‐dependent extravasation depends upon the permeability of the wall to the solute, which is a function of both vascular characteristics and the xenobiotic of interest, in particular the size and surface charge of the solute relative to the capillary wall porosity and charge. Conductivity, a measure of how effectively fluid can extravasate into the interstitium, is a function of the porosity of the microvasculature, and determines the bulk‐transport mediated extravasation of macromolecules based on the reflection coefficient of the wall, which is a function of the relative size and surface charge of the solute and capillary wall pores. (As a reference, while free drugs are generally <1 nm in size, mAbs are generally ≤10 nm wide, and NPs may range in diameter from 1–100 nm; the size of microvascular fenestrations may vary from 1–5 nm in brain, lungs, and muscle, ~6–10 nm in kidneys, ~180–280 nm in liver, and ~5 µm in spleen (Sarin, 2010)). Of note, the build‐up of interstitial fluid pressure due to poor lymphatic drainage and solid stress due to growth in a tumor causes the hydrostatic pressure difference between the vasculature and interstitium to diminish. As a result, diffusion is the key mechanism of macromolecular extravasation in tumors (R. K. Jain & Stylianopoulos, 2010). Further, as demonstrated by Wittrup et al., the concentration of macromolecules attained in the tumor interstitium is much lesser than the plasma (systemic) concentration of macromolecules due to permeability being the limiting factor in the diffusive extravasation of macromolecules (Thurber et al., 2008).
Once macromolecules have crossed the capillary wall into the interstitium, they are transported via bulk transport with the interstitial fluid, or through diffusion to reach the target cells, represented as (R. K. Jain & Stylianopoulos, 2010):
where v is the interstitial fluid velocity, D is the diffusion coefficient of the macromolecules, and the term R accounts for the sinks that cause macromolecular binding and degradation in the interstitium. However, due to elevated interstitial fluid pressure and solid stress, diffusion is the primary mechanism of transport in the tumor interstitium (Boucher & Jain, 1992; Pascal et al., 2013; Stylianopoulos et al., 2013). As a result, diffusion is the primary mechanism of transport in the tumor interstitium (Pascal, Bearer, et al., 2013). Finally, once in the proximity of cells, macromolecules maybe internalized through clathrin‐dependent/independent endocytosis,caveolin‐dependent/independent endocytosis, or receptor‐mediated endocytosis, depending upon their size and surface characteristics (Belleudi et al., 2012; Perera et al., 2007; S. Zhang, Gao, & Bao, 2015; Xiao, & Gan, 2013).
As described, many factors during the transvascular and interstitial transport ultimately affect the accumulation of macromolecules in tumors, demonstrating why plasma concentration kinetics cannot serve as an accurate surrogate for the tumor site concentration kinetics, and highlighting the importance of estimating macromolecular concentration at the site of action. In vivo imaging is a powerful tool to visualize the dynamics of macromolecules and to understand their transport via the processes involved in delivery to the site of action, which may help to overcome the limitations of plasma concentration kinetics in PK studies. Imaging can also help quantify the various parameters involved in the transport process, and thus guide the development of mathematical models for descriptive and predictive purposes. We now discuss state‐of‐the‐art imaging modalities that are utilized to investigate the pharmacology of macromolecules.
3. STATE‐OF‐THE‐ART IMAGING MODALITIES FOR NPs AND mABs
3.1. Magnetic imaging
Over the past decades, the application of magnetic resonance imaging (MRI) has expanded significantly in clinical practice. MRI is a non‐ionizing imaging modality that uses a strong magnetic field and radio waves to produce detailed images of anatomical structures. It is based on the phenomenon of nuclear magnetic resonance (NMR), which involves the perturbation of spin‐alignment of nuclei in a strong magnetic field through the application of radio frequency (see Figure Figure3).3). The nuclear spin of atoms in a strong and constant magnetic field is either oriented parallel or anti‐parallel to the external magnetic field. When this system is subject to an electromagnetic pulse (e.g., radio waves) at resonance frequency (also called Larmor frequency), it excites the nuclei to a higher energy state such that their spins are flipped and oriented anti‐parallel to the magnetic field, and are now referred to as being in resonance with the magnetic field, hence the name NMR. Subsequently, when the electromagnetic field is switched off and the nuclei relax, they revert to their equilibrium state (parallel spin orientation) that is accompanied by the release of energy in the form of radio waves that can be captured by antennas to produce an image (Bushberg & Boone, 2011). Due to the abundance of water and fat in biological tissues, clinical MRI is based on the NMR of hydrogen ions (protons). MRI produces high‐resolution images by measuring the spin magnetization of protons and their respective longitudinal (T1) and transverse (T2) relaxation rates. MRI provides high spatial resolution and sufficient penetration depth for clinical imaging, with high contrast‐to‐noise ratio but low sensitivity (Figure (Figure1).1). Paramagnetic contrast agents (refer to Table Table1)1) that interfere with local magnetism are often injected into the patient, resulting in changes in proton density and spin characteristics that enhance the contrast of MRI images.

Schematic representation of nuclear magnetic resonance, the working principle of magnetic resonance imaging
TABLE 1
Summary of key magnetic resonance imaging (MRI)‐contrast enhancement agents and the corresponding macromolecules investigated through MRI
Commercial names of contrast agents with t 1/2 | NPs and mAbs investigated preclinically or clinically | References |
---|---|---|
Gadolinium‐based probes | ||
|
| Detappe et al. (2017), Mortezazadeh et al. (2019), Sharma et al. (2012), and Warram et al. (2014) |
Iron oxide‐based probes | ||
|
| Hope et al. (2015), Warram et al. (2014), and Xu et al. (2019) |
Abbreviations: mAb, monoclonal antibody; NP, nanoparticle; t 1/2, circulation half‐life.
MRI has been applied both preclinically and clinically for non‐invasive pharmacological assessment of macromolecules intended for applications in cancer treatment. Alsaid et al. (2017) used contrast enhanced MRI to study the impact of a mAb AccretaMab (anti HER3) on its HER3 positive tumor growth inhibitory effects in human xenograft tumor‐bearing mice. Using ultrasmall superparamagnetic iron oxide (USPIO) NPs (ferumoxytol) as contrast agent, they were able to detect immune system anti‐tumor response in the form of macrophage recruitment in mice treated with the novel antibody in contrast to the negative control, as assessed by greater reduction in signal‐to‐noise ratio after ferumoxytol injection. Palm et al. (2003) studied the in vivo disposition kinetics of trastuzumab mAb (anti‐HER2/neu) in mice bearing ovarian carcinoma using positron emission tomography (PET) imaging coregistered with standard MRI. Co‐registration with MRI was done to confirm the site of trastuzumab uptake (intra‐tumoral). The study revealed rapid systemic uptake of the mAbs following intraperitoneal injection, followed by tumor localization. Ramanathan et al. (2017) used the tumor deposition characteristics of ferumoxytol, evaluated through ferumoxytol enhanced MRI, to assess the therapeutic efficacy of a nanoliposomal irinotecan formulation in patients with advanced solid tumors. Their findings suggest a significant positive correlation between tumor accumulation of ferumoxytol at early time points and tumor regression caused by the therapeutic NPs. Together, these studies demonstrate the applicability of ferumoxytol enhanced MRI as a noninvasive biomarker to predict the efficacy of NP‐based drug delivery in solid tumors.
The challenges of using MRI in assessing the therapeutic effect of macromolecules include long image acquisition time and low sensitivity. Contrast‐enhanced MRI is also limited by rapid clearance of the contrast agent, and images obtained through this modality usually can only be collected for a short time window. Additionally, in the case of the work presented here, super paramagnetic iron‐oxide (SPIO) NP‐based MRI gives dark signals in the ROI that are sometimes difficult to differentiate from artifacts or local field inhomogeneity.
3.2. Nuclear imaging
Nuclear imaging modalities such as PET and single‐photon emission computed tomography (SPECT) utilize the γ‐ray signal emitted from radionuclides to visualize macromolecules in vivo. The superior properties of the γ‐ray signal, including excellent sensitivity, no limitation on tissue penetration, and facile quantification, have made nuclear imaging a prominent non‐invasive imaging tool (Figure (Figure1).1). With established radiolabeling technologies and the increased availability of radionuclides approved by the U.S. Food and Drug Administration (FDA) (Table (Table2),2), great interest has been spurred to deploy nuclear imaging for pre‐clinical assessment of the PK of macromolecules (F. Chen et al., 2016; Goel et al., 2019; Madru et al., 2012; Niemeijer et al., 2018).
TABLE 2
Summary of key radioisotopes and the corresponding macromolecules investigated through nuclear imaging
Commercial names of probes with t 1/2 a and D 1/2 a | NPs and mAbs investigated preclinically or clinically | References |
---|---|---|
Radioisotopes for PET imaging | ||
18F | ||
|
| Devaraj, Keliher, Thurber, Nahrendorf, and Weissleder (2009), Keinanen et al. (2017), Sirianni et al. (2014), and Zhu et al. (2014) |
64Cu | ||
|
| Du et al. (2019), Guo et al. (2015), Kobayashi et al. (2015), D. E. Lee, Choi, et al. (2013), Z. Liu et al. (2007), Mukai et al. (2019), Shi et al. (2017), Zhu et al. (2018) |
89Zr | ||
|
| Abou et al. (2013), Heskamp et al. (2017), McDonagh et al. (2018), Ruggiero et al. (2010) |
Radioisotopes for SPECT imaging | ||
99mTc | ||
|
| Dumoga et al. (2017), Morales‐Avila et al. (2011), Rainone et al. (2017), Song et al. (2017), Xing et al. (2019) |
111In | ||
|
| Banerjee et al. (2017), Chatterjee, Lesniak, and Nimmagadda (2017), Dogra et al. (2018), Helbok et al. (2010), Jones, Douglas, Shields, and Merkel (2018) |
131I | ||
|
| J. Cao et al. (2019), Chrastina and Schnitzer (2010), Kaminski et al. (1996), Press et al. (1993) |
Abbreviations: PEI, polyethyneimine; PS, polystyrene; PLA, poly(lactic acid); PLGA, poly(lactic‐co‐glycolic acid); PEG, polyethylene glycol; PGA, polyglutamic acid; MWCNT, multi walled carbon nanotubes; QDs, quantum dots; PGA, pteroylglutamic acid; t 1/2, circulation half‐life; D 1/2, radioactive decay half‐life.
Specific radionuclides, such as technetium‐99m (Madru et al., 2012), copper‐64 (64Cu) (Davis et al., 2017), indium‐111 (Dogra et al., 2018; Josefsson et al., 2016; Lindenberg et al., 2015), and zirconium‐89 (89Zr) (Börjesson et al., 2009; Dijkers et al., 2010; Pérez‐Medina et al., 2015), are preferred to conduct PK studies of macromolecules (Farzin, Sheibani, Moassesi, & Shamsipur, 2019) because of similarities between the timescale of the PK processes and the decay half‐life of the radionuclides. For instance, using 89Zr‐labeled PET imaging, specificity of high‐density lipoprotein NPs for tumor‐associated macrophages and their PK was assessed in an orthotopic mouse model of breast cancer (Pérez‐Medina et al., 2015). Meanwhile, F. Chen et al. (2013) pioneered the use of 64Cu‐labeled silica NPs to image tumor‐specific delivery with PET. In addition, it became feasible to establish the effects of NP properties on their in vivo disposition using imaging‐based PK derived from in vivo SPECT/CT imaging and ex vivo radiation dosimetry biodistribution (F. Chen et al., 2013; Dogra et al., 2018). Similarly, PET and SPECT have also been largely applied to determine the optimal dosage, biodistribution, and tumor uptake of mAbs in cancer patients. Of note, quantification of uptake in patients with metastatic breast cancer was feasibly assessed for 89Zr‐labeled mAb trastuzumab using PET imaging (Dijkers et al., 2010), 89Zr immuno‐PET imaging was practiced to assess safety of 89Zr‐labeled mAb U36 in patients with head and neck squamous cell carcinoma (Börjesson et al., 2009), and PK studies of PD‐1/PD‐L1 mAb using radiolabeled PET/SPECT highlighted new opportunities to optimize and monitor the efficacy of immune checkpoint blockade therapy (Josefsson et al., 2016; Maute et al., 2015; Natarajan et al., 2015; Teng, Meng, Kong, & Yu, 2018).
Obtaining highly precise measurements of macromolecule distributions via PET/SPECT imaging may be compromised by in vivo integrity or stability of the radiolabeling, which can result in dissociation of the radiolabel molecules from the macromolecules of interest, as these dissociated radioisotope molecules are still detected by the ultrasensitive γ‐ray signal (Goel, Chen, Ehlerding, & Cai, 2014). The mismatched biodistribution between macromolecules and the dissociated radionuclides may lead to incorrect assessment of macromolecule biodistribution from the in vivo imaging data. Also, high uptake of free 64Cu in liver and bladder, and significant uptake of free 89Zr in bones when dissociated from unstable radiolabeled macromolecules has been reported and can pose challenges in accurate assessment of PK (Deri, Zeglis, Francesconi, & Lewis, 2013; Y. Wang et al., 2012; Y. Zhang, Hong, & Cai, 2011). To overcome this problem, efforts were devoted to develop chelate‐free radiolabeling technologies, such as intrinsically radiolabeled NPs (F. Chen et al., 2015; Wall et al., 2017), protected surface coatings for radio‐stability of NPs (Frellsen et al., 2016). Also, novel chelators were developed for stable radionuclide‐mAb conjugates (Deri et al., 2014; Zhai et al., 2015). In any case, radiolabeling must be approached carefully, as radiolabeling may modify the surface properties of macromolecules and thus affect their PK. For example, systematic study of 64Cu‐labeled engineered antibody fragment ch12.18‐ΔCH2 with various chelators showed increased uptake in kidney with increased net positive charge of radiolabeled chelators (Dearling et al., 2015). Also, it is worth noting that, in practice, the fundamental spatial resolution limits of nuclear imaging often necessitate coscanning with an anatomical imaging modality such as CT. This spatial resolution limit may also be overcome by using integrated PET with MR multimodality imaging, which enables coregistration of morphologic and multifunctional information (Schlemmer et al., 2008). In yet another approach, Wall et al. (2017) attempted to expand the macroscopic resolution at organ level by integrating PET imaging with surface‐enhanced resonance Raman scattering imaging to obtain high‐precision intraoperative imaging.
3.3. Optical imaging
While their clinical application remains challenging, optical in vivo imaging systems (IVOI) are widely employed in preclinical studies to evaluate the biodistribution of a variety of compounds, including mAbs, cells, NPs, and microparticles (Martelli, Lo Dico, Diceglie, Lucignani, & Ottobrini, 2016). This is accomplished by fluorescent or bioluminescent labeling onto the entities that are to be visualized or tracked, and is often advantageous over other labeling approaches previously discussed due to the ease of conjugation and broad availability of commercial compounds and modification protocols to label non‐fluorescent macromolecules. The two main imaging modalities used in IVOI rely on the corresponding physical phenomena of fluorescence and bioluminescence. The working principle of Stoke's shift fluorescence emission is reported in Figure Figure4a4a (for brevity, we have chosen not to provide a detailed discussion of the mechanistic underpinnings of resonance fluorescence here), where a photon of energy E ~ λ ex −1 excites the ground state of a fluorescence compound (S 0) to its excited state S*. Following non‐radiative relaxation to the radiative excited state S r*, a photon of energy E em ~ λ em −1 is emitted. Similarly, the working principle of bioluminescence is reported in Figure Figure4b.4b. In bioluminescence, the excitation energy is typically produced by a chemical reaction occurring between an enzyme (luciferase) and a light‐emitting molecule (called a substrate; e.g., luciferin) in the presence of oxygen, which is necessary for the enzyme to oxidize the substrate. Regardless of the excitation or emission mechanism, in IVOI applications, emitted photons are then captured by a detector that converts their energy into an electric signal proportional to the number of emitting molecules found in the sample.

Non‐invasive optical imaging methods to evaluate in vivo biodistribution. (a) Principles of fluorescence and bioluminescence imaging. (b) Representative in vivo and ex vivo fluorescence imaging (IVIS Spectrum). (c) Geometrical demonstration of Abbe's diffraction limit for optical methods. (d) Biodistribution of fluorescent porous silica microdisks estimated via fluorescent signal (IVIS spectrum) vs quantitative optical imaging (homogenized organs). Left y‐axis refers to %ID/g as measured with quantitative optical imaging, right y‐axis to fluorescence intensity as measured via IVIS. Results are shown after background subtraction. (Reproduced with permission from Nizzero et al., 2019. Copyright 2019 Elsevier)
IVOI techniques are well‐established methods to study how the tumoritropic or organotropic potential of macromolecules influences the concentration kinetics at the target site. Under certain conditions, 3D image processing tools can also be used to reconstruct the geometrical volumetric features of distribution of compounds or cells from IVOI data, as shown by Abdelwahab, Sankar, Preul, and Scheck (2011) for mouse glioma development. While some materials exhibit intrinsic luminescence or fluorescence, the majority of compounds of interest for medical applications require additional chemical modification or conjugation with optically active compounds (Table (Table3).3). Analogous to the radiolabeling molecules previously discussed, fluorescent source molecules must be carefully selected, and care must be taken to attach them to their labeled substrates without changing the substrate functionality. It is established that fluorescent labeling may potentially alter the in vivo compound biodistribution, as demonstrated by Cilliers, Nessler, Christodolu, and Thurber (2017) for two clinically approved antibodies, trastuzumab and bevacizumab. In another notable example, (Peterson, Wilson, Huang, Dimasi, and Sachsenmeier (2016) showed that in vivo antibody biodistribution results varied according to the near‐infrared (NIR) labeling fluorophore emission wavelength used.
TABLE 3
Summary of fluorescent probes and the corresponding macromolecules investigated through optical imaging
Fluorescent probes | NPs and mAbs investigated preclinically or clinically | References |
---|---|---|
|
| Kumar et al. (2010), J. J. Lee, White, Rice, and Smith (2013), Meng et al. (2018), Popovic et al. (2010), W. Zhu et al. (2019) |
Abbreviations: PLGA, poly(lactic‐co‐glycolic acid); mAb, monoclonal antibody; NP, nanoparticle.
Despite wide use in preclinical studies, non‐invasive optical imaging methods suffer from the unavoidable attenuation effects due to interaction of emitted light with biological matter (Nizzero et al., 2019). While optical imaging resolution can be pushed down to the Abbe diffraction limit (Figure (Figure4c)4c) for confocal microscopy setups, the resolution for in vivo IVOI is generally poorer (Figure (Figure1)1) (Arms et al., 2018). Approaches to minimize this attenuation effect include the use of NIR dyes (λ
ex/λ
em =600 nm–1,000
nm) that can penetrate biological matter up to a few cm of tissue (Coll, 2011; Y. Liu, Tseng, & Huang, 2012; Vats et al., 2017). Alternatively, quantitative results can be obtained with ex vivo tissue optical methods that measure fluorescence from homogenized organ tissues (Bernhard et al., 2018; Mattu et al., 2018; Oliveira et al., 2012; Wolfram et al., 2017).
However, these invasive methods are challenging to translate to clinical practice. This has been recently demonstrated in a new bioluminescent reporter compound that was developed for in vivo imaging of extracellular vesicles (Gangadaran et al., 2017). This ex vivo study revealed similar accumulation between lung and liver tissues, followed by spleen and kidneys, in contrast to data collected at similar time points via in vivo measurements that showed the highest accumulation occurs in lungs, followed by liver, kidneys, and then spleen. In a different study, Nizzero et al. (2019) also observed tissue attenuation effects in IVOI by directly comparing biodistribution of the fluorescent porous silica microdisks as estimated with IVIS Spectrum with the percent of injected dose (%ID) calculated by fluorescence measured in homogenized organs (Figure (Figure4d).4d). Notably, the two techniques differ in their ability to remove bias due to tissue attenuation of fluorescent signal. In fact, conversion to absolute units (%ID/g) is only possible with the homogenized organ technique, by calculating attenuation factors in each organ through the use of a calibration curve. This is not possible with IVOI, so relative units (such as radiance) must be used for comparison. Thus, while a helpful tool to quickly compare in vivo targeting capabilities and PK of macromolecules and different compounds (Arms et al., 2018), the validity of noninvasive in vivo optical imaging methods to evaluate biodistribution may likely be considered semi‐quantitative at best (Nizzero et al., 2019).
4. IMAGE‐GUIDED MATHEMATICAL MODELING
Mathematical modeling has been increasingly perceived as a valuable tool in advancing a variety of research fields in nanotechnology and nanomedicine (Dogra, Butner, et al., 2019). Models informed by proper parameter quantification and validated through testing with different forms of multidimensional data can be used to provide quantitative mechanistic insights into, and to establish important quantitative correlations between, the biological, biophysical, and biomechanical processes of a particular biological or clinical problem that occur on or across multiple scales in time and space. In the field of cancer research in particular, biologists and medical scientists have been using mathematical models to generate new experimentally testable hypotheses, predict complex behaviors of the biological system of interest, and optimize drug delivery and therapeutic effect, thereby providing potentially clinically useful insights into different treatment systems (Cristini, Koay, & Wang, 2017; Dogra et al., 2019; Z. Wang, Butner, Kerketta, Cristini, & Deisboeck, 2015; Z. Wang & Deisboeck, 2019).
Mathematical models of NP and mAb delivery and their therapeutic effects in tumors have been integrated with and informed by in vivo and in vitro imaging of biodistribution and tumor kill, and are yielding valuable insights into the mechanisms of action of these cancer therapies (Clancy et al., 2016; Haddish‐Berhane, Rickus, & Haghighi, 2007). As discussed previously, the delivery of macromolecules to the site of action can be discretized into three key steps: i) blood‐flow driven vascular transport to the organ of interest, ii) transvascular migration into the interstitium, and iii) and interstitial diffusion. The key parameters that characterize these processes can be quantified through in vivo imaging to parameterize mathematical models for quantitative pharmacological evaluation of macromolecules (see Table Table44).
TABLE 4
List of key physiological parameters quantifiable through in vivo imaging
Parameter | Units | Imaging techniques | Source |
---|---|---|---|
Blood flow rate | ml/min | Doppler tomography; Tracer kinetics analysis of dynamic contrast enhanced MRI; functional MRI (specifically for cerebral blood flow) | Borogovac and Asllani (2012), Z. Chen et al. (1997), Henderson et al. (2000), O'Connor et al. (2011) |
Vascular volume or blood volume fraction | ml | Dynamic contrast enhanced MRI | Henderson et al. (2000) and Hindel et al. (2017) |
Extravascular volume | ml | Contrast enhanced MRI, computed tomography | Bandula et al. (2013), Kim, Savellano, Savellano, Weissleder, and Bogdanov (2004), Scully, Bastarrika, Moon, and Treibel (2018) |
Permeability | cm/s | Fluorescence microscopy; dynamic contrast enhanced MRI | Henderson et al. (2000), Reyes‐Aldasoro et al. (2008) |
Permeability‐surface area product | ml/min/100![]() | MRI; computed tomography; dynamic contrast enhanced MRI | Cha et al. (2006), R. Jain et al. (2008), Tofts et al. (1999), Weidman et al. (2016) |
Lymph flow rate | ml/min | Doppler optical coherence tomography; ultrasound; MRI; computed tomography | Blatter et al. (2016), F. Zhang, Niu, Lu, and Chen (2011), J. Zhu et al. (2019), Ruiz‐Ramírez, Ziemys, Dogra, and Ferrari (2020) |
Cellular uptake rate | 1/s | Optical imaging | Ahmed et al. (2017), Au et al. (2009), Liao‐Chan et al. (2015), van der Zwaag et al. (2016) |
Abbreviation: MRI, magnetic resonance imaging.
Derivation of prognostically correct and clinically meaningful information from imaging data often presents a significant challenge, in part due to the multiple interpretations that can arise from a single data set and lack of standardized data interpretation paradigms in many cases (Livingstone & Salt, 2005). This may be overcome by the construction of semi‐mechanistic or mechanistic mathematical models that not only fit the observations, but that can also offer some degree of predictive power (Brooks & Tobias, 1996). To date, a variety of mathematical models have been applied to the study of NP and mAb biodistribution kinetics, including physiologically based pharmacokinetic (PBPK) models (Abuqayyas & Balthasar, 2012; Dogra et al., 2018; Kuepfer et al., 2016; Li, Al‐Jamal, Kostarelos, & Reineke, 2010; Pascal, Ashley, et al., 2013; Shah & Betts, 2012; Z. Wang, Butner, Cristini, & Deisboeck, 2015) and other novel mechanistic models (Haddish‐Berhane et al., 2007; Sciumè et al., 2012; Sciumè et al., 2013), which are engineered to possess particular advantages over the others, depending on the nature of the problem under investigation.
A PBPK model is a mechanistic model that consists of a network of compartments that represent physiological volumes of the biological ROI, such as an organ, a tissue, or a tumor. These systems exchange quantifiable properties of interest, for example, blood, nutrients, drug molecules, macromolecules, etc., with a specific set of neighboring compartments, as determined by the underlying anatomy. Possible mechanisms of transport between the connected compartments include diffusion (Godin et al., 2010), convection (Y. Cao, Balthasar, & Jusko, 2013), or their combination, where the spatial dependency is homogenized through a lumping process that consists of integration over space, effectively reducing bivariate components (space and time) to time‐dependent averages (Thompson & Beard, 2011). Once the PBPK network has been defined, the complex reactions that take place within each compartment, and their interactions with and effects on surrounding neighbors, are described with a set of time dependent equations. PBPK models are easy to implement and modify (McNally, Cotton, & Loizou, 2011), robust numerical tools exist to solve them (Blancato, 1994), and several simulation and development packages that facilitate their implementation, sharing, and testing in the scientific community are available (Lin et al., 2017). Consequently, this has led to the wide acceptance of PBPK methods in the pharmaceutical industry (Rowland, 2013). However, this simplicity comes with a price, the most relevant is that standard PBPK methods are not suitable to model problems containing more than one independent variable, for example, systems that have a strong dependency on and significant variation in time, space, and potentially additional material dimensions. Nonetheless, efforts have been made to partially extend their range of applicability, inducing methods that use hybrid models that combine computational fluid dynamics (CFD) and PBPK modeling techniques (Andersen et al., 2000; Corley et al., 2009). To date, various imaging techniques have been successfully incorporated into the conception of these models, including SPECT, PET, fluorescence imaging, and MRI (Dogra, Butner, et al., 2019; Ng et al., 2020).
We recently demonstrated the application of image‐guided mathematical modeling to investigate the in vivo disposition of ultrasmall porous silica NPs in two mouse models of breast cancer (Figure (Figure5)5) (Goel et al., 2019). This was accomplished with a reduced PBPK model comprised of systemic circulation, liver, spleen, muscle, and tumor compartments. Literature‐derived blood and lymph flow rates were used to model the whole‐body transport of NPs, and quantified PET/CT imaging data was used to estimate empirical transport parameters related to the tumor compartment and phagocytic uptake rates of NPs in the liver and spleen. The study revealed that despite similar systemic circulation behaviors, the NPs demonstrated different tumor uptake profiles, which correlated with the degree of vascularization of the tumors, as confirmed through histopathological analysis (Goel et al., 2019).
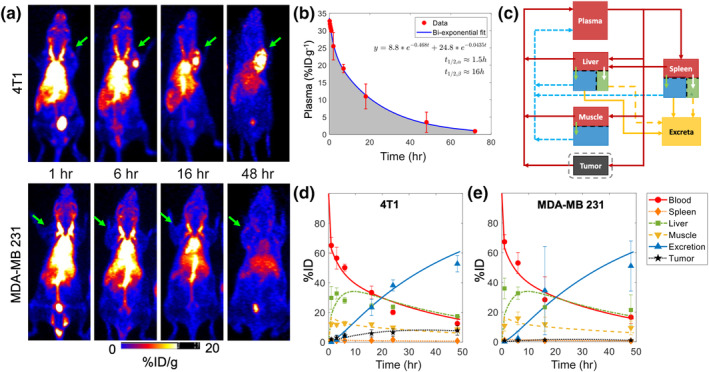
Noninvasive imaging‐guided mathematical modeling to evaluate the pharmacokinetic (PK) of ultrasmall porous silica nanoparticles (UPSNs). (a) Representative longitudinal positron emission tomography (PET)/computed tomography (CT) images of 4T1 (upper panel) and MDA‐MB 231 (lower panel) tumor‐bearing mice injected with 64Cu‐labeled ultrasmall porous silica nanoparticles (UPSNs). (b) Two‐compartment PK modeling of plasma concentration kinetics data of UPSNs obtained in healthy mice. (c) Schematic of reduced physiologically based pharmacokinetic (PBPK) model to investigate the disposition kinetic of UPSNs in tumor‐bearing mice. (d, e) PBPK‐model fits obtained through nonlinear regression of the data. (Reproduced with permission from Goel et al., 2019. Copyright 2019 Wiley)
Our group has also previously developed simplistic, semi‐mechanistic mathematical models based on quantified in vivo SPECT/CT imaging data to establish the relationship between physicochemical properties (size and surface chemistry) of mesoporous silica NPs (MSNs) and their in vivo pharmacokinetics (Figure (Figure6)6) (Dogra et al., 2018). The study revealed a monotonic decline in systemic bioavailability of NPs with systematic increase in particle size from ~32 to ~142nm, independent of the route of administration. This was accompanied by a simultaneous increase in NP accumulation in the liver and spleen. The study also highlighted the importance of surface chemistry in governing the biodistribution profile of MSNs, revealing that MSNs with surface exposed amines (polyethylene imine, PEI) had a much shorter circulation half‐life than size‐ and charge‐matched MSNs with shielded surface amines (quaternary amine, QA), due to rapid sequestration of the former in liver and spleen. Using the mathematical models, we were able to quantify important PK parameters and establish functional correlations between particle properties and biodistribution parameters (Dogra et al., 2018). Further, we also used the above data to develop a novel PBPK model to predict NP pharmacokinetics and tumor delivery in vivo. Through local and global parameter sensitivity analyses, we identified NP degradation rate, tumor blood viscosity, NP size, tumor vascular fraction, and tumor vascular porosity as the key parameters in governing NP kinetics in the tumor interstitium (Dogra et al., 2020).
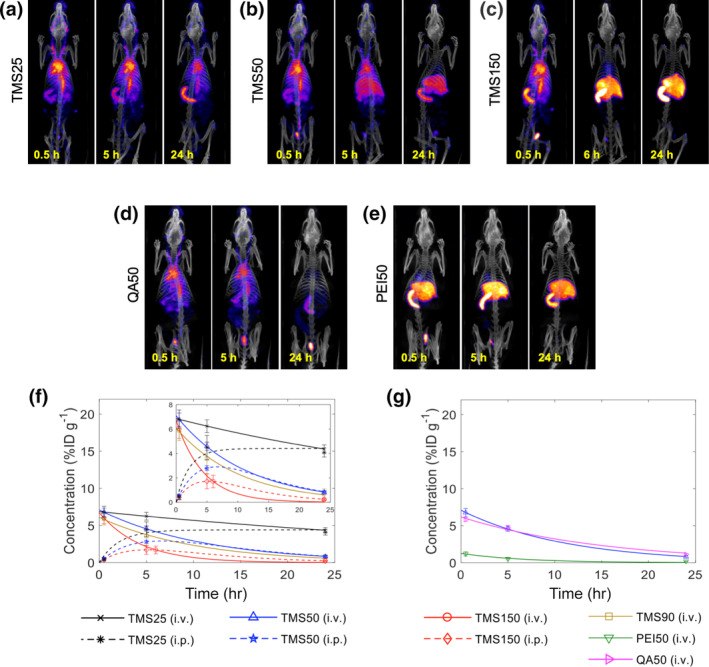
Representative single photon emission computed tomography/CT (SPECT/CT) images showing the whole‐body spatiotemporal evolution of trimethylsilane (TMS)‐, quaternary amine (QA)‐, and polyethylene imine (PEI)‐coated mesoporous silica NPs of variable sizes and surface charges (a) TMS25: 25nm, −5 mV, (b) TMS50: 50
nm, −7 mV, (c) TMS150: 150
nm, −4 mV, (d) QA50: 50
nm, +38
mV, and (e) PEI50: 50
nm, +37
mV, in vivo. (f, g) Model fits to the longitudinal systemic concentration kinetics data obtained from quantified SPECT/CT images of the heart region‐of‐interest. (Reproduced with permission from Dogra et al. (2018). Copyright 2018 Springer Nature)
Notable strides in the development of novel mechanistic mathematical models informed by imaging data have been achieved in recent years (T. A. Brocato et al., 2018, 2019; Cristini et al., 2017; Deisboeck, Wang, Macklin, & Cristini, 2011; Pascal, Bearer, et al., 2013; Z. Wang & Deisboeck, 2014; Zhihui Wang et al., 2016). To understand how NPs may be designed for optimal tumor capture and retention, T.‐R. Lee, Na, et al. (2013) examined how size affects bloodborne NP accumulation near the vasculature wall (a key factor in NP extravasation) with a mechanistic immersed finite element methods model, and compared model results against intravital video microscopy data in murine models. By representing NPs as Lagrangian solids in Eulerian fluid using a Navier–Stokes transport representation hybridized with discrete Mooney‐Rivilin material model representations of red blood cells (RBCs), their model provided valuable mechanistic understanding into why submicron sized NPs (500–1,000μm) achieve increased incidence with the vasculature wall via displacement by RBC movement, in good agreement with intravital microscopy observations. Their results suggest NP designs that may increase the likelihood of delivery to tumors through vasculature fenestration‐mediated extravasation, albeit at a cost of using larger NPs than may be likely to penetrate and deliver therapeutic cargo deep into tissues.
After NPs are retained in tumor vasculature, they must achieve cargo delivery, often through the enhanced permeability and retention (EPR) mechanisms, whereby “leakiness” in tumor vasculature due to defects in tumor vascular epithelial cells and epithelial fenestrations, as well as the lack of lymph drainage from tumor vasculature (among other mechanisms), tends to increase macromolecular accumulation in tumors relative to other tissues. van de Ven et al. (2012) implemented a mechanistic model of tumor kill due to NP extravasation and subsequent drug delivery, including mathematical descriptions of NP extravasation (the EPR effect), cellular uptake, drug release (NP decay rate), and subsequent drug diffusion into the tumor. Model parameters were quantified using experimental values from intravital microscopy imaging of in vivo NP distribution in human B16 melanoma murine model cells and from literature‐derived values, yielding valuable quantification of actual drug delivered into cancer cells via NP dosing (Figure (Figure7).7). Unfortunately, this result demonstrated that single administration of NPs was unable to deliver the IC50 for these tumor cells, suggesting that important further studies are needed to optimize dosing schedules and protocols for effective tumor kill. This model was later adapted and applied to an in vitro study of two‐ and three‐layer NP delivery of paclitaxel or cisplatin in human NSCLC monolayer/spheroid cultures by Curtis, England, Wu, Lowengrub, and Frieboes (2016), allowing the authors to quantitatively explain how longer half‐life and steady NP release rates of paclitaxel enabled increased tumor kill rates over NP delivered cisplatin; however it remains unclear how well these results may translate into in vivo models.

Intravital microscopic images of NP circulation in (a) healthy and (b) tumorous vessels. (c) Quantification of NP circulation and accumulation kinetics. (d) Computational modeling of therapeutic efficacy of NP‐delivered doxorubicin in a virtual tumor under different initial conditions. (Reproduced with permission from van de Ven et al. (2012). Copyright 2012 AIP Publishing)
Additional modeling insights into the EPR effect on liposomal NPs were provided by Stapleton et al. (2013) who developed a mechanistic intra‐tumoral transport model (ITTM), built on the biophysical transport of pressure‐driven fluid flow through tissues and across the vasculature endothelium, which was validated against CT imaging data from two xenograft mouse models and one syngeneic rabbit tumor model. This model was shown to accurately predict imaging‐derived experimental data (R
2 >0.9) for tumor NP accumulation due to EPR effects, however at the cost of neglecting the effects of variations in intratumoral interstitial fluid pressure, which is known to resist NP tumor penetration and lead to heterogeneous intratumoral NP delivery (Holback & Yeo, 2011). Hosoya et al. (2016) applied a mechanistic model of drug transport to theranostic doxorubicin‐containing heat sensitive‐based liposomes (HSL) embedded in targeted hydrogels, which were delivered in vivo into tumor‐bearing mice. Using imaging‐based tracking of NPs by replacing drug cargos with Gd‐DTPA and NIR dye to inform model parameters, these experiments revealed that HSL containing hydrogels showed a marked ~700% increase in tumor kill rates relative to traditional HSL delivery, confirming the predictions of their modeling work. While this model captures the pharmacodynamic effects of delivered drug, it only describes the effects of the NP delivered cargo and does not represent the NPs themselves, limiting its ability to quantitatively study many factors unique to NPs.
5. CONCLUSION
Advancements in in vivo imaging have enabled extensive pharmacological characterization of NPs and mAbs. Using state‐of‐the‐art imaging modalities, appropriately labeled macromolecules can be visualized over time throughout the body, which can allow the quantification of their disposition kinetics, facilitate the estimation of transport and physiological parameters, and help guide the development of mathematical models that can be employed for descriptive and predictive applications. Among the available macromolecule imaging modalities, we have chosen to focus only on those most commonly used in current practice, including MRI, SPECT, PET, and optical imaging. While MRI, PET, and SPECT are being used clinically, optical imaging has yet to be successfully transitioned to clinical use; nevertheless optical imaging is a widely used tool for preclinical development of macromolecules. Noninvasive whole‐body imaging not only provides a more comprehensive understanding of the disposition of macromolecules than traditional plasma concentration kinetics‐based pharmacological methods, but also sheds light on the underlying physiological changes in the diseased tissue, thereby opening avenues for the development of novel imaging‐based biomarkers for easy and early noninvasive disease diagnosis and improved prognosis. This also allows for the development and accurate parametrization of mathematical models based on correct biological and physical processes, in order to gain increased understanding of the key processes involved and increase understanding on how macromolecules may be designed to maximize their intended therapeutic effects.
Despite the remarkable potential of imaging‐based pharmacological assessment of macromolecules, there are caveats that must be considered during the quantification and interpretation of the imaging data obtained. It is essential to recognize what methodologies generate truly quantitative results (e.g. magnetic and nuclear imaging) that do not suffer from systematic errors (e.g. tissue attenuation for optical imaging). The stability of the bond between the imaging agent and the macromolecule it labels is critical for the accurate estimation of disposition kinetics and parameter values, and the breaking of this bond can result in leakage of the label from the macromolecule, confounding observations and providing inaccurate pharmacological assessments. The disposition behavior of macromolecules at early time points following administration is critical to accurately assess the pharmacokinetics of macromolecules, hence imaging modalities with low temporal resolution should be avoided in such circumstances, and are appropriate when only a semi‐quantitative assessment is required. Similarly, limitations of spatial resolution should be considered based on the nature of the assessment.
The integration of in vivo imaging with mathematical modeling is a valuable tool that, in addition to the pharmacological evaluation of macromolecules, can provide insights into mechanisms of transport barrier‐induced drug resistance or therapy failure, which can support the development of novel strategies to improve delivery and personalize treatment. Selection of which biological and physical phenomena should be included in such mathematical models, and their quantification, is improved when these are informed based on imaging‐based parameter estimates (rather than best fit estimates), which can support the development of mechanistic models for highly accurate predictive applications. Such models will not only provide greater insights into the complex interplay between pathophysiological states and the underlying transport mechanics, but will also serve as in‐silico tools to guide the design optimization of macromolecules for improved delivery and therapeutic efficacy. Further, the application of PBPK‐like modeling frameworks can provide reliable projections for human applications based on models developed for lower species (Aborig et al., 2019; Shah & Betts, 2012; Zhao, Cao, & Jusko, 2015). Taken together, these exciting advances are expected to result in an increased application of imaging‐based data to guide the development of mathematical models for quantitative investigation of macromolecules.
AUTHOR CONTRIBUTIONS
Prashant Dogra: Conceptualization; investigation; writing‐original draft; writing‐review and editing. Joseph Butner: Investigation; writing‐original draft; writing‐review and editing. Sara Nizzero: Writing‐original draft; writing‐review and editing. Javier Ruiz Ramírez: Writing‐original draft; writing‐review and editing. Achraf Noureddine: Writing‐original draft; writing‐review and editing. María Peláez: Writing‐original draft. Dalia Elganainy: Writing‐original draft. Zhen Yang: Writing‐original draft. Anh‐Dung Le: Writing‐original draft. Shreya Goel: Writing‐review and editing. Hon Sing Leong: Writing‐review and editing. Eugene Koay: Writing‐review and editing. C. Jeffrey Brinker: Writing‐review and editing. Vittorio Cristini: Writing‐review and editing. Zhihui Wang: Conceptualization; investigation; supervision; writing‐original draft; writing‐review and editing.
RELATED WIREs ARTICLE
Engineering of radiolabeled iron oxide nanoparticles for dual‐modality imaging
ACKNOWLEDGMENTS
This research has been supported in part by the National Science Foundation Grant DMS‐1930583 (V. C., Z. W.), the National Institutes of Health (NIH) Grants 1U01CA196403 (V. C., Z. W.), 1U01CA213759 (V. C., Z. W.), 1R01CA226537 (C. J. B., V. C., Z. W.), 1R01CA222007 (V. C., Z. W.), and U54CA210181 (V. C., Z. W.).
Notes
Dogra P, Butner JD, Nizzero S, et al. Image‐guided mathematical modeling for pharmacological evaluation of nanomaterials and monoclonal antibodies. WIREs Nanomed Nanobiotechnol. 2020;12:e1628 10.1002/wnan.1628 [Europe PMC free article] [Abstract] [CrossRef] [Google Scholar]
Funding information National Science Foundation, Grant/Award Number: 1930583; National Institutes of Health, Grant/Award Numbers: 1R01CA222007, 1R01CA226537, 1U01CA196403, 1U01CA213759, U54CA210181
Contributor Information
Vittorio Cristini, Email: gro.tsidohtemnotsuoh@initsircv.
Zhihui Wang, Email: gro.tsidohtemnotsuoh@gnawz.
REFERENCES
- Abdelwahab M. G., Sankar T., Preul M. C., & Scheck A. C. (2011). Intracranial implantation with subsequent 3D in vivo bioluminescent imaging of murine gliomas. Journal of Visualized Experiments, 57, e3403. [Europe PMC free article] [Abstract] [Google Scholar]
- Aborig M., Malik P. R. V., Nambiar S., Chelle P., Darko J., Mutsaers A., … Wettig S. (2019). Biodistribution and physiologically‐based pharmacokinetic modeling of gold nanoparticles in mice with interspecies extrapolation. Pharmaceutics, 11(4), 1–17. 10.3390/pharmaceutics11040179 [Europe PMC free article] [Abstract] [CrossRef] [Google Scholar]
- Abou D. S., Thorek D. L., Ramos N. N., Pinkse M. W., Wolterbeek H. T., Carlin S. D., … Lewis J. S. (2013). (89)Zr‐labeled paramagnetic octreotide‐liposomes for PET‐MR imaging of cancer. Pharmaceutical Research, 30(3), 878–888. 10.1007/s11095-012-0929-8 [Europe PMC free article] [Abstract] [CrossRef] [Google Scholar]
- Abuqayyas L., & Balthasar J. P. (2012). Application of PBPK modeling to predict monoclonal antibody disposition in plasma and tissues in mouse models of human colorectal cancer. Journal of Pharmacokinetics and Pharmacodynamics, 39(6), 683–710. 10.1007/s10928-012-9279-8 [Europe PMC free article] [Abstract] [CrossRef] [Google Scholar]
- Ahmed M., Cerroni B., Razuvaev A., Härmark J., Paradossi G., Caidahl K., & Gustafsson B. (2017). Cellular uptake of plain and SPION‐modified microbubbles for potential use in molecular imaging. Cellular and Molecular Bioengineering, 10(6), 537–548. [Europe PMC free article] [Abstract] [Google Scholar]
- Alsaid H., Skedzielewski T., Rambo M. V., Hunsinger K., Hoang B., Fieles W., … Jucker B. M. (2017). Non invasive imaging assessment of the biodistribution of GSK2849330, an ADCC and CDC optimized anti HER3 mAb, and its role in tumor macrophage recruitment in human tumor‐bearing mice. PLoS One, 12(4), e0176075 10.1371/journal.pone.0176075 [Europe PMC free article] [Abstract] [CrossRef] [Google Scholar]
- Andersen M., Sarangapani R., Gentry R., Clewell H., Covington T., & Frederick C. B. (2000). Application of a hybrid CFD‐PBPK nasal dosimetry model in an inhalation risk assessment: An example with acrylic acid. Toxicological Sciences, 57(2), 312–325. 10.1093/toxsci/57.2.312 [Abstract] [CrossRef] [Google Scholar]
- Arms L., Smith D. W., Flynn J., Palmer W., Martin A., Woldu A., & Hua S. (2018). Advantages and limitations of current techniques for analyzing the biodistribution of nanoparticles. Frontiers in Pharmacology, 9, 802 10.3389/fphar.2018.00802 [Europe PMC free article] [Abstract] [CrossRef] [Google Scholar]
- Au L., Zhang Q., Cobley C. M., Gidding M., Schwartz A. G., Chen J., & Xia Y. (2009). Quantifying the cellular uptake of antibody‐conjugated Au nanocages by two‐photon microscopy and inductively coupled plasma mass spectrometry. ACS Nano, 4(1), 35–42. [Europe PMC free article] [Abstract] [Google Scholar]
- Bandula S., Banypersad S. M., Sado D., Flett A. S., Punwani S., Taylor S. A., … Moon J. C. (2013). Measurement of tissue interstitial volume in healthy patients and those with amyloidosis with equilibrium contrast‐enhanced MR imaging. Radiology, 268(3), 858–864. [Europe PMC free article] [Abstract] [Google Scholar]
- Banerjee S. R., Foss C. A., Horhota A., Pullambhatla M., McDonnell K., Zale S., & Pomper M. G. (2017). (111)In‐ and IRDye800CW‐labeled PLA–PEG nanoparticle for imaging prostate‐specific membrane antigen‐expressing tissues. Biomacromolecules, 18(1), 201–209. 10.1021/acs.biomac.6b01485 [Europe PMC free article] [Abstract] [CrossRef] [Google Scholar]
- Belleudi F., Marra E., Mazzetta F., Fattore L., Giovagnoli M. R., Mancini R., … Ciliberto G. (2012). Monoclonal antibody‐induced ErbB3 receptor internalization and degradation inhibits growth and migration of human melanoma cells. Cell Cycle, 11(7), 1455–1467. [Abstract] [Google Scholar]
- Bernhard W., El‐Sayed A., Barreto K., Gonzalez C., Hill W., Parada A. C., … Geyer C. R. (2018). Near infrared fluorescence imaging of EGFR expression in vivo using IRDye800CW‐nimotuzumab. Oncotarget, 9(5), 6213–6227. 10.18632/oncotarget.23557 [Europe PMC free article] [Abstract] [CrossRef] [Google Scholar]
- Blancato J. N. (1994). Pharmacokinetics, chemical interactions, and toxicological risk assessment in perspective. Environmental Health Perspectives, 102(suppl 9), 133–137. 10.1289/ehp.94102s9133 [Europe PMC free article] [Abstract] [CrossRef] [Google Scholar]
- Blatter C., Meijer E. F., Nam A. S., Jones D., Bouma B. E., Padera T. P., & Vakoc B. J. (2016). In vivo label‐free measurement of lymph flow velocity and volumetric flow rates using Doppler optical coherence tomography. Scientific Reports, 6, 29035. [Europe PMC free article] [Abstract] [Google Scholar]
- Börjesson P. K. E., Jauw Y. W. S., de Bree R., Roos J. C., Castelijns J. A., Leemans C. R., … Boellaard R. (2009). Radiation dosimetry of 89Zr‐labeled chimeric monoclonal antibody U36 as used for immuno‐PET in head and neck cancer patients. Journal of Nuclear Medicine, 50(11), 1828–1836. [Abstract] [Google Scholar]
- Borogovac A., & Asllani I. (2012). Arterial spin labeling (ASL) fMRI: Advantages, theoretical constrains and experimental challenges in neurosciences. International Journal of Biomedical Imaging, 2012, 1–13. [Europe PMC free article] [Abstract] [Google Scholar]
- Boucher Y., & Jain R. K. (1992). Microvascular pressure is the principal driving force for interstitial hypertension in solid tumors: Implications for vascular collapse. Cancer Research, 52(18), 5110–5114. [Abstract] [Google Scholar]
- Brocato T., Dogra P., Koay E. J., Day A., Chuang Y. L., Wang Z., & Cristini V. (2014). Understanding drug resistance in breast cancer with mathematical oncology. Current Breast Cancer Report, 6(2), 110–120. 10.1007/s12609-014-0143-2 [Europe PMC free article] [Abstract] [CrossRef] [Google Scholar]
- Brocato T. A., Brown‐Glaberman U., Wang Z., Selwyn R. G., Wilson C. M., Wyckoff E. F., … Cristini V. (2019). Predicting breast cancer response to neoadjuvant chemotherapy based on tumor vascular features in needle biopsies. JCI Insight, 4(8), e126518 10.1172/jci.insight.126518 [Europe PMC free article] [Abstract] [CrossRef] [Google Scholar]
- Brocato T. A., Coker E. N., Durfee P. N., Lin Y. S., Townson J., Wyckoff E. F., … Wang Z. (2018). Understanding the connection between nanoparticle uptake and cancer treatment efficacy using mathematical modeling. [Research]. Scientific Reports, 8(1), 7538 10.1038/s41598-018-25878-8 [Europe PMC free article] [Abstract] [CrossRef] [Google Scholar]
- Brooks R. J., & Tobias A. M. (1996). Choosing the best model: Level of detail, complexity, and model performance. Mathematical and Computer Modelling, 24(4), 1–14. 10.1016/0895-7177(96)00103-3 [CrossRef] [Google Scholar]
- Bushberg J. T., & Boone J. M. (2011). The essential physics of medical imaging. Philadelphia, PA: Lippincott Williams & Wilkins. [Google Scholar]
- Cao J., Wei Y., Zhang Y., Wang G., Ji X., & Zhong Z. (2019). Iodine‐rich polymersomes enable versatile SPECT/CT imaging and potent radioisotope therapy for tumor in vivo. ACS Applied Materials & Interfaces, 11(21), 18953–18959. 10.1021/acsami.9b04294 [Abstract] [CrossRef] [Google Scholar]
- Cao Y., Balthasar J. P., & Jusko W. J. (2013). Second‐generation minimal physiologically‐based pharmacokinetic model for monoclonal antibodies. Journal of Pharmacokinetics and Pharmacodynamics, 40(5), 597–607. 10.1007/s10928-013-9332-2 [Europe PMC free article] [Abstract] [CrossRef] [Google Scholar]
- Cha S., Yang L., Johnson G., Lai A., Chen M.‐H., Tihan T., … Dillon W. (2006). Comparison of microvascular permeability measurements, Ktrans, determined with conventional steady‐state T1‐weighted and first‐pass T2*‐weighted MR imaging methods in gliomas and meningiomas. American Journal of Neuroradiology, 27(2), 409–417. [Abstract] [Google Scholar]
- Chatterjee S., Lesniak W. G., & Nimmagadda S. (2017). Noninvasive imaging of immune checkpoint ligand PD‐L1 in tumors and metastases for guiding immunotherapy. Molecular Imaging, 16, 1536012117718459 10.1177/1536012117718459 [Europe PMC free article] [Abstract] [CrossRef] [Google Scholar]
- Chen F., Goel S., Hernandez R., Graves S. A., Shi S., Nickles R. J., & Cai W. (2016). Dynamic positron emission tomography imaging of renal clearable gold nanoparticles. Small, 12(20), 2775–2782. [Europe PMC free article] [Abstract] [Google Scholar]
- Chen F., Goel S., Valdovinos H. F., Luo H., Hernandez R., Barnhart T. E., & Cai W. (2015). In vivo integrity and biological fate of chelator‐free zirconium‐89‐labeled mesoporous silica nanoparticles. ACS Nano, 9(8), 7950–7959. 10.1021/acsnano.5b00526 [Europe PMC free article] [Abstract] [CrossRef] [Google Scholar]
- Chen F., Hong H., Zhang Y., Valdovinos H. F., Shi S., Kwon G. S., … Cai W. (2013). In vivo tumor targeting and image‐guided drug delivery with antibody‐conjugated, radiolabeled mesoporous silica nanoparticles. ACS Nano, 7(10), 9027–9039. 10.1021/nn403617j [Europe PMC free article] [Abstract] [CrossRef] [Google Scholar]
- Chen Z., Milner T. E., Srinivas S., Wang X., Malekafzali A., van Gemert M. J., & Nelson J. S. (1997). Noninvasive imaging of in vivo blood flow velocity using optical Doppler tomography. Optics Letters, 22(14), 1119–1121. [Abstract] [Google Scholar]
- Chrastina A., & Schnitzer J. E. (2010). Iodine‐125 radiolabeling of silver nanoparticles for in vivo SPECT imaging. International Journal of Nanomedicine, 5, 653–659. 10.2147/IJN.S11677 [Europe PMC free article] [Abstract] [CrossRef] [Google Scholar]
- Cilliers C., Nessler I., Christodolu N., & Thurber G. M. (2017). Tracking antibody distribution with near‐infrared fluorescent dyes: Impact of dye structure and degree of labeling on plasma clearance. Molecular Pharmaceutics, 14(5), 1623–1633. 10.1021/acs.molpharmaceut.6b01091 [Europe PMC free article] [Abstract] [CrossRef] [Google Scholar]
- Clancy C. E., An G., Cannon W. R., Liu Y., May E. E., Ortoleva P., … Eckmann D. M. (2016). Multiscale modeling in the clinic: Drug design and development. Annals of Biomedical Engineering, 44(9), 2591–2610. 10.1007/s10439-016-1563-0 [Europe PMC free article] [Abstract] [CrossRef] [Google Scholar]
- Cole E. L., Kim J., Donnelly D. J., Smith R. A., Cohen D., Lafont V., … Hayes W. (2017). Radiosynthesis and preclinical PET evaluation of 89Zr‐nivolumab (BMS‐936558) in healthy non‐human primates. Bioorganic & Medicinal Chemistry, 25(20), 5407–5414. [Abstract] [Google Scholar]
- Coll J. L. (2011). Cancer optical imaging using fluorescent nanoparticles. Nanomedicine (London, England), 6(1), 7–10. 10.2217/nnm.10.144 [Europe PMC free article] [Abstract] [CrossRef] [Google Scholar]
- Corley R. A., Minard K. R., Kabilan S., Einstein D. R., Kuprat A. P., Harkema J. R., … Kinzell J. H. (2009). Magnetic resonance imaging and computational fluid dynamics (CFD) simulations of rabbit nasal airflows for the development of hybrid CFD/PBPK models. Inhalation Toxicology, 21(6), 512–518. 10.1080/08958370802598005 [Europe PMC free article] [Abstract] [CrossRef] [Google Scholar]
- Cristini V., Koay E., & Wang Z. (2017). An introduction to physical oncology: How mechanistic mathematical modeling can improve cancer therapy outcomes. Boca Raton, FL: CRC Press. [Google Scholar]
- Curtis L. T., England C. G., Wu M., Lowengrub J., & Frieboes H. B. (2016). An interdisciplinary computational/experimental approach to evaluate drug‐loaded gold nanoparticle tumor cytotoxicity. Nanomedicine (London, England), 11(3), 197–216. 10.2217/nnm.15.195 [Europe PMC free article] [Abstract] [CrossRef] [Google Scholar]
- Davis R. A., Rippner D. A., Hausner S. H., Parikh S. J., McElrone A. J., & Sutcliffe J. L. (2017). In vivo tracking of copper‐64 radiolabeled nanoparticles in Lactuca sativa . Environmental Science & Technology, 51(21), 12537–12546. 10.1021/acs.est.7b03333 [Abstract] [CrossRef] [Google Scholar]
- Dearling J. L. J., Paterson B. M., Akurathi V., Betanzos‐Lara S., Treves S. T., Voss S. D., … Packard A. B. (2015). The ionic charge of copper‐64 complexes conjugated to an engineered antibody affects biodistribution. Bioconjugate Chemistry, 26(4), 707–717. 10.1021/acs.bioconjchem.5b00049 [Abstract] [CrossRef] [Google Scholar]
- Deisboeck T. S., Wang Z., Macklin P., & Cristini V. (2011). Multiscale cancer modeling. Annual Review of Biomedical Engineering, 13, 127–155. 10.1146/annurev-bioeng-071910-124729 [Europe PMC free article] [Abstract] [CrossRef] [Google Scholar]
- Deri M. A., Ponnala S., Zeglis B. M., Pohl G., Dannenberg J. J., Lewis J. S., & Francesconi L. C. (2014). Alternative Chelator for 89Zr radiopharmaceuticals: Radiolabeling and evaluation of 3,4,3‐(LI‐1,2‐HOPO). Journal of Medicinal Chemistry, 57(11), 4849–4860. 10.1021/jm500389b [Europe PMC free article] [Abstract] [CrossRef] [Google Scholar]
- Deri M. A., Zeglis B. M., Francesconi L. C., & Lewis J. S. (2013). PET imaging with 89Zr: From radiochemistry to the clinic. Nuclear Medicine and Biology, 40(1), 3–14. 10.1016/j.nucmedbio.2012.08.004 [Europe PMC free article] [Abstract] [CrossRef] [Google Scholar]
- Detappe A., Thomas E., Tibbitt M. W., Kunjachan S., Zavidij O., Parnandi N., … Berbeco R. (2017). Ultrasmall silica‐based bismuth gadolinium nanoparticles for dual magnetic resonance‐computed tomography image guided radiation therapy. Nano Letters, 17(3), 1733–1740. 10.1021/acs.nanolett.6b05055 [Europe PMC free article] [Abstract] [CrossRef] [Google Scholar]
- Devaraj N. K., Keliher E. J., Thurber G. M., Nahrendorf M., & Weissleder R. (2009). 18F labeled nanoparticles for in vivo PET‐CT imaging. Bioconjugate Chemistry, 20(2), 397–401. 10.1021/bc8004649 [Europe PMC free article] [Abstract] [CrossRef] [Google Scholar]
- Dijkers E. C., Oude Munnink T. H., Kosterink J. G., Brouwers A. H., Jager P. L., de Jong J. R., … de Vries E. G. (2010). Biodistribution of 89Zr‐trastuzumab and PET imaging of HER2‐positive lesions in patients with metastatic breast Cancer. Clinical Pharmacology & Therapeutics, 87(5), 586–592. 10.1038/clpt.2010.12 [Abstract] [CrossRef] [Google Scholar]
- Ding H., & Wu F. (2012). Image guided biodistribution and pharmacokinetic studies of theranostics. Theranostics, 2(11), 1040. [Europe PMC free article] [Abstract] [Google Scholar]
- Dogra P., Adolphi N. L., Wang Z., Lin Y.‐S., Butler K. S., Durfee P. N., … Brinker C. J. (2018). Establishing the effects of mesoporous silica nanoparticle properties on in vivo disposition using imaging‐based pharmacokinetics. Nature Communications, 9(1), 4551 10.1038/s41467-018-06730-z [Europe PMC free article] [Abstract] [CrossRef] [Google Scholar]
- Dogra P., Butner J. D., Chuang, Y.l., Caserta S., Goel S., Brinker C. J., … Wang Z. (2019). Mathematical modeling in cancer nanomedicine: A review. [journal article]. Biomedical Microdevices, 21(2), 40. 10.1007/s10544-019-0380-2 [Europe PMC free article] [Abstract] [CrossRef]
- Dogra P., Butner JD, Ramírez JR., Y‐l Chuang., Noureddine A., Brinker CJ., … Wang Z. (2020). A mathematical model to predict nanomedicine pharmacokinetics and tumor delivery. Computational and Structural Biotechnology Journal, 18, 518–531. 10.1016/j.csbj.2020.02.014 [Europe PMC free article] [Abstract] [CrossRef] [Google Scholar]
- Dogra P., Ramirez J. R., Pelaez M. J., Wang Z., Cristini V., Parasher G., & Rawat M. (2019). Mathematical Modeling to address challenges in pancreatic Cancer. Current Topics in Medicinal Chemistry, 20(5), 367–376. 10.2174/1568026620666200101095641 [Europe PMC free article] [Abstract] [CrossRef] [Google Scholar]
- Dreher M. R., Liu W., Michelich C. R., Dewhirst M. W., Yuan F., & Chilkoti A. (2006). Tumor vascular permeability, accumulation, and penetration of macromolecular drug carriers. Journal of the National Cancer Institute, 98(5), 335–344. [Abstract] [Google Scholar]
- Du Y., Jin Y., Sun W., Fang J., Zheng J., & Tian J. (2019). Advances in molecular imaging of immune checkpoint targets in malignancies: Current and future prospect. European Radiology, 29(8), 4294–4302. 10.1007/s00330-018-5814-3 [Europe PMC free article] [Abstract] [CrossRef] [Google Scholar]
- Dumoga S., Rai Y., Bhatt A. N., Tiwari A. K., Singh S., Mishra A. K., & Kakkar D. (2017). Block copolymer based nanoparticles for Theranostic intervention of cervical Cancer: Synthesis, pharmacokinetics, and in vitro/in vivo evaluation in HeLa xenograft models. ACS Applied Materials & Interfaces, 9(27), 22195–22211. 10.1021/acsami.7b04982 [Abstract] [CrossRef] [Google Scholar]
- Farzin L., Sheibani S., Moassesi M. E., & Shamsipur M. (2019). An overview of nanoscale radionuclides and radiolabeled nanomaterials commonly used for nuclear molecular imaging and therapeutic functions. Journal of Biomedical Materials Research Part A, 107(1), 251–285. 10.1002/jbm.a.36550 [Abstract] [CrossRef] [Google Scholar]
- Frellsen A. F., Hansen A. E., Jølck R. I., Kempen P. J., Severin G. W., Rasmussen P. H., … Andresen T. L. (2016). Mouse positron emission tomography study of the biodistribution of gold nanoparticles with different surface coatings using embedded Copper‐64. ACS Nano, 10(11), 9887–9898. 10.1021/acsnano.6b03144 [Abstract] [CrossRef] [Google Scholar]
- Gabrielsson J., & Weiner D. (2001). Pharmacokinetic and pharmacodynamic data analysis: Concepts and applications (Vol. 2). Boca Raton, FL: CRC Press. [Google Scholar]
- Gangadaran P., Li X. J., Lee H. W., Oh J. M., Kalimuthu S., Rajendran R. L., … Ahn B. C. (2017). A new bioluminescent reporter system to study the biodistribution of systematically injected tumor‐derived bioluminescent extracellular vesicles in mice. Oncotarget, 8(66), 109894–109914. 10.18632/oncotarget.22493 [Europe PMC free article] [Abstract] [CrossRef] [Google Scholar]
- Godin S. J., DeVito M. J., Hughes M. F., Ross D. G., Scollon E. J., Starr J. M., … Tornero‐Velez R. (2010). Physiologically based pharmacokinetic Modeling of Deltamethrin: Development of a rat and human diffusion‐limited model. Toxicological Sciences, 115(2), 330–343. 10.1093/toxsci/kfq051 [Abstract] [CrossRef] [Google Scholar]
- Goel S., Chen F., Ehlerding E. B., & Cai W. (2014). Intrinsically radiolabeled nanoparticles: An emerging paradigm. Small (Weinheim an der Bergstrasse, Germany), 10(19), 3825–3830. 10.1002/smll.201401048 [Europe PMC free article] [Abstract] [CrossRef] [Google Scholar]
- Goel S., Ferreira C. A., Dogra P., Yu B., Kutyreff C. J., Siamof C. M., … Cai W. (2019). Size‐optimized Ultrasmall porous silica nanoparticles depict vasculature‐based differential targeting in triple negative breast cancer. Small, 15(46), e1903747 10.1002/smll.201903747 [Europe PMC free article] [Abstract] [CrossRef] [Google Scholar]
- Guo W., Sun X., Jacobson O., Yan X., Min K., Srivatsan A., … Chen X. (2015). Intrinsically radioactive [64Cu]CuInS/ZnS quantum dots for PET and optical imaging: Improved radiochemical stability and controllable Cerenkov luminescence. ACS Nano, 9(1), 488–495. 10.1021/nn505660r [Europe PMC free article] [Abstract] [CrossRef] [Google Scholar]
- Haddish‐Berhane N., Rickus J. L., & Haghighi K. (2007). The role of multiscale computational approaches for rational design of conventional and nanoparticle oral drug delivery systems. International Journal of Nanomedicine, 2(3), 315–331. [Europe PMC free article] [Abstract] [Google Scholar]
- Helbok A., Decristoforo C., Dobrozemsky G., Rangger C., Diederen E., Stark B., … von Guggenberg E. (2010). Radiolabeling of lipid‐based nanoparticles for diagnostics and therapeutic applications: A comparison using different radiometals. Journal of Liposome Research, 20(3), 219–227. 10.3109/08982100903311812 [Abstract] [CrossRef] [Google Scholar]
- Henderson E., Sykes J., Drost D., Weinmann H. J., Rutt B. K., & Lee T. Y. (2000). Simultaneous MRI measurement of blood flow, blood volume, and capillary permeability in mammary tumors using two different contrast agents. Journal of Magnetic Resonance Imaging, 12(6), 991–1003. [Abstract] [Google Scholar]
- Heskamp S., Raave R., Boerman O., Rijpkema M., Goncalves V., & Denat F. (2017). (89)Zr‐Immuno‐positron emission tomography in oncology: State‐of‐the‐art (89)Zr radiochemistry. Bioconjugate Chemistry, 28(9), 2211–2223. 10.1021/acs.bioconjchem.7b00325 [Europe PMC free article] [Abstract] [CrossRef] [Google Scholar]
- Hindel S., Söhner A., Maaß M., Sauerwein W., Möllmann D., Baba H. A., … Lüdemann L. (2017). Validation of blood volume fraction quantification with 3D gradient echo dynamic contrast‐enhanced magnetic resonance imaging in porcine skeletal muscle. PLoS One, 12(1), e0170841. [Europe PMC free article] [Abstract] [Google Scholar]
- Holback H., & Yeo Y. (2011). Intratumoral drug delivery with nanoparticulate carriers. Pharmaceutical Research, 28(8), 1819–1830. 10.1007/s11095-010-0360-y [Europe PMC free article] [Abstract] [CrossRef] [Google Scholar]
- Hope M. D., Hope T. A., Zhu C., Faraji F., Haraldsson H., Ordovas K. G., & Saloner D. (2015). Vascular imaging with ferumoxytol as a contrast agent. American Journal of Roentgenology, 205(3), W366–W373. 10.2214/AJR.15.14534 [Europe PMC free article] [Abstract] [CrossRef] [Google Scholar]
- Hosoya H., Dobroff A. S., Driessen W. H. P., Cristini V., Brinker L. M., Staquicini F. I., … Pasqualini R. (2016). Integrated nanotechnology platform for tumor‐targeted multimodal imaging and therapeutic cargo release. Proceedings of the National Academy of Sciences of the United States of America, 113(7), 1877–1882. [Europe PMC free article] [Abstract] [Google Scholar]
- Jain R., Ellika S., Scarpace L., Schultz L., Rock J., Gutierrez J., … Mikkelsen T. (2008). Quantitative estimation of permeability surface‐area product in astroglial brain tumors using perfusion CT and correlation with histopathologic grade. American Journal of Neuroradiology, 29(4), 694–700. [Europe PMC free article] [Abstract] [Google Scholar]
- Jain R. K., & Stylianopoulos T. (2010). Delivering nanomedicine to solid tumors. Nature Reviews Clinical Oncology, 7(11), 653–664. [Europe PMC free article] [Abstract] [Google Scholar]
- Jain R. K., & Stylianopoulos T. (2010). Delivering nanomedicine to solid tumors. Nat Rev Clin Oncol, 7(11), 653–664. 10.1038/nrclinonc.2010.139 [Europe PMC free article] [Abstract] [Google Scholar]
- Jones S. K., Douglas K., Shields A. F., & Merkel O. M. (2018). Correlating quantitative tumor accumulation and gene knockdown using SPECT/CT and bioluminescence imaging within an orthotopic ovarian cancer model. Biomaterials, 178, 183–192. 10.1016/j.biomaterials.2018.06.014 [Europe PMC free article] [Abstract] [CrossRef] [Google Scholar]
- Josefsson A., Nedrow J. R., Park S., Banerjee S. R., Rittenbach A., Jammes F., … Sgouros G. (2016). Imaging, biodistribution, and dosimetry of radionuclide‐labeled PD‐L1 antibody in an immunocompetent mouse model of breast cancer. Cancer Research, 76(2), 472 10.1158/0008-5472.CAN-15-2141 [Europe PMC free article] [Abstract] [CrossRef] [Google Scholar]
- Kaminski M. S., Zasadny K. R., Francis I. R., Fenner M. C., Ross C. W., Milik A. W., … Fisher S. (1996). Iodine‐131‐anti‐B1 radioimmunotherapy for B‐cell lymphoma. Journal of Clinical Oncology, 14(7), 1974–1981. [Abstract] [Google Scholar]
- Keinanen O., Makila E. M., Lindgren R., Virtanen H., Liljenback H., Oikonen V., … Airaksinen A. J. (2017). Pretargeted PET imaging of trans‐cyclooctene‐modified porous silicon nanoparticles. ACS Omega, 2(1), 62–69. 10.1021/acsomega.6b00269 [Europe PMC free article] [Abstract] [CrossRef] [Google Scholar]
- Kim Y., Savellano M., Savellano D., Weissleder R., & Bogdanov A. Jr. (2004). Measurement of tumor interstitial volume fraction: Method and implication for drug delivery. Magnetic Resonance in Medicine: An Official Journal of the International Society for Magnetic Resonance in Medicine, 52(3), 485–494. [Abstract] [Google Scholar]
- Kobayashi K., Sasaki T., Takenaka F., Yakushiji H., Fujii Y., Kishi Y., … Matsuura E. (2015). A novel PET imaging using (6)(4)Cu‐labeled monoclonal antibody against mesothelin commonly expressed on cancer cells. Journal of Immunology Research, 2015, 268172 10.1155/2015/268172 [Europe PMC free article] [Abstract] [CrossRef] [Google Scholar]
- Kuepfer L., Niederalt C., Wendl T., Schlender J., Willmann S., Lippert J., … Teutonico D. (2016). Applied concepts in PBPK modeling: How to build a PBPK/PD model. CPT: Pharmacometrics & Systems Pharmacology, 5(10), 516–531. 10.1002/psp4.12134 [Europe PMC free article] [Abstract] [CrossRef] [Google Scholar]
- Kumar R., Roy I., Ohulchanskky T. Y., Vathy L. A., Bergey E. J., Sajjad M., & Prasad P. N. (2010). In vivo biodistribution and clearance studies using multimodal organically modified silica nanoparticles. ACS Nano, 4(2), 699–708. 10.1021/nn901146y [Europe PMC free article] [Abstract] [CrossRef] [Google Scholar]
- Lee D. E., Na J. H., Lee S., Kang C. M., Kim H. N., Han S. J., … Kim K. (2013). Facile method to radiolabel glycol chitosan nanoparticles with (64)Cu via copper‐free click chemistry for microPET imaging. Molecular Pharmaceutics, 10(6), 2190–2198. 10.1021/mp300601r [Abstract] [CrossRef] [Google Scholar]
- Lee J. J., White A. G., Rice D. R., & Smith B. D. (2013). In vivo imaging using polymeric nanoparticles stained with near‐infrared chemiluminescent and fluorescent squaraine catenane endoperoxide. Chemical Communications (Cambridge, England), 49(29), 3016–3018. 10.1039/c3cc40630j [Europe PMC free article] [Abstract] [CrossRef] [Google Scholar]
- Lee T.‐R., Choi M., Kopacz A. M., Yun S.‐H., Liu W. K., & Decuzzi P. (2013). On the near‐wall accumulation of injectable particles in the microcirculation: Smaller is not better. Scientific Reports, 3, 2079 10.1038/srep02079 [Europe PMC free article] [Abstract] [CrossRef] [Google Scholar]
- Li M., Al‐Jamal K. T., Kostarelos K., & Reineke J. (2010). Physiologically based pharmacokinetic modeling of nanoparticles. ACS Nano, 4(11), 6303–6317. [Abstract] [Google Scholar]
- Liao‐Chan S., Daine‐Matsuoka B., Heald N., Wong T., Lin T., Cai A. G., … Theunissen J.‐W. (2015). Quantitative assessment of antibody internalization with novel monoclonal antibodies against Alexa fluorophores. PLoS One, 10(4), e0124708. [Europe PMC free article] [Abstract] [Google Scholar]
- Lin Z., Jaberi‐Douraki M., He C., Jin S., Yang R. S. H., Fisher J. W., & Riviere J. E. (2017). Performance assessment and translation of physiologically based pharmacokinetic models from acslX to Berkeley Madonna, MATLAB, and R language: Oxytetracycline and gold nanoparticles as case examples. Toxicological Sciences, 158(1), 23–35. 10.1093/toxsci/kfx070 [Abstract] [CrossRef] [Google Scholar]
- Lindenberg L., Thomas A., Adler S., Mena E., Kurdziel K., Maltzman J., … Hassan R. (2015). Safety and biodistribution of 111In‐amatuximab in patients with mesothelin expressing cancers using single photon emission computed tomography–computed tomography (SPECT‐CT) imaging. Oncotarget, 6(6), 4496–4504. 10.18632/oncotarget.2883 [Europe PMC free article] [Abstract] [CrossRef] [Google Scholar]
- Liu L. (2018). Pharmacokinetics of monoclonal antibodies and Fc‐fusion proteins. Protein & Cell, 9(1), 15–32. [Europe PMC free article] [Abstract] [Google Scholar]
- Liu Y., Tseng Y. C., & Huang L. (2012). Biodistribution studies of nanoparticles using fluorescence imaging: A qualitative or quantitative method? Pharmaceutical Research, 29(12), 3273–3277. 10.1007/s11095-012-0818-1 [Europe PMC free article] [Abstract] [CrossRef] [Google Scholar]
- Liu Z., Cai W., He L., Nakayama N., Chen K., Sun X., … Dai H. (2007). In vivo biodistribution and highly efficient tumour targeting of carbon nanotubes in mice. Nature Nanotechnology, 2(1), 47–52. 10.1038/nnano.2006.170 [Abstract] [CrossRef] [Google Scholar]
- Livingstone D. J., & Salt D. W. (2005). Judging the significance of multiple linear regression models. Journal of Medicinal Chemistry, 48(3), 661–663. 10.1021/jm049111p [Abstract] [CrossRef] [Google Scholar]
- Madru R., Kjellman P., Olsson F., Wingårdh K., Ingvar C., Ståhlberg F., … Strand S.‐E. (2012). 99mTc‐Labeled superparamagnetic iron oxide nanoparticles for multimodality SPECT/MRI of sentinel lymph nodes. Journal of Nuclear Medicine, 53(3), 459–463. [Abstract] [Google Scholar]
- Martelli C., Lo Dico A., Diceglie C., Lucignani G., & Ottobrini L. (2016). Optical imaging probes in oncology. Oncotarget, 7(30), 48753–48787. 10.18632/oncotarget.9066 [Europe PMC free article] [Abstract] [CrossRef] [Google Scholar]
- Mattu C., Brachi G., Menichetti L., Flori A., Armanetti P., Ranzato E., … Ciardelli G. (2018). Alternating block copolymer‐based nanoparticles as tools to modulate the loading of multiple chemotherapeutics and imaging probes. Acta Biomaterialia, 80, 341–351. 10.1016/j.actbio.2018.09.021 [Abstract] [CrossRef] [Google Scholar]
- Maute R. L., Gordon S. R., Mayer A. T., McCracken M. N., Natarajan A., Ring N. G., … Ring A. M. (2015). Engineering high‐affinity PD‐1 variants for optimized immunotherapy and immuno‐PET imaging. Proceedings of the National Academy of Sciences of the United States of America, 112(47), E6506 10.1073/pnas.1519623112 [Europe PMC free article] [Abstract] [CrossRef] [Google Scholar]
- McDonagh P. R., Sundaresan G., Yang L., Sun M., Mikkelsen R., & Zweit J. (2018). Biodistribution and PET imaging of 89‐zirconium labeled cerium oxide nanoparticles synthesized with several surface coatings. Nanomedicine, 14(4), 1429–1440. 10.1016/j.nano.2018.04.002 [Europe PMC free article] [Abstract] [CrossRef] [Google Scholar]
- McNally K., Cotton R., & Loizou G. (2011). A workflow for global sensitivity analysis of PBPK models. [Original Research]. Frontiers in Pharmacology, 2(31). 10.3389/fphar.2011.00031 [Europe PMC free article] [Abstract] [CrossRef] [Google Scholar]
- Meng F., Wang J., Ping Q., & Yeo Y. (2018). Quantitative assessment of nanoparticle biodistribution by fluorescence imaging, revisited. ACS Nano, 12(7), 6458–6468. 10.1021/acsnano.8b02881 [Europe PMC free article] [Abstract] [CrossRef] [Google Scholar]
- Morales‐Avila E., Ferro‐Flores G., Ocampo‐Garcia B. E., De Leon‐Rodriguez L. M., Santos‐Cuevas C. L., Garcia‐Becerra R., … Gomez‐Olivan L. (2011). Multimeric system of 99mTc‐labeled gold nanoparticles conjugated to c[RGDfK(C)] for molecular imaging of tumor alpha(v)beta(3) expression. Bioconjugate Chemistry, 22(5), 913–922. 10.1021/bc100551s [Abstract] [CrossRef] [Google Scholar]
- Mortezazadeh T., Gholibegloo E., Alam N. R., Dehghani S., Haghgoo S., Ghanaati H., & Khoobi M. (2019). Gadolinium (III) oxide nanoparticles coated with folic acid‐functionalized poly(beta‐cyclodextrin‐co‐pentetic acid) as a biocompatible targeted nano‐contrast agent for cancer diagnostic: in vitro and in vivo studies. Magma, 32(4), 487–500. 10.1007/s10334-019-00738-2 [Abstract] [CrossRef] [Google Scholar]
- Mukai H., Hatanaka K., Yagi N., Warashina S., Zouda M., Takahashi M., … Watanabe Y. (2019). Pharmacokinetic evaluation of liposomal nanoparticle‐encapsulated nucleic acid drug: A combined study of dynamic PET imaging and LC/MS/MS analysis. Journal of Controlled Release, 294, 185–194. 10.1016/j.jconrel.2018.12.006 [Abstract] [CrossRef] [Google Scholar]
- Natarajan A., Mayer A. T., Xu L., Reeves R. E., Gano J., & Gambhir S. S. (2015). Novel radiotracer for immunoPET imaging of PD‐1 checkpoint expression on tumor infiltrating lymphocytes. Bioconjugate Chemistry, 26(10), 2062–2069. 10.1021/acs.bioconjchem.5b00318 [Abstract] [CrossRef] [Google Scholar]
- Ng T. S., Garlin M. A., Weissleder R., & Miller M. A. (2020). Improving nanotherapy delivery and action through image‐guided systems pharmacology. Theranostics, 10(3), 968. [Europe PMC free article] [Abstract] [Google Scholar]
- Niemeijer A., Leung D., Huisman M., Bahce I., Hoekstra O., Van Dongen G., … Smith R. (2018). Whole body PD‐1 and PD‐L1 positron emission tomography in patients with non‐small‐cell lung cancer. Nature Communications, 9(1), 4664. [Europe PMC free article] [Abstract] [Google Scholar]
- Nizzero S., Li F., Zhang G., Venuta A., Borsoi C., Mai J., … Ferrari M. (2019). Systematic comparison of methods for determining the in vivo biodistribution of porous nanostructured injectable inorganic particles. Acta Biomaterialia, 97, 501–512. 10.1016/j.actbio.2019.08.002 [Abstract] [CrossRef] [Google Scholar]
- Nizzero S., Shen H., Ferrari M., & Corradetti B. (2019). Immunotherapeutic transport oncophysics: space, time, and immune activation in cancer. Trends in Cancer. [Abstract] [Google Scholar]
- Nizzero S., Ziemys A., & Ferrari M. (2018). Transport barriers and oncophysics in cancer treatment. Trends in Cancer, 4(4), 277–280. [Europe PMC free article] [Abstract] [Google Scholar]
- O'Connor J., Tofts P., Miles K., Parkes L., Thompson G., & Jackson A. (2011). Dynamic contrast‐enhanced imaging techniques: CT and MRI. The British Journal of Radiology, 84(special_issue_2), S112–S120. [Europe PMC free article] [Abstract] [Google Scholar]
- Oliveira S., Cohen R., Walsum M. S., van Dongen G. A., Elias S. G., van Diest P. J., … van Bergen En Henegouwen P. M. (2012). A novel method to quantify IRDye800CW fluorescent antibody probes ex vivo in tissue distribution studies. EJNMMI Research, 2(1), 50 10.1186/2191-219X-2-50 [Europe PMC free article] [Abstract] [CrossRef] [Google Scholar]
- Palm S., Enmon R. M. Jr., Matei C., Kolbert K. S., Xu S., Zanzonico P. B., … Sgouros G. (2003). Pharmacokinetics and biodistribution of (86)Y‐Trastuzumab for (90)Y dosimetry in an ovarian carcinoma model: Correlative microPET and MRI. Journal of Nuclear Medicine, 44(7), 1148–1155. [Abstract] [Google Scholar]
- Pascal J., Ashley C. E., Wang Z., Brocato T. A., Butner J. D., Carnes E. C., … Cristini V. (2013). Mechanistic modeling identifies drug‐uptake history as predictor of tumor drug resistance and nano‐carrier‐mediated response. ACS Nano, 7(12), 11174–11182. 10.1021/nn4048974 [Europe PMC free article] [Abstract] [CrossRef] [Google Scholar]
- Pascal J., Bearer E. L., Wang Z., Koay E. J., Curley S. A., & Cristini V. (2013). Mechanistic patient‐specific predictive correlation of tumor drug response with microenvironment and perfusion measurements. Proceedings of the National Academy of Sciences of the United States of America, 110(35), 14266–14271. 10.1073/pnas.1300619110 [Europe PMC free article] [Abstract] [CrossRef] [Google Scholar]
- Perera R. M., Zoncu R., Johns T. G., Pypaert M., Lee F.‐T., Mellman I., … Scott A. M. (2007). Internalization, intracellular trafficking, biodistribution of monoclonal antibody 806: A novel anti‐epidermal growth factor receptor antibody. Neoplasia, 9(12), 1099–1110. [Europe PMC free article] [Abstract] [Google Scholar]
- Pérez‐Medina C., Tang J., Abdel‐Atti D., Hogstad B., Merad M., Fisher E. A., … Reiner T. (2015). PET imaging of tumor‐associated macrophages with 89Zr‐Labeled high‐density lipoprotein nanoparticles. Journal of Nuclear Medicine, 56(8), 1272–1277. [Europe PMC free article] [Abstract] [Google Scholar]
- Peterson N. C., Wilson G. G., Huang Q., Dimasi N., & Sachsenmeier K. F. (2016). Biodistribution analyses of a near‐infrared, fluorescently labeled, bispecific monoclonal antibody using optical imaging. Comparative Medicine, 66(2), 90–99. [Europe PMC free article] [Abstract] [Google Scholar]
- Popovic Z., Liu W., Chauhan V. P., Lee J., Wong C., Greytak A. B., … Bawendi M. G. (2010). A nanoparticle size series for in vivo fluorescence imaging. Angewandte Chemie (International Ed. in English), 49(46), 8649–8652. 10.1002/anie.201003142 [Europe PMC free article] [Abstract] [CrossRef] [Google Scholar]
- Press O. W., Eary J. F., Appelbaum F. R., Martin P. J., Badger C. C., Nelp W. B., … Porter B. (1993). Radiolabeled‐antibody therapy of B‐cell lymphoma with autologous bone marrow support. New England Journal of Medicine, 329(17), 1219–1224. [Abstract] [Google Scholar]
- Rainone P., Riva B., Belloli S., Sudati F., Ripamonti M., Verderio P., … Prosperi D. (2017). Development of (99m)Tc‐radiolabeled nanosilica for targeted detection of HER2‐positive breast cancer. International Journal of Nanomedicine, 12, 3447–3461. 10.2147/IJN.S129720 [Europe PMC free article] [Abstract] [CrossRef] [Google Scholar]
- Ramanathan R. K., Korn R., Raghunand N., Sachdev J. C., Newbold R. G., Jameson G., … Kim J. (2017). Correlation between ferumoxytol uptake in tumor lesions by MRI and response to nanoliposomal irinotecan in patients with advanced solid tumors: A pilot study. Clinical Cancer Research, 23(14), 3638–3648. [Abstract] [Google Scholar]
- Reyes‐Aldasoro C. C., Wilson I., Prise V. E., Barber P. R., Ameer‐Beg M., Vojnovic B., … Tozer G. M. (2008). Estimation of apparent tumor vascular permeability from multiphoton fluorescence microscopic images of P22 rat sarcomas in vivo. Microcirculation, 15(1), 65–79. 10.1080/10739680701436350 [Abstract] [CrossRef] [Google Scholar]
- Rizk M. L., Zou L., Savic R. M., & Dooley K. E. (2017). Importance of drug pharmacokinetics at the site of action. Clinical and Translational Science, 10(3), 133–142. 10.1111/cts.12448 [Europe PMC free article] [Abstract] [CrossRef] [Google Scholar]
- Rowland M. (2013). Physiologically‐based pharmacokinetic (PBPK) modeling and simulations principles, methods, and applications in the pharmaceutical industry. CPT: Pharmacometrics & Systems Pharmacology, 2(7), e55–e55. 10.1038/psp.2013.29 [Europe PMC free article] [Abstract] [CrossRef] [Google Scholar]
- Ruggiero A., Villa C. H., Holland J. P., Sprinkle S. R., May C., Lewis J. S., … McDevitt M. R. (2010). Imaging and treating tumor vasculature with targeted radiolabeled carbon nanotubes. International Journal of Nanomedicine, 5, 783–802. 10.2147/IJN.S13300 [Europe PMC free article] [Abstract] [CrossRef] [Google Scholar]
- Ruiz‐Ramírez J., Ziemys A., Dogra P., & Ferrari M. (2020). A modeling platform for the lymphatic system. Journal of Theoretical Biology, 110193 10.1016/j.jtbi.2020.110193 [Europe PMC free article] [Abstract] [CrossRef] [Google Scholar]
- Sarin H. (2010). Physiologic upper limits of pore size of different blood capillary types and another perspective on the dual pore theory of microvascular permeability. Journal of Angiogenesis Research, 2(1), 14. [Europe PMC free article] [Abstract] [Google Scholar]
- Schlemmer H.‐P. W., Pichler B. J., Schmand M., Burbar Z., Michel C., Ladebeck R., … Claussen C. D. (2008). Simultaneous MR/PET imaging of the human brain: Feasibility study. Radiology, 248(3), 1028–1035. 10.1148/radiol.2483071927 [Abstract] [CrossRef] [Google Scholar]
- Schmidt M. M., & Wittrup K. D. (2009). A modeling analysis of the effects of molecular size and binding affinity on tumor targeting. Molecular Cancer Therapeutics, 8(10), 2861–2871. [Europe PMC free article] [Abstract] [Google Scholar]
- Sciumè G., Shelton S., Gray W., Miller C., Hussain F., Ferrari M., … Schrefler B. (2013). A multiphase model for three‐dimensional tumor growth. New Journal of Physics, 15, 015005 10.1088/1367-2630/15/1/015005 [Europe PMC free article] [Abstract] [CrossRef] [Google Scholar]
- Sciumè G., Shelton S. E., Gray W. G., Millers C. T., Hussain F., Ferrari M., … Schrefler B. A. (2012). Tumor growth modeling from the perspective of multiphase porous media mechanics. Molecular & Cellular Biomechanics, 9(3), 193–212. [Europe PMC free article] [Abstract] [Google Scholar]
- Scully P. R., Bastarrika G., Moon J. C., & Treibel T. A. (2018). Myocardial extracellular volume quantification by cardiovascular magnetic resonance and computed tomography. Current Cardiology Reports, 20(3), 15. [Europe PMC free article] [Abstract] [Google Scholar]
- Shah D. K., & Betts A. M. (2012). Towards a platform PBPK model to characterize the plasma and tissue disposition of monoclonal antibodies in preclinical species and human. Journal of Pharmacokinetics and Pharmacodynamics, 39(1), 67–86. [Abstract] [Google Scholar]
- Sharma P., Bengtsson N. E., Walter G. A., Sohn H. B., Zhou G., Iwakuma N., … Moudgil B. M. (2012). Gadolinium‐doped silica nanoparticles encapsulating indocyanine green for near infrared and magnetic resonance imaging. Small, 8(18), 2856–2868. 10.1002/smll.201200258 [Abstract] [CrossRef] [Google Scholar]
- Shi S., Xu C., Yang K., Goel S., Valdovinos H. F., Luo H., … Cai W. (2017). Chelator‐free radiolabeling of nanographene: Breaking the stereotype of chelation. Angewandte Chemie (International Ed. in English), 56(11), 2889–2892. 10.1002/anie.201610649 [Europe PMC free article] [Abstract] [CrossRef] [Google Scholar]
- Sirianni R. W., Zheng M. Q., Patel T. R., Shafbauer T., Zhou J., Saltzman W. M., … Huang Y. (2014). Radiolabeling of poly(lactic‐co‐glycolic acid) (PLGA) nanoparticles with biotinylated F‐18 prosthetic groups and imaging of their delivery to the brain with positron emission tomography. Bioconjugate Chemistry, 25(12), 2157–2165. 10.1021/bc500315j [Europe PMC free article] [Abstract] [CrossRef] [Google Scholar]
- Smith B. R., & Gambhir S. S. (2017). Nanomaterials for in vivo imaging. Chemical Reviews, 117(3), 901–986. [Abstract] [Google Scholar]
- Song M., Guo Z., Gao M., Shi C., Xu D., You L., … Zhang X. (2017). Synthesis and preliminary evaluation of a (99m) Tc‐labeled folate‐PAMAM dendrimer for FR imaging. Chemical Biology & Drug Design, 89(5), 755–761. 10.1111/cbdd.12899 [Abstract] [CrossRef] [Google Scholar]
- Stapleton S., Milosevic M., Allen C., Zheng J., Dunne M., Yeung I., & Jaffray D. A. (2013). A mathematical model of the enhanced permeability and retention effect for liposome transport in solid tumors. PLoS One, 8(12), e81157. [Europe PMC free article] [Abstract] [Google Scholar]
- Stylianopoulos T., Martin J. D., Snuderl M., Mpekris F., Jain S. R., & Jain R. K. (2013). Coevolution of solid stress and interstitial fluid pressure in tumors during progression: Implications for vascular collapse. Cancer Research, 73(13), 3833–3841. [Europe PMC free article] [Abstract] [Google Scholar]
- Tang L., Yang X., Yin Q., Cai K., Wang H., Chaudhury I., … Hartman J. A. (2014). Investigating the optimal size of anticancer nanomedicine. Proceedings of the National Academy of Sciences of the United States of America, 111(43), 15344–15349. [Europe PMC free article] [Abstract] [Google Scholar]
- Teng F., Meng X., Kong L., & Yu J. (2018). Progress and challenges of predictive biomarkers of anti PD‐1/PD‐L1 immunotherapy: A systematic review. Cancer Letters, 414, 166–173. 10.1016/j.canlet.2017.11.014 [Abstract] [CrossRef] [Google Scholar]
- Thompson M. D., & Beard D. A. (2011). Development of appropriate equations for physiologically based pharmacokinetic modeling of permeability‐limited and flow‐limited transport. Journal of Pharmacokinetics and Pharmacodynamics, 38(4), 405–421. [Europe PMC free article] [Abstract] [Google Scholar]
- Thurber G. M., Schmidt M. M., & Wittrup K. D. (2008). Antibody tumor penetration: transport opposed by systemic and antigen‐mediated clearance. Advanced Drug Delivery Reviews, 60(12), 1421–1434. [Europe PMC free article] [Abstract] [Google Scholar]
- Thurber G. M., & Weissleder R. (2011). A systems approach for tumor pharmacokinetics. PloS one, 6(9). [Europe PMC free article] [Abstract] [Google Scholar]
- Tofts P. S., Brix G., Buckley D. L., Evelhoch J. L., Henderson E., Knopp M. V., … Parker G. J. (1999). Estimating kinetic parameters from dynamic contrast‐enhanced T1‐weighted MRI of a diffusable tracer: Standardized quantities and symbols. Journal of Magnetic Resonance Imaging: An Official Journal of the International Society for Magnetic Resonance in Medicine, 10(3), 223–232. [Abstract] [Google Scholar]
- Tsoi K. M., MacParland S. A., Ma X.‐Z., Spetzler V. N., Echeverri J., Ouyang B., … Kaths J. M. (2016). Mechanism of hard‐nanomaterial clearance by the liver. Nature Materials, 15(11), 1212. [Europe PMC free article] [Abstract] [Google Scholar]
- van de Ven A. L., Wu M., Lowengrub J., McDougall S. R., Chaplain M. A. J., Cristini V., … Frieboes H. B. (2012). Integrated intravital microscopy and mathematical modeling to optimize nanotherapeutics delivery to tumors. AIP Advances, 2(1), 011208 10.1063/1.3699060 [Europe PMC free article] [Abstract] [CrossRef] [Google Scholar]
- van der Zwaag D., Vanparijs N., Wijnands S., De Rycke R., De Geest B. G., & Albertazzi L. (2016). Super resolution imaging of nanoparticles cellular uptake and trafficking. ACS Applied Materials & Interfaces, 8(10), 6391–6399. [Abstract] [Google Scholar]
- Vats M., Mishra S. K., Baghini M. S., Chauhan D. S., Srivastava R., & De A. (2017). Near infrared fluorescence imaging in nano‐therapeutics and photo‐thermal evaluation. International Journal of Molecular Sciences, 18(5), 924. 10.3390/ijms18050924 [Europe PMC free article] [Abstract] [CrossRef] [Google Scholar]
- Wall M. A., Shaffer T. M., Harmsen S., Tschaharganeh D.‐F., Huang C.‐H., Lowe S. W., … Kircher M. F. (2017). Chelator‐free radiolabeling of SERRS nanoparticles for whole‐body PET and intraoperative Raman imaging. Theranostics, 7(12), 3068–3077. 10.7150/thno.18019 [Europe PMC free article] [Abstract] [CrossRef] [Google Scholar]
- Wang Y., Liu Y., Luehmann H., Xia X., Brown P., Jarreau C., … Xia Y. (2012). Evaluating the pharmacokinetics and in vivo cancer targeting capability of Au nanocages by positron emission tomography imaging. ACS Nano, 6(7), 5880–5888. 10.1021/nn300464r [Europe PMC free article] [Abstract] [CrossRef] [Google Scholar]
- Wang Z., Butner J. D., Cristini V., & Deisboeck T. S. (2015). Integrated PK‐PD and agent‐based modeling in oncology. Journal of Pharmacokinetics and Pharmacodynamics, 42(2), 179–189. 10.1007/s10928-015-9403-7 [Europe PMC free article] [Abstract] [CrossRef] [Google Scholar]
- Wang Z., Butner J. D., Kerketta R., Cristini V., & Deisboeck T. S. (2015). Simulating cancer growth with multiscale agent‐based modeling. Seminars in Cancer Biology, 30, 70–78. 10.1016/j.semcancer.2014.04.001 [Europe PMC free article] [Abstract] [CrossRef] [Google Scholar]
- Wang Z., & Deisboeck T. S. (2014). Mathematical modeling in cancer drug discovery. Drug Discovery Today, 19(2), 145–150. 10.1016/j.drudis.2013.06.015 [Abstract] [CrossRef] [Google Scholar]
- Wang Z., & Deisboeck T. S. (2019). Dynamic targeting in Cancer treatment. Frontiers in Physiology, 10, 96 10.3389/fphys.2019.00096 [Europe PMC free article] [Abstract] [CrossRef] [Google Scholar]
- Wang Z., Kerketta R., Chuang Y.‐L., Dogra P., Butner J. D., Brocato T. A., … Cristini V. (2016). Theory and experimental validation of a spatio‐temporal model of chemotherapy transport to enhance tumor cell kill. PLoS Computational Biology, 12(6), e1004969 10.1371/journal.pcbi.1004969 [Europe PMC free article] [Abstract] [CrossRef] [Google Scholar]
- Warram J. M., de Boer E., Sorace A. G., Chung T. K., Kim H., Pleijhuis R. G., … Rosenthal E. L. (2014). Antibody‐based imaging strategies for cancer. Cancer Metastasis Reviews, 33(2–3), 809–822. 10.1007/s10555-014-9505-5 [Europe PMC free article] [Abstract] [CrossRef] [Google Scholar]
- Weidman E. K., Foley C. P., Kallas O., Dyke J. P., Gupta A., Giambrone A. E., … Sanelli P. C. (2016). Evaluating permeability surface‐area product as a measure of blood–brain barrier permeability in a murine model. American Journal of Neuroradiology, 37(7), 1267–1274. [Europe PMC free article] [Abstract] [Google Scholar]
- Wolfram J., Nizzero S., Liu H., Li F., Zhang G., Li Z., … Ferrari M. (2017). A chloroquine‐induced macrophage‐preconditioning strategy for improved nanodelivery. Scientific Reports, 7(1), 13738 10.1038/s41598-017-14221-2 [Europe PMC free article] [Abstract] [CrossRef] [Google Scholar]
- Xiao G., & Gan L.‐S. (2013). Receptor‐mediated endocytosis and brain delivery of therapeutic biologics. International Journal of Cell Biology, 2013. [Europe PMC free article] [Abstract] [Google Scholar]
- Xing Y., Chand G., Liu C., Cook G. J. R., O'Doherty J., Zhao L., … Zhao J. (2019). Early phase I study of a (99m)Tc‐labeled anti‐programmed death Ligand‐1 (PD‐L1) single‐domain antibody in SPECT/CT assessment of PD‐L1 expression in non‐small cell lung cancer. Journal of Nuclear Medicine, 60(9), 1213–1220. 10.2967/jnumed.118.224170 [Europe PMC free article] [Abstract] [CrossRef] [Google Scholar]
- Xu Y., Shan Y., Zhang Y., Yu B., Shen Y., & Cong H. (2019). Multifunctional Fe3O4@C‐based nanoparticles coupling optical/MRI imaging and pH/photothermal controllable drug release as efficient anti‐cancer drug delivery platforms. Nanotechnology, 30(42), 425102 10.1088/1361-6528/ab2e40 [Abstract] [CrossRef] [Google Scholar]
- Zhai C., Summer D., Rangger C., Franssen G. M., Laverman P., Haas H., … Decristoforo C. (2015). Novel bifunctional cyclic chelator for 89Zr labeling—Radiolabeling and targeting properties of RGD conjugates. Molecular Pharmaceutics, 12(6), 2142–2150. 10.1021/acs.molpharmaceut.5b00128 [Europe PMC free article] [Abstract] [CrossRef] [Google Scholar]
- Zhang F., Niu G., Lu G., & Chen X. (2011). Preclinical lymphatic imaging. Molecular Imaging and Biology, 13(4), 599–612. [Europe PMC free article] [Abstract] [Google Scholar]
- Zhang S., Gao H., & Bao G. (2015). Physical principles of nanoparticle cellular endocytosis. ACS Nano, 9(9), 8655–8671. [Europe PMC free article] [Abstract] [Google Scholar]
- Zhang Y., Hong H., & Cai W. (2011). PET tracers based on zirconium‐89. Current Radiopharmaceuticals, 4(2), 131–139. [Europe PMC free article] [Abstract] [Google Scholar]
- Zhao J., Cao Y., & Jusko W. J. (2015). Across‐species scaling of monoclonal antibody pharmacokinetics using a minimal PBPK model. Pharmaceutical Research, 32(10), 3269–3281. 10.1007/s11095-015-1703-5 [Europe PMC free article] [Abstract] [CrossRef] [Google Scholar]
- Zhu J., Chin J., Wangler C., Wangler B., Lennox R. B., & Schirrmacher R. (2014). Rapid (18)F‐labeling and loading of PEGylated gold nanoparticles for in vivo applications. Bioconjugate Chemistry, 25(6), 1143–1150. 10.1021/bc5001593 [Abstract] [CrossRef] [Google Scholar]
- Zhu J., Li H., Xiong Z., Shen M., Conti P. S., Shi X., & Chen K. (2018). Polyethyleneimine‐coated manganese oxide nanoparticles for targeted tumor PET/MR imaging. ACS Applied Materials & Interfaces, 10(41), 34954–34964. 10.1021/acsami.8b12355 [Europe PMC free article] [Abstract] [CrossRef] [Google Scholar]
- Zhu J., Lin S., Leow C. H., Rowland E. M., Riemer K., Harput S., … Tang M.‐X. (2019). High frame rate contrast‐enhanced ultrasound imaging for slow lymphatic flow: Influence of ultrasound pressure and flow rate on bubble disruption and image persistence. Ultrasound in Medicine & Biology, 45(9), 2456–2470. [Abstract] [Google Scholar]
- Zhu W., Guo J., Ju Y., Serda R. E., Croissant J. G., Shang J., … Brinker C. J. (2019). Modular metal‐organic polyhedra superassembly: From molecular‐level design to targeted drug delivery. Advanced Materials, 31(12), e1806774 10.1002/adma.201806774 [Europe PMC free article] [Abstract] [CrossRef] [Google Scholar]
Full text links
Read article at publisher's site: https://doi.org/10.1002/wnan.1628
Read article for free, from open access legal sources, via Unpaywall:
https://onlinelibrary.wiley.com/doi/pdfdirect/10.1002/wnan.1628
Citations & impact
Impact metrics
Article citations
Rational nanoparticle design: Optimization using insights from experiments and mathematical models.
J Control Release, 360:772-783, 22 Jul 2023
Cited by: 0 articles | PMID: 37442201 | PMCID: PMC10529591
Review Free full text in Europe PMC
Mathematical modeling of cancer immunotherapy for personalized clinical translation.
Nat Comput Sci, 2(12):785-796, 19 Dec 2022
Cited by: 11 articles | PMID: 38126024 | PMCID: PMC10732566
Protocol for mathematical prediction of patient response and survival to immune checkpoint inhibitor immunotherapy.
STAR Protoc, 3(4):101886, 30 Nov 2022
Cited by: 1 article | PMID: 36595890 | PMCID: PMC9719106
Zonal Intratumoral Delivery of Nanoparticles Guided by Surface Functionalization.
Langmuir, 38(45):13983-13994, 01 Nov 2022
Cited by: 2 articles | PMID: 36318182 | PMCID: PMC9671122
Opportunities for improving brain cancer treatment outcomes through imaging-based mathematical modeling of the delivery of radiotherapy and immunotherapy.
Adv Drug Deliv Rev, 187:114367, 30 May 2022
Cited by: 10 articles | PMID: 35654212
Review
Go to all (17) article citations
Data
Data behind the article
This data has been text mined from the article, or deposited into data resources.
BioStudies: supplemental material and supporting data
Similar Articles
To arrive at the top five similar articles we use a word-weighted algorithm to compare words from the Title and Abstract of each citation.
Theranostic small interfering RNA nanoparticles in cancer precision nanomedicine.
Wiley Interdiscip Rev Nanomed Nanobiotechnol, 12(2):e1595, 23 Oct 2019
Cited by: 10 articles | PMID: 31642207 | PMCID: PMC7360334
Review Free full text in Europe PMC
Targeted Nanocurcumin Therapy Using Annexin A2 Anitbody Improves Tumor Accumulation and Therapeutic Efficacy Against Highly Metastatic Breast Cancer.
J Biomed Nanotechnol, 12(7):1374-1392, 01 Jul 2016
Cited by: 15 articles | PMID: 29336533
Embracing nanomaterials' interactions with the innate immune system.
Wiley Interdiscip Rev Nanomed Nanobiotechnol, 13(6):e1719, 13 Apr 2021
Cited by: 6 articles | PMID: 33847441 | PMCID: PMC8511354
Review Free full text in Europe PMC
Recent advances in applications of nanoparticles in SERS in vivo imaging.
Wiley Interdiscip Rev Nanomed Nanobiotechnol, 13(2):e1672, 18 Oct 2020
Cited by: 21 articles | PMID: 33073511
Review
Funding
Funders who supported this work.
NCI NIH HHS (3)
Grant ID: R01 CA222007
Grant ID: U54 CA210181
Grant ID: R01 CA226537
NIH HHS (5)
Grant ID: U54CA210181
Grant ID: 1U01CA196403
Grant ID: 1R01CA226537
Grant ID: 1U01CA213759
Grant ID: 1R01CA222007
National Institutes of Health (5)
Grant ID: 1R01CA222007
Grant ID: U54CA210181
Grant ID: 1R01CA226537
Grant ID: 1U01CA213759
Grant ID: 1U01CA196403
National Science Foundation (1)
Grant ID: 1930583