Abstract
Free full text

Identification and functional characterization of new missense SNPs in the coding region of the TP53 gene
Abstract
Infrequent and rare genetic variants in the human population vastly outnumber common ones. Although they may contribute significantly to the genetic basis of a disease, these seldom-encountered variants may also be miss-identified as pathogenic if no correct references are available. Somatic and germline TP53 variants are associated with multiple neoplastic diseases, and thus have come to serve as a paradigm for genetic analyses in this setting. We searched 14 independent, globally distributed datasets and recovered TP53 SNPs from 202,767 cancer-free individuals. In our analyses, 19 new missense TP53 SNPs, including five novel variants specific to the Asian population, were recurrently identified in multiple datasets. Using a combination of in silico, functional, structural, and genetic approaches, we showed that none of these variants displayed loss of function compared to the normal TP53 gene. In addition, classification using ACMG criteria suggested that they are all benign. Considered together, our data reveal that the TP53 coding region shows far more polymorphism than previously thought and present high ethnic diversity. They furthermore underline the importance of correctly assessing novel variants in all variant-calling pipelines associated with genetic diagnoses for cancer.
Introduction
The evaluation of germline and/or somatic TP53 status is becoming mandatory in a number of clinical situations. In patients with Li–Fraumeni syndrome or families with hereditary breast and ovarian cancer syndrome, the early identification of germline TP53 mutations has been shown to be highly beneficial for disease surveillance [1]. In chronic lymphocytic leukemia, acute myeloid leukemia, and myelodysplastic syndrome, somatic TP53 variants are associated with poor prognosis, and furthermore, they are now investigated in routine clinical practice with the aim of identifying patients who would benefit from specific treatment [2, 3].
It is therefore essential that the identification and classification of TP53 variants meet quality requirements for clinical diagnostics used in personalized medicine. However, the analysis of somatic or germline TP53 variants faces a range of challenges. In tumors, the somatic origin of mutations can be inferred via comparison with matched normal DNA from the same patient. Unfortunately, this material is often missing, necessitating indirect assumptions made using databases that include the most frequent germline variants observed in the human population. For the analysis of germline variants, data are directly compared to population databases. In both cases, the accuracy of the results will depend on the characteristics of the population databases. Among those characteristics are not only the increasing number of rare variants found in the human population but also the ethnic diversity of this latter.
The advent of massively parallel sequencing (next-generation sequencing, or NGS) has shown that the human genome includes far more genetic variation than anticipated, with many variants detected at frequencies far below the formerly used 1% limit [4]. To illustrate, the most frequent build (151) of dbSNP includes 335 million variants. However, several studies have questioned the quality of the entries, among which both pathogenic and nonpathogenic TP53 variants are included [5, 6]. In contrast, data from the 1000 Genomes Project have been highly curated, but the small number of individuals (2504) included in the project does not enable the full coverage of low-frequency variants. To circumvent this issue, the Exome Aggregation Consortium released the ExAC database, which compiles whole-exome sequencing data from 60,706 individuals [7]. ExAC includes not only data from the 1000 Genomes Project but also constitutional data from unrelated individuals sequenced as part of various disease-specific and population genetics studies. In 2016, ExAC became the Genome Aggregation Database (gnomAD) with an enlarged dataset providing information from 123,136 exome sequences and 15,496 whole-genome sequences from unrelated individuals (http://gnomad.broadinstitute.org/). Both ExAC and gnomAD have been widely used as a substitute for or a complement to dbSNP and are currently used in multiple analytical pipelines.
Two missense SNPs in the TP53 gene have been identified and extensively characterized. SNP rs1042522 (p.P72R) is common in all world populations but the ancestral allele (Pro) shows a north-south gradient ranging between 0.2 and 0.7 [8]. The second SNP, rs1800371 (p.P47S), has been shown to be specific to the African population, albeit at a lower frequency (0.01–0.02) [9]. Both SNPs are included in ClinVar and considered benign according to American College of Medical Genetics and Genomics (ACMG) criteria.
In the present study, using a combination of in silico, in vitro, structural and genetic approaches, we addressed a two-tiered question: (i) is it possible to identify novel and infrequent TP53 SNPs in the human population and (ii) what is the potential pathogenicity of these variants? Using aggregated and population-specific databases, in silico and functional studies, and clinical data, we identify 19 new exonic TP53 SNPs including five variants specific to the Asian population.
Results
Identification of new TP53 SNPs in the human population
To identify novel infrequent TP53 SNPs in the human population, fourteen independent datasets compiled from individuals without neoplastic disease (202,767 individuals) were screened for variants located anywhere in the entire TP53 gene (Fig. 1A and Table S1). Three datasets included aggregated data from multiple studies, whereas 11 comprised data from specific countries. These latter were globally distributed to increase the identification of population-specific variants. Furthermore, data overlap between these datasets was minimal, which avoided excessive redundancy in the analysis (“Materials and methods”).
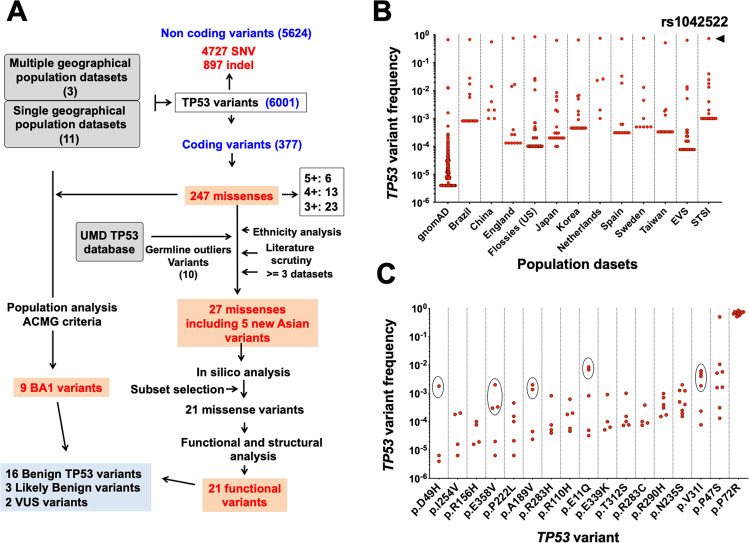
A Flow chart of the strategy used to identify new germline TP53 variants in the human population. Three general population datasets and 11 country-specific datasets, the latter not included in the former, were screened for TP53 variants. The 388 missense TP53 variants were analyzed for potential constitutional variants as described in the text. B Allele frequency distribution of exonic TP53 variants in the 14 populations. rs1042522 (p.P72R) was found at high frequency, regardless of the dataset as shown at the top of the figure. C Allele frequency of TP53 variants TP53 variants found in the present study. For each variant found in four or more population datasets, the allele frequency for each dataset is shown. For five variants, the Asian populations have been circled to show the preferential origin of these variants. (A full vesrion of tis figure is available in Fig S1d).
We thus uncovered 6001 different TP53 variants at frequencies ranging from 0.8 to 10−6, and, as expected, most of them were located in noncoding sequences of the TP53 gene (5628 noncoding and 373 coding) (Table S2a and Fig. S1). For the present work, we focused our analysis on missense variants. Other variants such as intronic, synonymous, or specific TP53 isoform-targeting variants are fully described in the Supplementary Information. The particular case of rs138729528, (p.R175C), a passenger mutation found at high frequency in human tumors and in the normal population is also discussed in the Supplementary Information. We identified 247 missense variants in the 14 datasets, with 4, 6, and 14 variants found in more than 5, 4, and 3 datasets, respectively (Figs. 1A, S1b and c, and Table S2c). Their frequencies ranged from 0.8 to 4×
10−6. The two most common benign TP53 SNPs found in the human population, i.e., rs1042522 (p.P72R) and rs1800371 (p.P47S), are included in this list (found in 14 and 7 datasets, respectively) (Figs. 1B, C and S1b and c). The statuses of the remaining variants were unknown, but their recurrences in various independent datasets suggested that they could also be rare constitutional TP53 SNPs.
The gnomAD dataset includes population data that enables subdivision into eight classes according to ancestry. rs1042522 (p.P72R) was found in all ethnic groups at a frequency ranging from 0.4 to 0.7 (Fig. 2A). Our analysis confirmed previous observations of a lower frequency for rs1042522 in the African population (0.4 in our analysis). Those observations have led some authors to hypothesize that the frequency of this polymorphism may be latitude-dependent [8]. In the present analysis, we also confirmed the quasi-exclusivity of rs1800371 (p.P47S) in the African population, as previously observed in other studies [10] (Figs. 2A and S2). Unexpectedly, our analysis uncovered five novel variants that were present in only the East Asian (EAS) population of gnomAD (rs201753350; (p.V31I), rs587780728; (p.D49H), rs201382018; (p.E11Q), rs121912665; (p.A189V), and rs773553186; (p.E358V)) (Fig. 2B). This specificity was confirmed by the analysis of datasets specific to Asian populations (Taiwan, Korea, China, and Japan) (Figs. 2B and S2). These five variants are also included in such cancer mutation databases as Cosmic, UMD, and IARC. Because normal DNA is usually not available, the constitutional origin of these variants may have been missed. The UMD_TP53 database included 146 entries for these five variants published in 64 reports. For all of these latter, the ethnicity of all patients was carefully checked and shown to be highly predominantly Asian (139 out of 146 patients) (Fig. 2C and Table S3). Notably, in two American multicenter studies that included patients from various geographical regions, these variants were only identified in Asian patients. We also noticed that the nine cell lines expressing any one of these variants originated from Japanese patients (Table S3). Taken together, these results clearly showed that the five novel constitutional variants were indeed specific to the Asian population. Whether any of these infrequent variants originated from a single event, i.e., a founder mutation, is currently unknown. A sixth rare variant, rs72661119; (p.N263D), was identified specifically in the South Asian (SAS) population of gnomAD with multiple reports originating from India (Fig. 2C and Table S3).
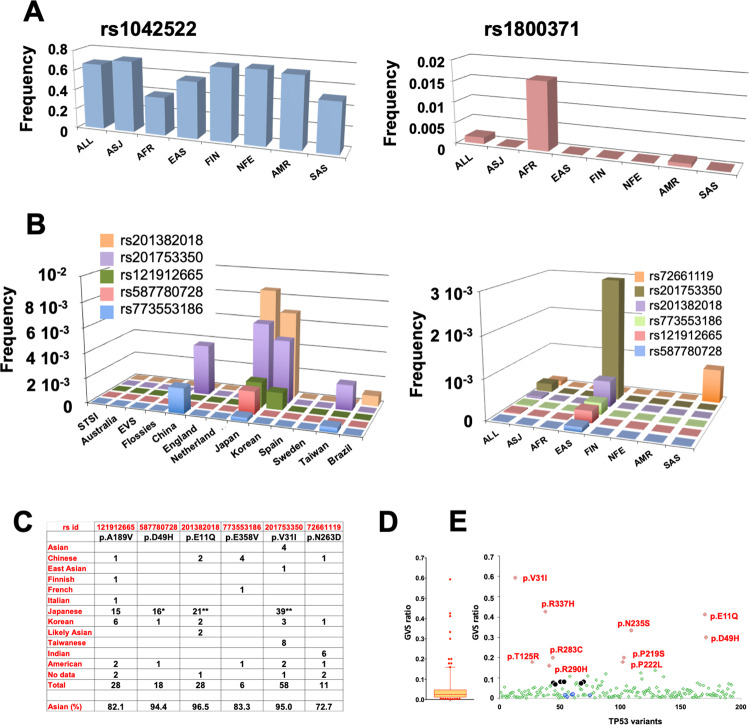
A Geographical distribution of rs1042522 (p.P72R) and rs1800371 (p.P47S) in the various subsets of gnomAD. B Distribution of six new potential constitutional TP53 variants in the various subsets of gnomAD (left) and in the specific population datasets (right). ALL all populations, AFR African/African American, AMR admixed American, ASJ Ashkenazi Jewish, EAS East Asian, FIN, Finnish, NFE non-Finnish European, OTH other unassigned populations, SAS South Asian. C Ethnicity origin of the six variants described in the literature of the UMD_TP53 database. * and **: including two or three different cell lines, respectively. D, E Germline-to-somatic (GVS) ratio of TP53 variants in the UMD_TP53 database. D A box-plot analysis of the GVS ratio shows that the vast majority of TP53 variants had similar values. Ten outlier variants above the 95% confidence interval had high frequency as germline variants in the database and could be benign SNPs. TP53 variants found below the 5% interval were never found as germline variants in the database; they corresponded to specific TP53 variants associated with carcinogen exposure, such as p.R249S found in aflatoxin B1-associated hepatocellular carcinoma, and p.V157F found in tobacco-associated lung cancer. The box-and-whisker plot shows the interquartile range (box), median value (horizontal line inside the box), and full-range distribution (whiskers) for the GVS ratio. E Detailed analysis of the germline ratio. The ten outlier variants are shown in red. Values for six hot spot TP53 variants are shown in black. This analysis was performed on 195 TP53 variants with at least five occurrences as germline variants in the database.
The various TP53 mutations databases include both somatic variants from multiple types of cancer and germline variants from a range of families or patient cohorts with Li–Fraumeni syndrome or hereditary breast and ovarian cancer syndrome. As discussed above, normal tissue was not available for many of the 80,000 tumors included in the UMD_TP53 database; thus assessments of variant origin had often been done by comparison with dbSNP, which includes a limited number of TP53 population variants. It is therefore likely that infrequent germline variants were mistakenly identified as somatic variants, leading to database contamination. We therefore evaluated the germline-to-somatic ratio for each TP53 variant in the UMD_TP53 database. As shown in Fig. 2D, the distribution of this ratio for the majority of TP53 variants, including all TP53 mutation hot spots, was quite low, ranging from 0.03 to 0.056. Ten outlier variants displayed a high ratio, suggesting that they may be undetected germline variants (Fig. 2E). Indeed, nine of these outlier variants are included in the 247 missense variants described above, with seven of them identified in three or more datasets. They also included three of the variants detected in the Asian population.
In a study published by our group, p.N235S was detected in a patient with lung cancer and defined as somatic [11]. However, when we reanalyzed this patient by sequencing normal DNA, we found that the variant was germline (Fig. S3a). Similarly, reanalysis of normal DNA from chronic lymphocytic leukemia patients confirmed the germline origin of this variant as well as variants p.R290H, p.R283C, and p.G360A (Fig. S3b–f).
Our analysis suggests that the coding region of the TP53 gene includes far more ethnically distributed SNPs than was previously thought, and that some of them are mistakenly included as somatic variants in a number of databases. It is therefore essential to determine whether or not the p53 protein encoded by these SNPs is functional and how they must be classified according to ACMG criteria.
Predictive and functional analysis of TP53 variants; in silico analysis
Computational algorithms to predict the functional effect of missense variants were performed on 27 SNPs (set 27) found in at least three datasets, and on the outlying germline variants identified in the analysis of the UMD_TP53 database described in the previous section (Table S2c). First, we used the prediction scores from dbNSFP, a database that compiles scores from several popular algorithms along with a conservation score and other related information for every potential single nucleotide variant in the human genome [12] (“Material and methods”). Most of these algorithms are based on sequence homology and/or the physical properties of amino acids (Table S4). They are widely used for a global estimation of the deleteriousness of missense variants but they are not specific to any protein. We also used new in silico mutation prediction tools such as Revel or Envision that have been trained on known pathogenic and neutral nsSNVs. Among the 27 analyzed TP53 variants, 4 were shown to be included in the cancer shared dataset (CSD), including the hot spot variant p.R248Q (Fig. 3). Although the majority of individuals included in the various datasets were selected for nonneoplastic disease, it is known that the frequency of de novo germline TP53 mutation in the human population is high, ranging from 1/5000 to 1/20,000 [13, 14]. It is therefore not surprising that among the 138,632 individuals in gnomAD as well as those in other population datasets, some may have been carrying such de novo mutations, although without cancer at the time of inclusion [15]. The rank scores of TP53 variants found in set 27 were always associated with a low pathogenic score, in contrast to the scores of the CSD-associated variants (Fig. 3). We note too that except for a few variants, there was considerable heterogeneity among the employed predictive tools.
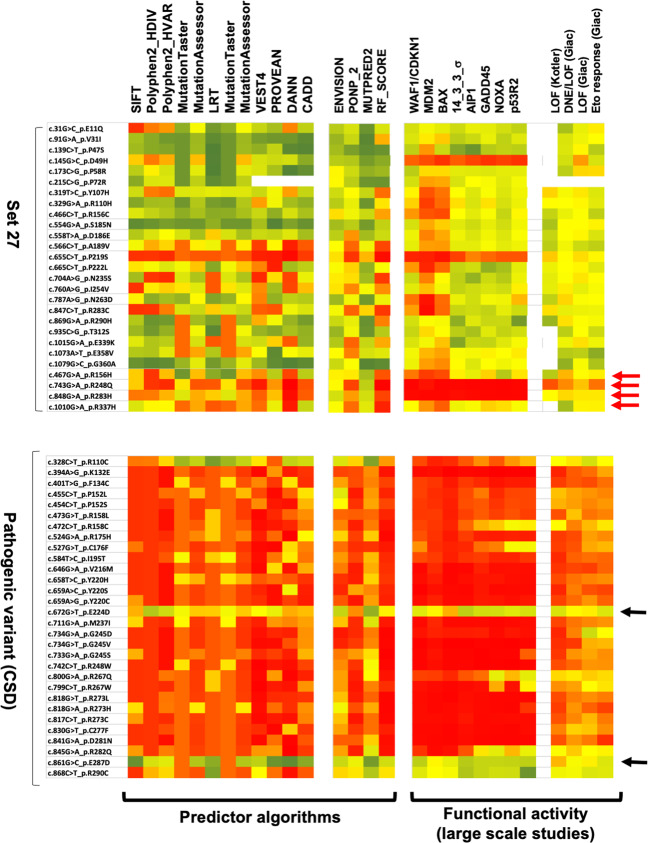
The heat map corresponds to either the loss of function (right panels) or the predictive impact (left panels) of each TP53 variant ranging from 0 (red) to 1 (green), with the lowest score being the most deleterious. TP53 variants from set 27 and from pathogenic TP53 from the CSD are shown, respectively, in the upper and lower part of the figure. Functional activities defined experimentally by the three large-scale analyses performed by Kato et al. (transcriptional activity in yeast using eight different TP53 response elements), Giacomelli et al. (dominant negative activity, growth arrest i.e., loss of function (LOF) or response to etoposide), and Kotler et al. (growth arrest in mammalian cells i.e., LOF) are shown in the right panels of the figure. Computational algorithms used to predict the deleteriousness of TP53 activity are shown in the left panels of the figure. Scores from the 12 algorithms in the left panels were derived from the dbSNFP, whereas the four scores were obtained from each predictor. A full description of the scorings used in the figure can be found in Table S4. Red arrows indicate pathogenic CSD TP53 variants included in gnomAD; black arrows indicate CSD TP53 variants known to impair TP53 splicing.
A unique benefit TP53 has over other genes is the availability of quantitative functional data for all missense variants. In a pioneer study, Kato et al. engineered 2100 TP53 variants and assessed their transactivation activity in a yeast assay using eight different TP53 response elements. Their approach yielded a quantitative score for each variant [16]. Our analysis of the residual activity of the variants showed clearly that most TP53 variants, with the exception of those included in the CSD, were still functional (Fig. 3).
Two recent studies looked at large libraries to explore the loss of function of TP53 variants in mammalian cells and extend the functional analysis to a range of properties including growth arrest, apoptosis, dominant negative effect, or response to etoposide stress [17, 18]. In both studies, each TP53 variant was given a score indicating its potency to alter TP53 function. These novel scores agreed well with the transcriptional data collected in the yeast studies and showed clearly that there was good correlation between yeast and mammalian data (Fig. 3). Ranking data from the work of Kotler et al. showed that the 11 variants in the central DNA-binding domain (DBD) did not cause loss of activity (Fig. S4) [17].
This analysis using functional data from large-scale, saturating mutagenesis showed that most of these variants were likely to be functional, in contrast to their CSD-associated counterparts. Furthermore, as expected, using TP53-functional criteria improved the classification of the different variants compared to predictive algorithms.
Functional analysis
Although our population and prediction analyses suggested strongly that these newly discovered variants were functional, we nonetheless chose a subset of 21 TP53 variants (set 21) for a detailed functional analysis (Tables 1 and S1). Transcriptional activities were tested using three different assays. FASAY, a yeast assay, initially described by Iggo et al. has been widely used in the clinic for rapid screening of TP53 status and was furthermore used by Kato et al. to define TP53 activity as described in the previous section [19]. FASAY enables the detection of variants with particular temperature sensitivities (Fig. S5). In our work, all tested variants were shown to be fully active at three temperatures (25, 30, and 35°C) using the WAF1 promoter [20]. Cancer hot spot variants p.I254T (temperature-sensitive) and p.R175H (totally defective) were used as controls (Tables 1 and S5). TP53 variants were also expressed in the p53-null cell line H1299 to determine their function based on transactivation of a TP53-dependent luciferase reporter with various TP53 response elements. These variants had profiles indistinguishable from that of wild-type TP53 (Figs. 4A–C, S6 and Tables Tables11, S5).
Table 1
Functional analysis and classification of 21 TP53 variants found in the human populationa.
TP53_variant | Functional analysisb | Growth suppression (Kotler et al.) | DNE/LOF (Giacomelli et al.) | LOF (Giacomelli et al.) | Population classification (ACMG) | Population classification (ACMG)c | ClinVar datad |
---|---|---|---|---|---|---|---|
c.31G>C_E11Q | Functional | No data | Functional | Functional | BA1 (Japan; Korea); BS1(EAS) | Benign (BA1; BS3; BP4) | LB (2) VUS (4) |
c.91G>A_V31I | Functional | No data | Functional | Functional | BA1 (China; Japan; Korea; Taiwan; gnomAD_EAS) | Benign (BA1; BS3; BP4) | B (1) LB (4) LP (1) VUS (3) Star (1) |
c.139C>T_P47S | Functional | No data | Functional | Functional | BA1 (Brazil; Flossies; GnomAD; Kaviar; EVS; gnomAD_AFR) | Benign (BA1; BS3; BP4) | B (10) VUS (1) Star (1) |
c.145G>C_D49H | Functional | No data | Functional | Functional | BA1 (Japan) | Benign (BA1; BS3; BP4) | VUS |
c.173C>G_P58R | Functional | No data | Functional | Functional | BS1 (gnomAD_AFR; Flossies; EVS) | Benign (BA1; BS3; BP4) | LB (6) VUS (1) |
c.215C>G_P72R | Functional | No data | Functional | Functional | BA1 (all datasets) | Benign (BA1; BS3; BP4) | B Star (3) |
c.319T>C_Y107H | Functional | Functional | Functional | Functional | BA1 (gnomAD_AFR); BS1 (Flossies) | Benign (BA1; BS3; BP4) | LB (3) VUS (4) |
c.329G>A_R110H | Functional | Functional | Functional | Functional | – | Likely benign (BS3; BP4) | VUS |
c.466C>T_R156C | Functional | Functional | Functional | Functional | – | Likely benign (BS3; BP4) | VUS |
c.554G>A_S185N | Functional | Functional | Functional | Functional | – | Likely benign (BS3; BP4) | VUS |
c.566C>T_A189V | Functional | Functional | Functional | Functional | BA1 (China) | Benign (BA1; BS3; BP4) | VUS |
c.665C>T_P222L | Functional | Functional | Functional | Functional | – | VUS (BS3) | VUS |
c.704A>G_N235S | Functional | Functional | Functional | Functional | BA1 (Australia); BS1 (FIN; Finland; NFE) | Benign (BA1;BS1;BP4) | B (1)LB (5) VUS (3) Star (1) |
c.760A>G_I254V | Functional | Functional | Functional | Functional | – | VUS (PP3; BS3) | VUS |
c.787A>G_N263D | Functional | Functional | Functional | Functional | SAS | Benign (BS1; BS3) | LB (1) VUS (4) |
c.847C>T_R283C | Functional | Functional | Functional | Functional | EVS | Benign (BS1; BS3) | LB (1) LP (1) VUS (12) |
c.869G>A_R290H | Functional | Functional | Functional | Functional | BS1 (Finland; Flossies; FIN) | Benign (BS1; BS3) | B (1) LB (1) VUS (10) Star (1) |
c.935C>G_T312S | Functional | No data | Functional | Functional | BS1 (gnomAD_AFR) | Benign (BS1; BS3) | B (1)LB (1) VUS (4) Star (1) |
c.1015G>A_E339K | Functional | No data | Functional | Functional | BS1 (gnomAD_EAS) | Benign (BS1; BS3) | LB (2) VUS (3) |
c.1073A>T_E358V | Functional | No data | Functional | Functional | BA1 (China) | Benign (BA1; BS3; BP4) | LB (1) VUS (2) Star (2) |
c.1079G>C_G360A | Functional | No data | Functional | Functional | BS1 (gnomAD_LAT; gnomAD_AFR) | Benign (BS1; BS3) | B LB |
c.524G>A_R175H | Defective | Defective | Defective | Defective | – | Pathogenic (PS3; PS4; PM1; PP3) | P |
c.761T>C_I254T | Defective | Defective | Defective | Defective | – | Pathogenic (PS3; PS4; PM1; PP3) | No data |
c.1025G>C_R342P | Defective | Functional | Functional | Defective | – | Pathogenic (PS3; PS4; PM1; PP3) | LP P |
c.428T>C_V143A | Defective | Defective | Defective | Defective | – | Pathogenic (PS3; PS4; PM1; PP3) | No data |
DNE dominant negative effect, LOF loss of function.
aA complete version of this table with detailed description of all data is available in Table S5.
bFunctional analysis includes transcriptional activity, apoptosis, and cell proliferation assays performed both in human cells or in yeast in the present study.
cFor each classification, identity of the dataset is indicated in brackets. gnomAD data were split into eight geographical datasets (EAS, AFR, SAS, FIN, LAT, EAS, ASJ, and OTH) leading to a total of 22 datasets.
dAssessed June 2020.
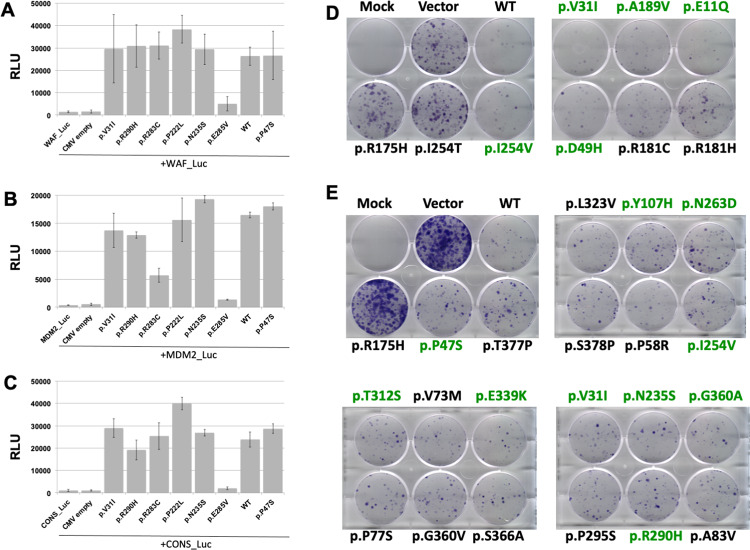
Transcriptional activity analysis: WAF1 (A), MDM2 (B), and CONS (C) promoters upstream of the luciferase reporter were transiently transfected in H1299 cells with a range of TP53 variants. Luciferase activity in the cell lysates was determined at 24h after transfection (see also Fig. S6 for other TP53 variants). Colony growth arrest: H1299 cells were transfected with various TP53 variants and selected for 2 weeks in a selective medium with G418 before staining with crystal violet. Mock: non-transfected cells. TP53 variants with impaired activities (p.R175H and p.I254T) were used as controls. Two independent experiments are shown in this figure (D, E). Variants from set 21 are shown in green. Other experiments and quantitative analyses of colony numbers are available in Fig. S8.
To confirm the validity of this analysis on TP53 regulation, the expression levels of endogenous TP53 target genes were also analyzed by qPCR after transfection of several variants into H1299 cells (Fig. S7a, b and Table S5). Genes involved in several TP53 pathways such as cellular response to DNA damage (WAF1, ZMAT3, MDM2), DNA repair (DDB2), metabolism (TIGAR), apoptosis (FAS, APAF1), or reactive oxygen species regulation (SESN1) were fully activated compared with pathogenic TP53 variants.
Two other functional assays, colony-formation assay and apoptosis, were also performed to assess the biological function of these variants (Figs. 4D, E and S8, S9a–c, and Table S5). Except for CSD TP53 variants used as a negative control, no loss of activity was observed for the various SNPs. The collective results of our analysis showed that these latter were indistinguishable from wild-type TP53.
Location of TP53 variants and predicted impact
We mapped the identified TP53 variants within the structure of p53. Quite a few SNPs were located in the intrinsically disordered N-terminal transactivation domain (TAD) and proline-rich region. They did not modify the molecular recognition feature for binding of the negative regulators MDM2/X or the TAZ1 domain of transcriptional coactivator p300. Interestingly, Asp49 within the nascent helix of the p53 TAD forms an intermolecular salt bridge with an arginine in complex with the nuclear receptor coactivator binding domain of the CREB binding protein (Fig. 5A), which has been suggested to contribute to binding specificity [21]. This specific salt-bridge partner was lost in p.D49H variants. The p.P72R variant reduced slightly the rigidity of the proline-rich region, which may serve as a stiff linker for projecting the TADs away from the core of the p53-DNA complex for optimal interaction with coactivators [22]. Whether this variant has an effect on cancer predisposition remains controversial/inconclusive [23].
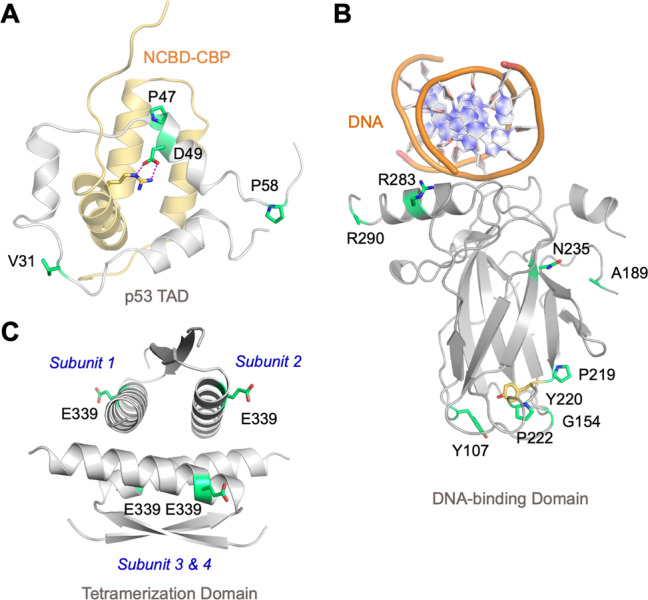
A Solution structure of p53 TAD in complex with the nuclear receptor coactivator binding domain of CREB binding protein (NCBD-CBP) (PDB entry 2L14). B Crystal structure of the DBD bound to target DNA (PDB entry 3KZ8, chain A). C Crystal structure of the tetramerization domain (PDB entry 1C26). p53 domain structures in A–C are shown as cartoons in gray, with the SNP sites highlighted as green stick models. NCBD-CBP is shown as a light-brown cartoon, and the side chain of the arginine involved in an intermolecular salt bridge with p53 is shown as a stick model.
All eight DBD variants affected residues on or close to the protein surface (Figs. 5B and S10a–f). Four of them, p.Y107H, p.G154S, p.P219L, and p.P222L, clustered on a surface patch, distant from the DNA-binding surface (and other functional interfaces), around the site of the cancer hot spot mutation p.Y220C. This latter creates an extended surface crevice and is highly destabilizing; it lowers the melting temperature of the DBD by about 8°C, causing it to rapidly unfold and aggregate at body temperature [24]. Structural models of the variant DBDs indicated minor local structural rearrangements at the mutation sites. Stability estimates using the HoTMuSiC server predicted only relatively small changes of <2
°C in the melting temperature of the DBD for all DBD SNP variants, except for p.G154S and p.P219S, which were predicted to be destabilized by 3–4
°C compared with the wild type (Table S6). This remains significantly below the stability loss observed for the most deleterious structural cancer mutations, such as p.Y220C or the classical temperature-sensitive TP53 variant p.V143A. Interestingly, Tyr107 is conserved in mammalian p53, but a histidine is found in many fish species and in the more thermodynamically stable sister proteins p63/p73. In the structure of the p.Y107H variant, the imidazole side chain of His107 potentially forms a hydrogen bond with the backbone oxygen of Ser149 (Fig. S10a).
The stability predictions were consistent with the observation of full activity at body temperature for all the variants. Although small changes in stability may have no functional impact, they may predispose carriers of the respective SNP to functional inactivation by other mildly-destabilizing mutations that would otherwise be harmless. Comparisons of the predictions with experimentally determined melting temperatures for a panel of cancer hot spot variants showed that they generally agreed well qualitatively, but that there were also notable exceptions. For example, in the case of the thermolabile p.R282W and zinc-binding deficient p.R175H cancer variants, the program failed to predict the drastic stability loss observed experimentally (Table S6). It will thus be of interest to determine the stability of the DBD SNP variants experimentally, to fully assess their impact on cancer risk.
The side chain of Ala189 in the extended L2 loop faces inwards, and the model of the p.A189V variant displayed altered hydrophobic packing against Arg196 and Tyr205 (Fig. S10c). The side chain of Asn235 in beta-strand S8 formed hydrogen bonds with two backbone oxygens (Lys139 and Tyr234) and the carboxylate group of Glu198 (Fig. S10d). This polar interaction network was lost in the p.N235S variant but may have been compensated for, at least in part, by alternative, potentially water-mediated hydrogen bonds formed by the serine hydroxyl. In the mouse structure, there is a lysine at this position, and a serine is found in various fish species.
The other two DBD variants, p.R283C and p.R290H, were located on the C-terminal helix that binds to the major groove of the p53 response elements (Fig. 5B). Arg283 sits close to the protein-DNA interface, but in most p53-DNA complexes, it does not interact directly with the phosphate backbone [25, 26], so no significant effect on DNA-binding affinity and specificity is expected. The closest distance between the guanidinium group and the phosphate backbone of DNA in Protein Data Bank (PDB) entry 3KZA, for example, is more than 6Å. In some fish species and the paralogs p63/p73, a lysine is found at this position. The Arg290 side chain does not interact with the rest of the DBD and is generally disordered in DBD crystal structures, as for example, in PDB entries 2XWR [27] and 3KZ8 [28]. This residue is not conserved across vertebrate p53; a histidine is found at this position in beluga whale and feline p53.
Variant p.T312S was located in the adjacent flexible linker between the DBD and the tetramerization domain. Only one variant, p.E339K, was located in the tetramerization domain itself. Glu339 sits on the surface of the tetramer, facing the solvent, and is not involved in any inter-subunit contacts stabilizing the tetramer (Fig. 5C). Therefore, no major effects on the oligomerization equilibrium of p53 were expected for the lysine variant. This variant is, for example, also found in hamsters.
The remaining three variants affected the C-terminal regulatory region, an intrinsically disordered promiscuous binding module that can adopt different structural motifs, depending on posttranslational modification patterns and binding partners [22, 29]. Interestingly, one of those variants, p.S366A, removed a C-terminal phosphorylation site. Phosphorylation of Ser366 (and other serines in this region) by CHK1 and CHK2 modulates p53 C-terminal acetylation and the transactivation of p53 target genes [30].
ACMG classification of TP53 variants
Variant classification according to guidelines proposed by the American College of Medical Genetics and Genomics (ACMG) and the Association for Molecular Pathology are very useful for clinicians assessing genetic analyses [31]. However, the classification of TP53 variants according to these guidelines remains highly controversial. In the wake of heated debates, several ACMG-derived criteria have been suggested (Table S5) [32, 33]. Furthermore, TP53 variant classification according to the ClinVar platform shows that only a single TP53 variant (p.P72R) has been classified benign with three stars, whereas the entries for other variants have remained controversial (Table S5). This debate is partially due to the fact that the ACMG criteria were initially defined for Mendelian disorders in a specific clinical context. Also, they include many parameters that are not suitable for cancer. Furthermore, the prediction software, as well as population criteria used for an analysis, can influence the various scores. As TP53 variants may be somatic or germline, the ACMG guidelines must be adapted to ensure accurate classification. Allele frequency in the human population is a strong criterion to define a variant as benign. A minor allele frequency (MAF) threshold of 0.001 and minimal allele count (AC) of five in the population are currently used to define the stand-alone BA1 criterion, which is sufficient to classify a variant as benign. In the present study, using the three aggregated datasets, only rs1042522 (p.P72R) could be ranked as BA1 (Table 1 and Fig. 6A). One of the main issues with an aggregated database including multiple ethnic populations is the dilution of the signals for variants specific to an ethnic group. Furthermore, these databases are skewed toward European ancestry (more than 50% for gnomAD), an aspect that can bias analyses. Using the aforementioned criteria, we analyzed the TP53 variants using the eight population subsets from gnomAD and identified only two new BA1 variants, i.e., p.V31I in the Asian population (AF=
0.003 and AC
=
65) and p.Y107H in the African population (AF
=
0.00116 and AC
=
23). The analysis of the 11 national datasets was far more informative, leading finally to the identification of nine TP53 variants that should be defined as benign using ACMG criteria (Tables 1, S5 and Figs. 6A, S11). Among those nine variants were the five EAS-specific SNP variants described above. Similarly, the analysis for the strong BS1 criterion (MAF
≥
0.0003 and AC
≥
5) with the whole-gnomAD database did not uncover new variants, whereas that of the various datasets led to BS1 classification for eight variants (Tables 1, S5 and Figs. 6A, S11). BS1 classification requires a supporting criterion, such as BS3 defined by the loss of activity using “well established in vitro or in vivo assays”. Combining these criteria based on population frequency and multiple functional studies, including the present work, led to the classification of 16 of the set 21 variants as benign (Tables 1, S5 and Figs. 6A, S11). For the remaining six TP53 variants, other ACMG criteria were too heterogeneous for a clear-cut classification. We thus defined them as variants of uncertain significance (VUS).
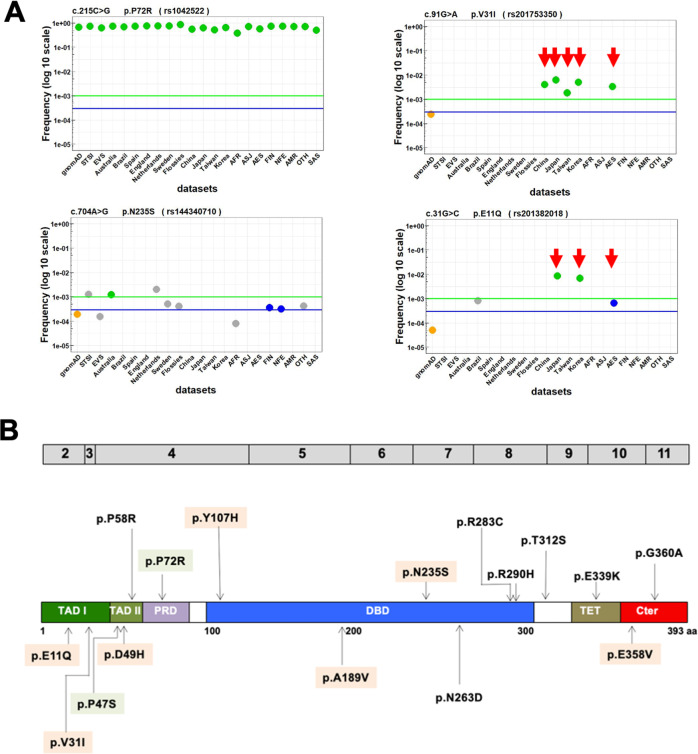
A For the 14 population datasets used in this study as well as for the eight population-specific subsets of gnomAD, the frequency of each TP53 SNP is shown as a colored dot: green: BA1 variants (AF≥
0.001 and AC
≥
5); blue: BS1 variants (AF
≥
0.0003 and AC
≥
5); orange: variants with an AC
≥
5 but falling short of the BA1 or BS1 allele frequency limit of 0.001 (green line) or 0.0003 (blue line); gray: variants with low AF and AC. A specific clustering in the Asian population can be distinguished for variants rs201753350 (p.V31I) and rs201382018 (p.E11Q) (red arrows). An extended analysis for all TP53 variants is shown in Fig. S11. B Distribution of novel benign TP53 variants analyzed in this study in the TP53 protein. Green: benign TP53 variants as defined previously. Orange: benign TP53 variants identified in this study. Variants specific to an ethnic group are shown below the TP53 protein. TAD I transactivation domain I, TAD II transactivation domain II, PRD proline-rich domain, DBD DNA-binding domain, TET oligomerization domain, Cter carboxy-terminal region. Only variants found in four or more different TP53 datasets are shown. TP53 exons 2–11 are shown in gray above the protein.
Discussion
Until recently, human genetic variation studies were largely restricted to constitutional variants occurring at frequencies above roughly 1–5%. That threshold was due to not only technological and cost limitations but also our own lack of knowledge of human genome diversity. Consequently, most medical genetic analyses were developed based on information derived from these common variants. Today, high-throughput DNA sequencing technologies enable the analysis of thousands of samples and the identification of infrequent SNPs. Recent explosive population growth has led to a great number of rare SNPs occurring at frequencies below 0.5% [34, 35]. These low-frequency variants are rich in protein-coding sequences that could predispose to disease. For genes with high clinical impact, it is therefore essential to (i) identify all relevant variants present in the human population and (ii) define their potential pathogenicity.
The TP53 gene is a paradigm for such analyses. Indeed, the accurate diagnosis of somatic mutations in it is mandatory in multiple clinical settings, for example in chronic lymphocytic leukemia, where it is used to improve patient stratification and optimize therapeutic decisions [2]. Furthermore, the diagnosis of germline TP53 mutations for genetic counseling is proving pertinent in such pathologies as hereditary breast and ovarian cancer syndrome and pediatric cancer [1, 36].
Recently, the ACMG—Association for Molecular Pathology provided a systematic method for the interpretation of sequence variants for genetic disorders [31]. Unfortunately, a high fraction of variants is relegated to uncertain significance (VUS), often due to the rarity and conflictual nature of results in existing databases and the primary literature. Several recent publications sought to improve the classification of TP53 variants but their results were heterogeneous and thus a source of discord.
Historically, two exonic missense TP53 SNPs have been identified and defined as benign constitutional variants [37]. Somatic TP53 gene mutations have been described in more than 100,000 patients. However, in most of these cases, matched normal DNA was not available and, thus, the somatic origin of the mutation was determined by consulting reference databases. It is therefore highly likely that some of those variants were infrequent constitutional variants mistakenly identified as somatic mutations.
In the present study, using a combination of in silico, in vitro, structural, and genetic approaches, we addressed a two-tiered question: (i) is it possible to identify novel and infrequent TP53 SNPs in the human population and (ii) what is the potential pathogenicity of these variants?
The use of multiple independent population databases has proven very helpful for the selection of novel potential SNPs. Although gnomAD is an outstanding resource for population analyses, the addition of novel datasets of individuals from different ethnic groups has led to a more accurate selection of potential SNPs [38].
In silico predictive software enables the assessment of the effects of amino acid substitutions on protein structure or function with, in principle, no imperative need for functional studies. However, in silico and functional approaches each have their own strengths and weaknesses. Depending on the algorithm, the performance of in silico approaches will vary based on the specific gene and protein being considered. As all algorithms focus on protein function based on the homology of sequences and/or the physical properties of amino acids, confusion has arisen between deleteriousness and pathogenicity, which should be considered as two different features. Great caution has been advised for the use of these algorithms, including for TP53 [39–41]. Concerning this latter, mutations in the mdm2 binding domain, localized in the amino-terminus of the protein, are predicted to be deleterious and consequently classified as pathogenic. In fact, these variants are known to impair cell growth and ultimately lead to cell death, which is the opposite of what would be expected for cell transformation. This aspect is supported by the total absence of cancer-associated mutation in the two highly conserved codons 19 and 23 of this TP53 protein domain [42].
One major advantage for TP53 is the availability of three unbiased large-scale functional studies for all potent TP53 missense variants [16–18]. A correlation analysis showed excellent agreement between the three datasets despite their different readouts [43]. Although predicted as “nonfunctional” by several algorithms, variants such as p.N235S or p.E11Q do not display loss of activity. This confusion has led to a controversial classification of several TP53 variants. p.N235S has been defined as pathogenic, likely pathogenic or VUS in different studies [32, 44–46]. It remains defined as pathogenic in the COSMIC database (version 92) despite the fact that it is considered as likely benign in Clinvar since spring 2020 and was found to be thus in the present study as well. For TP53, we strongly advocate for the use of functional studies to assess TP53 variant deleteriousness.
The consequences of TP53 mutation on protein function and their repercussions on the various pathways regulated by TP53 are highly heterogeneous. Although a few variants can be considered as amorph with a total loss of function, the complex structure of the TP53 protein is known to give rise to variants with multiple consequences with hypomorph, neomorph or antimorph properties [47–49]. TP53 regulates transcription through specific binding to highly degenerated response elements in promoters or introns of target genes [50]. The affinity of TP53 for these various biological sites is variable, and several TP53 variants can display only partial loss of their DNA-binding activity, allowing the mutant protein to bind only to a subset of TP53 response elements. This feature is linked to differential transactivation activities that may depend on p53 protein levels, as well as target sequences [51, 52]. This heterogeneity for both wild-type and mutant TP53 is reflected by our transcriptional analysis performed on endogenous genes (Fig. S7). Genes activated by wild-type TP53 have been extensively analyzed and scrutinized in normal and tumor cells. However, recent studies have shown that genes repressed by TP53 are also key players in the tumor-suppressive effect of TP53. Within this latter aspect is the downregulation of cell-cycle genes via the p53–WAF1–DREAM–E2F/CHR pathway (p53–DREAM pathway). Upon p53 induction, p21WAF1/CIP1 activates the transcriptional repressor DREAM, leading to the shutdown of more than 200 cell-cycle genes [53, 54]. Derepression of these genes has been shown to be of better prognostic value than TP53 mutations [42]. Whether or not the loss of function of TP53 variants toward the WAF1 promoter and the DREAM pathway is essential for promoting tumorigenicity remains to be elucidated. As of this writing, there is no “gold standard” assay to define a TP53, suppressive effect, but the observation of defective transcription in the large majority of TP53 variants found in human cancer indicates that this activity is a key target. Although none of the variants in the present study displayed any obvious functional defects, we cannot exclude that any one of them may be partially defective for a particular function. Nonetheless, their high frequency in the human population and low frequency in cancer mutation databases suggest that any deleterious clinical impacts will be very low.
Using different functional assays, we showed that a subset of 21 germline TP53 variants found recurrently in multiple population datasets from different ethnic groups was indistinguishable from wild-type TP53. Through the analysis of multiple national datasets, we were able to definitively validate nine of the 21 variants as BA1, i.e., the ACMG stand-alone criterion for benignity. Seven other variants met the ACMG criterion BS1+
BS3, also indicating benignity. Thus, we identified 16 variants that may only be defined as natural polymorphisms. Among those variants, we identified for the first time five variants that were specific to the Asian population (present only at very low frequency in other populations). Our analysis of the UMD_TP53 database confirmed the Asian origin of the five variants and furthermore showed that they were mistakenly defined as somatic pathogenic mutations. We also identified another TP53 variant specific to the Indian population. Our analysis emphasizes the need to exercise great caution when using population datasets to check patient cohorts. Matching the ethnicity of the patient or the cohort to the reference datasets appears essential for increasing the accuracy of the analysis [55]. Also, supplementing these datasets with data from normal populations and from all geographical areas is necessary to minimize variant misclassification. For example, the Asian variant rs201753350 (p.V31I), classified as benign (BA1) in our analysis using multiple criteria, had been classified as pathogenic in a family with gastric cancer in the Japanese population [56]. Such “false positive” results could have detrimental consequences for patients and families, who may end up experiencing anxiety, undergoing unnecessary examinations, etc.
We cannot exclude that a number of the variants described in the present study may be associated with specific hereditary traits associated or not with cancer, or with other genetic characteristics. To illustrate this point, variant rs1042522 (p.P72R) does not impair TP53 activity but whether it is associated with an increased risk of cancer remains highly controversial [23]. Similarly, variant rs1800371 (p.P47S), restricted to the African population, has been shown to be associated with an increased risk of breast cancer [57].
Most of the variants described in set 21 are described as pathogenic or likely pathogenic in multiple databases and pipelines for the assessment of pathogenicity. To improve the accuracy of TP53 mutation diagnostics, they should be updated with the present data. The 2020 release of the UMD_TP53 database as well as the web application Seshat have been updated with these novel data.
This analysis was made possible by the numerous functional and structural analyses available for TP53. Unfortunately, the quantity of research for other cancer genes is much more limited. Nevertheless, the availability of large, independent datasets representing ethnic populations should be sufficient to identify novel benign variants and emphasize genetic heterogeneity. For an accurate assessment of somatic and germline variants, human genome reference databases used in analytical pipelines must include accurate ethnicity information to ensure that genetic analyses provide the most pertinent information possible for patients.
Materials and methods
Details on the cell lines, western blot, luciferase assay, plasmid construction, and flow cytometry analysis for the experimental validation of TP53 variants identified in this study are provided in the Supplementary Information.
Population datasets
TP53 variants from a range of population databases were downloaded from their respective portals (Table S1). The origin of each dataset was carefully checked to avoid redundancies. Because a part of the 1000 Genomes Project was included in gnomAD, the data of the former were not used in the present study. To ensure accurate comparisons between the various datasets, data from all databases were obtained using the genomic coordinates of the entire TP53 gene as defined by RefSeq. In a first step, minimal genomic data, such as genomic coordinates and genetic events, were extracted from each dataset to define the correct annotation according to HGVS recommendations. In a second step, variant annotation was validated by using the Name Checker tool developed by Mutalyzer (https://mutalyzer.nl/). This latter handles all types of TP53 gene variations, such as substitutions, insertions, duplications, deletions, and more complex insertion/deletions [58]. The current version (2.0.26) of Mutalyzer uses the stable NCBI sequence NG_017013.2 as reference for TP53. This is an essential issue for avoiding problems due to the use of multiple genome references (NCBI Build 36.1/hg18, Genome Reference Consortium GRCh37/hg19 or Genome Reference Consortium GRCh38/hg38) by the various NGS pipelines as well as noncompliant nomenclature. To minimize database contamination by potential carriers of germline TP53 variants, the non-cancer version of gnomAD was used in the present analysis. Taken together, exonic TP53 variants issued from gnomAD correspond to 56% of the variants issued from the 14 cohorts.
Cancer-associated TP53 variants were downloaded from the UMD_TP53 database (Released 2017), which includes 80,402 mutations (6870 different TP53 variants) identified in tumors, in cell lines (somatic mutations) or in patients with hereditary cancers (germline mutations) [37]. This release includes mutations identified by conventional sequencing or by NGS (Table S1).
The sequence nomenclature used for TP53 variants in this work is in accordance with the Human Genome Variation Society’s guidelines using the NM_000546.5 transcript sequence and the full-length protein NP_000537.3 [59].
We previously defined and validated a specific set of pathogenic TP53 variants called the “CSD” [15]. Briefly, TP53 variants were extracted from four different nonoverlapping datasets on various types of cancer. The Sanger dataset includes 4299 variants extracted from the latest version of the UMD_TP53 mutation database excluding all NGS studies (Leroy et al. [37]). The other three sets of TP53 variants were compiled from large independent cancer sequencing projects: TCGA [60], ICGC [61], and MSK-IMPACT [62]. Each of these studies described more than 2000 different TP53 variants occurring at various frequencies in the coding regions or splice sites (+2/−2) of TP53. We created the CSD by combining these four datasets to define a core of 471 recurrent TP53 variants found at least once in each database [15]. The four datasets resulted from independent studies using different patients and different methodologies. We showed that shared variants were true recurrent pathogenic variants [15].
Functional analysis from large-scale mutagenesis data
The UMD_TP53 database includes three sets of functional data for TP53 variants. The first set was described in detail in a previous report [16, 63]. Briefly, haploid yeast transformants containing 2314 TP53 variants and a green fluorescent protein reporter plasmid were constructed. TP53 activity was tested by measuring the fluorescent intensity of green fluorescent protein that is controlled by eight different promoter sequences regulated by the p53 protein after 3 days of growth at 37°C. The second set of functional data integrated in the UMD_TP53 database corresponds to the analysis of 5300 TP53 variants performed by Kotler et al. [17]. The growth activity of all variants localized in the DBD of TP53 was assessed in H1299 cells. The third set corresponds to the study by Giacomelli et al. [18]. In this study, dominant negative activities, losses of function, and responses to etoposide were analyzed in mammalian cells for 8258 TP53 variants (Table S4). Functional scores were extracted from these 12 datasets associated with the three studies and normalized for all the analyses of TP53 variants.
Selection of TP53 variants for in silico and in vitro analysis
A subset of 27 TP53 variants (set 27) were chosen for in silico analyses based on their occurrence in multiple datasets (more than 2). We also added the five Asian-specific variants and eight of the ten outlier germline variants found in the UMD_TP53 database. The variant p.T125R, found at high frequency as a germline variant in the database, was excluded because missense and synonymous mutations in this codon close to a splice site are known to impair TP53 splicing [64, 65]. The pathogenic variant p.R337H (known as the Brazilian mutation) associated with adrenocortical carcinoma was also excluded [66].
Set 21, used for in vitro analyses, is derived from set 27 using more stringent criteria. It includes variants found in multiple datasets (more than 4) at frequencies higher than 0.0005. Asian and outlier germline variants were retained but variants p.R248Q, p.R283H, and p.R156H found, respectively, in five, four, and four datasets were not included as they are well-known pathogenic variants included in the CSD. Set 21 thus included 19 novel TP53 variants as well as rs1042522 (p.P72R) and rs1800371 (p.P47S).
Molecular modeling and stability predictions
Models of p53 DBD mutants were generated using the SWISS-MODEL server [67], with PDB entry 2XWR as a template [27]. Differences in the melting temperatures of the mutant proteins compared with the wild-type protein were predicted using the HoTMuSiC server [68] based on PDB entry 2XWR and a wild-type DBD melting temperature of 46°C.
Variant effect prediction
dbNSFP v3.5 was downloaded from the Jpopgen website (https://sites.google.com/site/jpopgen/dbNSFP). This database compiles prediction or conservation scores from multiple prediction algorithms [12]. Prediction scores from each algorithm were available as normalized data from 0 (less deleterious) to 1 (most deleterious).
Five other predictors not included in dbNSFP were also used: Revel [69], PON-P2 [70], MutPred2 [71], Mut_Pred [72], and Envision [73]. Brief descriptions of these predictors are provided in Table S4.
Literature survey and ethnicity analysis
The 2017 release of the UMD_TP53 database includes data from 4600 publications. A manual survey of the database was performed for the five TP53 variants suspected to be constitutional variants specific to the Asian population. Publications describing any one of these variants were retrieved for analysis. In most cases, the hospitals and/or institutions used for patient recruitment were available.
Supplementary information
Acknowledgements
This study was supported by the Radiumhemmets Forskningsfonder (to TS), the Czech Science Foundation (GA19-15737S) (to SP), and the German Research Foundation (DFG) grant JO 1473/1-1 (to ACJ). We would also like to thank the core facility at Novum, BEA, Bioinformatics and Expression Analysis, which is supported by the board of research at the Karolinska Institute and the research committee at the Karolinska hospital. We thank Vladimir Bykov (Bioclinicum, Karolinska Institute) for help with the FACS analysis, Patrick Muller (BEA, Karolinska Institute) for help in QPCR analyses, and Alan Sauvat (INSERM U1138, Centre de Recherche des Cordeliers) for data analyses.
Author contributions
KGW, GK, and TS designed research. FD, MT, JS, and IM performed research; JSMM, PM, SP, and SP performed clinical analyses. VC and BL contributed new reagents/analytic tools. ACJ performed structural analyses; FD and TS analyzed data. TS wrote the paper with input from all authors.
Compliance with ethical standards
KGW is a cofounder and a shareholder of Aprea Therapeutics AB, a company that develops p53-based cancer therapies including APR-246. KGW is a member of its Clinical Advisory Board and has received salary and research funding from Aprea Therapeutics AB.
Footnotes
Edited by G. Melino
Publisher’s note Springer Nature remains neutral with regard to jurisdictional claims in published maps and institutional affiliations.
Supplementary information
The online version of this article (10.1038/s41418-020-00672-0) contains supplementary material, which is available to authorized users.
References
Articles from Cell Death and Differentiation are provided here courtesy of Nature Publishing Group
Full text links
Read article at publisher's site: https://doi.org/10.1038/s41418-020-00672-0
Read article for free, from open access legal sources, via Unpaywall:
https://www.nature.com/articles/s41418-020-00672-0.pdf
HAL Open Archive
https://hal.sorbonne-universite.fr/hal-03095188
Citations & impact
Impact metrics
Citations of article over time
Alternative metrics
Smart citations by scite.ai
Explore citation contexts and check if this article has been
supported or disputed.
https://scite.ai/reports/10.1038/s41418-020-00672-0
Article citations
Functional evaluation of germline TP53 variants identified in Brazilian families at-risk for Li-Fraumeni syndrome.
Sci Rep, 14(1):17187, 26 Jul 2024
Cited by: 0 articles | PMID: 39060302 | PMCID: PMC11282216
Structural basis of p53 inactivation by cavity-creating cancer mutations and its implications for the development of mutant p53 reactivators.
Cell Death Dis, 15(6):408, 11 Jun 2024
Cited by: 1 article | PMID: 38862470
Emerging insights into ethnic-specific TP53 germline variants.
J Natl Cancer Inst, 115(10):1145-1156, 01 Oct 2023
Cited by: 3 articles | PMID: 37352403 | PMCID: PMC10560603
Review Free full text in Europe PMC
COSMIC-based mutation database enhances identification efficiency of HLA-I immunopeptidome.
J Transl Med, 22(1):144, 10 Feb 2024
Cited by: 1 article | PMID: 38336780 | PMCID: PMC10858511
Prolidase-proline oxidase axis is engaged in apoptosis induction by birch buds flavonol santin in endometrial adenocarcinoma cell line.
Front Mol Biosci, 10:1247536, 06 Sep 2023
Cited by: 1 article | PMID: 37745688 | PMCID: PMC10512030
Go to all (19) article citations
Data
Data behind the article
This data has been text mined from the article, or deposited into data resources.
BioStudies: supplemental material and supporting data
Protein structures in PDBe (5)
-
(3 citations)
PDBe - 2XWRView structure
-
(2 citations)
PDBe - 3KZ8View structure
-
(1 citation)
PDBe - 1C26View structure
-
(1 citation)
PDBe - 3KZAView structure
-
(1 citation)
PDBe - 2L14View structure
RefSeq - NCBI Reference Sequence Database (2)
- (1 citation) RefSeq - NM_000546.5
- (1 citation) RefSeq - NG_017013.2
SNPs (Showing 9 of 9)
- (5 citations) dbSNP - rs1042522
- (5 citations) dbSNP - rs1800371
- (3 citations) dbSNP - rs201753350
- (2 citations) dbSNP - rs201382018
- (1 citation) dbSNP - rs72661119
- (1 citation) dbSNP - rs773553186
- (1 citation) dbSNP - rs138729528
- (1 citation) dbSNP - rs587780728
- (1 citation) dbSNP - rs121912665
Show less
Similar Articles
To arrive at the top five similar articles we use a word-weighted algorithm to compare words from the Title and Abstract of each citation.
Benign SNPs in the Coding Region of TP53: Finding the Needles in a Haystack of Pathogenic Variants.
Cancer Res, 82(19):3420-3431, 01 Oct 2022
Cited by: 2 articles | PMID: 35802772
Review
An updated quantitative model to classify missense variants in the TP53 gene: A novel multifactorial strategy.
Hum Mutat, 42(10):1351-1361, 04 Aug 2021
Cited by: 6 articles | PMID: 34273903
A quantitative model to predict pathogenicity of missense variants in the TP53 gene.
Hum Mutat, 40(6):788-800, 18 Mar 2019
Cited by: 15 articles | PMID: 30840781
Comprehensive Identification of Deleterious TP53 Missense VUS Variants Based on Their Impact on TP53 Structural Stability.
Int J Mol Sci, 22(21):11345, 20 Oct 2021
Cited by: 5 articles | PMID: 34768775 | PMCID: PMC8583684
Funding
Funders who supported this work.
Radiumhemmets Forskningsfonder (1)
Grant ID: -
Radiumhemmets Forskningsfonder (Cancer Research Foundations of Radiumhemmet) (1)
Grant ID: -