Abstract
Free full text

Link between glucose metabolism and epithelial-to-mesenchymal transition drives triple-negative breast cancer migratory heterogeneity
Summary
Intracellular and environmental cues result in heterogeneous cancer cell populations with different metabolic and migratory behaviors. Although glucose metabolism and epithelial-to-mesenchymal transition have previously been linked, we aim to understand how this relationship fuels cancer cell migration. We show that while glycolysis drives single-cell migration in confining microtracks, fast and slow cells display different migratory sensitivities to glycolysis and oxidative phosphorylation inhibition. Phenotypic sorting of highly and weakly migratory subpopulations (MDA+, MDA−) reveals that more mesenchymal, highly migratory MDA+ preferentially use glycolysis while more epithelial, weakly migratory MDA− utilize mitochondrial respiration. These phenotypes are plastic and MDA+ can be made less glycolytic, mesenchymal, and migratory and MDA− can be made more glycolytic, mesenchymal, and migratory via modulation of glucose metabolism or EMT. These findings reveal an intrinsic link between EMT and glucose metabolism that controls migration. Identifying mechanisms fueling phenotypic heterogeneity is essential to develop targeted metastatic therapeutics.
Introduction
Intratumor heterogeneity is an emerging hallmark of cancer that complicates clinical diagnostics and therapeutics (Fisher et al., 2013; Hapach et al., 2021; Sun and Yu, 2015). As cancer cells have uniquely high proliferation rates, cancer metabolism research has historically focused on identifying aerobic glycolysis as the driving force behind this proliferation (Lunt and Vander Heiden, 2011; Vander Heiden et al., 2009; Vaupel et al., 2019; Warburg, 1925, p. 19; Ward and Thompson, 2012). Recent work has identified heterogeneity in breast cancer metabolic state in both primary tumors and metastatic sites, with increased glycolysis in primary tumors and increased mitochondrial oxidative phosphorylation at metastatic sites (Davis et al., 2020). Therefore, the energy produced via glycolysis and oxidative phosphorylation may fuel different cell behaviors with different metastatic advantages.
One of the first steps of breast cancer metastasis is invasion through the basement membrane and migration through the extracellular matrix (Engbring and Kleinman, 2003; Hapach et al., 2019; Lambert et al., 2017); therefore, migratory ability is often considered an indicator of metastatic success (Fares et al., 2020; Liu et al., 2020; Palmer et al., 2011; van Zijl et al., 2011). To complete this first step and escape the primary tumor, cancer cells can reprogram their metabolism to fuel migration through a mechanically and chemically complex tumor microenvironment (Mosier et al., 2021). It has been suggested that cancer cells undergo epithelial-to-mesenchymal transition (EMT) to promote invasion and migration through the tumor microenvironment (Banyard and Bielenberg, 2015; Polireddy et al., 2016; Son and Moon, 2010; Wang et al., 2016). Cancer cell EMT phenotype and metabolic state appear to exist in a dynamic, reciprocal relationship. Metabolic dysregulation and glycolytic enzymes can induce EMT (Ahmad et al., 2011; Chen et al., 2018; Funasaka et al., 2009; Kang et al., 2019; Liu et al., 2017). Alternatively, drivers of EMT can induce metabolic reprogramming and upregulate glycolysis in transitioning cancer cells (Han et al., 2018; Kang et al., 2019; Kim et al., 2017; Masin et al., 2014; Sciacovelli and Frezza, 2017; Sun and Yang, 2020; Zhang et al., 2018). Although it is known that cancer cell bioenergetics and EMT are intrinsically linked, the consequences of this relationship on intratumor migratory heterogeneity are unclear.
Here, we observe significant heterogeneity in the metabolic signatures and EMT phenotype of migrating breast cancer cells. Cell migration in the tumor microenvironment was modeled using microfabricated 3D microtracks which recapitulate matrix confinement encountered in vivo (Carey et al., 2015; Kraning-Rush et al., 2013). Although MDA-MB-231 breast cancer cells primarily utilize glycolysis to fuel their migration in confinement, single-cell analysis of MDA-MB-231 revealed that while highly migratory cells were sensitive to the inhibition of glycolysis and unaffected by the inhibition of oxidative phosphorylation, inhibition of oxidative phosphorylation increased weakly migratory cancer cell migration. To further investigate this migratory heterogeneity, MDA-MB-231 breast cancer cells were phenotypically sorted to obtain highly and weakly migratory subpopulations of MDA-MB-231 that were then thoroughly characterized. We show that highly migratory populations (MDA+) exhibit increased mesenchymal features and increased glycolytic genes, while weakly migratory populations (MDA−) are more epithelial and are more associated with oxidative phosphorylation. Further exploration of this characterization revealed that migratory ability in confinement can be controlled by inducing EMT or modulating metabolic pathways utilized by cells. Thus, our findings reveal that a dynamic, complementary relationship between glucose metabolism and EMT phenotype drives heterogeneous migratory phenotypes in triple-negative breast cancer.
Results
Glycolysis fuels MDA-MB-231 breast cancer cell migration in confined environments
Both glycolysis and oxidative phosphorylation have been implicated in single-cell migration in cancer (Cunniff et al., 2016; Kondo et al., 2021; LeBleu et al., 2014; Thejer et al., 2020). However, microenvironmental mechanical cues such as confinement are known to regulate cancer cell migration and bioenergetics (Mosier et al., 2019; Zanotelli et al., 2019). To determine the mode of glucose metabolism that drives breast cancer migration in confined spaces, MDA-MB-231 breast cancer cell (MDAPAR) migration was analyzed with inhibitors of glycolysis or mitochondrial respiration in engineering, in vitro collagen microtracks which mimic the natural tracks and pores found in the tumor microenvironment, allowing accurate quantification of 3D, directional migration (Carey et al., 2015; Kraning-Rush et al., 2013). To modulate breast cancer glucose metabolism, MDAPAR were treated with either iodoacetate (IA), an inhibitor of GAPDH in glycolysis, or antimycin-A (AMA), an inhibitor of complex III of the electron transport chain in the mitochondria. Dose curves analyzing the effect of 10, 100, or 500 μM IA on glucose uptake, measured with 2-NBDG, or AMA on mitochondrial membrane potential, measured with TMRM, were completed to determine the concentration of each inhibitor that would result in a change in metabolism in MDAPAR in 3D collagen gels (Figures 1A and 1B). Additionally, LIVE/DEAD assays (Thermofisher) were completed for the same concentrations to ensure viability was not disrupted by the inhibitors (Figure 1C). Although significant changes in glucose uptake were observed in all doses of IA, significant death was observed in the 100 and 500 μM concentrations of IA. In contrast, even at 500 μM, no significant death was observed owing to AMA treatment in 3D, while significant changes in mitochondrial membrane potential, measured using TMRM, were only observed at the highest dose. For all future experiments, 500 μM AMA and 10 μM IA were used. MDAPAR treated with IA were additionally supplemented with 100 μM sodium pyruvate (PYR) to reduce toxicity from glycolysis inhibition and permit continued mitochondrial respiration (Dad et al., 2013; Lam et al., 2016).
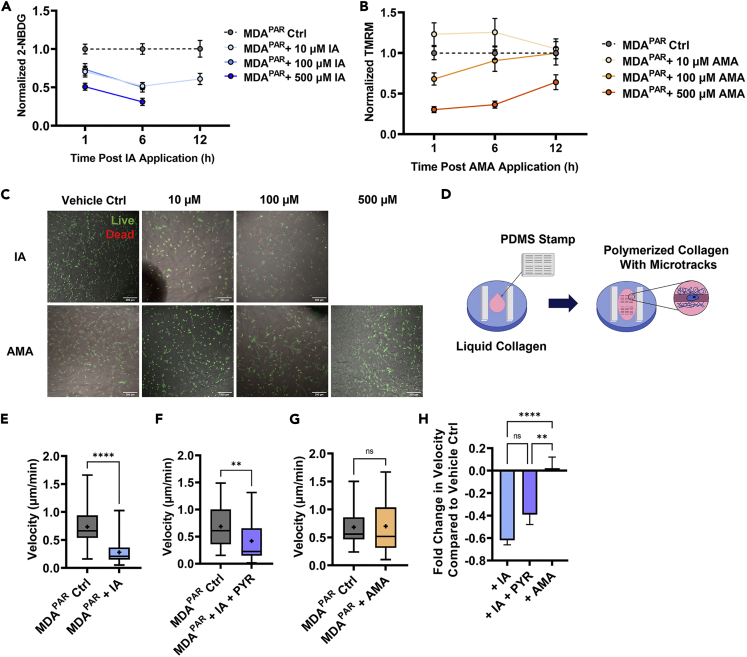
Glycolysis fuels MDA-MB-231 migration in confinement
(A) Dose curve of 2-NBDG fluorescence corrected for the background of MDAPAR treated with 10, 100, or 500 μM IA or vehicle control.
(B) dose curve of TMRM fluorescence corrected for the background of MDAPAR treated with 10, 100, or 500 μM AMA or vehicle control.
(C) representative images of MDAPAR viability after treatment with 10 or 100 μM IA or vehicle control, or 10, 100, or 500 μM AMA or vehicle control with live cells in green and dead cells in red.
(D) schematic of microtrack micromolding. PDMS stamp used to mold liquid drop of collagen in between plastic spacers in a 6 well plate; Average MDAPAR microtrack velocity 6-12 h after.
(E) vehicle control (diH2O) (Ctrl) or 10 μM IA application (+IA) (n = 42-46).
(F) vehicle control (diH2O) or 10 μM IA + 100 μM PYR application (+ IA + PYR) (n = 35-36).
(G) vehicle control (DMSO) or 500 μM AMA application (+AMA) (n = 37-45).
(H) fold change in velocity compared to vehicle control from (E–G) (n = 36-46); denotes p < 0.01,
denotes p < 0.0001, ns = not significant. Mean +SEM is shown for bar graphs and min to max for box-and-whisker plots with mean represented as “+.”
To measure migration, microtracks were fabricated using custom-made PDMS template stamps on liquid collagen. After collagen polymerization, microtracks were seeded with low densities of cells to permit single-cell time-lapse imaging (Figure 1D). MDAPAR treated with IA and IA + PYR (MDAPAR + IA, MDAPAR + IA + PYR) displayed significantly reduced migration velocity 6-12 h after drug application compared to vehicle control conditions (MDAPAR Ctrl) (Figures 1E and 1F). MDAPAR treated with AMA (MDAPAR + AMA) displayed no change in velocity 6-12 h after drug application compared to vehicle control conditions (Figure 1G). To directly compare the response to different inhibitors with different vehicle controls, fold change in velocity was calculated. MDAPAR treated with IA and IA + PYR displayed significantly decreased fold changes in velocity compared to cells treated with AMA (Figure 1H). These data suggest that MDAPAR migration in confinement is primarily fueled by glycolysis compared to mitochondrial respiration.
MDA-MB-231 breast cancer cells exhibit heterogeneous migration in response to glucose metabolism inhibitors
Given that MDAPAR breast cancer cells are highly heterogenous and display different morphologies, migration potentials, and EMT phenotypes (Hapach et al., 2021; Shah et al., 2017; Shen et al., 2020; Zhang et al., 2021), we investigated the distribution of MDAPAR velocity in response to glucose metabolism inhibitors. MDAPAR migration without glucose metabolism inhibition (MDAPAR Ctrl) was largely heterogeneous with cells displaying a wide range of velocities in confinement (Figures 2A–2C). MDAPAR migration in response to IA was largely homogeneous, with a shift towards decreased velocities evident in the velocity histogram (Figure 2A). Migration response to IA + PYR also resulted in a shift in the velocity histogram towards decreased velocities (Figure 2B). MDAPAR migration in response to AMA treatment was more heterogeneous, with certain cells exhibiting decreased migration velocities and others exhibiting increased migration velocities compared to MDAPAR Ctrl (Figure 2C).
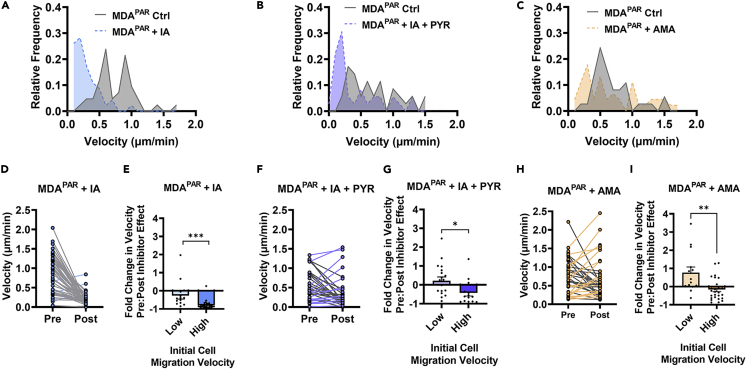
MDA-MB-231 exhibit heterogeneous migration in response to glucose metabolism inhibitors in confinement
(A) Average microtrack velocity histogram of MDAPAR 6-12 h after vehicle control (Ctrl) or IA application (+IA) (N = 3, n = 42-46).
(B) average microtrack velocity histogram of MDAPAR 6-12 h after vehicle control (Ctrl) or IA + PYR application (+ IA + PYR) (N = 3, n = 35-36).
(C) average microtrack velocity histogram of MDAPAR 6-12 h after vehicle control (Ctrl) or AMA application (+AMA) (N = 3, n = 37-45).
(D) pre-treatment effect (1-2 h) and post-treatment effect (11-12 h) average microtrack velocity after IA application (N = 3, n = 46). Gray lines = decreasing velocity. Blue lines = increasing velocity.
(E) fold change in velocity shown in (D) for cells with low migration velocities (velocity<0.5 μm/min) and high migration velocities (velocity >0.5 μm/min).
(F) pre-treatment effect (1-2 h) and post-treatment effect (11-12 h) average microtrack velocity after IA + PYR application (N = 3, n = 35). Gray lines = decreasing velocity. Purple lines = increasing velocity.
(G) fold change in microtrack velocity shows in (F) for cells with low migration velocities (velocity<0.5 μm/min) and high migration velocities (velocity >0.5 μm/min).
(H) pre-treatment effect (1-2 h) and post-treatment effect (11-12 h) average microtrack velocity after AMA application (N = 3, n = 44). Gray lines = decreasing velocity. Orange lines = increasing velocity.
(I) fold change in microtrack velocity shown in (H) for cells with low migration velocities (velocity<0.5 μm/min) and high migration velocities (velocity >0.5 μm/min); denotes p < 0.05,
denotes p < 0.01,
denotes p < 0.001. Mean +SEM with dot plot shown for bar graphs.
Given that cells within MDAPAR exhibited different velocities in response to glucose metabolism inhibition, we investigated whether cells with low or high migration velocities responded differently to the inhibition of glycolysis or oxidative phosphorylation. Cells with migration velocities greater than 0.5 μm/min were considered to have high migration velocities, and cells with migration velocities less than 0.5 μm/min were considered to have low migration velocities. As inhibitors of glucose metabolism had no effect on cell velocity until 2 h post drug application (data not shown), we analyzed the effects of glucose inhibitors on individual cells by comparing velocity pre-inhibitor effect (1-2h after inhibitor application, Pre) to post-inhibitor effect (11-12h after inhibitor application, Post). MDAPAR response to IA was largely homogenous, with cells displaying greatly decreased velocity post-inhibitor effect (Figure 2D). Interestingly, cells with initially high migration velocities had a significantly larger fold decrease in velocity compared to cells with initially low migration velocities, suggesting that IA decreased the velocity of highly migratory cells to a greater extent (Figure 2E). MDAPAR response to IA + PYR was more heterogeneous, with cells with initially low migration velocities displaying very little change in velocity while cells with initially high migration velocities displayed significantly decreased velocity (Figures 2F and 2G). MDAPAR treated with AMA exhibited highly heterogeneous responses in velocity. Although MDAPAR with initially high migration velocities displayed a slight decrease in velocity, cells with initially low migration velocities displayed a fold increase in velocity after AMA treatment (Figures 2H and 2I). These results reveal that the migration of highly and weakly migratory cells within the heterogeneous MDAPAR cell line varies in their relationship to different routes of glucose metabolism. These findings suggest that metabolic heterogeneity within MDAPAR may contribute to migratory heterogeneity.
Phenotypically sorted weakly and highly migratory subpopulations of MDAPAR preferentially utilize different pathways for glucose metabolism
Given that MDAPAR of low and high migration velocities displayed heterogeneous responses to glycolysis and oxidative phosphorylation inhibition, we sought to determine whether weakly or highly migratory cancer cells preferentially utilize different metabolic pathways to fuel migratory heterogeneity. MDAPAR were phenotypically sorted based on their ability to migrate through a collagen coated Transwell (Figure 3A). Cells that migrated through the Transwell were purified over 20 rounds of sorting and termed highly migratory or MDA+. Cells that never migrated through the Transwell were purified over 20 subsequent rounds of sorting and termed weakly migratory, or MDA−. Consistent with previous characterization (Hapach et al., 2021), MDA+ exhibited a higher motile fraction in 3D collagen gels compared to MDA− (Figure 3B).
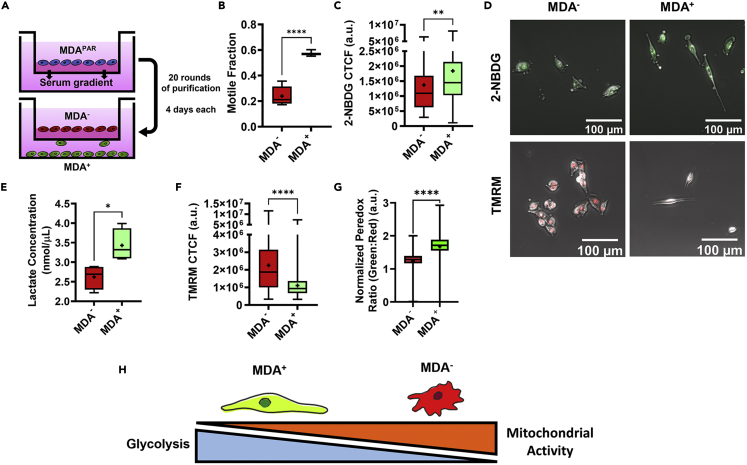
Weakly and highly migratory MDA-MB-231 subpopulations utilize different pathways for glucose metabolism
(A) Schematic of phenotypic sorting.
(B) motile fraction of weakly and highly migratory cells (N = 3).
(C) 2-NBDG of MDA− and MDA+ (N = 3, n = 106-142).
(D) representative images of 2-NBDG (green) and TMRM (red) in MDA− and MDA+.
(E) lactate concentration of MDA− and MDA+ (N = 3).
(F) TMRM of MDA+ and MDA− (N = 3, n = 157-160).
(G) normalized Peredox signal (green:red ratio) of MDA+ and MDA− (n > 350).
(H) Schematic of metabolic differences between MDA− and MDA+. denotes p < 0.05,
denotes p < 0.01,
denotes p < 0.0001. Min to max shown for box-and-whisker plots with mean represented as “+.”
Given that MDA+ and MDA− vary in migratory capability and that migration after treatment with glucose metabolism inhibitors differed between MDAPAR of low and high migration velocities, we investigated the metabolic behaviors of phenotypically sorted MDA+ and MDA−. MDA+ displayed increased glucose uptake (Figures 3C and 3D), quantified via 2-NBDG uptake, increased lactate output (Figure 3E), and decreased mitochondrial membrane potential (Figures 3D and 3F), quantified via TMRM, compared to MDA−. To further probe the metabolic differences between highly and weakly migratory cells, cells were stably transduced with the ratiometric Peredox sensor and calibrated as previously described to assess cytoplasmic NADH to NAD + as a metric for glycolysis (Hung et al., 2011; Hung and Yellen, 2014). MDA+ exhibited higher Peredox signal (Figure 3G), indicating that highly migratory MDA+ are more glycolytically active than the weakly migratory MDA−. In contrast, high mitochondrial membrane potential and low NADH:NAD+ and 2-NBDG uptake in MDA− indicated that weakly migratory cells are more mitochondrially active compared to MDA+ (Figure 3H).
Modulation of epithelial-to-mesenchymal transition phenotype regulates glucose metabolism and drives migration
Given the known relationship between EMT and glucose metabolism (Ahmad et al., 2011; Chen et al., 2018; Funasaka et al., 2009; Han et al., 2018; Kang et al., 2019; Kim et al., 2017; Liu et al., 2017; Masin et al., 2014; Sciacovelli and Frezza, 2017; Zhang et al., 2018), we sought to investigate the relationship between cancer cell bioenergetics, EMT phenotype, and migratory ability between phenotypically sorted triple-negative breast cancer cells. In addition to MDA-MB-231, human triple-negative SUM159 breast cancer cells were phenotypically sorted into highly migratory SUM+ and weakly migratory SUM− subpopulations. MDA+, MDA−, SUM+, and SUM− RNA sequencing were scored based on bioenergetics and EMT status. EMT Score was calculated as (Mesenchymal Score/Epithelial Score). Energy Score was calculated as (Glycolysis Score/Oxidative Phosphorylation Score). Both MDA+ and SUM+ had higher EMT Scores (more mesenchymal) and higher Energy Scores (more glycolytic) than their weakly migratory counterpart (Figure 4A), revealing that phenotypic sorting of triple-negative breast cancer cells isolates EMT and metabolically distinct subpopulations of cancer cells. For the cell types tested, highly migratory cells are more mesenchymal with higher rates of glucose uptake than weakly migratory cells. Taken together, these findings reveal that migratory heterogeneity within triple-negative breast cancer cell lines may be driven by the link between glucose metabolism and EMT status.
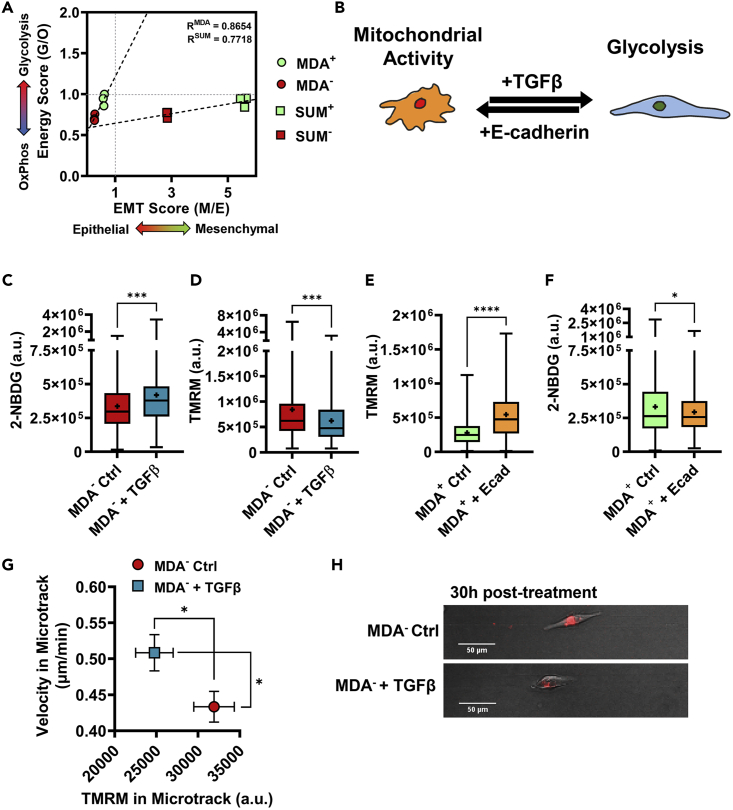
Modulation of EMT phenotype regulates glucose metabolism and drives migration
(A) Energy (glycolysis/mitochondrial) vs EMT (mesenchymal/epithelial) scores for highly and weakly migratory subpopulations for MDA-MB-231 cells (MDA+, MDA−) and SUM159 cells (SUM+, SUM−) as quantified using RNA sequencing, fit with linear regression. R values are shown. (N = 3).
(B) schematic of EMT regulation using E-cadherin and TGFβ.
(C) 2-NBDG of MDA− and MDA− treated with 5 ng/mL TGFβ (N = 3, n = 191-239).
(D) TMRM signal in MDA− and MDA− treated with 5 ng/mL TGFβ (N = 3, n = 236-258).
(E) TMRM of MDA+ and MDA+ with E-cadherin overexpression (N = 3, n = 92-118).
(F) 2-NBDG of MDA+ and MDA+ with E-cadherin overexpression (N = 3, n = 289-320).
(G) velocity and TMRM of MDA− and MDA− treated with 50 ng/mL TGFβ in microtracks (N = 3, n = 59-78).
(H) representative images of cells labeled with TMRM in microtracks; denotes p < 0.05,
denotes p < 0.001,
denotes p < 0.0001. Mean +SEM is shown for (G) and min to max for box-and-whisker plots with mean represented as “+.”
To further determine how the link between metabolism and EMT can drive migratory heterogeneity, we investigated whether the modulation of EMT phenotype conversely alters glucose metabolism and migration capability. To induce EMT of the more epithelial MDA−, MDA− were treated with TGFβ for 24 h (Figure 4B). After treatment, MDA− exhibited increased glucose uptake (Figure 4C), quantified with 2-NBDG, and decreased mitochondrial membrane potential (Figure 4D), measured with TMRM. These data are consistent with previous findings that cells undergoing EMT undergo metabolic reprogramming to increase glycolysis (Kang et al., 2019; Kim et al., 2017; Masin et al., 2014; Sciacovelli and Frezza, 2017; Sun and Yang, 2020; Zhang et al., 2018). Conversely, MET of the more mesenchymal MDA+ was simulated by overexpression of E-cadherin using shRNA (Figure 4B). E-cadherin expressing MDA+ demonstrated increased mitochondrial membrane potential (Figure 4E) and decreased glucose uptake (Figure 4F), consistent with MET inducing mitochondrial activity (Ahmad et al., 2011; Funasaka et al., 2009; Han et al., 2018; Kang et al., 2019).
As EMT and MET are linked to glucose metabolism utilization, we investigated whether these altered bioenergetics regulate downstream migration (Jia et al., 2021; Kondaveeti et al., 2015; Nilchian et al., 2020). We previously showed that E-cadherin-knock-in MDA+ exhibits reduced cell migration velocities in confined microtracks compared to MDA+ control cells (Hapach et al., 2021). Those findings, in addition to those presented here, correlate increased mitochondrial activity with decreased migratory ability in weakly migratory cells. Conversely, treatment with MDA− with TGFβ, known to induce a complete EMT phenotype (Aiello et al., 2018), increased MDA− migration velocities and decreased mitochondrial membrane potential relative to MDA− control cells (Figures 4G and 4H). Although it is widely known that the EMT phenotype correlates with migratory ability (Aiello et al., 2018; Hapach et al., 2021), these findings suggest that EMT-induced metabolic reprogramming may fuel enhanced migration.
Modulation of glycolysis and oxidative phosphorylation shift migration phenotypes of phenotypically sorted weakly and highly migratory subpopulations
Given that weakly and highly migratory subpopulations of MDAPAR have different glycolytic and mitochondrial activities (Figure 3) and given that changes in EMT induce metabolic reprogramming and drive migration (Figure 4), we investigated whether the modulation of glycolysis and oxidative phosphorylation would be sufficient to alter the migration phenotypes of highly and weakly migratory breast cancer cells. As MDA+ has higher rates of glucose uptake than MDA−, we first investigated whether inhibiting glycolysis was sufficient to block single-cell migration in confinement. MDA− treated with IA + PYR displayed reduced migration velocity compared to control conditions (Figures 5A and 5D). Similarly, MDA+ treated with IA + PYR displayed significantly reduced migration velocity compared to vehicle control conditions (Figures 5B and 5D), revealing that glycolysis contributes to the migration of both weakly and highly migratory cancer cells. IA + PYR had a significantly greater effect on the migration of MDA+ compared to MDA− (Figure 5C), highlighting that glycolysis is utilized to different extents by weakly and highly migratory cells for migration in confinement.
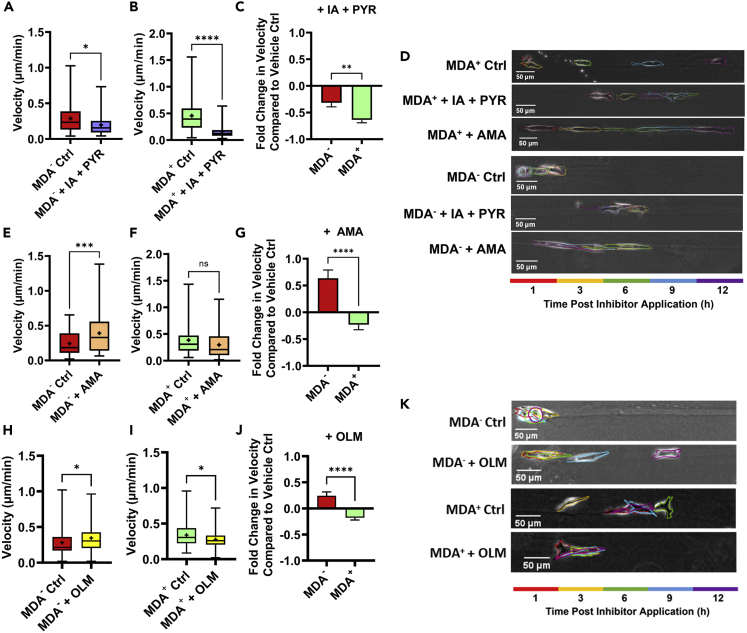
Modulation of glucose metabolism changes migration phenotypes of highly and weakly migratory subpopulations
(A) average velocity of MDA− 6-12 h after 10 μM IA + 100 μM PYR or vehicle control application (N = 3+, n = 46-51).
(B) average velocity of MDA+ 6-12 h after 10 μM IA + 100 μM PYR or vehicle control application (N = 3+, n = 34-36).
(C) fold change in velocity between treated and control conditions for MDA+ and MDA− (A and B).
(D) representative images of cell migration in microtracks from 1 to 12 h after vehicle control or inhibitor application.
(E) average velocity of MDA− 6-12 h after 500 μM AMA or vehicle control application (N = 3+, n = 57-69).
(F) average velocity of MDA+ 6-12 h after 500 μM AMA or vehicle control application (N = 3+, n = 48-57).
(G) fold change in velocity between treated and control conditions for MDA+ and MDA− (E and F).
(H) average velocity of MDA− 6-12 h after 100 μM OLM or vehicle control application (N = 3. n = 79-88).
(I) average velocity of MDA+ 6-12 h after 100 μM OLM or vehicle control application (N = 3. n = 66-87).
(J) fold change in velocity between treated and control conditions for MDA+ and MDA− (H and I).
(K) representative images of cell migration in microtracks from 1 to 12 h after vehicle control or inhibitor application. denotes p < 0.05,
denotes p < 0.01,
denotes p < 0.001,
denotes p < 0.0001. Mean +SEM is shown for bar graphs and min to max for box-and-whisker plots with mean represented as “+.”
Given that migration is primarily thought to be glycolysis driven (Shiraishi et al., 2015) and disruption of mitochondrial energy production can induce cell migration (Han et al., 2018), we next investigated whether the inhibition of mitochondrial energy production would increase cancer cell migration. MDA− treated with AMA displayed a robust increase in migration velocity (Figures 5D and 5E), revealing that the inhibition of oxidative phosphorylation was sufficient to increase weakly migration cancer cell migration. Conversely, MDA+ treated with AMA displayed no significant change in migration velocity (Figures 5D and 5F), revealing that the inhibition of oxidative phosphorylation does not increase the migration of highly migratory cancer cells. Importantly, AMA had a significantly different effect on the migration of MDA− compared to MDA+ revealing that the inhibition of oxidative phosphorylation energy production in weakly migratory cells that preferentially utilize mitochondrial energy can increase cell migration velocity (Figure 3G). As further confirmation that the disruption of mitochondrial energy production has differential effects in each subpopulation, we also tested the effects of oligomycin (OLM), an inhibitor of ATP synthase. Similar to AMA treatment in weakly migratory cells, OLM resulted in an increase in microtrack velocity (Figure 5H). Treatment with OLM in highly migratory cells significantly decreased velocity (Figure 5I). Treatment with OLM had a significantly different effect on the migration of MDA+ compared to MDA− (Figures 5J and 5K), with MDA− increasing velocity upon treatment and MDA+ decreasing speed. These findings further confirm the differential effects of disrupting mitochondrial energy on highly and weakly migratory populations.
Treatment with mitochondrial inhibitors can lead to many off-target effects, including increased production of reactive oxygen species (ROS) in MDA-MB-231 cells (Sarmiento-Salinas et al., 2019). Furthermore, increased ROS has been reported to contribute to both EMT and cancer cell migration (Aggarwal et al., 2019; Kumari et al., 2018). To determine whether ROS production increases in response to AMA and OLM, carboxy-H2DCFDA was used to fluorescently label ROS in MDA+ and MDA− in 2D. Both highly and weakly migratory cells significantly increased ROS production in response to treatment with AMA and OLM (Figures S1A–S1F), though OLM caused significantly more ROS production than AMA in both MDA+ and MDA− (Figures S1C–S1F). To parse whether migratory changes observed after treatment with inhibitors were owing to the disruption of mitochondrial energy or induced ROS production, we utilized the ROS activator, tert-butyl hydroperoxide (TBHP). ROS activation decreased both MDA− and MDA+ microtrack migration. Migration speeds were decreased significantly more in MDA+ compared to MDA− (Figure S1G), suggesting that highly migratory cancer cells may be more susceptible to ROS-induced decreased migration than weakly migratory cancer cells. As MDA+ treated with OLM exhibited increased ROS and decreased migration compared to control conditions, it is possible that OLM decreased MDA+ migration through increased ROS rather than decreased ATP synthase activity. As weakly migratory cancer cell migration did not increase after ROS induction, the increased migration of MDA− after oxidative phosphorylation inhibition is regulated by a mechanism separate from ROS.
Modulation of glucose metabolism regulates the epithelial-to-mesenchymal transition phenotype to drive migration
Given that weakly and highly migratory cells preferentially utilize different modes of glucose metabolism (Figure 3), that the modulation of EMT phenotype reprograms metabolism to regulate migration (Figure 4), and that the inhibition of oxidative phosphorylation increases migration velocity of weakly migratory cancer cells (Figure 5), we sought to determine whether EMT status drives the differential migration response by weakly and highly migratory cancer cells to glucose metabolism inhibitors. Previous work suggests that glycolytic enzymes and mitochondrial dysfunction can induce EMT (Ahmad et al., 2011; Funasaka et al., 2009; Han et al., 2018; Kang et al., 2019). Thus, we investigated whether glucose metabolism inhibitors could shift the EMT phenotype to drive migratory changes (Figure 6A). As mesenchymal cells display increased aspect ratios compared to epithelial cells (Leggett et al., 2016; Maier et al., 2016; Ribatti et al., 2020), we first quantified cell aspect ratio after treatment with IA + PYR or AMA to assess changes in EMT status. MDA− exhibited decreased aspect ratios compared to MDA+ in bulk collagen gels, consistent with more mesenchymal cells being more elongated (Figure 6B). MDA− and MDA+ treated with IA + PYR displayed no change in aspect ratio (Figures 6C and 6D), suggesting that IA + PYR has no effect on EMT status. Treatment with AMA significantly increased the elongation of both the more epithelial, weakly migratory MDA− and the more mesenchymal, highly migratory MDA+ (Figures 6E and 6F).
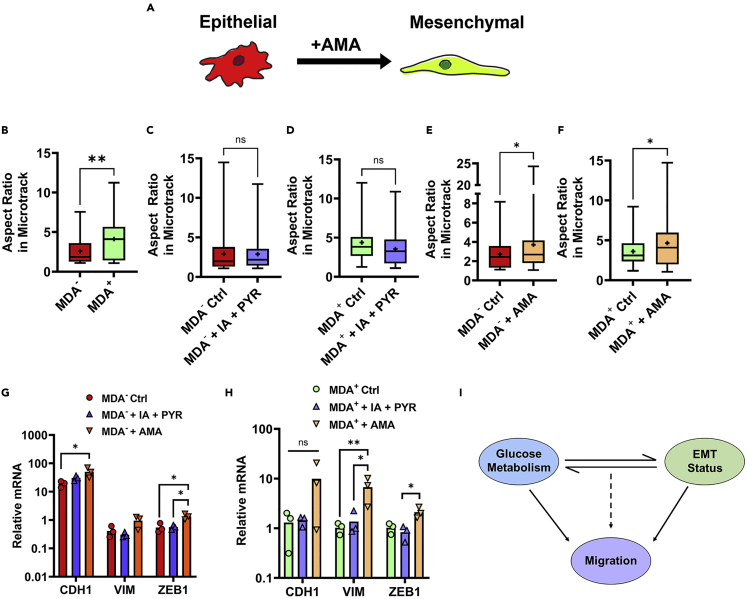
Modulation of glucose metabolism differentially regulates EMT phenotype
(A) Schematic of metabolism-regulated EMT.
(B) aspect ratio of MDA− and MDA+ (N = 3, n = 30-39).
(C) aspect ratio of MDA− 6-12 h after vehicle control (Ctrl) or IA + PYR application (+IA + PYR) (N = 3+, n = 34-36).
(D) aspect ratio of MDA+ 6-12 h after vehicle control or IA + PYR (N = 3+, n = 34-67).
(E) aspect ratio of MDA− 6-12 h after vehicle control or AMA (+AMA) (N = 3+, n = 61-64).
(F) aspect ratio of MDA+ 6-12 h after vehicle control or AMA (N = 3+, n = 48-85).
(G) relative mRNA of MDA− treated with vehicle control, IA + PYR, or AMA for 12 h. Normalized to β-actin. (N = 3).
(H) Relative mRNA of MDA+ treated with vehicle control, IA + PYR, or AMA for 12 h. Normalized to β-actin. (N = 3);
(I) schematic of link between glucose metabolism and EMT driving cell migration; denotes p < 0.05,
denotes p < 0.01, ns = not significant. Mean + individual data points is shown for bar graphs and min to max for box-and-whisker plots with mean represented as “+.”
To further assess EMT phenotype after treatment with glucose inhibitors, RNA expression of epithelial marker E-cadherin (CDH1) and mesenchymal markers vimentin (VIM) and Zeb1 (ZEB1) were quantified using quantitative RT-PCR. Treatment of MDA− or MDA+ with IA + PYR resulted in no significant change in CDH1, VIM, or ZEB1 mRNA (Figures 6G and 6H), further reinforcing that the inhibition of GAPDH in glycolysis has no effect on the EMT status of the phenotypically sorted weakly and highly migratory breast cancer cells. Conversely, the treatment of MDA− with AMA significantly increased both CDH1 and ZEB1 mRNA (Figure 6G) and treatment of MDA+ with AMA significantly increased VIM and ZEB1 mRNA (Figure 6H). These results reveal that AMA increased mesenchymal gene expression in both MDA− and MDA+. Interestingly, treatment with AMA selectively increased MDA− migration and not MDA+ migration compared to vehicle control conditions.
Altogether, these findings suggest that while glycolysis inhibition significantly impairs migration velocity, it has little effect on EMT phenotype. Instead, we observe a significant change in EMT markers upon mitochondrial impairment with AMA, indicating that while modulating glucose metabolism through glycolysis or mitochondrial inhibition can affect migration velocity, the EMT phenotype is more dependent on mitochondrial function.
Discussion
Cancer cell phenotypic heterogeneity is associated with poor clinical outcomes and complicates cancer therapy (Fisher et al., 2013; Hapach et al., 2021; Ramón y Cajal et al., 2020); therefore, a better understanding of the cancer cell populations that exist and work cooperatively in the TME is essential to develop effective cancer therapies. It was recently described that primary tumors and micrometastases exhibit distinct metabolic transcriptomes, with primary tumors primarily utilizing glycolysis as described by the Warburg Effect and micrometastases displaying a preference for mitochondrial oxidative phosphorylation (Davis et al., 2020). These different metabolic behaviors indicate that metabolism is highly plastic and context-dependent and that glycolysis and oxidative phosphorylation may fuel different cell behaviors, such as proliferation and colonization, with different metastatic advantages. Here, we investigate the role of glucose metabolism in cell migration and the relationship between heterogeneity in metabolism and migratory ability in triple-negative breast cancer. We identify that the interplay between glucose metabolism and EMT phenotype drives heterogeneous migratory behaviors.
Cancer cell bioenergetics and migration are linked in that ATP fuels cell progression through specific steps in the metastatic cascade (Bergers and Fendt, 2021). As it is technically challenging to precisely quantify single-cell metabolism during in vivo metastasis, physiologically relevant in vitro platforms that model steps in cancer metastasis may provide valuable insight that is otherwise unachievable. Here, we sought to isolate the roles of glycolysis and oxidative phosphorylation in breast cancer cell migration using an engineered microtrack platform that mimics the confinement and material composition of the ECM found in vivo. Conflicting evidence exists as to whether breast cancer single-cell migration is primarily driven by glycolysis, oxidative phosphorylation, or a combination of both pathways (LeBleu et al., 2014; Shiraishi et al., 2015; Zhao et al., 2013). Glycolysis regulates cytoskeletal dynamics required by cell migration (Costanza et al., 2019; DeCamp et al., 2020; Kondo et al., 2021; Park et al., 2020; Shiraishi et al., 2015) and extracellular acidification owing to lactate output increases MMP activation, matrix degradation, and the reorganization of cytoskeletal machinery (Gatenby and Gawlinski, 2003; Lardner, 2001), suggesting that cell migration is glycolysis driven. However, it has also been shown that enhanced oxidative phosphorylation and mitochondria localization to the leading edge increases cell migratory ability (Cunniff et al., 2016; Kelley et al., 2019; Porporato et al., 2014). Importantly, our results suggest that glycolysis plays a major role in fuel breast cancer cell migration in confined 3D environments. The dichotomy in these findings may result from differing in vitro models used to monitor cell migration. 3D hydrogels, transwell assays, and microfabricated systems have different mechanical parameters, including stiffness, confinement, and alignment, all of which are known to impact cell metabolism (Ponce et al., 2021; Sullivan et al., 2018; Zanotelli et al., 2019). Importantly, our microtrack system models protease-independent migration as cells do not need to degrade the matrix to migrate (Kraning-Rush et al., 2013). Although our results suggest that protease-independent migration is fueled primarily by glycolysis, other forms of single-cell migration may require mitochondrial energy utilization.
Using this microtrack platform, we show that oxidative phosphorylation inhibition did not elicit a homogenous migratory response. Although highly migratory cells showed no change in migration, weakly migratory cells displayed increased migration in response to AMA treatment, suggesting that highly and weakly migratory cancer cells differentially utilize oxidative phosphorylation. We further investigated this phenomenon by phenotypically sorting MDA-MB-231 triple-negative breast cancer cells based on their in vitro migratory potential. Highly migratory cancer cells demonstrated increased glucose uptake while weakly migratory cells exhibited higher levels of mitochondrial membrane potential. We previously showed that phenotypically sorted weakly migratory MDA-MB-231 and SUM159 are more metastatic in a mouse model of breast cancer metastasis than their highly migratory counterparts (Hapach et al., 2021). Our results reveal that even within a single cancer cell line, heterogeneity in migratory ability and metastatic ability (Hapach et al., 2021) is accompanied by heterogeneity in bioenergetics. Although glycolysis may fuel primary tumor growth and cancer cell migration and dissemination away from the primary tumor, oxidative phosphorylation may be metabolically advantageous for metastasis. In agreement, oxidative phosphorylation inhibition has little effect on primary tumor growth, but significantly decreased metastatic seeding of breast cancer cells (LeBleu et al., 2014). These data, in addition to others showing that oxidative phosphorylation is upregulated in secondary metastatic sites (Chen et al., 2007; Dupuy et al., 2015; Porporato et al., 2014; Porporato and Sonveaux, 2014), correspond with our previous findings that weakly migratory cells that preferentially utilize oxidative phosphorylation are more metastatic than their highly migratory counterparts (Hapach et al., 2021).
RNA sequencing of phenotypically sorted triple-negative breast cancer cell lines revealed that highly migratory cells had more mesenchymal and glycolysis-associated features than their weaky migratory counterparts, which had higher oxidative phosphorylation and epithelial-associated features. To determine if EMT status regulates glucose metabolism and controls migratory potential, EMT of the highly and weakly migratory cancer cells was modulated, and metabolic and migratory changes were assessed. Inducing a mesenchymal phenotype with TGFβ caused a metabolic switch resulting in increased glucose uptake and decreased mitochondrial membrane potential and was accompanied by increased migration velocity. Inducing a more epithelial phenotype by knocking in E-cadherin resulted in a switch towards resulting in decreased glucose uptake and increased mitochondrial membrane potential and decreased migration velocity. These findings are consistent with previous studies that found that drivers of EMT can upregulate glycolysis (Han et al., 2018; Kang et al., 2019; Kim et al., 2017; Masin et al., 2014; Sciacovelli and Frezza, 2017; Sun and Yang, 2020; Zhang et al., 2018). Although others have reported that E-cadherin overexpression can increase oxidative phosphorylation (Park et al., 2017), the mechanism and consequences of this signaling are unclear. Here, we show that these metabolic changes are associated with downstream migration, suggesting that the link between cell metabolism and EMT may fuel different migratory potentials. It is important to note that while we can manipulate EMT status in our subpopulations, we do not recapitulate a fully epithelial or fully mesenchymal status. We show that even minute changes in EMT status result in migratory and metabolic changes. Our ability to move cells along this spectrum rather than inducing one state or the other allows us to capture the intermediate partial-EMT phenotypes that may be essential in cooperative progression through the metastatic cascade.
We also investigated whether changes in glucose metabolism subsequently result in EMT and migratory changes. Inhibition of glycolysis decreased the migration of the highly migratory cells, while the inhibition of oxidative phosphorylation increased the migration of the weakly migratory cells. Although inhibiting glycolysis may weaken migratory potential through reduced ATP availability (Garde and Sherwood, 2021; Shiraishi et al., 2015; Zanotelli et al., 2018), it was unclear why the inhibition of oxidative phosphorylation would promote the migration of weakly migratory cells. As such, we investigated whether metabolic changes induce changes in EMT status and migration. Although inhibiting glycolysis had little effect on EMT status as evidenced by cell aspect ratio and mRNA expression of EMT markers, we were able to induce changes in EMT phenotype by inhibiting mitochondrial energy, suggesting a link between metabolism and EMT that may be more dependent on mitochondrial ability than glucose metabolism as a whole.
The role of metabolism in cell migration continues to be explored in the context of cancer progression and metastasis. Here, we highlight a novel link between glucose metabolism and EMT status as the driving factor in migratory heterogeneity in breast cancer cells. We show that while highly and weakly migratory breast cancer cells exhibit unique metabolic and EMT profiles, we are able to control migration by manipulating either metabolism or EMT. Specifically, weakly migratory cells utilizing primarily oxidative phosphorylation for ATP can be pushed to a more migratory phenotype by inhibiting mitochondrial respiration and forcing cells to utilize glycolysis instead, or by inducing a more mesenchymal phenotype with TGFβ. Similarly, the migration of highly migratory cells can be attenuated by blocking their main ATP source, glycolysis, or inducing an epithelial phenotype with E-cadherin. Although our primary focus here is single-cell migration in a representative engineered model of the primary tumor microenvironment, our data in combination with further characterization of the relationship between EMT and glucose metabolism pathways in each step of the metastatic cascade may reveal key therapeutic targets for inhibiting cancer metastasis.
Limitations of the study
While glucose metabolism, primarily glycolysis and oxidative phosphorylation, is a key method cell utilize to generate ATP, cancer metabolism encompasses a wide range of pathways and intermediates that contribute to cellular energetics. For example, we observed a decrease in migration in response to ROS activation (Figure S1G). However, because the changes observed owing to ROS activation were inconsistent with those induced by metabolism inhibitors, it is likely that a different mechanism is involved. It is possible that the link between ROS and migration is dependent on an optimal level of ROS that may be challenging to parse in our microtrack system. However, these findings warrant further investigation into the role of ROS in differential migratory phenotypes.
Recently, glutamine metabolism has gained increasing attention as one of the main sources of cancer cell energy. Here, we chose to investigate the roles of glycolysis and oxidative phosphorylation as these have been specifically implicated in migratory processes in cancer cells. However, our current study lacks investigation into compensatory mechanisms from other energy sources when glucose metabolism is externally modulated. Future work characterizing the link between other forms of metabolism and EMT status is required for a complete understanding of the role of bioenergetics in regulating heterogeneity in cell migration.
Our engineered platform provides a unique and unparalleled characterization of confined migration in a physiologically relevant environment. Owing to the nature of the device, however, our study is limited to observing only what happens in real time in the microtracks and removing cells from the system for further bulk analysis, such as mRNA and protein analysis, is technically challenging, if not impossible. This limits our ability to probe confinement as a mechanical cue altering epigenetic expression of different factors that may be pivotal in determining cell migratory ability. However, we use the platform to first identify a preliminary relationship between EMT, glucose metabolism, and migration that is then further parsed in 2D and 3D settings.
STAR
Methods
Key resources table
REAGENT or RESOURCE | SOURCE | IDENTIFIER |
---|---|---|
Bacterial and virus strains | ||
FUW-E-cadherin-E2A | In-house | N/A |
pMSCV-Peredox-mCherry-NLS | Hung et al. Cell Metab. 2011 | Addgene #32385; RRIDL Addgene_32385 |
Chemicals, peptides, and recombinant proteins | ||
Iodoacetate | Millipore Sigma | I2512 |
Antimycin-A | Millipore Sigma | A8674 |
Oligomycin | Sigma-Aldrich | O4876-25MG |
Transforming Growth Factor-Beta | Sigma-Aldrich | T7039 |
2NBDG | Invitrogen | N13195 |
PureZOL RNA isolation reagent | Bio-Rad | 7326880 |
SYBR Green | Bio-Rad | 1708880 |
Critical commercial assays | ||
Image-iT LIVE Green ROS Detection Kit (containing Tertbutylhydroperoxide, Hoechst 3342, and carboxy-H2DCFDA) | ThermoFisher | I36007 |
Image-iT TMRM | Invitrogen | I34361 |
LIVE/DEAD™ Cell Imaging Kit 488/570 | Thermofisher | R37601 |
RNeasy Mini Kit | Qiagen | 74004 |
Lactate Assay Kit | Abcam | ab65331 |
iScript cDNA Synthesis Kit | Bio-Rad | 1708890 |
Deposited data | ||
Raw and Analyzed Data | This paper | Dryad: https://doi.org/10.5061/dryad.6djh9w149 |
Experimental models: Cell lines | ||
Human MDA-MB-231 mammary adenocarcinoma epithelial cells | ATCC | HTB-26; RRID: CVCL_0062 |
Human SUM159PT breast epithelial cells | BioIVT | HUMANSUM_0003006; RRID: CVCL_5423 |
Oligonucleotides | ||
qPCR Primer (see Table S1) | This paper | N/A |
Software and algorithms | ||
Fiji | Schindelin et al., 2012 | https://imagej.net/software/fiji/; RRID: SCR_002285 |
Resource availability
Lead contact
Further information and requests for resources or reagents should be addressed to Cynthia Reinhart-King ([email protected])
Materials availability
FUW-E-cadherin-E2A plasmid is available from the lead contact upon request.
Data and code availability
This paper does not report original code.
Raw data will be deposited at Dryad (Dryad Dataset: https://doi.org/10.5061/dryad.6djh9w149) and be publicly available upon date of publication.
Any additional information is available from the lead contact upon request.
Experimental model and subject details
Cell culture and reagents
MDA-MB-231 female human mammary adenocarcinoma cells (HTB-26, ATCC, Manassas, VA) were maintained in Dulbecco’s Modified Eagle’s Media (DMEM) (ThermoFisher Scientific, Waltham, PA) supplemented with 10% fetal bovine serum (Atlanta Biologicals, Flowery Branch, GA) and 1% penicillin-streptomycin (ThermoFisher Scientific) at 37°C and 5% CO2. Iodoacetate (10 μM, Millipore Sigma, I2512), sodium pyruvate (100 μM, ThermoFisher Scientific, 11360070), oligomycin (100 μM, Sigma-Aldrich, O4876), and antimycin-A (250 μM in 2D, 500 μM in microtracks, Millipore Sigma, A8674) were used to inhibit glucose metabolism. TGFβ (5 ng/mL in 2D, 50 ng/mL in microtracks) was used to induce EMT. Tertbutylhydroperoxide (SUM159PT human breast epithelial cells (BioIVT) were maintained in Ham’s F12 Medium (ThermoFisher Scientific) supplemented with 5% fetal bovine serum, 1 μg/mL hydrocortisone (Millipore Sigma), 5 μg/mL insulin (Millipore Sigma), and 1% penicillin-streptomycin. Cell culture and time-lapse imaging were performed at 37°C and 5% CO2.
Method details
Microtrack fabrication
Collagen microtracks of 10 μm width were prepared as previously described (Carey et al., 2015). Briefly, 100-mm diameter silicon wafer molds were coated with S1813 photoresist and Bosch etched to pattern with microtrack geometries. Polydimethylsiloxane (PDMS; Sylgard) was mixed in a 1:10 ratio of cross-linker to monomer, poured over the wafer, and cured to be used as a template stamp. Collagen Type I extracted from rat tail tendons was prepared in-house and resuspended as a 10 mg/mL stock solution in acetic acid. The stock solution was diluted to 3 mg/mL, neutralized with 1 N NaOH, and used to lightly coat PDMS stamps. Stamps were inverted onto a drop of diluted collagen between two thin PDMS spacers and left to polymerize for 90 min at 37°C. To finalize 3D microtracks, patterned collagen matrices were seeded with either parental (MDAPAR), highly migratory (MDA+), or weakly migratory MDA-MB-231 (MDA−) (~100,000 cells/mL to achieve low seeding density) and left to settle for 2 min at 37°C. A thin layer of collagen was applied to a glass coverslip and inverted over the microtracks as a lid. Microtrack system was supplied with fresh media was fully polymerized, and cells were left to incubate overnight at 37°C. At ~18 h post cell seeding, glucose metabolism inhibitors, ROS activator, or vehicle controls were added to microtracks in fresh media. Timelapse images of cells were acquired every 20 min from 1-12 h post inhibitor application, acquired using a Zeiss Axio Observer Z1 inverted microscope equipped with a Hamamatsu ORCA-ER camera with a 10×/0.3 NA objective for migration studies. AxioVision software was used to operate device. Where applicable, cells were supplemented with 50 ng/mL TGFβ 24 h before imaging, followed by 100 nM of TMRM (Invitrogen, U34361) 2 h before imaging. Timelapse images were acquired every 20 min 24–30 h after TGFβ treatment using Zeiss LSM800 Confocal Microscope with a 10×/0.3 NA Zeiss Objective with a 10X objective.
Analysis of cell migration in microtracks
Cell migration was analyzed by using Fiji ImageJ (National Institute of Health, Bethesda, MD) (Schindelin et al., 2012) to outline individual cells in collagen microtracks. The cell body was manually outlined, and cell area, centroid, and aspect ratio were quantified. Cell displacement was calculated as the distance between cell centroids between each frame. Average velocity was then calculated by dividing the displacement by the time step. For each frame, the number of microtrack walls the cell contacted was logged. For TGFβ studies, cell velocity was measured and TMRM corrected total cell fluorescence (CTCF = Integrated Density – (Mean Intensity of BackgroundCell Area)) was calculated where applicable. Cells undergoing cell division, apoptosis, or interacting with other cells were excluded from analysis.
Phenotypic sorting of breast cancer cells
Breast cancer cell lines were sorted as previously described (Hapach et al., 2021). Briefly, breast cancer cells were seeded on top of a transwell insert (Greiner Bio-One, Kremsmunster, Austria) with 8 μm pores coated in 1 mg/mL collagen. A serum gradient was applied, and cells were able to migrate through the transwell insert for four days. After four days, highly migratory and weakly migratory cells were collected and purified over 20 rounds of sorting. Cells that repeatedly migrated through the transwell were termed ‘highly migratory’ (MDA+, SUM+) and cells that never migrated through the transwell were termed ‘weakly migratory’ (MDA−, SUM−).
Motile fraction
MDA+ or MDA− were seeded into 1.5 mg/mL collagen gels at a density of 200,000 cells/mL. Cells were incubated for 24 h at 37°C. Timelapse images of cells were acquired every 20 min for 12 h, acquired using a Zeiss Axio Observer Z1 inverted microscope equipped with a Hamamatsu ORCA-ER camera with a 10×/0.3 NA objective. AxioVision software was used to operate device. Motile fraction was quantified as the fraction of cells that migrated at least one cell body every 2 h. Aspect ratio of cells was quantified using ImageJ.
RNA sequencing
RNA was isolated using the RNeasy mini kit (Qiagen, 74004) and RNAase-Free DNase Set according to manufacturer protocols. RNA sequencing was performed by the Vanderbilt Technologies for Advanced Genomics core (VANTAGE) as previously described (Hapach et al., 2021). EMT and Energy Scores were computed from the average normalized expression of manually curated lists of epithelial, mesenchymal, glycolysis, and oxidative phosphorylation genes.
Glucose uptake
Cells were cultured for 24 h in DMEM +10% FBS. After 24 h, fresh media supplemented with 0.146 mM 2-NBDG (Life Technologies) was added and cells were supplemented with 5 ng/mL TGFβ if applicable. After 24 h, cells were fixed in 3.2% paraformaldehyde and imaged on a Zeiss LSM800 Confocal Microscope with a 10×/0.3 NA Zeiss Objective with a 10X objective. To calculate 2-NBDG uptake, cells were manually outlined and the corrected total cell fluorescence (CTCF = Integrated Density – (Mean Intensity of BackgroundCell Area)) was calculated.
Mitochondrial membrane potential
Cells were cultured for 24 h in DMEM +10% FBS and cells were supplemented with 5 ng/mL TGFβ if applicable. After 24 h, fresh media supplemented with 75 nM TMRM (Invitrogen, U34361) was added. After 30 min, cells were washed with PBS and fixed in 3.2% paraformaldehyde. Cells were imaged on a Zeiss LSM800 Confocal Microscope with a 10×/0.3 NA Zeiss Objective. To calculate TMRM, cells were manually outlined and the corrected total cell fluorescence (CTCF = Integrated Density – (Mean Intensity of BackgroundCell Area)) was calculated.
Lactate output
MDA+ and MDA− were cultured for 24 h in DMEM +10% FBS. Conditioned media was removed, and lactate output was measured using the L-Lactate Assay Kit (Abcam, ab65331).
DNA constructs
FUW-E-cadherin-E2A plasmid was created in-house and was used to stably transduce MDA+. pMSCV-Peredox-mCherry-NLS was a gift from Gary Yellen (Addgene plasmid # 32385; http://n2t.net/addgene:32385; RRID:Addgene_32385). The Peredox probe was calibrated to lactate and pyruvate levels as previously described (Hung and Yellen, 2014).
ROS labeling and activation
Cells were cultured for 24 h on glass in DMEM +10% FBS. After 24 h, fresh media supplemented with mitochondrial inhibitors, 250 μM antimycin-A or 100 μM oligomycin, was added and incubated for 6 h. At 6 h, cells were washed in Hank’s Balanced Salt Solution with Calcium and Magnesium (Corning) (HBSS) and 25 μM carboxy-H2DCFDA green-fluorescent ROS label (Invitrogen, I36007) in HBSS was added. Cells were incubated for 30 min at 37°, with 1 μM Hoechst’s 3342 (Invitrogen) added in the last 5 min of incubation. Cells were washed in HBSS and imaged immediately with a Zeiss LSM800 Confocal Microscope with a 20×/0.8 NA Zeiss Objective. To calculate ROS, cells were manually outlined and the corrected total cell fluorescence (CTCF = Integrated Density – (Mean Intensity of BackgroundCell Area)) was calculated. For activation of ROS in microtracks, ROS activator tert-butyl hydroperoxide (TBHT) (Invitrogen, I36007) or vehicle control was added in fresh media at the recommended dose of 100 μM 1 h before imaging migration.
Metabolism inhibitor dose curves
MDAPAR were seeded into 1.5 mg/mL collagen gels at a density of 200,000 cells/mL. Cells were incubated overnight at 37°C. Either antimycin-A (10, 100, or 500 μM), iodoacetate (10, 100, or 500 μM), or vehicle controls were supplied to cells in fresh media and incubated for 1,6, or 12 h at 37°C. For iodoacetate treated cells, 0.146 mM 2-NBDG (Life Technologies) was supplied at the same time as treatment. At each timepoint, cells were washed with PBS and fixed in 3.2% paraformaldehyde. For antimycin-A treated cells, fresh media supplemented with 75 nM TMRM (Invitrogen, U34361) was added at each timepoint. After 30 min, cells were washed with PBS and fixed in 3.2% paraformaldehyde. Both treatment groups were imaged on a Zeiss LSM800 Confocal Microscope with a 20×/0.8 NA Zeiss Objective. To calculate 2-NBDG or TMRM, cells were manually outlined and the corrected total cell fluorescence (CTCF = Integrated Density – (Mean Intensity of BackgroundCell Area)) was calculated.
Cell viability
MDAPAR were seeded into 1.5 mg/mL collagen gels at a density of 200,000 cells/mL. Cells were incubated overnight at 37°C. After incubation, cells were treated with either antimycin-A (10, 100, or 500 μM), oligomycin (10, 100, or 500 μM), iodoacetate (10 or 100 μM), or vehicle controls and allowed to incubate at 37°C for 12 h. At 12 h, cells were supplied with equal volumes of media and Live Green/Dead Red solution (Thermofisher, LIVE/DEAD™ Cell Imaging Kit 488/570, R37601). After 15 min incubation, cells were imaged on a Zeiss LSM800 Confocal Microscope with a 20×/0.8 NA Zeiss Objective.
Quantitative PCR
mRNA was isolated from cells using PureZOL RNA isolation reagent (Bio-Rad). cDNA was synthesized from mRNA using iScript cDNA Synthesis Kit (Bio-Rad). QPCR was completed using SYBR green (Bio-Rad). Relative mRNA expression was calculated as 2−ddCt and β-actin was used to normalize results. Primers listed in Table S1.
Quantification and statistical analysis
All statistical analysis was performed using GraphPad Prism. Box-and-whisker plots show mean, 25th/75th percentile, and whiskers represent maximum and minimum values. Fold change bar graphs and dose curves show mean ± SEM. Dot plots show individual data points. denotes p value < 0.05,
denotes <0.01,
denotes <0.001, and
denotes <0.0001.
Acknowledgments
This work was supported by the W.M. Keck Foundation and the National Institutes of Health (GM131178) to C.A.R.-K and National Science Foundation Graduate Research Fellowship Awards under Grant. No. 1937963 to S.C.S. and J.A.M and Grant No. DGE-1650441 to L.A.H. We thank Dr. Thong Cao for the plasmid design. We thank Emily Chu, Emily Fabiano, and Chelsea Mariano for the preliminary analysis.
Author contributions
Conceptualization: C.A.R.-K., S.C.S., J.A.M., L.A.H.
Formal analysis: S.C.S., J.A.M., P.V.T., R.S.P., A.W., Q.X., I.O.
Investigation: S.C.S., J.A.M., L.A.H., P.V.T.
Writing-original draft: S.C.S., J.A.M.
Resources: C.A.R.-K.
Supervision: C.A.R.-K.
Funding Acquisition: C.A.R.-K.
Writing-review and editing: C.A.R.-K.
Declaration of interests
No conflicts of interest are declared by the authors.
Footnotes
Supplemental information can be found online at https://doi.org/10.1016/j.isci.2022.105190.
References
- Aggarwal V., Tuli H.S., Varol A., Thakral F., Yerer M.B., Sak K., Varol M., Jain A., Khan M.A., Sethi G. Role of reactive oxygen species in cancer progression: molecular mechanisms and recent advancements. Biomolecules. 2019;9:735. 10.3390/biom9110735. [Europe PMC free article] [Abstract] [CrossRef] [Google Scholar]
- Ahmad A., Aboukameel A., Kong D., Wang Z., Sethi S., Chen W., Sarkar F.H., Raz A. Phosphoglucose isomerase/autocrine motility factor mediates epithelial-mesenchymal transition regulated by miR-200 in breast cancer cells. Cancer Res. 2011;71:3400–3409. 10.1158/0008-5472.CAN-10-0965. [Europe PMC free article] [Abstract] [CrossRef] [Google Scholar]
- Aiello N.M., Maddipati R., Norgard R.J., Balli D., Li J., Yuan S., Yamazoe T., Black T., Sahmoud A., Furth E.E., et al. EMT subtype influences epithelial plasticity and mode of cell migration. Dev. Cell. 2018;45:681–695.e4. 10.1016/j.devcel.2018.05.027. [Europe PMC free article] [Abstract] [CrossRef] [Google Scholar]
- Banyard J., Bielenberg D.R. The role of EMT and MET in cancer dissemination. Connect. Tissue Res. 2015;56:403–413. 10.3109/03008207.2015.1060970. [Europe PMC free article] [Abstract] [CrossRef] [Google Scholar]
- Bergers G., Fendt S.-M. The metabolism of cancer cells during metastasis. Nat. Rev. Cancer. 2021;21:162–180. 10.1038/s41568-020-00320-2. [Europe PMC free article] [Abstract] [CrossRef] [Google Scholar]
- Carey S.P., Rahman A., Kraning-Rush C.M., Romero B., Somasegar S., Torre O.M., Williams R.M., Reinhart-King C.A. Comparative mechanisms of cancer cell migration through 3D matrix and physiological microtracks. Am. J. Physiol. Cell Physiol. 2015;308:C436–C447. 10.1152/ajpcell.00225.2014. [Europe PMC free article] [Abstract] [CrossRef] [Google Scholar]
- Chen E.I., Hewel J., Krueger J.S., Tiraby C., Weber M.R., Kralli A., Becker K., Yates J.R., Felding-Habermann B. Adaptation of energy metabolism in breast cancer brain metastases. Cancer Res. 2007;67:1472–1486. 10.1158/0008-5472.CAN-06-3137. [Abstract] [CrossRef] [Google Scholar]
- Chen G., Zhang Y., Liang J., Li W., Zhu Y., Zhang M., Wang C., Hou J. Deregulation of hexokinase II is associated with glycolysis, autophagy, and the epithelial-mesenchymal transition in tongue squamous cell carcinoma under hypoxia. BioMed Res. Int. 2018;2018:e8480762. 10.1155/2018/8480762. [Europe PMC free article] [Abstract] [CrossRef] [Google Scholar]
- Costanza B., Rademaker G., Tiamiou A., De Tullio P., Leenders J., Blomme A., Bellier J., Bianchi E., Turtoi A., Delvenne P., et al. Transforming growth factor beta-induced, an extracellular matrix interacting protein, enhances glycolysis and promotes pancreatic cancer cell migration. Int. J. Cancer. 2019;145:1570–1584. 10.1002/ijc.32247. [Abstract] [CrossRef] [Google Scholar]
- Cunniff B., McKenzie A.J., Heintz N.H., Howe A.K. AMPK activity regulates trafficking of mitochondria to the leading edge during cell migration and matrix invasion. Mol. Biol. Cell. 2016;27:2662–2674. 10.1091/mbc.E16-05-0286. [Europe PMC free article] [Abstract] [CrossRef] [Google Scholar]
- Dad A., Jeong C.H., Pals J.A., Wagner E.D., Plewa M.J. Pyruvate remediation of cell stress and genotoxicity induced by haloacetic acid drinking water disinfection by-products. Environ. Mol. Mutagen. 2013;54:629–637. 10.1002/em.21795. [Europe PMC free article] [Abstract] [CrossRef] [Google Scholar]
- Davis R.T., Blake K., Ma D., Gabra M.B.I., Hernandez G.A., Phung A.T., Yang Y., Maurer D., Lefebvre A.E.Y.T., Alshetaiwi H., et al. Transcriptional diversity and bioenergetic shift in human breast cancer metastasis revealed by single-cell RNA sequencing. Nat. Cell Biol. 2020;22:310–320. 10.1038/s41556-020-0477-0. [Abstract] [CrossRef] [Google Scholar]
- DeCamp S.J., Tsuda V.M.K., Ferruzzi J., Koehler S.A., Giblin J.T., Roblyer D., Zaman M.H., Weiss S.T., Kılıç A., De Marzio M., et al. Epithelial layer unjamming shifts energy metabolism toward glycolysis. Sci. Rep. 2020;10:18302. 10.1038/s41598-020-74992-z. [Europe PMC free article] [Abstract] [CrossRef] [Google Scholar]
- Dupuy F., Tabariès S., Andrzejewski S., Dong Z., Blagih J., Annis M.G., Omeroglu A., Gao D., Leung S., Amir E., et al. PDK1-Dependent metabolic reprogramming dictates metastatic potential in breast cancer. Cell Metab. 2015;22:577–589. 10.1016/j.cmet.2015.08.007. [Abstract] [CrossRef] [Google Scholar]
- Engbring J.A., Kleinman H.K. The basement membrane matrix in malignancy. J. Pathol. 2003;200:465–470. 10.1002/path.1396. [Abstract] [CrossRef] [Google Scholar]
- Fares J., Fares M.Y., Khachfe H.H., Salhab H.A., Fares Y. Molecular principles of metastasis: a hallmark of cancer revisited. Signal Transduct. Target. Ther. 2020;5:28. 10.1038/s41392-020-0134-x. [Europe PMC free article] [Abstract] [CrossRef] [Google Scholar]
- Fisher R., Pusztai L., Swanton C. Cancer heterogeneity: implications for targeted therapeutics. Br. J. Cancer. 2013;108:479–485. 10.1038/bjc.2012.581. [Europe PMC free article] [Abstract] [CrossRef] [Google Scholar]
- Funasaka T., Hogan V., Raz A. Phosphoglucose isomerase/autocrine motility factor mediates epithelial and mesenchymal phenotype conversions in breast cancer. Cancer Res. 2009;69:5349–5356. 10.1158/0008-5472.CAN-09-0488. [Europe PMC free article] [Abstract] [CrossRef] [Google Scholar]
- Garde A., Sherwood D.R. Fueling cell invasion through extracellular matrix. Trends Cell Biol. 2021;31:445–456. 10.1016/j.tcb.2021.01.006. [Europe PMC free article] [Abstract] [CrossRef] [Google Scholar]
- Gatenby R.A., Gawlinski E.T. The glycolytic phenotype in carcinogenesis and tumor invasion: insights through mathematical models. Cancer Res. 2003;63:3847–3854. [Abstract] [Google Scholar]
- Han S.-Y., Jeong Y.-J., Choi Y., Hwang S.-K., Bae Y.-S., Chang Y.-C. Mitochondrial dysfunction induces the invasive phenotype, and cell migration and invasion, through the induction of AKT and AMPK pathways in lung cancer cells. Int. J. Mol. Med. 2018;42:1644–1652. 10.3892/ijmm.2018.3733. [Abstract] [CrossRef] [Google Scholar]
- Hapach L.A., Carey S.P., Schwager S.C., Taufalele P.V., Wang W., Mosier J.A., Ortiz-Otero N., McArdle T.J., Goldblatt Z.E., Lampi M.C., et al. Phenotypic heterogeneity and metastasis of breast cancer cells. Cancer Res. 2021;81:3649–3663. 10.1158/0008-5472.CAN-20-1799. [Europe PMC free article] [Abstract] [CrossRef] [Google Scholar]
- Hapach L.A., Mosier J.A., Wang W., Reinhart-King C.A. Engineered models to parse apart the metastatic cascade. Npj Precis. Oncol. 2019;3:20–28. 10.1038/s41698-019-0092-3. [Europe PMC free article] [Abstract] [CrossRef] [Google Scholar]
- Hung Y.P., Albeck J.G., Tantama M., Yellen G. Imaging cytosolic NADH-NAD(+) redox state with a genetically encoded fluorescent biosensor. Cell Metab. 2011;14:545–554. 10.1016/j.cmet.2011.08.012. [Europe PMC free article] [Abstract] [CrossRef] [Google Scholar]
- Hung Y.P., Yellen G. Live cell imaging of cytosolic NADH-NAD+ redox state using a genetically encoded fluorescent biosensor. Methods Mol. Biol. 2014;1071:83–95. 10.1007/978-1-62703-622-1_7. [Europe PMC free article] [Abstract] [CrossRef] [Google Scholar]
- Jia D., Park J.H., Kaur H., Jung K.H., Yang S., Tripathi S., Galbraith M., Deng Y., Jolly M.K., Kaipparettu B.A., et al. Towards decoding the coupled decision-making of metabolism and epithelial-to-mesenchymal transition in cancer. Br. J. Cancer. 2021;124:1902–1911. 10.1038/s41416-021-01385-y. [Europe PMC free article] [Abstract] [CrossRef] [Google Scholar]
- Kang H., Kim H., Lee S., Youn H., Youn B. Role of metabolic reprogramming in epithelial–mesenchymal transition (EMT) Int. J. Mol. Sci. 2019;20:2042. 10.3390/ijms20082042. [Europe PMC free article] [Abstract] [CrossRef] [Google Scholar]
- Kelley L.C., Chi Q., Cáceres R., Hastie E., Schindler A.J., Jiang Y., Matus D.Q., Plastino J., Sherwood D.R. Adaptive F-actin polymerization and localized ATP production drive basement membrane invasion in the absence of MMPs. Dev. Cell. 2019;48:313–328.e8. 10.1016/j.devcel.2018.12.018. [Europe PMC free article] [Abstract] [CrossRef] [Google Scholar]
- Kim N.H., Cha Y.H., Lee J., Lee S.-H., Yang J.H., Yun J.S., Cho E.S., Zhang X., Nam M., Kim N., et al. Snail reprograms glucose metabolism by repressing phosphofructokinase PFKP allowing cancer cell survival under metabolic stress. Nat. Commun. 2017;8:14374. 10.1038/ncomms14374. [Europe PMC free article] [Abstract] [CrossRef] [Google Scholar]
- Kondaveeti Y., Guttilla Reed I.K., White B.A. Epithelial-mesenchymal transition induces similar metabolic alterations in two independent breast cancer cell lines. Cancer Lett. 2015;364:44–58. 10.1016/j.canlet.2015.04.025. [Abstract] [CrossRef] [Google Scholar]
- Kondo H., Ratcliffe C.D.H., Hooper S., Ellis J., MacRae J.I., Hennequart M., Dunsby C.W., Anderson K.I., Sahai E. Single-cell resolved imaging reveals intra-tumor heterogeneity in glycolysis, transitions between metabolic states, and their regulatory mechanisms. Cell Rep. 2021;34:108750. 10.1016/j.celrep.2021.108750. [Europe PMC free article] [Abstract] [CrossRef] [Google Scholar]
- Kraning-Rush C.M., Carey S.P., Lampi M.C., Reinhart-King C.A. Microfabricated collagen tracks facilitate single cell metastatic invasion in 3D. Integr. Biol. 2013;5:606–616. 10.1039/c3ib20196a. [Europe PMC free article] [Abstract] [CrossRef] [Google Scholar]
- Kumari S., Badana A.K., G S., Malla R. Reactive oxygen species: a key constituent in cancer survival. Biomark. Insights. 2018;13 10.1177/1177271918755391. 117727191875539. [Europe PMC free article] [Abstract] [CrossRef] [Google Scholar]
- Lam W.Y., Becker A.M., Kennerly K.M., Wong R., Curtis J.D., Llufrio E.M., McCommis K.S., Fahrmann J., Pizzato H.A., Nunley R.M., et al. Mitochondrial pyruvate import promotes long-term survival of antibody-secreting plasma cells. Immunity. 2016;45:60–73. 10.1016/j.immuni.2016.06.011. [Europe PMC free article] [Abstract] [CrossRef] [Google Scholar]
- Lambert A.W., Pattabiraman D.R., Weinberg R.A. Emerging biological principles of metastasis. Cell. 2017;168:670–691. 10.1016/j.cell.2016.11.037. [Europe PMC free article] [Abstract] [CrossRef] [Google Scholar]
- Lardner A. The effects of extracellular pH on immune function. J. Leukoc. Biol. 2001;69:522–530. [Abstract] [Google Scholar]
- LeBleu V.S., O’Connell J.T., Gonzalez Herrera K.N., Wikman H., Pantel K., Haigis M.C., de Carvalho F.M., Damascena A., Domingos Chinen L.T., Rocha R.M., et al. PGC-1α mediates mitochondrial biogenesis and oxidative phosphorylation in cancer cells to promote metastasis. Nat. Cell Biol. 2014;16:992–1003. 10.1038/ncb3039. 1–15. [Europe PMC free article] [Abstract] [CrossRef] [Google Scholar]
- Leggett S.E., Sim J.Y., Rubins J.E., Neronha Z.J., Williams E.K., Wong I.Y. Morphological single cell profiling of the epithelial-mesenchymal transition. Integr. Biol. 2016;8:1133–1144. 10.1039/c6ib00139d. [Europe PMC free article] [Abstract] [CrossRef] [Google Scholar]
- Liu K., Tang Z., Huang A., Chen P., Liu P., Yang J., Lu W., Liao J., Sun Y., Wen S., et al. Glyceraldehyde-3-phosphate dehydrogenase promotes cancer growth and metastasis through upregulation of SNAIL expression. Int. J. Oncol. 2017;50:252–262. 10.3892/ijo.2016.3774. [Abstract] [CrossRef] [Google Scholar]
- Liu Z., Lee S.J., Park S., Konstantopoulos K., Glunde K., Chen Y., Barman I. Cancer cells display increased migration and deformability in pace with metastatic progression. FASEB J. 2020;34:9307–9315. 10.1096/fj.202000101RR. [Europe PMC free article] [Abstract] [CrossRef] [Google Scholar]
- Lunt S.Y., Vander Heiden M.G. Aerobic glycolysis: meeting the metabolic requirements of cell proliferation. Annu. Rev. Cell Dev. Biol. 2011;27:441–464. 10.1146/annurev-cellbio-092910-154237. [Abstract] [CrossRef] [Google Scholar]
- Maier J., Traenkle B., Rothbauer U. Visualizing epithelial–mesenchymal transition using the chromobody technology. Cancer Res. 2016;76:5592–5596. 10.1158/0008-5472.CAN-15-3419. [Abstract] [CrossRef] [Google Scholar]
- Masin M., Vazquez J., Rossi S., Groeneveld S., Samson N., Schwalie P.C., Deplancke B., Frawley L.E., Gouttenoire J., Moradpour D., et al. GLUT3 is induced during epithelial-mesenchymal transition and promotes tumor cell proliferation in non-small cell lung cancer. Cancer Metab. 2014;2:11. 10.1186/2049-3002-2-11. [Europe PMC free article] [Abstract] [CrossRef] [Google Scholar]
- Mosier J.A., Rahman-Zaman A., Zanotelli M.R., VanderBurgh J.A., Bordeleau F., Hoffman B.D., Reinhart-King C.A. Extent of cell confinement in microtracks affects speed and results in differential matrix strains. Biophys. J. 2019;117:1692–1701. 10.1016/j.bpj.2019.09.024. [Europe PMC free article] [Abstract] [CrossRef] [Google Scholar]
- Mosier J.A., Schwager S.C., Boyajian D.A., Reinhart-King C.A. Cancer cell metabolic plasticity in migration and metastasis. Clin. Exp. Metastasis. 2021;38:343–359. 10.1007/s10585-021-10102-1. [Abstract] [CrossRef] [Google Scholar]
- Nilchian A., Giotopoulou N., Sun W., Fuxe J. Different regulation of Glut1 expression and glucose uptake during the induction and chronic stages of TGFβ1-induced EMT in breast cancer cells. Biomolecules. 2020;10:E1621. 10.3390/biom10121621. [Europe PMC free article] [Abstract] [CrossRef] [Google Scholar]
- Palmer T.D., Ashby W.J., Lewis J.D., Zijlstra A. Targeting tumor cell motility to prevent metastasis. Adv. Drug Deliv. Rev. 2011;63:568–581. 10.1016/j.addr.2011.04.008. [Europe PMC free article] [Abstract] [CrossRef] [Google Scholar]
- Park J.S., Burckhardt C.J., Lazcano R., Solis L.M., Isogai T., Li L., Chen C.S., Gao B., Minna J.D., Bachoo R., et al. Mechanical regulation of glycolysis via cytoskeleton architecture. Nature. 2020;578:621–626. 10.1038/s41586-020-1998-1. [Europe PMC free article] [Abstract] [CrossRef] [Google Scholar]
- Park S.Y., Shin J.-H., Kee S.-H. E-cadherin expression increases cell proliferation by regulating energy metabolism through nuclear factor-κB in AGS cells. Cancer Sci. 2017;108:1769–1777. 10.1111/cas.13321. [Europe PMC free article] [Abstract] [CrossRef] [Google Scholar]
- Polireddy K., Dong R., McDonald P.R., Wang T., Luke B., Chen P., Broward M., Roy A., Chen Q. Targeting epithelial-mesenchymal transition for identification of inhibitors for pancreatic cancer cell invasion and tumor spheres formation. PLoS One. 2016;11:e0164811. 10.1371/journal.pone.0164811. [Europe PMC free article] [Abstract] [CrossRef] [Google Scholar]
- Ponce I., Garrido N., Tobar N., Melo F., Smith P.C., Martínez J. Matrix stiffness modulates metabolic interaction between human stromal and breast cancer cells to stimulate epithelial motility. Metabolites. 2021;11:432. 10.3390/metabo11070432. [Europe PMC free article] [Abstract] [CrossRef] [Google Scholar]
- Porporato P.E., Payen V.L., Pérez-Escuredo J., De Saedeleer C.J., Danhier P., Copetti T., Dhup S., Tardy M., Vazeille T., Bouzin C., et al. A mitochondrial switch promotes tumor metastasis. Cell Rep. 2014;8:754–766. 10.1016/j.celrep.2014.06.043. [Abstract] [CrossRef] [Google Scholar]
- Porporato P.E., Sonveaux P. Paving the way for therapeutic prevention of tumor metastasis with agents targeting mitochondrial superoxide. Mol. Cell. Oncol. 2014;2:e968043. 10.4161/23723548.2014.968043. [Europe PMC free article] [Abstract] [CrossRef] [Google Scholar]
- Ramón Y Cajal S., Sesé M., Capdevila C., Aasen T., De Mattos-Arruda L., Diaz-Cano S.J., Hernández-Losa J., Castellví J. Clinical implications of intratumor heterogeneity: challenges and opportunities. J. Mol. Med. 2020;98:161–177. 10.1007/s00109-020-01874-2. [Europe PMC free article] [Abstract] [CrossRef] [Google Scholar]
- Ribatti D., Tamma R., Annese T. Epithelial-mesenchymal transition in cancer: a historical overview. Transl. Oncol. 2020;13:100773. 10.1016/j.tranon.2020.100773. [Europe PMC free article] [Abstract] [CrossRef] [Google Scholar]
- Sarmiento-Salinas F.L., Delgado-Magallón A., Montes-Alvarado J.B., Ramírez-Ramírez D., Flores-Alonso J.C., Cortés-Hernández P., Reyes-Leyva J., Herrera-Camacho I., Anaya-Ruiz M., Pelayo R., et al. Breast cancer subtypes present a differential production of reactive oxygen species (ROS) and susceptibility to antioxidant treatment. Front. Oncol. 2019;9:480. [Europe PMC free article] [Abstract] [Google Scholar]
- Schindelin J., Arganda-Carreras I., Frise E., Kaynig V., Longair M., Pietzsch T., Preibisch S., Rueden C., Saalfeld S., Schmid B., et al. Fiji: an open-source platform for biological-image analysis. Nat. Methods. 2012;9:676–682. 10.1038/nmeth.2019. [Europe PMC free article] [Abstract] [CrossRef] [Google Scholar]
- Sciacovelli M., Frezza C. Metabolic reprogramming and epithelial-to-mesenchymal transition in cancer. FEBS J. 2017;284:3132–3144. 10.1111/febs.14090. [Europe PMC free article] [Abstract] [CrossRef] [Google Scholar]
- Shah A.T., Cannon T.M., Higginbotham J.N., Coffey R.J., Skala M.C. Autofluorescence flow sorting of breast cancer cell metabolism. J. Biophotonics. 2017;10:1026–1033. 10.1002/jbio.201600128. [Europe PMC free article] [Abstract] [CrossRef] [Google Scholar]
- Shen Y., Schmidt B.U.S., Kubitschke H., Morawetz E.W., Wolf B., Käs J.A., Losert W. Detecting heterogeneity in and between breast cancer cell lines. Cancer Converg. 2020;4:1. 10.1186/s41236-020-0010-1. [Europe PMC free article] [Abstract] [CrossRef] [Google Scholar]
- Shiraishi T., Verdone J.E., Huang J., Kahlert U.D., Hernandez J.R., Torga G., Zarif J.C., Epstein T., Gatenby R., McCartney A., et al. Glycolysis is the primary bioenergetic pathway for cell motility and cytoskeletal remodeling in human prostate and breast cancer cells. Oncotarget. 2015;6:130–143. 10.18632/oncotarget.2766. [Europe PMC free article] [Abstract] [CrossRef] [Google Scholar]
- Son H., Moon A. Epithelial-mesenchymal transition and cell invasion. Toxicol. Res. 2010;26:245–252. 10.5487/TR.2010.26.4.245. [Europe PMC free article] [Abstract] [CrossRef] [Google Scholar]
- Sullivan W.J., Mullen P.J., Schmid E.W., Flores A., Momcilovic M., Sharpley M.S., Jelinek D., Whiteley A.E., Maxwell M.B., Wilde B.R., et al. Extracellular matrix remodeling regulates glucose metabolism through TXNIP destabilization. Cell. 2018;175:117–132.e21. 10.1016/j.cell.2018.08.017. [Europe PMC free article] [Abstract] [CrossRef] [Google Scholar]
- Sun N.-Y., Yang M.-H. Metabolic reprogramming and epithelial-mesenchymal plasticity: opportunities and challenges for cancer therapy. Front. Oncol. 2020;10:792. 10.3389/fonc.2020.00792. [Europe PMC free article] [Abstract] [CrossRef] [Google Scholar]
- Sun X.x., Yu Q. Intra-tumor heterogeneity of cancer cells and its implications for cancer treatment. Acta Pharmacol. Sin. 2015;36:1219–1227. 10.1038/aps.2015.92. [Europe PMC free article] [Abstract] [CrossRef] [Google Scholar]
- Thejer B.M., Adhikary P.P., Kaur A., Teakel S.L., Van Oosterum A., Seth I., Pajic M., Hannan K.M., Pavy M., Poh P., et al. PGRMC1 phosphorylation affects cell shape, motility, glycolysis, mitochondrial form and function, and tumor growth. BMC Mol. Cell Biol. 2020;21:24. 10.1186/s12860-020-00256-3. [Europe PMC free article] [Abstract] [CrossRef] [Google Scholar]
- van Zijl F., Krupitza G., Mikulits W. Initial steps of metastasis: cell invasion and endothelial transmigration. Mutat. Res. 2011;728:23–34. 10.1016/j.mrrev.2011.05.002. [Europe PMC free article] [Abstract] [CrossRef] [Google Scholar]
- Vander Heiden M.G., Cantley L.C., Thompson C.B. Understanding the Warburg effect: the metabolic requirements of cell proliferation. Science. 2009;324:1029–1033. 10.1126/science.1160809. [Europe PMC free article] [Abstract] [CrossRef] [Google Scholar]
- Vaupel P., Schmidberger H., Mayer A. The Warburg effect: essential part of metabolic reprogramming and central contributor to cancer progression. Int. J. Radiat. Biol. 2019;95:912–919. 10.1080/09553002.2019.1589653. [Abstract] [CrossRef] [Google Scholar]
- Wang Y., Liu J., Ying X., Lin P.C., Zhou B.P. Twist-mediated epithelial-mesenchymal transition promotes breast tumor cell invasion via inhibition of hippo pathway. Sci. Rep. 2016;6:24606. 10.1038/srep24606. [Europe PMC free article] [Abstract] [CrossRef] [Google Scholar]
- Warburg O. The metabolism of carcinoma cells. J. Cancer Res. 1925;9:148–163. 10.1158/jcr.1925.148. [CrossRef] [Google Scholar]
- Ward P.S., Thompson C.B. Metabolic reprogramming: a cancer hallmark even Warburg did not anticipate. Cancer Cell. 2012;21:297–308. 10.1016/j.ccr.2012.02.014. [Europe PMC free article] [Abstract] [CrossRef] [Google Scholar]
- Zanotelli M.R., Goldblatt Z.E., Miller J.P., Bordeleau F., Li J., VanderBurgh J.A., Lampi M.C., King M.R., Reinhart-King C.A. Regulation of ATP utilization during metastatic cell migration by collagen architecture. Mol. Biol. Cell. 2018;29:1–9. 10.1091/mbc.E17-01-0041. [Europe PMC free article] [Abstract] [CrossRef] [Google Scholar]
- Zanotelli M.R., Rahman-Zaman A., VanderBurgh J.A., Taufalele P.V., Jain A., Erickson D., Bordeleau F., Reinhart-King C.A. Energetic costs regulated by cell mechanics and confinement are predictive of migration path during decision-making. Nat. Commun. 2019;10:4185–4212. 10.1038/s41467-019-12155-z. [Europe PMC free article] [Abstract] [CrossRef] [Google Scholar]
- Zhang J., Zhang W., Zhang T., Zhou Q., Liu J., Liu Y., Kong D., Yu W., Liu R., Hai C. TGF-β1 induces epithelial-to-mesenchymal transition via inhibiting mitochondrial functions in A549 cells. Free Radic. Res. 2018;52:1432–1444. 10.1080/10715762.2018.1500020. [Abstract] [CrossRef] [Google Scholar]
- Zhang X., Chan T., Mak M. Morphodynamic signatures of MDA-MB-231 single cells and cell doublets undergoing invasion in confined microenvironments. Sci. Rep. 2021;11:6529. 10.1038/s41598-021-85640-5. [Europe PMC free article] [Abstract] [CrossRef] [Google Scholar]
- Zhao J., Zhang J., Yu M., Xie Y., Huang Y., Wolff D.W., Abel P.W., Tu Y. Mitochondrial dynamics regulates migration and invasion of breast cancer cells. Oncogene. 2013;32:4814–4824. 10.1038/onc.2012.494. [Europe PMC free article] [Abstract] [CrossRef] [Google Scholar]
Articles from iScience are provided here courtesy of Elsevier
Full text links
Read article at publisher's site: https://doi.org/10.1016/j.isci.2022.105190
Read article for free, from open access legal sources, via Unpaywall:
http://www.cell.com/article/S2589004222014626/pdf
Citations & impact
Impact metrics
Citations of article over time
Alternative metrics

Discover the attention surrounding your research
https://www.altmetric.com/details/137048828
Article citations
Lactate-Induced HBEGF Shedding and EGFR Activation: Paving the Way to a New Anticancer Therapeutic Opportunity.
Cells, 13(18):1533, 13 Sep 2024
Cited by: 0 articles | PMID: 39329717 | PMCID: PMC11430493
Epithelial-mesenchymal plasticity in cancer: signaling pathways and therapeutic targets.
MedComm (2020), 5(8):e659, 01 Aug 2024
Cited by: 0 articles | PMID: 39092293 | PMCID: PMC11292400
Review Free full text in Europe PMC
Gedatolisib shows superior potency and efficacy versus single-node PI3K/AKT/mTOR inhibitors in breast cancer models.
NPJ Breast Cancer, 10(1):40, 05 Jun 2024
Cited by: 2 articles | PMID: 38839777 | PMCID: PMC11153628
Assessing the impact of extracellular matrix fiber orientation on breast cancer cellular metabolism.
Cancer Cell Int, 24(1):199, 05 Jun 2024
Cited by: 0 articles | PMID: 38840117
Characterizing heterogeneous single-cell dose responses computationally and experimentally using threshold inhibition surfaces and dose-titration assays.
NPJ Syst Biol Appl, 10(1):42, 18 Apr 2024
Cited by: 1 article | PMID: 38637530 | PMCID: PMC11026493
Go to all (10) article citations
Data
Data behind the article
This data has been text mined from the article, or deposited into data resources.
BioStudies: supplemental material and supporting data
Data Citations
- (2 citations) DOI - 10.5061/dryad.6djh9w149
Similar Articles
To arrive at the top five similar articles we use a word-weighted algorithm to compare words from the Title and Abstract of each citation.
Phenotypically sorted highly and weakly migratory triple negative breast cancer cells exhibit migratory and metastatic commensalism.
Breast Cancer Res, 25(1):102, 30 Aug 2023
Cited by: 6 articles | PMID: 37649089 | PMCID: PMC10468890
Phenotypic Heterogeneity and Metastasis of Breast Cancer Cells.
Cancer Res, 81(13):3649-3663, 11 May 2021
Cited by: 29 articles | PMID: 33975882 | PMCID: PMC9067366
Cyanidin-3-glucoside induces mesenchymal to epithelial transition via activating Sirt1 expression in triple negative breast cancer cells.
Biochimie, 162:107-115, 12 Mar 2019
Cited by: 14 articles | PMID: 30876970
Exploring the Metabolic Vulnerabilities of Epithelial-Mesenchymal Transition in Breast Cancer.
Front Cell Dev Biol, 8:655, 24 Jul 2020
Cited by: 14 articles | PMID: 32793598 | PMCID: PMC7393287
Review Free full text in Europe PMC
Funding
Funders who supported this work.
NIGMS NIH HHS (1)
Grant ID: R01 GM131178
National Institutes of Health (1)
Grant ID: GM131178
National Science Foundation (2)
Grant ID: 1937963
Grant ID: DGE-1650441