Open Access
ARTICLE
New Denial of Service Attacks Detection Approach Using Hybridized Deep Neural Networks and Balanced Datasets
1 L2IS, Department of Computer Sciences, Faculty of Sciences and Technology, Cadi Ayyad University, Marrakech, 40000, Morocco
2 Mathematics and Informatics Department, Royal Air School, Marrakech, 40000, Morocco
* Corresponding Author: Ouail Mjahed. Email:
Computer Systems Science and Engineering 2023, 47(1), 757-775. https://doi.org/10.32604/csse.2023.039111
Received 10 January 2023; Accepted 20 March 2023; Issue published 26 May 2023
Abstract
Denial of Service (DoS/DDoS) intrusions are damaging cyber-attacks, and their identification is of great interest to the Intrusion Detection System (IDS). Existing IDS are mainly based on Machine Learning (ML) methods including Deep Neural Networks (DNN), but which are rarely hybridized with other techniques. The intrusion data used are generally imbalanced and contain multiple features. Thus, the proposed approach aims to use a DNN-based method to detect DoS/DDoS attacks using CICIDS2017, CSE-CICIDS2018 and CICDDoS 2019 datasets, according to the following key points. a) Three imbalanced CICIDS2017-2018-2019 datasets, including Benign and DoS/DDoS attack classes, are used. b) A new technique based on K-means is developed to obtain semi-balanced datasets. c) As a feature selection method, LDA (Linear Discriminant Analysis) performance measure is chosen. d) Four metaheuristic algorithms, counting Artificial Immune System (AIS), Firefly Algorithm (FA), Invasive Weeds Optimization (IWO) and Cuckoo Search (CS) are used, for the first time together, to increase the performance of the suggested DNN-based DoS attacks detection. The experimental results, based on semi-balanced training and test datasets, indicated that AIS, FA, IWO and CS-based DNNs can achieve promising results, even when cross-validated. AIS-DNN yields a tested accuracy of 99.97%, 99.98% and 99.99%, for the three considered datasets, respectively, outperforming performance established in several related works.Keywords
Cite This Article
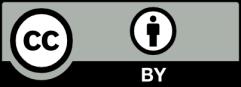
This work is licensed under a Creative Commons Attribution 4.0 International License , which permits unrestricted use, distribution, and reproduction in any medium, provided the original work is properly cited.