Open Access
ARTICLE
CD-FL: Cataract Images Based Disease Detection Using Federated Learning
1 Department of Computer Science, College of Computing, Khon Kaen University, Khon Kaen, 40002, Thailand
2 College of Computer Engineering and Sciences, Prince Sattam bin Abdulaziz University, Alkharj, Saudi Arabia
3 School of Telecommunication Engineering, Suranaree University of Technology, Nakhon Ratchasima, 30000, Thailand
4 Department of Computer Engineering and Networks, College of Computer and Information Sciences, Jouf University, Sakaka, 72388, Saudi Arabia
5 Information Systems Department, Faculty of Management, Comenius University in Bratislava, Odbojárov, Bratislava, 440, Slovakia
6 Faculty of Computers & Information Technology, Computer Science Department, University of Tabuk, Tabuk, 71491, Saudi Arabia
7 Department of Computer Science and Software Engineering, International Islamic University, Islamabad, 44000, Pakistan
* Corresponding Authors: Chitapong Wechtaisong. Email: ; Natalia Kryvinska. Email:
Computer Systems Science and Engineering 2023, 47(2), 1733-1750. https://doi.org/10.32604/csse.2023.039296
Received 21 January 2023; Accepted 13 April 2023; Issue published 28 July 2023
Abstract
A cataract is one of the most significant eye problems worldwide that does not immediately impair vision and progressively worsens over time. Automatic cataract prediction based on various imaging technologies has been addressed recently, such as smartphone apps used for remote health monitoring and eye treatment. In recent years, advances in diagnosis, prediction, and clinical decision support using Artificial Intelligence (AI) in medicine and ophthalmology have been exponential. Due to privacy concerns, a lack of data makes applying artificial intelligence models in the medical field challenging. To address this issue, a federated learning framework named CD-FL based on a VGG16 deep neural network model is proposed in this research. The study collects data from the Ocular Disease Intelligent Recognition (ODIR) database containing 5,000 patient records. The significant features are extracted and normalized using the min-max normalization technique. In the federated learning-based technique, the VGG16 model is trained on the dataset individually after receiving model updates from two clients. Before transferring the attributes to the global model, the suggested method trains the local model. The global model subsequently improves the technique after integrating the new parameters. Every client analyses the results in three rounds to decrease the over-fitting problem. The experimental result shows the effectiveness of the federated learning-based technique on a Deep Neural Network (DNN), reaching a 95.28% accuracy while also providing privacy to the patient’s data. The experiment demonstrated that the suggested federated learning model outperforms other traditional methods, achieving client 1 accuracy of 95.0% and client 2 accuracy of 96.0%.Keywords
Cite This Article
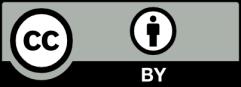
This work is licensed under a Creative Commons Attribution 4.0 International License , which permits unrestricted use, distribution, and reproduction in any medium, provided the original work is properly cited.