Abstract
Objectives
1) To determine the regional pattern of reduced N-acetylaspartate (NAA) in subcortical ischemic vascular dementia (SIVD); 2) to explore the relationship between NAA reduction and subcortical vascular disease; and 3) to test if MR spectroscopic imaging (MRSI) in combination with structural MRI improves differentiation between SIVD and Alzheimer disease (AD).Methods
Thirteen patients with SIVD (71 +/- 8 years old) and 43 patients with AD of comparable age and dementia severity were studied using MRSI and MRI. Patients were compared to 52 cognitively normal subjects with and without lacunes.Results
Compared to controls, patients with SIVD had lower NAA by 18% (p < 0.001) in frontal cortex and by 27% (p < 0.003) in parietal cortex, but no significant NAA reduction in white matter and medial temporal lobe. Compared to patients with AD, patients with SIVD had lower NAA by 13% (p < 0.02) in frontal cortex and by 20% (p < 0.002) in left parietal cortex. Cortical NAA decreased in SIVD with increasing white matter lesions (r = 0.54, p < 0.02) and number of lacunes (r = 0.59, p < 0.02). Thalamic lacunes were associated with greater NAA reduction in frontal cortex than were lacunes outside the thalamus (p < 0.02) across groups, after adjusting for cognitive impairments. Adding parietal NAA to MRI-derived hippocampal atrophy improved separation between SIVD and AD (p = 0.02) from 79 to 89%.Conclusions
These results emphasize the importance of cortical dysfunction as a factor in SIVD and indicate a characteristic pattern of metabolite change that might serve as a basis for improved diagnosis.Free full text

Different patterns of N-acetylaspartate loss in subcortical ischemic vascular dementia and AD
Abstract
Objectives
1) To determine the regional pattern of reduced N-acetylaspartate (NAA) in subcortical ischemic vascular dementia (SIVD); 2) to explore the relationship between NAA reduction and subcortical vascular disease; and 3) to test if MR spectroscopic imaging (MRSI) in combination with structural MRI improves differentiation between SIVD and Alzheimer disease (AD).
Methods
Thirteen patients with SIVD (71 ± 8 years old) and 43 patients with AD of comparable age and dementia severity were studied using MRSI and MRI. Patients were compared to 52 cognitively normal subjects with and without lacunes.
Results
Compared to controls, patients with SIVD had lower NAA by 18% (p < 0.001) in frontal cortex and by 27% (p < 0.003) in parietal cortex, but no significant NAA reduction in white matter and medial temporal lobe. Compared to patients with AD, patients with SIVD had lower NAA by 13% (p < 0.02) in frontal cortex and by 20% (p < 0.002) in left parietal cortex. Cortical NAA decreased in SIVD with increasing white matter lesions (r = 0.54, p < 0.02) and number of lacunes (r = 0.59, p < 0.02). Thalamic lacunes were associated with greater NAA reduction in frontal cortex than were lacunes outside the thalamus (p < 0.02) across groups, after adjusting for cognitive impairments. Adding parietal NAA to MRI-derived hippocampal atrophy improved separation between SIVD and AD (p < 0.02) from 79 to 89%.
Conclusions
These results emphasize the importance of cortical dysfunction as a factor in SIVD and indicate a characteristic pattern of metabolite change that might serve as a basis for improved diagnosis.
The contribution of subcortical ischemic vascular disease (SIVD) to cognitive impairment and dementia is poorly understood. Disruption of subcortical-cortical connections by strategically located infarctions is considered one important mechanism for cognitive impairment in SIVD.1 MRI studies from our group found that cognitive deficits in patients with SIVD were strongly correlated with brain atrophy, whereas subcortical infarctions made little contributions.2,3 Aside from greater numbers of ischemic lesions and more extensive white matter hyperintensities (WMH), other MRI studies comparing SIVD and Alzheimer disease (AD) have shown atrophy in the hippocampus and entorhinal cortex in both dementias, although less prominent in SIVD than in AD.4,5 Furthermore, some patients with SIVD without AD pathology confirmed by autopsy had reduced hippocampal and cortical gray matter volumes.2 Therefore, MRI has limited ability to differentiate between SIVD and AD.
In vivo proton magnetic resonance spectroscopic imaging (1H MRSI) can measure the regional distribution of N-acetylaspartate (NAA) in the brain. Because NAA is present at high concentration only in living neurons and is virtually undetectable in other cell types, such as glia,6 it is thought to be an indicator of neuronal density and neuronal metabolism.7 In AD, a 1H MRSI study found the most prominent NAA losses in parietal gray matter and in the hippocampus, but not in white matter.8 However, regional differences in NAA reductions between SIVD and AD have not been investigated thoroughly.
Therefore, the first aim of this study was to determine the regional pattern of NAA in gray matter, white matter, and WMH in SIVD. We hypothesized that SIVD would be associated with a different regional pattern of diminished NAA concentration than AD, because neuronal damage in SIVD would be related to subcortical vascular disease. The second aim of the study was to examine the relationship between subcortical vascular disease and cortical NAA reduction. Specifically, we predicted that NAA reduction in the frontal cortex would be associated with thalamic lacunes, because a large number of connections from thalamic nuclei project generally to zones in the frontal cortex.9,10 The third aim of this study was to test whether NAA measurements when added to MRI measurements of brain volumes improve discrimination between patients with SIVD and AD and control subjects.
Methods
Subjects
Participants with lacunes (as seen on MRI) included 13 demented patients with SIVD diagnosis, according to California Alzheimer’s Disease Diagnostic and Treatment Centers criteria11 (3 women, 10 men, mean age 71.5 ± 8.0 years, mean Mini-Mental State Examination12 score [MMSE] 20.5 ± 4.5); 11 patients with a diagnosis of AD (AD+L), according to National Institute of Neurological and Communicative Disorders and Stroke–Alzheimer’s Disease and Related Disorders Association criteria13 (6 women, 5 men, age 79.6 ± 5.6 years, MMSE 20.5 ± 4.5), and 16 cognitively normal subjects (CN+L) (6 women, 10 men, 75.6 ± 7.1 years, MMSE 28.9 ± 0.9). Participants without lacunes included 32 patients with AD (AD−L) (19 women, 13 men, 75.4 ± 6.0 years, MMSE 20.6 ± 5.1) and 36 cognitively normal subjects (CN−L) (19 women, 17 men, 73.7 ± 8.5 years, MMSE 29.0 ± 0.8). AD+L and AD−L patients in this study were selected from a larger patient group to match dementia severity and age range of the patients with SIVD. Similarly, CN+L and CN−L subjects were chosen from a cognitively normal cohort to match approximately the age distribution of patients. MRSI data of AD−L and CN−L subjects have been reported in part earlier.8 Patients with SIVD and AD were recruited from the Memory and Aging Clinic of the University of California at San Francisco (UCSF) and the Alzheimer Center of the University of California at Davis (UCD). The CN subjects were recruited from the community and received standard neurologic examinations at the same centers. CN subjects had cognitive test scores within the normal, age-adjusted range and no clinical history of alcoholism, psychiatric illness, epilepsy, hypertension, diabetes, major heart disease, or head trauma. Furthermore, a neuroradiologist evaluated the MRI data of all subjects for subcortical lacunar infarcts and, if present, counted their number by anatomic region. The definition of lacunar infarcts was based on original work by Fisher,14 combined with current clinical concepts. Evaluation was performed using axial proton density–weighted and coronal T1-weighted MR images. Focal areas of increased signal intensity in subcortical gray and white matter on proton density–weighted MRI measuring 5 to 15 mm in diameter were characterized as lacunes. The intensity on T1-weighted MRI may be variable. All areas of increased signal intensity on proton density MRI in the corona radiata and centrum semiovale were characterized as WMH, unless they exhibited discrete low intensity on T1-weighted MRI with a diameter range of 5 to 15 mm, in which case they were characterized as lacunes. All lesions complied with expected perforating vessel distribution.
Eleven patients (all AD) reported taking cholinesterase inhibitors and four patients (3 AD, 1 SIVD) anticholinergic drugs; two patients had incomplete records of medication. Medication information was collected at annual clinical visits, which were close to the time of MRI. Furthermore, one patient with SIVD and four patients with AD were given sedative (lorazepam 1 mg) to relax during MR scans. The committees of human research at UCSF, UCD, and the Veterans Affairs Medical Center in San Francisco approved the study, and written informed consent was obtained from each subject or a legal guardian before participation.
MRI and 1H MRSI acquisition and processing
Data acquisition and processing have been described in detail.15 In brief, MRI and 1H MRSI data were obtained in one session on a 1.5 T Siemens Vision System (Siemens Inc., Iselin, NJ). The protocol for structural MRI included axially oblique double spin echo (DSE) images with repetition time (TR)/echo time (TE)1/TE2 = 2500/20/80 msec, 1.0 × 1.4 mm2 inplane resolution, and 3-mm-thick sections with no section gap. In addition, volumetric magnetization-prepared rapid gradient echo (MPRAGE) images were acquired with TR/TE/inversion time (TI) = 10/7/300 msec, 15° flip angle, 1.0 × 1.0 mm2 inplane resolution, and 1.4-mm-thick coronal partitions, oriented approximately orthogonal to the long axis of the hippocampus. Proton density and T2-weighted images from DSE and T1-weighted images from MPRAGE were used together for tissue segmentation and T1-weighted images from MPRAGE were used for manual tracing of the hippocampus. The 1H MRSI protocol included a point resolved spectroscopy (PRESS) 1H MRSI16 sequence to acquire water suppressed 1H MR spectra simultaneously from the left and right hippocampal region and a multislice MRSI sequence for contiguous sampling of large regions in the cortex, which is difficult to accomplish with the box-like volume selection of PRESS. The timing of both PRESS and multi-slice MRSI was TR/TE = 1800/135 msec. The nominal spatial resolution of PRESS was 8.5 × 8.5 mm2 inplane with a 15-mm-thick section, aligned approximately along the long axis of the hippocampus, and the nominal spatial resolution of multislice MRSI was 7.8 × 7.8 mm2 with two 15-mm-thick axially oblique sections to cover frontal and parietal lobes. Acquisitions of structural MRI and 1H MRSI, including shimming and adjustments, required together about 90 minutes.
Volumes of left and right hippocampus were measured by drawing the boundaries manually on coronal T1-weighted MR images.17 Tissue segmentation of MRI data into gray matter, white matter, and CSF was achieved automatically with software developed inhouse.18 Additional operator-assisted segmentation classified gray matter into cortical gray matter and subcortical gray matter and white matter into normal-appearing white matter and WMH. Furthermore, the interhemispheric fissure and the central sulcus were marked on MR images to determine the boundaries between the right and left cerebral hemispheres and frontal and parietal lobes for coanalysis with MRSI. Finally, the masks of the left and right hippocampus from manual tracing were also incorporated into the segmentation data. The raters were blinded to all clinical information. Consistency of manual ratings (expressed as a coefficient of variation) was about 1.0% for hippocampus and better than 2% for tissue segmentation.
Spectral processing and MRI–MRSI coanalysis
After Fourier transformation of 1H MRSI, the peak areas of NAA, creatine (Cr), and choline (Cho) containing compounds were estimated using fully automated spectral fitting software developed inhouse.19 Metabolite concentrations (denoted [NAA], [Cho], and [Cr]) were obtained separately for gray matter and white matter of the left and right, frontal and parietal lobes, first by estimating the tissue composition of each MRSI voxel based on segmented MRI data coregistered to MRSI, and second by regressing metabolite intensities against tissue composition of MRI voxels. This allowed estimation of metabolite concentrations of “pure” gray matter and white matter.15 Spurious signal contributions from neighboring regions, such as subcortical gray matter or occipital lobe, were accounted for by screening out voxels from these regions. Similarly, metabolite concentrations of hippocampus and WMH were determined by selecting MRSI voxels, which contained mainly hippocampal or WMH tissue, and by correcting for partial volumes of CSF.20 Because metabolite signals were not corrected for T1 and T2 relaxation, concentrations in this study were expressed in arbitrary units rather than in millimoles/liter. In analyzing MRSI data, two patients with SIVD and three patients with AD had corrupt multislice MRSI data, mainly because of movements between MRI and MRSI acquisitions, and were therefore not included in analyses that involved frontal and parietal lobes. PRESS MRSI data from all subjects had acceptable quality and no exclusions were necessary in analyses that involved medial temporal lobe.
Statistical analysis
Effects of diagnosis on regional variations of metabolite concentrations were tested using multivariate analysis of variance (MANOVA), followed by post hoc univariate ANOVA tests to determine differences between groups. The dependent variables were metabolite concentrations and the independent variables were regions and diagnosis. Age and sex were added as covariate when appropriate. To reduce the probability of finding regional effects on metabolites by chance, the level of significance was raised to α = 0.05/3 ≈ 0.02 (based on three independent tests for gray matter, white matter, and hippocampus), implementing Bonferroni correction for multiple comparisons. Tests for WMH were not counted as multiple comparisons, because they did not involve regions. To enable comparisons between PRESS and multislice MRSI data irrespectively of experimental differences, metabolite concentrations obtained with each method were transformed into z-scores, according to zMr = (Mr − mean(Mr CN))/stdev(Mr CN). Here, zMr represents a patient’s z-score of metabolite M in a brain region r (r = hippocampus, frontal or parietal, left or right gray matter or white matter and mean(Mr CN) are mean values and stdev(Mr CN) standard deviations in each brain region in CN subjects. Classification of AD, SIVD, and CN subjects based on MRI and MRSI was tested using logistic regressions with MRI and MRSI measures predicting diagnosis. Furthermore, receiver operator characteristics (ROC) analysis was performed to provide a means of comparing MRI and MRSI classifiers in terms of sensitivity and specificity. Group values are listed as means and standard deviations, unless otherwise noted.
Results
Table 1 lists the demographic data of the subjects as well as the load of cerebrovascular disease in each group, evaluated by WMH and number of lacunes. Patients with SIVD were not markedly younger than the other groups (F = 2.2, df = 4,103, p < 0.08). MMSE scores of patients were comparable between AD and SIVD (F = 0.02, df = 2,53, p > 0.9), but substantially lower than the scores of CN subjects (F = 147, df = 1,106, p < 0.001), as expected. CN subjects with and without lacunes did not differ with respect to MMSE scores (F = 0.2, df = 1,50, p > 0.6). Sex was reasonably balanced in the AD and CN groups (all p > 0.5 by χ2 tests), but women were under-represented in the SIVD group (p = 0.03).
Table 1
Subject demographics
Parameters | SIVD | AD+L | AD+L | CN+L | CN+L |
---|---|---|---|---|---|
Lacunes on MRI | Yes | Yes | No | Yes | No |
Number of subjects | 13 | 11 | 32 | 16 | 36 |
Women/men | 3/10 | 6/5 | 19/13 | 6/10 | 19/17 |
Age, y | 71.5 ± 8.0 | 79.6 ± 5.6 | 75.4 ± 6.0 | 75.6 ± 7.1 | 73.7 ± 8.5 |
Age range, y | 56–81 | 63–88 | 63–85 | 63–85 | 56–88 |
MMSE | 20.5 ± 4.5 | 20.9 ± 4.9 | 20.6 ± 5.1 | 28.9 ± 0.9 | 29.0 ± 0.8 |
WMH | 7.2 ± 6.9 | 6.5 ± 5.3 | 3.1 ± 3.0 | 2.3 ± 1.4 | 1.8 ± 2.2 |
Lacune number | 3.3 ± 1.8 | 1.1 ± 0.6 | 0 | 2.9 ± 2.4 | 0 |
SIVD = subcortical ischemic vascular dementia; AD = Alzheimer’s disease; +L = presence of lacunes; −L = absence of lacunes; CN = cognitive normal; MMSE = Mini-Mental State Examination; WMH = white matter hyperintensities, expressed in percent of normal-appearing white matter.
In analyzing MRSI, we first determined whether metabolite differences between AD+L and AD−L patients and between CN+L and CN−L subjects needed to be considered. MANOVA tests indicated that there were no differences in MRSI data between AD+L and AD−L (p > 0.5) and between CN+L and CN−L (p > 0.9). Therefore, comparisons between SIVD and the other groups were performed without differentiating between individuals with and without lacunes. Table 2 lists [NAA] concentrations (in arbitrary units) in medial temporal lobe, including the hippocampus, in SIVD, AD, and CN. [NAA] losses in medial temporal lobe were strongly associated with diagnosis (p < 0.001). The effect remained significant after accounting for potential bias due to undersampling of small hippocampi, stemming from partial volume limitations of MRSI. However, 11% lower [NAA] in medial temporal lobe of patients with SIVD compared to CN subjects was not significant in post hoc tests to explain the main effect of diagnosis. In contrast, 21% lower [NAA] in patients with AD compared to CN subjects explained the main effect of diagnosis on medial temporal lobe [NAA] (left: F = 19.9, df = 2,82, p < 0.001; right: F = 29.6, df = 2,82, p < 0.001). Despite substantial [NAA] loss in AD but moderate [NAA] loss in SIVD relative to CN, differences between patients with AD and patients with SIVD in medial temporal lobe were not significant. For the numbers of patients tested and 80% power, the minimum detectable difference for [NAA] in medial temporal lobe was 16%.
Table 2
[NAA] of medial temporal lobe, including the hippocampus, and of frontal and parietal lobes
Lobes | Side | Tissue | SIVD | AD | CN |
---|---|---|---|---|---|
Temporal | Left | Undiff. | 2.08 ± 0.51 | 1.87 ± 0.37* | 2.32 ± 0.41 |
Right | Undiff. | 2.00 ± 0.53 | 1.81 ± 0.39* | 2.34 ± 0.39 | |
Frontal | Left | Gray | 0.83 ± 0.21†‡ | 0.94 ± 0.17 | 1.00 ± 0.16 |
Right | Gray | 0.79 ± 0.22†‡ | 0.93 ± 0.16 | 0.99 ± 0.18 | |
Parietal | Left | Gray | 0.67 ± 0.17†‡ | 0.84 ± 0.21* | 0.98 ± 0.18 |
Right | Gray | 0.77 ± 0.29† | 0.90 ± 0.21* | 1.03 ± 0.23 |
[NAA] is in arbitrary units. Values of medial temporal lobe should not be compared directly to frontal and parietal lobes, because signal calibration differs.
SIVD = subcortical ischemic vascular dementia; AD = Alzheimer’s disease; CN = cognitive normal; undiff. = undifferentiated.
Outside the medial temporal lobe in frontal and parietal lobe regions, cortical [NAA] was also associated with diagnosis (p < 0.003). [NAA] concentrations of frontal and parietal lobes in the different groups are listed in table 2. Compared to CN, patients with SIVD had about 18% lower cortical [NAA] in the frontal lobe (left: F = 10.7, df = 2,62, p < 0.002; right: F = 10.3, df = 2,62, p < 0.002) and about 27% lower cortical [NAA] in the parietal lobe (left: F = 36.2, df = 2,62, p < 0.0001; right: F = 12.6, df = 2,62, p < 0.001). This contrasts with results from the medial temporal lobe, where no significant [NAA] differences between SIVD and CN subjects were found. Compared to AD, patients with SIVD had about 13% lower cortical [NAA] in both left and right frontal lobes (F = 6.0, df = 2,50, p < 0.02) and, in addition, 20% lower cortical [NAA] in the left parietal lobe (F = 10.9, df = 2,50, p < 0.002), whereas differences in the right parietal cortex were not significant. In contrast to SIVD, patients with AD had no cortical [NAA] loss in the frontal lobe (both left and right p > 0.1) when compared to CN subjects. In the parietal lobe, however, patients with AD had—like SIVD—about 13% lower cortical [NAA] than CN subjects (left: F = 7.2, df = 2,91, p < 0.01; right: F = 5.6, df = 2,91, p < 0.01). [NAA] of white matter was not different between groups (all p > 0.2). For the numbers of subjects tested and 80% power, the minimum detectable difference of [NAA] in white matter was 13%.
Neither [Cho] nor [Cr] of medial temporal lobe was related to diagnosis (p > 0.4). Outside the medial temporal lobe, there was a trend for lower cortical [Cho] (p = 0.03) as a function of diagnosis. This was driven by lower cortical [Cho] in the left parietal lobe in SIVD relative to AD (F = 10.6, df = 2,42, p < 0.002) and to CN (F = 13.1, df = 2,53, p < 0.001) (data not shown). Variations of cortical [Cr] were not related with diagnosis.
We also tested [NAA], [Cho], and [Cr] of WMH and normal-appearing white matter for differences in 11 patients with SIVD, 17 patients with AD, and 11 CN subjects, who had WMH that were large enough to place MRSI voxels (approximately 1 cm3 volume). There was no significant effect of diagnosis on [NAA] variations in WMH. Across groups, however, [NAA] in WMH was lower by about 15% (F = 11.3, df = 2,37, p < 0.005) than in normal-appearing white matter. There was also no significant effect of diagnosis on [Cho] and [Cr] variations in WMH and neither [Cho] nor [Cr] differed significantly between WMH and normal-appearing white matter.
To test whether AD and SIVD are associated with different regional patterns of [NAA] loss, we transformed [NAA] values into z-scores to account for systematic technical differences between PRESS and multislice MRSI, such as different point spread functions and different T1 weighting. Z-scores of [NAA] in patients with SIVD and patients with AD are listed in table 3 for various brain regions. In SIVD, cortical [NAA] was lower in the frontal and parietal lobes compared to the medial temporal lobe (F = 3.4, df = 2,113, p < 0.05). In contrast to SIVD, patients with AD had lower cortical [NAA] in the medial temporal lobe than in the frontal and parietal lobes (F = 4.6, df = 2,113, p < 0.02). For comparison, z-scores of MRI derived volumes of hippocampus and cortical gray matter in these patients are also listed in table 3.
Table 3
[NAA] z-scores of medial temporal lobe and parietal and frontal lobe gray matter in SIVD and AD and z-scores of MRI-derived volumes for comparison
Z-scores | SIVD | AD |
---|---|---|
[NAA] | ||
![]() | −0.4 ± 0.8 | −1.0 ± 0.6† |
![]() | −1.1 ± 0.8‡ | −0.5 ± 0.8 |
![]() | −1.7 ± 0.8‡ | −0.9 ± 0.9 |
Volume§ | ||
![]() | −1.2 ± 0.9 | −2.1 ± 1.2 |
![]() | −1.8 ± 1.2 | −1.4 ± 0.9 |
SIVD = subcortical ischemic vascular dementia; AD = Alzheimer’s disease.
According to our hypothesis that subcortical vascular disease would cause damage to cortical neurons by disrupting subcortical-cortical connections, we expected that cortical [NAA] losses should be related to the extent of WMH and subcortical infarctions, independent of cognitive status. As predicted, cortical [NAA] losses in patients with SIVD, averaged across the frontal and parietal lobe, were correlated with increased WMH volumes (r = −0.54, p < 0.02) and lacune numbers (r = −0.59, p < 0.02). Similarly, cortical [NAA] losses in CN subjects were also correlated with increased WMH volumes (r = −0.31, p > 0.02) and lacune numbers (r = −0.65, p < 0.02). Figure 1 illustrates the relationships between cortical [NAA] loss and WMH or lacunes, separately for patients with SIVD and CN subjects. Patients with AD, in contrast, showed no correlation between cortical [NAA] and WMH (r = −0.22, p > 0.05). However, power to detect a correlation of about 0.2 was not above chance with this number of patients. Because patients with AD had fewer than two lacunes by definition, correlations between cortical [NAA] and lacunes were not tested for AD. To explore further the idea that lacunar infarctions in strategic subcortical regions induce damage to remote cortical neurons, we tested whether [NAA] loss in the frontal cortex would be related to thalamic lacunes. This analysis was performed with data from SIVD, AD, and CN groups pooled. Using presence or absence of thalamic lacunes as criterion to dichotomize subjects with at least one lacune and accounting for MMSE differences between the groups, we found that thalamic lacunes were associated with 16% lower [NAA] (F = 6.7, df = 2,31, p < 0.02) in the frontal cortex but not in the parietal cortex (F = 2.2, df = 2,31, p > 0.1) compared to lacunes outside the thalamus. The association between cortical [NAA] and thalamic lacunes is shown in figure 2.
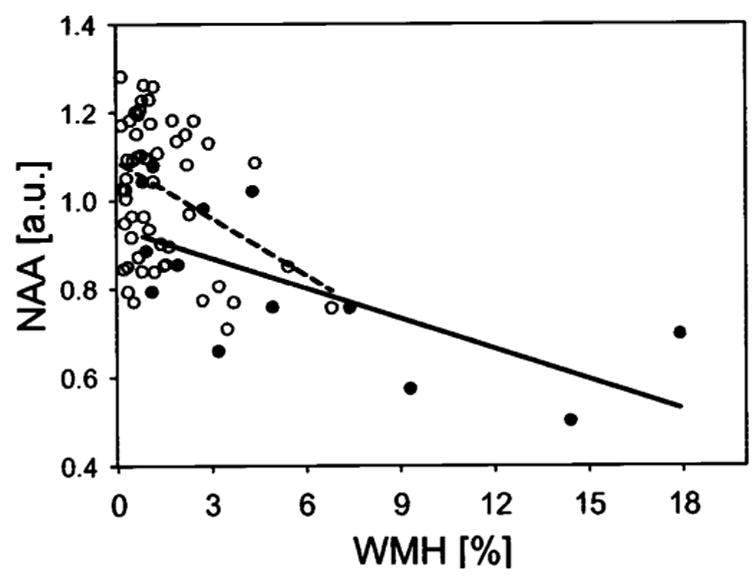
Relationship between cortical [NAA] and subcortical vascular disease, assessed by white matter hyperintensities (WMH) and number of lacunes in patients with subcortical ischemic vascular disease (●) and cognitively normal subjects (○). WMH volume was indexed to percent of normal white matter and [NAA] is represented in arbitrary units (au).
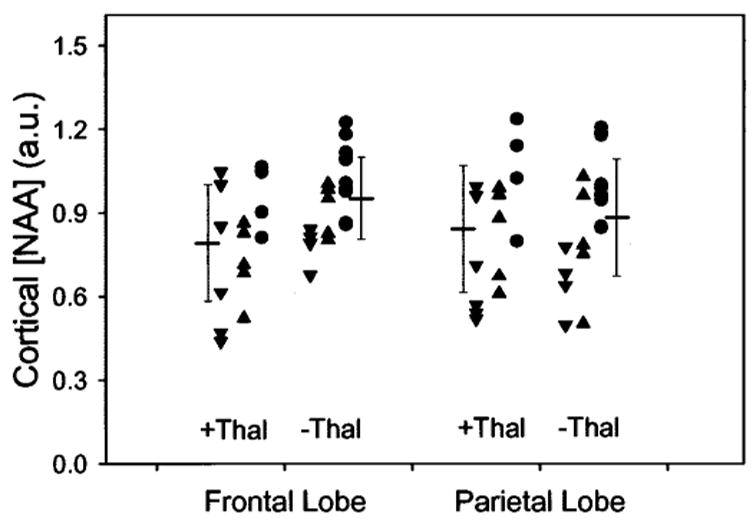
Cortical [NAA] of frontal and parietal lobe as a function of presence (+Thal) or absence (−Thal) of thalamic lacunes in subjects with subcortical infarctions. Also indicated are mean values and 1.0 standard deviations for each subgroup. Data from patients with subcortical ischemic vascular disease (), patients with Alzheimer disease (▲), and cognitively normal subjects (●) with lacunes are pooled.
Finally, we tested the extent to which [NAA] measurements added to MRI volume measurements improve differentiation between SIVD and AD. Results are summarized in table 4. Using logistic regression to predict diagnosis, we first entered hippocampal volume into the regression model, because the hippocampus yielded the largest difference of z-scores between patients with SIVD and patients with AD (z = −1.2 in table 3). The contribution of hippocampal volume in predicting diagnosis was substantial (p = 0.005), achieving an overall classification of 79% with specificity for SIVD of 92%, but sensitivity of only 36%. The area under the ROC curve was 0.83. Adding [NAA] of parietal cortex, which had the second largest z-score difference (−1.7 vs −0.9), improved overall correct classification from 79% to 89% (p = 0.02). This was primarily due to an increase for SIVD in sensitivity from 36% to 73% and a slight increase in specificity from 92% to 94%. Consequently, the area under the ROC curve also increased from 0.83 to 0.92. The scatter plot in figure 3 depicts the separation between patients with SIVD and patients with AD with hippocampal volume and [NAA] of parietal cortex. Adding more measures, such as WMH volume, gray matter volume, or frontal lobe cortical [NAA], yielded no further significant improvement. To test separation of patients with SIVD from CN subjects, we first entered cortical gray matter volumes from MRI into the regression model, because this measure had the largest z-score for SIVD (−1.8 according to table 3). The contribution from gray matter volume in predicting group was significant and yielded for SIVD an overall correct classification of 88% with 55% sensitivity and 96% specificity and an area under the ROC curve of 0.88. Adding [NAA] of parietal cortex or other measures did not further improve classification (all p > 0.2).
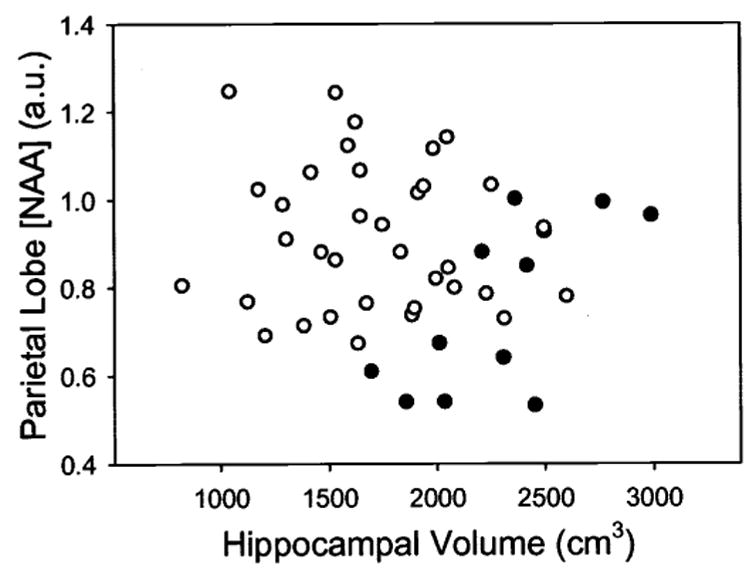
Discrimination between patients with subcortical ischemic vascular disease (●) and Alzheimer disease (○) by MRI-derived hippocampal volume and magnetic resonance spectroscopic imaging–derived cortical [NAA] of parietal lobe.
Table 4
Separation of SIVD from AD and CN based on MRI-derived atrophy alone and with addition of MRSI-derived [NAA]
Classification | Statistics | Hippocampal atrophy | Hippocampal atrophy and [NAA]* |
---|---|---|---|
SIVD from AD | p † | 0.005 | 0.02 |
Classification† | 79% | 89% | |
Sensitivity† | 36% | 73% | |
Specificity† | 92% | 94% | |
AUC-ROC | 0.83 | 0.92 | |
Gray matter atrophy | Gray matter atrophy and [NAA]* | ||
SIVD from CN | p † | 0.001 | Not significant |
Classification† | 88% | 89% | |
Sensitivity† | 55% | 55% | |
Specificity† | 96% | 98% | |
AUC-ROC | 0.88 | 0.90 |
SIVD = subcortical ischemic vascular dementia; AD = Alzheimer’s disease; CN = cognitive normal; MRSI = magnetic resonance spectroscopic imaging; AUC-ROC = area under the curve of a receiver operator characteristics analysis.
Discussion
[NAA] reductions predominantly in frontal and parietal gray matter in patients with SIVD, while sparing white matter and medial temporal lobe, are consistent with findings from several previous MRSI reports on SIVD.21–24 However, previous MRSI studies sampled data from only a few, manually selected brain regions and were confounded by gray/white matter partial volumes, potentially introducing a sampling bias. The current study, utilizing all 1H MRSI voxels in brain slices for analysis and regressing signal variations against voxel tissue composition, obtained metabolite intensities differentially for “pure” gray matter and white matter, greatly reducing complications from partial volume sampling. Therefore, findings from this study establish more firmly than before that cortical [NAA] reductions in SIVD were independent of morphologic brain changes and, furthermore, that regional [NAA] differences between SIVD and AD are not simply a sampling artifact. Although some [NAA] losses in the temporal and parietal cortex in patients with SIVD could be explained by concomitant AD, this does not explain [NAA] losses in the frontal cortex in SIVD, because AD was not associated with [NAA] losses in the frontal brain. Another important finding in SIVD was that [NAA] losses in cortical regions correlated with subcortical infarction load and WMH, implying that subcortical vascular disease was responsible for cortical changes in SIVD. There are several possible explanations for this finding. First, and most likely, is that subcortical infarctions cause functional deafferentation of the cerebral cortex, sometimes termed subcortical-cortical diaschisis. This is consistent with PET studies showing in SIVD hypometabolism and hypoperfusion in cortical regions,25,26 especially in the frontal lobe.27 In support of this view, MRS studies on animals have shown trans-synaptic decrease of NAA levels following acute deafferentation without neuronal loss.28 It is therefore conceivable that the cortical [NAA] losses in SIVD could indicate deafferented neurons in a state of functional inactivity with a possibility for recovery rather than frank neuron loss. A second explanation is that neurons are damaged or lost via transneuronal degeneration, secondary to subcortical infarctions.29 With the assumption that [NAA] reflects neuron density, the results imply further that a secondary degeneration causes disproportionately greater loss of neuronal than non-neuronal cells.
A third possibility is that cortical [NAA] reductions are due to cortical ischemia, with or without microinfarctions in the cortex, undetectable with MRI. There are two arguments against this view. First, quite remarkably, we found no [NAA] reduction in white matter in SIVD (or in AD). It would seem reasonable that a widespread ischemic process, which affected cortex and caused subcortical infarctions and WMH, would also produce [NAA] reduction in white matter. Second, [NAA] reductions can occur preferentially in the frontal lobe, as the comparison between subjects with and without thalamic lacunes showed. In presence of a generalized ischemic process, however, one would expect that the entire cortex would be affected, not that the frontal region would be singled out. Eventually, it will be necessary to obtain autopsy information to exclude microinfarctions and concurrent AD as potential cause of cortical [NAA] reduction in SIVD.
The finding of unchanged [NAA] in normal-appearing white matter in SIVD, while [NAA] was reduced in the cortex and regions of WMH, is surprising because the predominant disease process in these patients is thought to be subcortical. In another quantitative MRSI study of SIVD,24 which used analysis of a few voxels of interest, we reported reduced [NAA] of white matter. However, this result was not independent of spurious contributions from [NAA] of WMH in SIVD, which is difficult to correct if only a small number of voxels is sampled. Our finding that the addition of parietal [NAA] to MRI measurements of hippocampal volume improved differentiation between SIVD and AD may be of clinical importance as treatments for the two disorders will be likely different. However, an overlap between the groups remained whether cortical [NAA] and hippocampal volume were used individually or together. Therefore, this will not likely lead to individual patient diagnosis, unless more information is incorporated, such as MRS measurements of myo-inositol30 or topologic measurements of brain atrophy.31–33
A major limitation of this study is that we used clinical and not autopsy data for patient classification, because it is generally recognized that an accurate prediction whether a patient has AD or SIVD is difficult to make antemortem. So far, autopsy data are available from only five patients with AD and three control subjects, but not yet from patients with SIVD. Two of the five autopsied patients were confirmed AD cases, whereas one had diffuse Lewy body disease and two had mixed AD/SIVD pathology, indicating the complications with accurate antemortem diagnoses. Thus, some patients with SIVD in this study may have had concurrent AD pathology, and some of the patients with AD likely had vascular disease and may have lacked AD pathology. Nevertheless, the finding of a different regional pattern of [NAA] loss in SIVD compared to AD is emphasized by the possibility of such misclassifications, because the ability to distinguish patterns between groups is reduced by misdiagnosis of subjects. There are several other limitations. First, a few patients with AD were taking cholinesterase inhibitors and anticholinergic drugs that could have altered brain metabolite levels, potentially enhancing metabolite differences between AD and SIVD. Secondly, relaxation measurements could not be performed owing to prohibitively long acquisition times. Therefore, [NAA] reduction in SIVD could be due in part to T2/T1 changes rather than to reduced concentration. Finally, detection of cortical atrophy and lacunes was compromised, because tissue segmentation relied on T2-weighted MR images with relatively low resolution (4.2 mm3). It is possible that different results would be obtained if tissue segmentation was performed with higher resolution.
Acknowledgments
The authors thank Marybeth Kedzior, Megan Bradley, Meera Krishnan, and Diana Sacrey for data processing and Sean Steinman for scanning most of the patients.
Footnotes
Supported in part by a research applications grant of the Department of Veterans Affairs REAP and by NIH grants AG10897 and AG12435.
References
Full text links
Read article at publisher's site: https://doi.org/10.1212/01.wnl.0000078942.63360.22
Read article for free, from open access legal sources, via Unpaywall:
https://europepmc.org/articles/pmc1820863?pdf=render
Citations & impact
Impact metrics
Citations of article over time
Article citations
Proposing a "Brain Health Checkup (BHC)" as a Global Potential "Standard of Care" to Overcome Reward Dysregulation in Primary Care Medicine: Coupling Genetic Risk Testing and Induction of "Dopamine Homeostasis".
Int J Environ Res Public Health, 19(9):5480, 30 Apr 2022
Cited by: 8 articles | PMID: 35564876 | PMCID: PMC9099927
Discriminating subcortical ischemic vascular disease and Alzheimer's disease by diffusion kurtosis imaging in segregated thalamic regions.
Hum Brain Mapp, 42(7):2018-2031, 08 Jan 2021
Cited by: 8 articles | PMID: 33416206 | PMCID: PMC8046043
Effects of Alterations of Post-Mortem Delay and Other Tissue-Collection Variables on Metabolite Levels in Human and Rat Brain.
Metabolites, 10(11):E438, 29 Oct 2020
Cited by: 13 articles | PMID: 33138273 | PMCID: PMC7694048
Proton Magnetic Resonance Spectroscopy in Common Dementias-Current Status and Perspectives.
Front Psychiatry, 11:769, 06 Aug 2020
Cited by: 11 articles | PMID: 32848938 | PMCID: PMC7424040
Review Free full text in Europe PMC
Multimodality Imaging Approaches in Alzheimer's disease. Part II: 1H MR spectroscopy, FDG PET and Amyloid PET.
Dement Neuropsychol, 9(4):330-342, 01 Oct 2015
Cited by: 4 articles | PMID: 29213982 | PMCID: PMC5619315
Review Free full text in Europe PMC
Go to all (37) article citations
Similar Articles
To arrive at the top five similar articles we use a word-weighted algorithm to compare words from the Title and Abstract of each citation.
Regional gray and white matter metabolite differences in subjects with AD, with subcortical ischemic vascular dementia, and elderly controls with 1H magnetic resonance spectroscopic imaging.
Arch Neurol, 53(2):167-174, 01 Feb 1996
Cited by: 56 articles | PMID: 8639067 | PMCID: PMC2733342
Subcortical ischemic vascular dementia: assessment with quantitative MR imaging and 1H MR spectroscopy.
AJNR Am J Neuroradiol, 21(4):621-630, 01 Apr 2000
Cited by: 33 articles | PMID: 10782769 | PMCID: PMC1945115
Effects of subcortical ischemic vascular dementia and AD on entorhinal cortex and hippocampus.
Neurology, 58(11):1635-1641, 01 Jun 2002
Cited by: 78 articles | PMID: 12058091 | PMCID: PMC1820858
1H-MRS evaluation of metabolism in Alzheimer's disease and vascular dementia.
Neurol Res, 26(5):488-495, 01 Jul 2004
Cited by: 55 articles | PMID: 15265265
Review
Funding
Funders who supported this work.
NIA NIH HHS (4)
Grant ID: AG10897
Grant ID: AG12435
Grant ID: R01 AG010897
Grant ID: P01 AG012435