Abstract
Free full text

UniFrac: a New Phylogenetic Method for Comparing Microbial Communities
Abstract
We introduce here a new method for computing differences between microbial communities based on phylogenetic information. This method, UniFrac, measures the phylogenetic distance between sets of taxa in a phylogenetic tree as the fraction of the branch length of the tree that leads to descendants from either one environment or the other, but not both. UniFrac can be used to determine whether communities are significantly different, to compare many communities simultaneously using clustering and ordination techniques, and to measure the relative contributions of different factors, such as chemistry and geography, to similarities between samples. We demonstrate the utility of UniFrac by applying it to published 16S rRNA gene libraries from cultured isolates and environmental clones of bacteria in marine sediment, water, and ice. Our results reveal that (i) cultured isolates from ice, water, and sediment resemble each other and environmental clone sequences from sea ice, but not environmental clone sequences from sediment and water; (ii) the geographical location does not correlate strongly with bacterial community differences in ice and sediment from the Arctic and Antarctic; and (iii) bacterial communities differ between terrestrially impacted seawater (whether polar or temperate) and warm oligotrophic seawater, whereas those in individual seawater samples are not more similar to each other than to those in sediment or ice samples. These results illustrate that UniFrac provides a new way of characterizing microbial communities, using the wealth of environmental rRNA sequences, and allows quantitative insight into the factors that underlie the distribution of lineages among environments.
Sequencing of 16S rRNA genes from environmental samples has revealed that microbial diversity is far more extensive than had ever been imagined from studies of cultured microorganisms alone and that microorganisms represent the majority of the phylogenetic diversity of life on earth (34). Culture-independent studies of microbial populations were pioneered in the Pace lab in 1985 (35, 36); the technique is now so prevalent that an estimated 151,339 sequences from small-subunit-rRNA environmental clones had been deposited in GenBank as of 1 August 2005. [We estimated the total number of environmental clone small-subunit-rRNA gene sequences published in GenBank with an Entrez search with the string “(SSU OR 16S OR 18S OR small subunit) AND (rRNA OR rDNA OR ribosomal RNA) AND (uncult* OR unidentified OR unknown)” (modified from reference 37).] Only half of the 52 major bacterial lineages described in the last comprehensive review have cultivated representatives, and widespread, numerically dominant phylotypes are often only distantly related to culturable strains (37). Thus, our sole source of information about the biology of much of the diversity of life is the environmental distribution of sequences.
Several statistical techniques have been developed to use environmental 16S rRNA clone sequences to compare microbial communities between samples. Unfortunately, many of these techniques are limited because they do not account for the different degrees of similarity between sequences. Sequences are usually grouped if their 16S rRNA genes are 95 to 99% identical (16, 30); with a cutoff of 98%, such techniques would treat sequences with 3% and 40% sequence divergence equally. This results in a substantial loss of information since 16S rRNA and phenotypic variances are positively correlated (33). Techniques with this limitation include the Sørenson and Jaccard indices of group overlap (28), the LibShuff (40; http://www.arches.uga.edu/~whitman/libshuff.html) and ∫-LibShuff (39; http://www.plantpath.wisc.edu/fac/joh/S-LibShuff.html) methods, and hierarchical clustering and ordination of samples based on the distribution of sequences belonging to different groups (17).
Phylogenetic distance measures can provide far more power because they exploit the degree of divergence between different sequences. Two phylogenetic approaches that assess whether communities differ significantly in composition, the P and FST tests, have recently been developed (30). The P test uses parsimony to determine whether the distribution of modern sequences in different environments reflects a history of fewer changes between environments than would be expected by chance. The FST test identifies cases where more sequence variation exists between two communities than within a single community. Although these techniques greatly increase our ability to test for differences between pairs of communities, they have only been applied to determining whether samples are significantly different and have not been used to compare many samples simultaneously with clustering or ordination techniques. In addition, neither measure accounts for branch length information when comparing samples.
Here we introduce a new phylogenetic method, called UniFrac, that measures the distance between communities based on the lineages they contain. UniFrac can be used to compare many samples simultaneously because it satisfies the technical requirements for a distance metric (it is always positive, is transitive, and satisfies the triangle inequality) and can thus be used with standard multivariate statistics such as unweighted-pair group method using average linkages (UPGMA) clustering (9) and principal coordinate analysis (23). Similarly, UniFrac is more powerful than nonphylogenetic distance measures because it exploits the different degrees of similarity between sequences. To demonstrate the utility of the UniFrac metric for comparing multiple community samples and determining the factors that explain the most variation, we compared bacterial populations in different types of geographically dispersed marine environments.
Small-subunit-rRNA gene surveys have been performed in many marine environments, including oligotrophic open- ocean (11), coastal temperate (1, 22) and polar (2, 8) seawater, polar sea ice (3, 6, 7), and marine sediments (4, 5, 25, 38). Comparing this range of samples using the UniFrac technique provides a coherent picture of the distribution of bacterial lineages that provides a context for many individual published observations while allowing us to test specific ideas about the distribution of bacterial lineages. In particular, we asked the following questions.
How does culturing affect similarities between samples?
Few organisms in environmental samples are culturable, but it is unknown whether the cultured isolates from an environment yield communities that resemble the communities from the original habitat. We addressed this issue by comparing gene libraries from both cultured bacteria and uncultured environmental samples of marine ice, water, and sediment to test whether the cultured samples appeared more similar to uncultured samples from the same environment or to each other.
How cosmopolitan are bacterial lineages?
Although many studies have suggested that bacteria are mostly cosmopolitan (11, 13, 32, 45), others have suggested that for certain habitats, such as the Arctic and Antarctic, geographical separation plays a major role in structuring communities because of difficulties in dispersal (in this case, of transferring psychrophilic bacteria across the warm equatorial region) (2, 42). We addressed this controversy by comparing marine ice and sediment from the Arctic and Antarctic.
Are marine ice, sediment, and seawater three distinct, homogeneous habitats?
Marine ice, sediment, and water are generally treated in the literature as distinct habitat types with distinct challenges. We compared 16S rRNA libraries from geographically diverse marine water, sediment, and ice samples to test whether these habitat types harbor consistent bacterial communities that differ from one another.
UniFrac metric.
The unique fraction metric, or UniFrac, measures the phylogenetic distance between sets of taxa in a phylogenetic tree as the fraction of the branch length of the tree that leads to descendants from either one environment or the other, but not both (Fig. (Fig.1).1). This measure thus captures the total amount of evolution that is unique to each state, presumably reflecting adaptation to one environment that would be deleterious in the other. rRNA is used purely as a phylogenetic marker, indicating the relative amount of sequence evolution that has occurred in each environment. Intuitively, if two environments are similar, few adaptations would be needed to transfer from one community to the other. Consequently, most nodes in a phylogenetic tree would have descendants from both communities, and much of the branch length in the tree would be shared (Fig. (Fig.1A).1A). In contrast, if two communities are so distinct that an organism adapted to one could not survive in the other, then the lineages in each community would be distinct, and most of the branch length in the tree would lead to descendants from only one of the two communities (Fig. (Fig.1B1B).
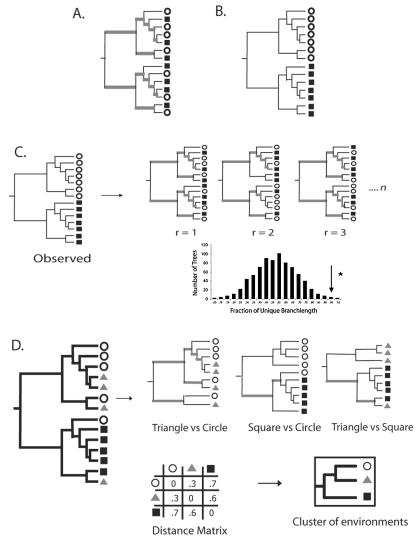
Calculation of the UniFrac distance metric. Squares, triangles, and circles denote sequences derived from different communities. Branches attached to nodes are colored black if they are unique to a particular environment and gray if they are shared. (A) Tree representing phylogenetically similar communities, where a significant fraction of the branch length in the tree is shared (gray). (B) Tree representing two communities that are maximally different so that 100% of the branch length is unique to either the circle or square environment. (C) Using the UniFrac metric to determine if the circle and square communities are significantly different. For n replicates (r), the environment assignments of the sequences were randomized, and the fraction of unique (black) branch lengths was calculated. The reported P value is the fraction of random trees that have at least as much unique branch length as the true tree (arrow). If this P value is below a defined threshold, the samples are considered to be significantly different. (D) The UniFrac metric can be calculated for all pairwise combinations of environments in a tree to make a distance matrix. This matrix can be used with standard multivariate statistical techniques such as UPGMA and principal coordinate analysis to compare the biotas in the environments.
Like the P test and the FST test, UniFrac can be used to determine whether two communities differ significantly by using Monte Carlo simulations. Two communities are considered different if the fraction of the tree unique to one environment is greater than would be expected by chance. We performed randomizations by keeping the tree constant and randomizing the environment that was assigned to each sequence in the tree (Fig. (Fig.1C1C).
UniFrac can also be used to produce a distance matrix describing the pairwise phylogenetic distances between the sets of sequences collected from many different microbial communities (Fig. (Fig.1D).1D). We compared two samples by removing from the tree all sequences that were not in either sample and computing the UniFrac for each reduced tree. Standard multivariate statistics, such as UPGMA clustering (9) and principal coordinate analysis (23), can then be applied to the distance matrix to allow comparisons between the biotas in different environments (Fig. (Fig.1D1D).
MATERIALS AND METHODS
Environmental samples.
We analyzed 20 small-subunit-rRNA sequence libraries generated in 12 different studies of marine environments (Table (Table1).1). For studies reporting both cultured and uncultured sequences or sampling from multiple environment types, we used sequence annotations to distinguish the different sampling methods and to assign sequences to specific environmental samples.
TABLE 1.
Gene library information
Samplea | Reference | No. of sequences | Water column depth (m) | Sediment depth (cm) | Latitude, longitude | Temp (°C) |
---|---|---|---|---|---|---|
SRU1 | 38 | 79 | 155 | 0-1.1 | 76°58′N, 15°34′E | 2.6 |
STU2 | 25 | 33 | 6,400 | 40°06′N, 144°11′E | ||
SNU3 | 4 | 36 | 709-940 | 1-2 | 66°S, 143°E | |
SNC4 | 4 | 31 | 709-940 | 66°S, 143°E | ||
SNU5 | 5 | 101 | 761 | 0-0.4 | 66°32′S 143°38′E | |
SNU6 | 5 | 146 | 761 | 1.5-2.5 | 66°32′S 143°38′E | |
SNU7 | 5 | 231 | 761 | 20-21 | 66°32′S 143°38′E | |
WRU8 | 2 | 87 | 55, 131 | 72-88°N, 51-356°E | ||
WTC9 | 8 | 36 | 1 | 54°09′N, 7°52′E | ||
WTU10 | 22 | 75 | 1-2 | 40°N, 73°E | 23.8-29.2 | |
WTC11 | 22 | 21 | 1-2 | 40°N, 72°E | 23.8-29.2 | |
WTU12 | 1 | 544 | 42°N, 71°E | 16 | ||
WPU13 | 11 | 17 | 10 | 32°37′N 64°57′W | ||
WPU14 | 11 | 40 | 100, 500 | 31°49′N 64°57′W | ||
INC15 | 3 | 58 | 68°S, 78°E | |||
IRU16 | 6 | 62 | 80°N, 0°E | |||
IRC17 | 6 | 109 | 80°N, 0°E | |||
INU18 | 6 | 20 | 70°S, 15°E | |||
INC19 | 6 | 87 | 70°S, 15°E | |||
INU20 | 7 | 75 | 62-77°S, 74-165°E |
Three of the studies evaluated bacterial communities in Arctic and/or Antarctic sea ice based on cultured isolates (3), environmental clones (7), or both (6). Five of the studies derived sequences from the water columns of marine environments, including pelagic bacteria from the North Sea (8), bacterioplankton assemblages from the Arctic Ocean (2), subsurface subtropical waters of the Atlantic and Pacific oceans (11), and temperate coastal water in the Great South Bay in Long Island (22) and from the marine end of the Plum Island Sound estuary in northeastern Massachusetts (1). The remaining four studies examined marine sediment, including sediments from off the coast of Spitzbergen in the Arctic Ocean (38), associated with Calyptogena communities in the deepest cold-seep area in the Japan Trench (25), and from the Antarctic continental shelf (4, 5). While three of the sediment papers reported sequences from multiple sediment cores in the same region (4, 25, 38), one reported sequences from three different depths within a single sediment core (5).
Sequences from the 12 studies were initially assigned to 23 samples. After the removal of sequences with many sequencing errors and nonbacterial sequences, the samples contained between 9 and 544 sequences. Small samples could produce misleading results because of stochastic variation in the subset of the lineages sampled. To avoid these effects, we excluded from the analysis three samples represented by 12 or fewer sequences. These included samples containing 9 sequences from uncultured clones in the North Sea (8), 10 sequences from Arctic sea ice (7), and 12 sequences from cultured isolates in Arctic seawater underlying sea ice (3). After the removal of these sequences, each sample was represented by at least 17 sequences (Table (Table11).
Data analysis.
We implemented UniFrac and associated analyses in Python 2.3.4 and ran all calculations on a Macintosh G4 computer running OSX 10.3.8. All code is available at http://bayes.colorado.edu/unifrac.zip. We implemented UPGMA clustering (9) and principal coordinate analysis (23) as described previously.
We downloaded small-subunit-rRNA sequences generated in the 12 different studies of marine environments (Table (Table1)1) from GenBank, imported them into the Arb package (26), and aligned them using a combination of the Arb auto-aligner and manual curation. Because several studies used bacterium-specific primers, we excluded all nonbacterial sequences from the analysis. We added the aligned sequences to a tree representing a range of phylogenetic groups from the Ribosomal Database Project II (29) by Phil Hugenholtz (15). This sequence addition used the parsimony insertion tool and a lane mask (lanemaskPH) supplied in the same database so that only phylogenetically conserved regions were considered. We exported the tree from Arb and annotated each sequence with 1 of 20 sample designations (Table (Table1).1). We then performed significance tests, UPGMA clustering, and principal coordinate analysis using UniFrac.
Jackknifing.
We used jackknifing to determine how the number and evenness of sequences in the different environments affected the UPGMA clustering results. Specifically, we repeated the UniFrac analysis with trees that contained only a subset of the sequences and measured the number of times we recovered each node that occurred in the UPGMA tree from the full data set. In each simulation, we evaluated 100 reduced trees in which all of the environments were represented by the same specified number of sequences, using sample sizes of 17, 20, 31, 36, 40, and 58 sequences. These thresholds reflect the sample sizes from different environments in our original data set. If an environment had more than the specified number of sequences, we removed sequences at random; environments with fewer sequences were removed from the tree entirely.
RESULTS
We used UniFrac to determine which of the microbial communities represented by the 20 different samples were significantly different (Table (Table2)2) and as the basis for a distance matrix to cluster the samples using UPGMA (Fig. (Fig.2)2) and to perform principal coordinate analysis (Fig. (Fig.3).3). We used jackknifing to assess confidence in the nodes of the UPGMA tree (Table (Table3).3). The results show biologically meaningful patterns that unite many individual observations in the literature and reveal several striking features of microbial communities in marine environments.
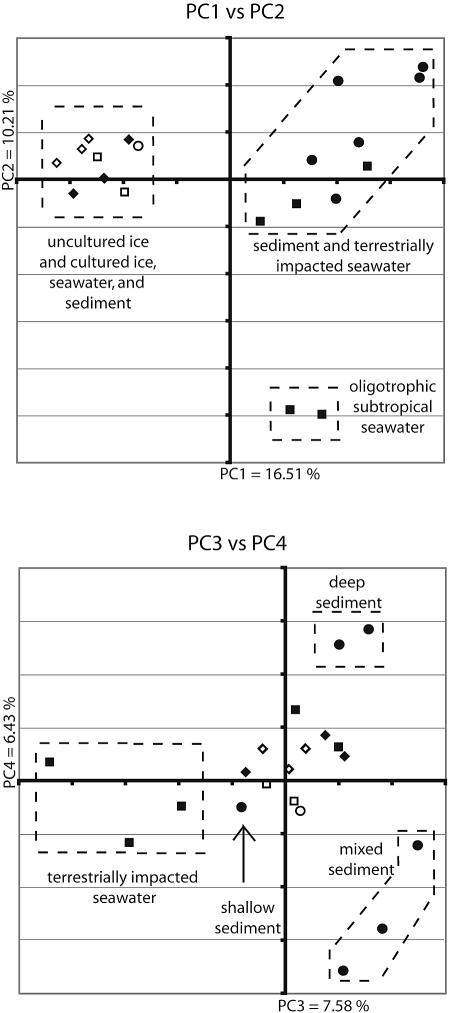
First four principal coordinates from a principal coordinate analysis of marine samples. Samples from marine ice are represented by diamonds, sediment samples are represented by circles, and water samples are represented by squares. Shapes representing samples derived from cultured isolates are open, and those representing samples from environmental clones are filled. The percentages in the axis labels represent the percentages of variation explained by the principal coordinates.
TABLE 2.
UniFrac P valuesa
Sample | Compared sample(s) (P value) |
---|---|
SRU1 | SNU3 (0.118), STU2 (0.111) |
STU2 | SNU3 (0.201), SRU1 (0.111), SNU5 (0.066), SNU6 (0.107) |
SNU3 | SNU6 (0.802), SNU7 (0.070), SRU1 (0.118), STU2 (0.201) |
SNC4 | WTC11 (0.105), SNU5 (0.053) |
SNU5 | SNC4 (0.053), STU2 (0.066) |
SNU6 | SNU7 (0.394), SNU3 (0.802), STU2 (0.107) |
SNU7 | SNU6 (0.394), SNU3 (0.070) |
WRU8 | |
WTC9 | WTC11 (0.639), INU20 (0.076), INC19 (0.155), INU18 (0.097) |
WTU10 | |
WTC11 | SNC4 (0.105), WTC9 (0.639), INC19 (0.055) |
WTU12 | |
WPU13 | WPU14 (0.238) |
WPU14 | WPU13 (0.238) |
INC15 | |
IRU16 | INU18 (0.257) |
IRC17 | |
INU18 | WTC9 (0.097), INU20 (0.055), INC19 (0.233), IRC17 (0.257) |
INC19 | WTC11 (0.055), WTC9 (0.155), INU18 (0.233) |
INU20 | WTC9 (0.076), INU18 (0.055) |
TABLE 3.
UPGMA jackknifing results
Node | % of trials with nodea
| |||||
---|---|---|---|---|---|---|
17 | 20 | 31 | 36 | 40 | 58 | |
N1 | 3 | 14 | 31 | 27 | 12 | NA |
N2 | 8 | 1 | 29 | 33 | 48 | 63 |
N3 | 1 | 8 | 7 | 11 | NA | NA |
N4 | 14 | 16 | 11 | NA | NA | NA |
N5 | 1 | 0 | 0 | 1 | 27 | 37 |
N6 | 27 | 36 | 57 | 67 | 53 | 63 |
N7 | 23 | 23 | 36 | 44 | 52 | 66 |
N8 | 22 | 17 | 17 | 39 | 31 | 37 |
N9 | 52 | 58 | 64 | NA | NA | NA |
N10 | 8 | 16 | 79 | 96 | 94 | 100 |
N11 | 6 | 12 | 40 | 46 | NA | NA |
N12 | 13 | 31 | NA | NA | NA | NA |
N13 | 16 | 38 | 41 | 38 | 64 | 79 |
N14 | 34 | 50 | 29 | 23 | 12 | 6 |
N15 | 69 | 77 | NA | NA | NA | NA |
N16 | 18 | 40 | 27 | 28 | 28 | 21 |
N17 | 24 | 35 | 43 | 46 | 37 | 50 |
N18 | 97 | NA | NA | NA | NA | NA |
Samples from cultured isolates resemble each other rather than uncultured samples from the same environment.
Although most bacteria in seawater and sediment cannot be cultivated with standard techniques (8, 10, 18, 38), most bacteria in sea ice are thought to be culturable, as sea ice samples have a high viable/total count ratio (6, 14, 19) and considerable overlap in phylotypes between cultured and uncultured samples (6, 7). To test this hypothesis, we examined the relationship between cultured isolates and environmental clone sequences derived from the same locations for sediment (SNC4 and SNU3), seawater (WTC11 and WTU10), and ice from both the Arctic (IRC17 and IRU16) and Antarctic (INC19 and INU18) (see Table Table11 for an explanation of sample abbreviations). We also included additional cultured samples from seawater (WTC9) and cultured and uncultured samples from ice (INC15 and INU20).
Cultured and uncultured sea ice bacteria cluster with each other and with the other cultured isolates (Fig. (Fig.2).2). This association is well supported by jackknife values (Table (Table3).3). The node that groups the cultured and uncultured ice samples together (Fig. (Fig.2,2, N10) is recovered 100% of the time, with 58 sequences per sample (note that at this point only five of the six ice samples are still in the tree because one sample has only 20 sequences). Pairwise significance tests for differences between environments further support this observation (Table (Table2).2). The cultured component of the Antarctic ice sample (INC19) does not differ significantly from environmental clones from the same sample (INU18).
In contrast, bacteria cultured from sediment (SNC4) and seawater (WTC11 and WTC9) cluster with other cultured samples rather than with environmental clones from the same studies (SNU3 and WTU10) in the UPGMA tree. This observation is again supported by jackknife values (Table (Table3).3). With 31 sequences, SNC4 clusters with the other cultured sequences 64% of the time (Table (Table3,3, N9) but never clusters with SNU3 or exclusively with the sediment samples (data not shown). Likewise, with 36 sequences per sample, WTC9 clusters with other cultured sequences 96% of the time (Table (Table3,3, N10). In addition, pairwise significance tests (Table (Table2)2) show that the culturable components of a seawater sample (WTC11) and a sediment sample (SNC4) differ significantly from the environmental clone sequences from the same environment (WTU10 and SNU3, respectively) but not from cultured samples from different environments.
The sequences of the culturable components of the seawater and sediment samples most resemble the environmental clone sequences from sea ice. This observation is best illustrated by principal coordinate analysis (Fig. (Fig.3).3). In principal coordinate analysis, a distance matrix is used to plot n samples in n-dimensional space. The vector through the space that describes as much variation as possible is principal coordinate 1. Orthogonal axes are subsequently assigned to explain as much of the variation not yet explained by previously assigned axes as possible. When few independent factors cause most of the variation, the first two or three principal coordinates often explain most of the variation in the data. In this case, the first four principal coordinates describe 41% of the variation, suggesting that many independent factors cause variation between samples (as might be expected for such diverse environments). Strikingly, plots of the principal components produce biologically meaningful clusters of samples, even though the individual components account for little of the variation (Fig. (Fig.33).
The first principal coordinate, which explains 17% of the variation in the data, clearly separates all samples of cultured isolates and uncultured ice from all samples of uncultured sediment and seawater (Fig. (Fig.3).3). This result suggests that bacteria capable of growing either in sea ice or in pure culture share some property, such as the ability to grow rapidly in the absence of symbionts, that is the largest factor contributing to the variation between these samples.
Geography plays a minor role in structuring communities compared to the environment type.
Our analyses support the hypothesis that geography plays a minimal role in structuring bacterial communities and that bacterial types are dispersed widely in similar habitat types across the globe (11, 13, 32). Sea ice samples from the Arctic (IRU16 and IRC17) did not separate from those from the Antarctic (INC15, INU18, INC19, and INU20) in either the UPGMA or principal coordinate clusters (Fig. (Fig.22 and and3).3). Similarly, bacterial community samples from Antarctic sediment (SNU3 and -5 to -7) cluster with those from sediments from the Arctic (SRU1) and Japan (STU2) (Fig. (Fig.2).2). This result shows that, as expected, the environment type (ice, seawater, or sediment) dominates the differences between communities. Within an environment type, we found no support for the hypothesis that samples from each pole would form a discrete cluster. This result may indicate that other differences between the samples had a greater impact on bacterial composition than being located on opposite sides of the earth and contradicts the prediction that the communities in the Arctic and Antarctic would differ because of difficulties in dispersing psychrophilic bacteria across the warm equatorial region (42). However, the poor jackknife values for resolving these nodes (Table (Table2,2, nodes N3, N14, and N16) may indicate that more sequences and more samples are needed to resolve this issue definitively.
Uncultured bacterial communities in sediment and ice form distinct clusters, but communities in seawater samples do not.
Finally, our analyses support the hypothesis that each marine sediment and ice community analyzed here forms a distinct group. Environmental clone libraries from each of these types of environment cluster together by UPGMA (Fig. (Fig.2),2), even though they were retrieved from very different locations (Table (Table1).1). In addition, uncultured sediment samples always differ significantly from seawater and ice samples but differ little from each other (Table (Table2).2). For instance, bacteria in sediment cores from the Antarctic continental shelf (SNU3), the Arctic coastal region (SRU1), and the deepest cold-seep area of the Japan Trench (STU2) did not significantly differ, despite large differences in depth, proximity to land, and geographical location. With 58 sequences, the four remaining sediment samples grouped together 63% of the time (Table (Table3,3, N2).
In contrast, seawater samples do not all cluster together but are grouped in biologically meaningful ways. For instance, an Arctic seawater sample (WRU8) clusters with an Arctic sea ice sample in the UPGMA cluster (Fig. (Fig.2).2). Nutrient-rich coastal seawater communities (WTU10 and WTU12) cluster together, as do oligotrophic open ocean communities (WPU13 and WPU14) (Fig. (Fig.22 and and3).3). These associations are often supported by jackknife values. For instance, with only 17 sequences, WPU12 and WPU14 group together 97% of the time (Table (Table2,2, N18), and with 58 sequences, WTU12 groups with WTU10 50% of the time (Table (Table2,2, N17) and with WRU8 49% of the time (data not shown). In contrast, nodes that group all of the seawater samples together are only rarely observed (5% of the time for groups with 17 sequences and 4% of the time for groups with 40 sequences). Thus, there are major differences in bacterial communities between different types of seawater, suggesting that, unlike marine sediment and ice, seawater should not be considered a distinct, homogeneous environment.
The bacterial community in the Arctic seawater sample (WRU8) appears to be more similar to those in coastal water (WTU10 and WTU12) than to those in open ocean seawater (WPU13 and WPU14). This community clusters closer to the coastal communities in both UPGMA and principal coordinate analyses (Fig. (Fig.22 and and3).3). One possible explanation is that like the coastal communities, the Arctic Ocean has high inputs of terrigenous matter: it is estimated that 25% of the dissolved organic carbon in the Arctic Ocean is derived from river runoff (44). The terrestrially impacted seawater communities (WTU10, WTU12, and WRU8) also resemble the communities in sediment samples. This is most clearly shown in principal coordinate analyses, where they cluster near each other in PC1 and PC2 but clearly separate along PC3 (Fig. (Fig.33).
DISCUSSION
The detection of biologically meaningful patterns of variation between marine samples illustrates the utility of UniFrac for explaining the distribution of bacterial lineages in the environment. The ability of UniFrac to integrate sequence data from many diverse studies makes it suitable for large-scale comparisons between environments. The ability of UniFrac to integrate sequence data from many diverse studies makes it suitable for large-scale comparisons, between environments despite variability in data collection techniques. For instance, different studies used different sequencing primers, and thus little of the 16S rRNA molecule was present in all sequences in the alignment. This made it impossible to use algorithms that require the same sequence region to create the phylogenetic tree for analysis. Potential imperfections in the Arb parsimony insertion tool for creating the phylogeny, however, were not great enough to confound the detection of biologically meaningful patterns of variation.
Those who performed the previous studies also chose clones for sequencing using different methods: some screened clones with restriction enzyme-based techniques (6-8, 22, 25, 38) or denaturing gradient gel electrophoresis (2, 4) prior to sequencing, while some sequenced samples directly (1, 5, 11). Since UniFrac does not count the number of times each sequence is observed, these data can still be compared, although the “evenness” component that is a standard measure of diversity (27) is not currently represented in UniFrac. Since we compared environments on a large scale, the ability of particular lineages of organisms to survive in each environment is more likely to represent the relevant aspects of similarity between environments than the relative abundance of each surviving lineage. However, although the practice of predicting abundance from environmental clone data is sometimes questioned because of PCR bias and differences in genomic DNA extraction methods and rRNA copy numbers (21, 43), such data can be useful, especially on smaller spatial and temporal scales (20, 24, 31, 41). We have thus also developed a variant of the algorithm that weights the phylogenetic differences according to the abundance of each lineage, which will allow questions about evenness to be addressed.
Jackknifing the UPGMA tree revealed that surprisingly small sample sizes can be sufficient to detect associations between groups of samples. For example, the oligotrophic seawater samples WPU13 and WPU14 cluster together stably with only 17 sequences. However, samples that are more diverse, such as those from sediments, or less distinct, such as the Antarctic and Arctic ice samples, require more sequences for robust conclusions to be drawn. We recently demonstrated that UniFrac is robust even for very similar samples when the sample size is large. We were able to detect an association between kinship and gut microbial community structure in related mice, using sequence sets of 200 to 500 per mouse (24). We thus expect that the utility of UniFrac will increase as larger environmental samples become available.
Our analysis provides a unified framework for explaining previous observations in the literature, such as the observation that culturing affects the observed diversity in seawater and sediment but not that in ice. It also allows broader conclusions, such as the observation that terrestrially impacted seawater samples from polar and temperate climates resemble each other and sediment samples but differ greatly from tropical oligotrophic seawater samples. Terrestrially impacted seawater probably resembles sediment more than oligotrophic seawater for reasons other than relative nutrient availability, since one sediment sample (STU2) was obtained from 6,400 m below sea level and received low inputs of organic carbon (25). The resemblance may instead arise because terrestrially impacted seawater has a higher concentration of particles, and particle-associated and freely suspended marine bacteria are known to differ (12).
The large differences between different seawater communities are surprising, since the ubiquity of certain bacterial lineages in pelagic systems, such as SAR11 and SAR86, has been taken as evidence that much of the ocean harbors similar bacteria (11, 22). In contrast, the different sediment samples are remarkably similar. This supports the hypothesis that large portions of the sea floor have similar biotas because of similar environmental conditions such as nutrient availability and temperature (e.g., 90% of the sea floor has temperatures below 4°C) and similar processes such as sulfate reduction (7, 38).
Conclusion.
The utility of UniFrac for making broad comparisons between the biotas of different environments based on 16S rRNA sequences has enormous potential to shed light on biological factors that structure microbial communities. The vast wealth of 16S rRNA sequences in GenBank and of environmental information about these sequences in the literature, combined with powerful phylogenetic tools, will greatly enhance our understanding of how microbial communities adapt to unique environmental challenges.
Acknowledgments
Catherine Lozupone was supported in part by NIH predoctoral training grant T32 GM08759.
We thank Mike Yarus, Norm Pace, Scott Kelley, Ruth Ley, Shelley Copley, and Corrella Detweiler for their comments on the manuscript.
REFERENCES
Articles from Applied and Environmental Microbiology are provided here courtesy of American Society for Microbiology (ASM)
Full text links
Read article at publisher's site: https://doi.org/10.1128/aem.71.12.8228-8235.2005
Read article for free, from open access legal sources, via Unpaywall:
https://europepmc.org/articles/pmc1317376?pdf=render
Citations & impact
Impact metrics
Article citations
Changes in social environment impact primate gut microbiota composition.
Anim Microbiome, 6(1):66, 13 Nov 2024
Cited by: 0 articles | PMID: 39538341 | PMCID: PMC11562706
Unravelling spatial scale effects on elevational diversity gradients: insights from montane small mammals in Kenya.
BMC Ecol Evol, 24(1):139, 08 Nov 2024
Cited by: 0 articles | PMID: 39516748 | PMCID: PMC11545329
Multi-omics evaluation of clinical-grade human umbilical cord-derived mesenchymal stem cells in synergistic improvement of aging related disorders in a senescence-accelerated mouse model.
Stem Cell Res Ther, 15(1):383, 29 Oct 2024
Cited by: 0 articles | PMID: 39468666 | PMCID: PMC11520580
Temporal Changes in Jejunal and Ileal Microbiota of Broiler Chickens with Clinical Coccidiosis (<i>Eimeria maxima</i>).
Animals (Basel), 14(20):2976, 15 Oct 2024
Cited by: 0 articles | PMID: 39457906 | PMCID: PMC11503835
Gut-microbiome profiles among Soil-transmitted helminths (STHs) infected Ethiopian children enrolled in the school-based mass deworming program.
PLoS Negl Trop Dis, 18(10):e0012485, 15 Oct 2024
Cited by: 0 articles | PMID: 39405336 | PMCID: PMC11478818
Go to all (4,260) article citations
Similar Articles
To arrive at the top five similar articles we use a word-weighted algorithm to compare words from the Title and Abstract of each citation.
UniFrac--an online tool for comparing microbial community diversity in a phylogenetic context.
BMC Bioinformatics, 7:371, 07 Aug 2006
Cited by: 860 articles | PMID: 16893466 | PMCID: PMC1564154
Review Free full text in Europe PMC
Diversity and structure of bacterial communities in Arctic versus Antarctic pack ice.
Appl Environ Microbiol, 69(11):6610-6619, 01 Nov 2003
Cited by: 181 articles | PMID: 14602620 | PMCID: PMC262250
[Phylogenetic diversity and cold-adaptive hydrolytic enzymes of culturable psychrophilic bacteria associated with sea ice from high latitude ocean, Artic].
Wei Sheng Wu Xue Bao, 46(2):184-190, 01 Apr 2006
Cited by: 2 articles | PMID: 16736573
Quantitative and qualitative beta diversity measures lead to different insights into factors that structure microbial communities.
Appl Environ Microbiol, 73(5):1576-1585, 12 Jan 2007
Cited by: 1289 articles | PMID: 17220268 | PMCID: PMC1828774
Funding
Funders who supported this work.
NIGMS NIH HHS (3)
Grant ID: T32 GM08759
Grant ID: T32 GM008759
Grant ID: T32 GM142607