Abstract
Free full text

fMRI study of effort and information processing in a working memory task
Abstract
It is unclear how effort translates into brain function. In this study we endeavored to identify the activity in a working memory task that is related to the allocation of mental resources. Such activity, if present, would be a likely candidate to explain how effort works in terms of brain function. Eleven healthy participants performed a Sternberg task with a memory‐set of one, three, or five consonants in an fMRI study. Probe stimuli were either one consonant or one digit. We expected digits to be processed automatically and consonants to require working memory. Because the probe type was unpredictable and subjects had to respond as fast as possible, we expected subjects to allocate mental resources on the basis of the memory‐set size, not the probe type. Accordingly, we anticipated that activity in regions involved in effort would be a function of the size of the memory‐set, but independent of the type of probe. We found that the reaction‐time for digits increased in line with our expectation of automatic processing and the reaction time for letters increased in line with our expectation of controlled processing. fMRI revealed that activity in the right ventral‐prefrontal cortex changed as a function of effort. The ventral anterior cingulate cortex and hypothalamus showed reduced activity as a function of effort. Activity in regions regarded as pivotal for working memory (among others, the left dorsolateral prefrontal cortex, anterior cingulate cortex) appeared to be predominantly related to information processing and not involved in effort. Hum Brain Mapp, 2007. © 2006 Wiley‐Liss, Inc.
INTRODUCTION
How well we perform a task is not only related to the difficulty of the task, but also to how much effort we put into performing it. Yet despite the fact that the concept of effort is commonly used in relation to performance, it is not very well understood how this concept translates into brain function. This may be in part because the concept of effort is not well defined. Previous articles that examined the effects of effort typically related it to task difficulty, but did not provide a definition of effort in terms of brain function [Barch et al., 1997; Grady et al., 1997; Petersen et al., 1998]. In this article we propose a definition of effort in terms of brain function and present the results of a study that we designed to identify activity related to effort based on that definition.
It can be expected that several brain functions are competing for mental resources at any given time, given the limited nature of its capacity. We postulate that if a specific task has to be executed, the brain needs to allocate mental resources so that they can be applied to the task without the interference of other competing processes. If it is possible to detect activation related to this allocation of mental resources, then we believe this is a likely candidate for defining effort in terms of brain function. In accordance with this working definition, for the remainder of this article we will refer to the allocation of resources as “effort” and to the use of mental resources as “information processing.”
In our experiment we examined if the execution of a working memory task evokes activity that is specifically related to effort, or by our definition, the allocation of mental resources. Our experiment was based on the rationale that if the task‐difficulty varies within a task in an unpredictable manner, then under certain circumstances it can be expected that the level of effort will be sustained at a consistently high level adequate to perform the entire task. For instance, in a task that requires a subject to react as fast as possible, any adjustment of effort after the fact would lead to a reaction time that is not optimal. In contrast, the level of information processing required to perform that task may still vary within the task.
In our experiment we used a task based on a Sternberg [1966] paradigm to detect activity related to effort. The difficulty of a Sternberg task, measured by reaction time and the accuracy of response, can be varied by manipulating the memory‐set size or by manipulating the probe type. Typically, the difficulty of processing a probe, as indicated by the reaction time, increases with the size of the memory‐set. But if a probe stimulus can be processed automatically, the difficulty is independent of the memory‐set size [Schneider and Shiffrin, 1977]. If the probe type is varied randomly within a task and subjects are required to react as fast as possible, the level of effort cannot be adjusted on the basis of the probe type. In that case, the level of effort will be a function of the size of the memory‐set: It will be constant over a run, adequate to process the most difficult probe (and consequently sometimes too high for probes that can be processed automatically). However, the level of information processing can still vary within a run, being low for stimuli that can be processed automatically, and high for stimuli that cannot be processed automatically.
We used fMRI in a combined block‐ and event‐related paradigm to measure brain activity related to effort and information processing. On the basis of previous studies of automatic and controlled processing in a Sternberg task, we expected performance to be a function of the memory‐set size for letters, but either not at all or significantly less for digits [Schneider and Shiffrin, 1977]. With regard to brain activity, we hypothesized that: 1. regions involved in effort to show a change in activity—either positive or negative—for digits as a function of the memory‐set size. 2. regions involved in information processing were expected not to show an increase in activity for digits, but only for letters.
SUBJECTS AND METHODS
Participants
Eleven right‐handed subjects (M/F 6/5, mean age 33.0 ± 2.9) participated in the study. Before the scanning session participants gave written informed consent to participate in the study, which was approved by the Intramural Review Board (IRB) of the National Institute of Neurological Disorders and Stroke at the National Institutes of Health under protocol #00‐N‐0082. Participants were provided with earplugs to protect their hearing from the acoustic noise generated by the MRI gradient system.
Tasks
We based the task used in our study on a Sternberg task‐paradigm (see Fig. Fig.1).1). This task has been used extensively in functional MRI (fMRI) studies. It has been shown to reliably activate regions associated with working memory [Jansma et al., 2001; Manoach et al., 1997; Rypma and d'Esposito, 1999]. It also allows a trial‐by‐trial measurement of the level of performance (reaction time and accuracy) to verify that subjects are executing the task as required.

Schematic presentation of the task. Subjects were shown a memory set of one, three, or five consonants for 5400 ms. After this, 18 probe stimuli were displayed for 1000 ms at a rate of one per 1800 ms, comprising one block. Then a new memory‐set was presented for 7200 ms for a second block. Each subject completed a total of eight blocks of each task level (memory‐set size of one, three or five letters). The order of the blocks in each of the four runs is shown at the bottom of the figure (M = task, numbers represent memory load).
In our experiments, participants first had to memorize a specific set of letters referred to as a “memory‐set.” To increase the verbal and spatial similarity of the stimuli, all of the letters used in the task were consonants. After memorizing the memory‐set, the participants then saw a series of “probe stimuli”—both letters and digits—that could either be part of the memory‐set (i.e., a “target”) or not (i.e., a “nontarget”). Participants had to decide as fast as possible whether each probe stimulus was a target or nontarget.
Nontargets could be either letters or digits. Previous research has shown that performance and brain activity will increase as a function of the memory‐set size [Manoach et al., 1997; Rypma and d'Esposito, 1999] when probe stimuli are in the same category as the items of the memory‐set (e.g., when both are letters). In addition, it has been shown that probe stimuli that are from a different category, such as digits, can be processed automatically. Theoretically, this means that the reaction time for digits should be independent of the memory‐set size. In practice, it has turned out that even if categories are long overlearned and full automatic processing can be expected, there typically still is a small increase in the reaction time of about 10 ms per item with each additional item in the memory‐set [Schneider and Shiffrin, 1977].
We varied the size of the memory‐set between one, three, and five consonants. This manipulation was expected to increase the reaction time and information‐processing load for probe letters, but not for digits. In addition, it was expected to increase the effort, or allocation of mental resources, over a whole run, independently of the type of probe. This expectation was based on the fact that the subject was not able to predict the type of probe. Because the subject had to react as fast as possible, it was expected that he or she could not adjust effort after the probe was presented, but maintained it at a level adequate for the most difficult probe over the whole run.
A video projector presented the probe stimuli visually on a small screen attached to the headcoil in the scanner. Participants could see the screen via a mirror also attached to the headcoil. Subjects were instructed to respond to each probe stimulus as quickly as possible by pushing a button with a finger of the right hand if the probe was a target or with the middle finger of the right hand if the probe was a nontarget.
Tasks were presented in blocks of one memory‐set followed by 18 probe stimuli. Memory sets were computer‐generated and randomly chosen for each block. The memory‐set was presented for 5400 ms, followed by a focus cross for 1800 ms. Each probe stimulus was displayed for 1000 ms, followed by a focus cross for 800 ms (comprising one trial) (see Fig. Fig.1).1). The three categories of probe stimuli—target consonant, nontarget consonant, and digit—were presented the same number of times within each block, and each letter and digit was presented the same number of times over the course of the experiment. Subjects completed four runs of six blocks, so that all subjects performed eight blocks with a memory‐set size of one letter (M1), eight blocks with a memory‐set size of three letters (M3), and eight blocks with a memory‐set size of five letters (M5) (see Fig. Fig.1,1, bottom). Within each run we added three rest periods (of similar duration as one block) to measure signal levels during the resting state.
Because digits were by definition always nontargets, we only compared them with nontarget letters to exclude a possible confounding effect of the differences between targets and nontargets. For convenience, when we use the term “letters” in the remainder of the article we refer to nontarget letters.
Data Analysis
Behavioral measurements
We measured reaction times as well as response accuracy. Reaction times were computed over correct responses. Performance was averaged over all runs within subjects for each category of stimulus in each task. To test the performance hypotheses, we used paired sample t‐tests (analysis performed in SPSS 11.0, Chicago, IL). A P‐value of 0.05 was considered significant, while a P‐value of 0.1 is reported as a trend.
Image acquisition and processing
We performed functional MRI using a 1.5 T Siemens (Erlangen, Germany) scanner equipped with an 8‐element receiver coil (Nova‐coil) [de Zwart et al., 2002a] connected to an 8‐channel receiver chain (scan parameters: 64 × 48 × 28 matrix, field of view (FOV): 256 × 256 mm2, voxel size: 4.0 mm2, slice thickness: 4.0 mm (0.4 mm gap), TE/TR 40/1800 ms). We executed scan reconstruction according to a protocol described in detail elsewhere [de Zwart et al., 2002b]. After reconstruction, we registered all scans to the last scan of the last run to correct for subject movement during the experiment [Thevenaz et al., 1998]. Using a Gaussian filter with a full‐width at half‐maximum (FWHM) of 8 mm in each direction, we spatially smoothed individual scans.
Statistical analysis
We used two separate analyses to generate activation maps. A block design analysis enabled us to maximize the power to detect those regions involved in executing the task. This design used a multiple‐regression analysis with separate regressors for each task (M1, M3, and M5), which were orthogonal. Two regressors were used for trend correction per block and three regressors to correct for the general level difference between run (see Fig. Fig.2a;2a; for details, see Waldvogel et al. [ 2000]). The significance tests to detect active voxels were based on the t‐values associated with the three regressors that modeled task‐rest differences for the three tasks M1, M3, and M5. The other regressors were not used for further analysis, but were only added to account for either trends within a scan period or baseline shifts between scan periods.
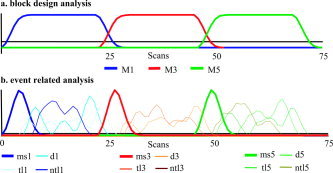
Regressors used for the blocked analysis (a) and event‐ related analysis (b). The event‐related regressors were created by convoluting the timing of each category of probe stimulus with a fixed BOLD model curve. The regressors for the presentation of the memory‐set (thick lines, below) were added to increase the fit of the model, but results were not further analyzed and included in this article (ms, memory‐set; tl, target letter; ntl, nontarget letter; d, digit; number represents memory‐set size).
To determine the level of activity for each stimulus category within each task, we used an event‐related design, with separate regressors for each stimulus in each task, which yielded in total nine task regressors (target‐letters, nontarget letters, and digits, for M1, M3, and M5). To generate these regressors we convoluted a blood oxygenation level‐dependent (BOLD) model with the presentation times of each stimulus category within each task [see Waldvogel et al., 2000].
We spatially normalized individual activation maps through a linear transformation using FSL software (FMRIB Software Library, 3.2 Beta, University of Oxford, UK; parameters: affine transformation with 12 parameters, cost function: correlation ratio, interpolation: trilinear). Transformation parameters were determined by registering one functional scan to a standard echo‐planar imaging (EPI) template. We transformed the original resolution (4.0 × 4.0 × 4.0 mm) to a standard resolution of the EPI template of 2.0 × 2.0 × 2.0 mm in the spatially normalized activation maps.
Using the normalized activation maps of the blocked‐design analysis, we created regions of interest (ROIs) for further analysis. Voxels were considered significantly different from baseline activation if the group t‐level was either higher than 3.09 or lower than − 3.09, which corresponds to a P‐level of 0.001 (uncorrected for multiple comparisons). We chose a lenient threshold for this first step to ensure that we included most regions showing signal changes related to the task; we did not use this threshold to test the experimental hypotheses. Regions smaller than eight voxels of original scan resolution (or a total volume of 512 mm3) were excluded from the analysis. We generated group‐level activation maps by applying a t‐test voxelwise over the normalized signals of all subjects. Voxels that were neighbors and that reached the significance threshold in either the M1, M3, or M5 group‐level activation map were combined to generate a group of ROIs. Further analysis was restricted to the ROIs selected with this method. The activation levels for digits and letters for the M1, M3, and M5 tasks generated by the event‐related analyses were averaged over all voxels within each ROI. We then used these values to test our hypotheses.
To be able to characterize the signal changes, we applied three tests: first, in order to test if a region showed activity conforming to our expectation of effort, we tested whether the activity level for digits was a function of the memory‐set size; second, in order to test if a region was sensitive to the level of information processing, we tested whether the activity level for letters was a function of the memory‐set size; and third, in order to test if the effect of effort and that of information processing were significantly different, we tested whether the effect of the size of the memory‐set on activity was different for letters than for digits. The first two tests were based on t‐tests (one‐sided P‐values). The third test was conducted by comparing the change between M1 and M5 for digits and letters. The activation for M3 was used to select ROIs, but was not directly included in the hypothesis testing, because in an analysis for linear change with three points, the middle point does not affect the test result. Omitting the middle point allowed for direct tests of our hypotheses. We conducted all three tests for the signal changes in each ROI separately, for the signal changes averaged over those ROIs showing a signal increase, and for those ROIs showing a signal decrease. All image analysis was performed using software developed in‐house (NINDS, LFMI, advanced MRI) and implemented in IDL™ (Research Systems, Boulder, CO). We conducted all hypothesis tests on the fMRI data in SPSS 11.0, using a significance level of P = 0.05. Results with a significance level between 0.05 and 0.1 are mentioned as a trend.
RESULTS
Performance
All subjects performed the tasks according to the instructions, indicated by the fact that each individual responded correctly on more than 90% of the trials for each stimulus category and each task. The reaction time for digits increased significantly with the size of the memory‐set (t(10) = 56.7; P < 0.001). The reaction times for letters increased significantly as the size of the memory‐set increased (t(10) = 75.2; P < 0.001). The increase in reaction time for digits was significantly smaller than the increase for letters, (t(10) = 79.2; P < 0.001, see Fig. Fig.3).3). The accuracy of task performance for letters lessened as the size of the memory‐set increased (t(10) = 6.33; P = 0.003, see Fig. Fig.3b).3b). For digits, accuracy was consistently 100%, independent of the memory‐set size.
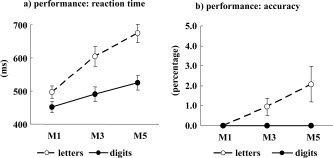
Performance results. a: Reaction times for the different stimulus categories in the four tasks. b: Standard deviation of reactions. Nontarget consonants showed a strong increase in reaction times with increasing memory‐load (M, memory‐set size, 1, 3, or 5 letters). This increase was significantly smaller for digits. The error rate was not significantly increased by the memory load.
Functional MRI
Selection of ROIs
To evaluate the fMRI data, we first analyzed the block‐design results to identify regions showing significant brain activity in any of the tasks. This preselection was used to further limit analysis to regions most likely to be involved in executing the task. This procedure resulted in nine regions that showed a signal increase and seven regions that showed a signal decrease compared with the resting‐state baseline (see Table TableI,I, Fig. Fig.55).

Brain activity levels in regions showing increased task‐related activity. All regions showed significantly higher activity for letters than for digits, except the right VPFC. Activity in the right VPFC showed a significant increase for both digits and letters with memory‐set size, indicating effort‐related activity. Activity in the left DLPFC, dACC, rINS, and lVLPFC showed an increase in activity for letters related to the memory‐set size, but not for digits, indicating that activity was predominantly related to the level of information processing (M, memory‐set size, 1, 3, or 5 letters; au, arbitrary units).
Table I
Selected regions of interest (ROIs)
Region of interest | Hemisphere | Reference (abbreviation) | Brodmann area | Size (cm3) | Talairach coordinates | ||
---|---|---|---|---|---|---|---|
x | y | Z | |||||
A. Regions showing a signal increase | |||||||
ROI 1 | Right | Occipital cortex (rOCC) | 18 | 25.1 | 24 | −92 | 14 |
ROI 2 | Left | Occipital cortex (lOCC) | 18 | 23.8 | −32 | −90 | 6 |
ROI 3 | Left | Dorsolateral prefrontal cortex (lDLPFC) | 9 | 9.5 | −52 | 6 | 30 |
ROI 4 | Left and right | Dorsal anterior cingulate cortex (dACC) | 32 | 8.7 | −8 | 4 | 56 |
ROI 5 | Right | Dorsolateral prefrontal cortex (rDLPFC) | 9 | 4.5 | 28 | −2 | 64 |
ROI 6 | Left | Superior parietal cortex (lSPC) | 7 | 2.8 | −24 | −58 | 52 |
ROI 7 | Right | Insula (rINS) | 2.8 | 30 | 18 | 4 | |
ROI 8 | Right | Ventrolateral prefrontal cortex (rVPFC) | 10 | 2.0 | 32 | 40 | 22 |
ROI 9 | Left | Ventrolateral prefrontal cortex (lVPFC) | 45 | 1.3 | −48 | 32 | 28 |
B. Regions showing a signal decrease | |||||||
ROI 1 | Left and right | Anterior cingulate cortex(vACC) | 23 | 13.47 | 2 | 28 | −10 |
ROI 2 | Left | Angular gyrus (lAG) | 39 | 8.49 | −44 | −76 | 28 |
ROI 3 | Right | Post central gyrus (rPCG) | 3 | 3.44 | 18 | −28 | 64 |
ROI 4 | Left | Insula (lIns) | 13 | 2.18 | −38 | −16 | 14 |
ROI 5 | Right | Middle frontal gyrus (rMFG) | 46 | 1.47 | 58 | 34 | 6 |
ROI 6 | Left | Posterior cingulate cortex (lPCC) | 30 | 0.81 | −10 | −48 | 4 |
ROI 7 | Left and right | Hypothalamus (HTM) | 25 | 0.73 | −4 | 6 | −10 |
Regions showing a signal increase were found in the occipital cortex in the right hemisphere (rOCC, approximately in Brodmann area 18 (BA 18)) and left hemisphere (lOCC, BA 18). Five regions were located in the frontal cortex: Two regions in the left and right frontal cortex were located in the dorsolateral prefrontal cortex (DLPFC, BA9). Two other regions were located in a more ventral part of the prefrontal cortex in the left hemisphere (lVPFC, BA 45) and right hemisphere (rVPFC, BA 10), while in the left hemisphere there was also an ROI located in the right insula (rINS). A bilateral ROI was located in the dorsal part of the anterior cingulate cortex (dACC, BA 32). In the parietal cortex, we also identified a lateralized region in the left superior parietal cortex (lSPC, BA 7).
Regions that showed task‐related signal decreases (see Table TableI,I, Fig. Fig.6)6) were found in the ventral part of the anterior cingulate cortex (vACC, BA 32), the left angular gyrus (lAG, BA 39), the postcentral gyrus (rPCG, BA 3), the left insula (lINS, BA 13), the right middle frontal gyrus (rMFG, 46), the left posterior cingulate cortex (lPCC, BA 30), and the bilateral hypothalamus (HTM, BA 25).

Brain activity levels in regions showing decreased activity. None of the regions showed a significant difference in activity between letters and digits. The decrease in activity in the vACC and HTM was correlated with the memory‐set size for both digits and letters, indicating effort‐related activity (M, memory‐set size, 1, 3, or 5 letters; au, arbitrary units).
Testing of Hypotheses in ROIs Showing Signal Increases
For each ROI we evaluated the average signal change as a function of the size of the memory‐set.
On the basis of our hypotheses, we expected brain activity to increase as a function of memory‐set size for letters and digits if a region was involved in effort. Combined over all ROIs, the increase was a function of the size of the memory‐set for letters (t(10) = 2.77; P = 0.01), but not for digits (t(10) = 0.32; P = 0.64; see Table TableII,II, part A, Fig. Fig.4a).4a). The signal increase from M1 to M5 was also greater for letters than for digits (t(10) = 2.04; P = 0.035); see Table TableII,II, part A). Of the nine ROIs the right VPFC showed a increase in the signal for digits (t(10) = 2.93; P = 0.008).

Figures showing the probe‐related activity in regions showing task‐related increases and decreases in activity. Signal increases were predominantly related to the level of information processing (a). Signal decreases were predominantly related to effort, and not to information processing (b: M, memory‐set size, 1, 3, or 5 letters; au, arbitrary units).
Table II
Significance tests per region of interest (ROI), effect memory‐set size ondigits, effect memory‐set size on letters difference in effect on digits and letters, and characterization of each region, based on the tests (x = P < 0.05; o = P < 0.10 also included)
Digits (M1 – M5) | Letters (M1 – M5) | Digits – letters (M1 – M5) | Effort | Information processing | ||||||
---|---|---|---|---|---|---|---|---|---|---|
t(10) | P | t(10) | P | t(10) | P | |||||
A. Regions showing a signal increase | ||||||||||
All ROIs | 0.48 | 0.32 | 2.77 | 0.01 | 2.04 | 0.04 | X | |||
rOCC | −0.23 | 0.41 | 1.40 | 0.09 | 1.61 | 0.07 | O | |||
lOCC | 0.31 | 0.38 | 2.39 | 0.02 | 2.22 | 0.03 | X | |||
lDLPFC | 1.14 | 0.14 | 2.88 | 0.01 | 1.03 | 0.16 | X | |||
dACC | 0.96 | 0.18 | 2.08 | 0.032 | 1.34 | 0.11 | X | |||
rDLPFC | −1.52 | 0.08 | 0.97 | 0.17 | 3.9 | 0.002 | ||||
lSPC | −1.37 | 0.10 | 1.71 | 0.059 | 0.37 | 0.72 | O | |||
rINS | 0.59 | 0.29 | 2.03 | 0.04 | 2.24 | 0.025 | X | |||
rVPFC | 2.93 | 0.008 | 2.38 | 0.02 | −1.02 | 0.17 | X | X | ||
lVPFC | 1.46 | 0.09 | 3.51 | 0.003 | 0.71 | 0.25 | O | X | ||
B. Regions showing a signal decrease | ||||||||||
All ROIs | 1.75 | 0.06 | 2.88 | 0.008 | 0.69 | 0.28 | O | |||
vACC | 2.26 | 0.02 | 2.89 | 0.008 | 0.98 | 0.17 | X | |||
lAG | 0.189 | 0.42 | 0.76 | 0.23 | 0.38 | 0.36 | ||||
rPCG | 1.45 | 0.09 | 2.77 | 0.01 | 0.23 | 0.42 | O | |||
lINS | 1.61 | 0.07 | 1.37 | 0.09 | −0.22 | 0.41 | O | |||
rMFG | 1.39 | 0.09 | 3.45 | 0.003 | 1.26 | 0.12 | O | |||
lPCC | 0.25 | 0.41 | 0.37 | 0.36 | −0.075 | 0.47 | ||||
HTM | 2.44 | 0.02 | 1.81 | 0.05 | 1.11 | 0.15 | X |
For abbreviations, see Table TableII.
Six of the nine ROIs showed a increase for letters in the signal as a function of the size of the memory‐set. These regions were located in the lOCC (t(10) = 2.39; P = 0.019), the lDLPFC (t = 2.88; P = 0.009), the dACC (t = 2.08; 0.032), the rINS (t = 2.03; 0.035), the rVPFC (t = 2.38; P = 0.019), and the lVPFC (t(10) = 3.51; P = 0.003).
The increase in activity for letters was significantly larger than for digits in the lOCC (t(10) = 2.22; P = 0.026), the rDLPFC (t(10) = 3.9; P = 0.0015), and the rINS (t = 2.24; P = 0.025) (see second column in Table TableII,II, part A).
Testing of Hypotheses in ROIs Showing Signal Decreases
We applied similar tests to the ROIs that showed a signal decrease in comparison with the resting state (see Table TableII,II, part B).
Taken over all ROIs, the signal change for digits as a function of the size of the memory‐set was in the expected direction and bordering on significance, but did not reach it (t(10) = 1.75; P = 0.06). This indicates that activity in deactivated regions shows a trend towards effort. The activity level for letters decreased as a function of the size of the memory‐set (t(10) = 2.88; P = 0.0008; see Fig. Fig.4b),4b), but there was no significant difference in the signal decrease from M1 to M5 between digits and letters (t = 0.69; P = 0.28). This indicates that brain activity in the deactivated regions did not reflect the level of information processing.
Tests per ROI showed that the signal decrease for digits in the vACC (t(10) = 2.26; P = 0.024) and the HTM (t(10) = 2.44; P = 0.017) was larger for M5 than for M1.
The signal decrease for letters in the vACC (t(10) = 2.89; P = 0.008), the right PCG (t(10) = 2.77; P = 0.01), the rMFG (t(10) = 3.45; P = 0.003), and the HTM (t(10) = 1.81; P = 0.05) increased with the memory‐set size. None of the ROIs showed a larger change in activity for letters than for digits from M1 to M5.
DISCUSSION
The goal of this study was to detect brain activity related to effort. We defined effort as the allocation of resources when performing a task and information processing as the use of resources to perform the task. We used a Sternberg task with a memory‐set of one, three, or five letters and probe stimuli that we expected subjects to process either automatically (i.e., digits) or in a controlled mode (i.e., letters). We expected regions that were involved in effort to show a change in activity as a function of the size of the memory‐set, but not as a function of the type of probe. We expected brain regions involved in information processing to change as a function of the memory‐set size if the probe was a letter, but to be independent of the memory‐set size if the probe was a digit.
The behavioral data confirmed our expectation that letters would be processed in a controlled mode, while digits would be processed automatically. There was a small increase in the reaction time for digits, conforming to what can be expected if digits are processed automatically. The increase in the reaction time for letters was significantly larger, which also conforms to what can be expected if probes are processed in a controlled mode [Schneider and Shiffrin, 1977]. The accuracy data showed a profile similar to that of the reaction time data, indicating automatic processing for digits and controlled processing for letters.
We determined a network of nine regions showing positive activity related to the task. Activity in this network appeared to change as a function of the memory‐set size for letters, but not for digits, indicating that this network predominantly reflected information processing and not effort. Results for separate regions in this network revealed that activity in the rVPFC changed expressly as a function of effort, while activity in the left VPFC showed a trend in this direction. The majority of the other regions involved in task execution showed activity related predominantly to information processing (lOCC, lDLPFC, dACC, rINS, lVPFC, and rVPFC), while there was a trend in this direction for the rOCC and the lSPC. Activity in the rDLPFC could not be characterized as related to either effort or information processing on the basis of our definitions, because it did not show an increase for letters, but a decrease for digits.
We also identified a network showing negative activity (i.e., activity below the resting state baseline) during the execution of a task. Signals in this network appeared to change as a function of the memory‐set size (significant for letters and approaching significance for digits), while the level of activity did not differ for letters vs. digits. This indicates that as a network these regions showed activity in line with our expectations for effort and not conform to our expectation of information processing. Results for separate regions in this network indicated that the vACC and the HTM were correlated specifically with the level of effort exerted, while there was a trend toward effort‐related activity in the rPCG, the lINS, and the rMFG.
The results of our study indicate that positive activity related to the execution of a working memory task is predominantly related to the demand for information processing. Regions that traditionally have been regarded as pivotal for working memory and executive functioning—the left DLPFC and the dACC [d'Esposito et al., 1995; Smith et al., 1998]—did not show activity related to effort. With regard to the left DLPFC, previous studies have shown that activity in this region does not change if the perceptual difficulty of a task is increased [Barch et al., 1997; Grady et al., 1996]. These studies provide converging evidence that the left DLPFC is not involved in effort.
In contrast to the notion that the DLPFC performs an information‐processing function, an influential theory about the dACC postulates that it serves as a “detector of conflict.” A conflict can occur when an automatic response needs to be inhibited or no automatic response is available. In either of these situations the dACC signals to other executive regions such as the DLPFC if there is a need to adapt cognitive control [Botvinick et al., 2001]. Our results can be explained within this theory, in that they showed that the dACC was more active if the need to change cognitive control during a task was larger. Furthermore, the dACC increased activity only if an automatic response was unavailable. Alternatively, it is also possible that the dACC activity reflected error detection [Coles et al., 1995]—the dACC was more active in conditions where subjects committed more errors. However, the number of errors made by the subjects was relatively small, which makes it questionable whether the number of trials with errors could generate enough statistical power to be detected in activity changes in the dACC.
We identified two regions in the VPFC that showed positive activity in line with our definition of effort. These regions may play a role in the allocation of resources needed to perform a mental task. Previous studies have shown that these regions are active during tasks that require functions such as problem solving and planning [Duncan and Owen, 2000; Owen, 1997]. Koechlin et al. [ 1999] proposed an even more precise role for them: They showed that the VPFC became active specifically if a subject had to keep a certain goal in mind while performing other tasks. These descriptions all seem to fit within our definition of effort as resource allocation. On the other hand, the study of Koechlin et al. also indicated that there was no increase in activity in the VPFC if the task was made more difficult through stimulus degradation. But our study indicated that activity in these two regions scaled with the anticipated difficulty of the task, which seems to contradict Koechlin et al.'s findings. Further studies will have to show which finding can be reproduced, or possibly that a slightly different region or different function was targeted by the current study compared with the Koechlin et al. study.
We also found a network of regions that was inhibited during task execution. The majority of the regions that we identified as showing reduced activity during the execution of a task (vACC, lAG, lINS lMFG, and lPCC) were close to regions that have been identified previously as belonging to a network of default activity [Shulman et al., 1997]. In addition to the regions mentioned by Shulman et al., we also found decreased activity in the right postcentral gyrus and the hypothalamus. Future studies will have to indicate if the latter two regions are indeed part of a resting state network, or whether they are incidental findings. The inhibition of this network of ROIs appeared to be related to effort and, in contrast to previous hypotheses [Greicius et al., 2003; McKiernan et al., 2003], relatively insensitive to the demand of information processing.
While there is still much debate over how to interpret task‐induced deactivations, two main views seem to have emerged. One common view is that regions showing deactivation during execution of a task are involved in functions that need to be inhibited in order to execute the task well. This view is based on findings that a fairly consistent network of deactivation is seen for many different tasks [Greicius et al., 2003; Mazoyer et al., 2001; Shulman et al., 1997; Simpson et al., 2001]. The inhibited functions are hypothesized to be related either to a general awareness of the environment or one's body, or to nonconscious processes—for instance, those involved in the recuperation of the brain or used to reinforce new associations. Also, it has been suggested that the vACC plays an important role in linking the “vegetative somatic” functions of the brain associated with the subgenual cingulate region (BA 25), and cognitive functions located in the dorsal part of the ACC, as well as the prefrontal and parietal regions of the brain [Goldapple et al., 2004; Mayberg, 1997].
An alternative view of the default network, proposed by Binder et al. [1999], suggests that whether an increase or decrease is detected is merely related to the design of the experiment. According to Binder et al., regions that are typically found to show deactivations are related to “conceptual thinking,” which Binder et al. argue occurs during conscious rest. The study of Binder et al. showed that deactivations did not occur if a subject performed a conceptual task, which can be explained by the fact that the task also activates regions that belong to the default network. As the task we used was not a conceptual task, it is possible that the task‐induced deactivation we found is similarly related to the inhibition of conceptual language processes.
Our concept of effort as the allocation of resources may seem close to the concept of sustained attention. However, the concept of sustained attention is typically associated with alertness, measured for instance by tasks in which the subject must monitor the frequent occurrence of a certain stimulus [Posner and Raichle, 1998; Sturm et al., 1999]. It can be argued that effort can be manipulated between tasks without affecting the need for sustained attention. In our study all tasks required sustained attention, because they were all reaction time tasks. But the tasks also required different levels of effort. Previous studies have indicated that the right DLPFC and bilateral SPC are important regions for sustained attention [Sturm et al., 1999]. In our study we found for both of these regions a nonsignificant increase in activity with increasing memory‐set size and a decrease (also nonsignificant) for digits. The difference in activity between digits and letters was significant for the rDLPFC. Although these results do not contradict previous findings related to sustained attention, they do suggest that the right DLPFC is also involved in some function related to information processing.
Our findings provide support for a model of effort as the allocation of mental resources, but some limitations have to be taken into account. A possible criticism of our study is that activity that we attributed to effort could also be related to information processing. For instance, activity related to rehearsal of the memory‐set can be expected to increase with the size of the memory‐set, but not to react to the type of stimulus. However, previous studies have shown that the rehearsal of a memory‐set does not lead to a prolonged increase of activity. Jha et al. [ 2000] showed that activity related to maintaining the memory‐set is a function of the size of the memory‐set only for a very short period after it is presented: After about 10 s, activity in the prefrontal cortex is independent of the size of the memory‐set. In our study we presented the first probe stimulus 7200 ms after showing the memory‐set, while the total run for each memory‐set lasted about 40 s. In addition, Rypma et al. [ 1999] showed that the size of the memory‐set was not associated with an increase in activity in the VPFC. It is therefore unlikely that the signal changes we found in the VPFC are related to maintaining the memory‐set. In sum, the VPFC is a likely candidate to play a role in effort, but its precise function cannot be determined from the current study.
We did not include in our study alternative measurements of effort, such as a subjective report by the subject. This may be an interesting addition in a follow‐up study. On the basis of our model the level of effort exerted would only be related to the memory‐set size in the Sternberg task, and not to the probe type. This could be tested in a design that would vary the relative number of digits and letters within a task. In our model this should change the information‐processing activity, but not the activity related to effort. Consequently, it should not change the subjective level of effort as reported by a subject.
Because of the spatial normalization, spatial smoothing of data, and group averaging, the actual spatial resolution of our activation maps is relatively low. Therefore, our study was not sensitive to differences between signals reflecting effort and information processing at a high spatial resolution. In addition, if activated regions showed very large differences in anatomical location among subjects, they may not have been identified in our group activation map as being task‐related. Similarly, brain regions identified as related to the task may not have been active in all subjects.
CONCLUSION
The working memory task in our study evoked activity in line with our expectation that effort involves the allocation of mental resources, as well as activity that conformed to our expectation that information processing involves the use of resources to perform a task. Our results indicate that the right VLPC as well as the vACC and HTM are brain regions possibly involved in the allocation of mental resources. Activity in regions considered pivotal for information processing—the left DLPFC, SPC, dACC, and the lINS—appeared to be unrelated to the level of effort exerted.
REFERENCES
- Barch DM, Braver TS, Nystrom LE, Forman SD, Noll DC, Cohen JD 1997: Dissociating working memory from task difficulty in human prefrontal cortex. Neuropsychologia 35: 1373–1380. [Abstract] [Google Scholar]
- Botvinick MM, Braver TS, Barch DM, Carter CS, Cohen JD 2001: Conflict monitoring and Cognitive control. Psychol Rev 108: 624–652. [Abstract] [Google Scholar]
- Coles MG, Scheffers MK, Fournier L 1995: Where did you go wrong? Errors, partial errors, and the nature of human information processing. Acta Psychol (Amst) 90: 129–144. [Abstract] [Google Scholar]
- d'Esposito M, Detre JA, Alsop DC, Shin RK, Atlas S, Grossman M 1995: The neural basis of the central executive system of working memory. Nature 378: 279–281. [Abstract] [Google Scholar]
- de Zwart JA, Ledden PJ, Kellman P, van Gelderen P, Duyn JH 2002a: Design of a SENSE‐optimized high‐sensitivity MRI receive coil for brain imaging. Magn Reson Med 47: 1218–1227. [Abstract] [Google Scholar]
- de Zwart JA, van Gelderen P, Kellman P, Duyn JH 2002b: Application of sensitivity‐encoded echo‐planar imaging for blood oxygen level‐dependent functional brain imaging. Magn Reson Med 48: 1011–1020. [Abstract] [Google Scholar]
- Duncan J, Owen AM 2000: Common regions of the human frontal lobe recruited by diverse cognitive demands. Trends Neurosci 23: 475–483. [Abstract] [Google Scholar]
- Goldapple K, Segal Z, Garson C, Lau M, Bieling P, Kennedy S, Mayberg H 2004: Modulation of cortical‐limbic pathways in major depression: treatment‐specific effects of cognitive behavior therapy. Arch Gen Psychiatry 61: 34–41. [Abstract] [Google Scholar]
- Grady CL 1996: Age‐related changes in cortical blood flow activation during perception and memory. Ann N Y Acad Sci 777: 14–21. [Abstract] [Google Scholar]
- Greicius MD, Krasnow B, Reiss AL, Menon V 2003: Functional connectivity in the resting brain: a network analysis of the default mode hypothesis. Proc Natl Acad Sci U S A 100: 253–258. [Europe PMC free article] [Abstract] [Google Scholar]
- Jansma JM, Ramsey NF, Slagter HA, Kahn RS 2001: Functional anatomical correlates of controlled and automatic processing. J Cogn Neurosci 13: 730–743. [Abstract] [Google Scholar]
- Jha AP, McCarthy G 2000: The influence of memory load upon delay‐interval activity in a working‐memory task: an event‐related functional MRI study. J Cogn Neurosci 12( Suppl 2): 90–105. [Abstract] [Google Scholar]
- Koechlin E, Basso G, Pietrini P, Panzer S, Grafman J 1999: The role of the anterior prefrontal cortex in human cognition. Nature 399: 148–151. [Abstract] [Google Scholar]
- Manoach DS, Schlaug G, Siewert B, Darby DG, Bly BM, Benfield A, Edelman RR, Warach S 1997: Prefrontal cortex fMRI signal changes are correlated with working memory load. Neuroreport 8: 545–549. [Abstract] [Google Scholar]
- Mayberg HS 1997: Limbic‐cortical dysregulation: a proposed model of depression. J Neuropsychiatry Clin Neurosci 9: 471–481. [Abstract] [Google Scholar]
- Mazoyer B, Zago L, Mellet E, Bricogne S, Etard O, Houde O, Crivello F, Joliot M, Petit L, Tzourio‐Mazoyer N 2001: Cortical networks for working memory and executive functions sustain the conscious resting state in man. Brain Res Bull 54: 287–298. [Abstract] [Google Scholar]
- McKiernan KA, Kaufman JN, Kucera‐Thompson J, Binder JR 2003: A parametric manipulation of factors affecting task‐ induced deactivation in functional neuroimaging. J Cogn Neurosci 15: 394–408. [Abstract] [Google Scholar]
- Owen AM 1997: The functional organization of working memory processes within human lateral frontal cortex: the contribution of functional neuroimaging. Eur J Neurosci 9: 1329–1339. [Abstract] [Google Scholar]
- Petersen SE, van Mier H, Fiez JA, Raichle ME 1998: The effects of practice on the functional anatomy of task performance. Proc Natl Acad Sci U S A 95: 853–860. [Europe PMC free article] [Abstract] [Google Scholar]
- Posner MI, Raichle ME 1998: The neuroimaging of human brain function. Proc Natl Acad Sci U S A 95: 763–764. [Europe PMC free article] [Abstract] [Google Scholar]
- Raichle ME, MacLeod AM, Snyder AZ, Powers WJ, Gusnard DA, Shulman GL 2001: A default mode of brain function. Proc Natl Acad Sci U S A 98: 676–682. [Europe PMC free article] [Abstract] [Google Scholar]
- Rypma B, d'Esposito M 1999: The roles of prefrontal brain regions in components of working memory: effects of memory load and individual differences. Proc Natl Acad Sci U S A 96: 6558–6563. [Europe PMC free article] [Abstract] [Google Scholar]
- Schneider W, Shiffrin RM 1977: Controlled and automatic human information processing. I. Detection, search, and attention. Psychol Rev 84: 1–66. [Google Scholar]
- Shulman GL, Fiez JA, Corbetta M, Buckner RL, Miezin FM, Petersen SE, Raichle ME 1997: Common blood flow changes across visual tasks. II. Decreases in cerebral cortex. J Cogn Neurosci 9: 648–663. [Abstract] [Google Scholar]
- Simpson JR Jr, Snyder AZ, Gusnard DA, Raichle (ME): 2001. Emotion‐induced changes in human medial prefrontal cortex. I. During cognitive task performance. Proc Natl Acad Sci U S A 98: 683–687. [Europe PMC free article] [Abstract] [Google Scholar]
- Smith EE, Jonides J, Marshuetz C, Koeppe RA 1998: Components of verbal working memory: evidence from neuroimaging. Proc Natl Acad Sci U S A 95: 876–882. [Europe PMC free article] [Abstract] [Google Scholar]
- Sturm W, de Simone A, Krause BJ, Specht K, Hesselmann V, Radermacher I, Herzog H, Tellmann L, Muller‐Gartner HW, Willmes K 1999: Functional anatomy of intrinsic alertness: evidence for a fronto‐parietal‐thalamic‐brainstem network in the right hemisphere. Neuropsychologia 37: 797–805. [Abstract] [Google Scholar]
- Thevenaz P, Ruttimann UE, Unser M 1998: A pyramid approach to subpixel registration based on intensity. IEEE Trans Med Imaging 7: 27–41. [Abstract] [Google Scholar]
- Waldvogel D, van Gelderen P, Immisch I, Pfeiffer C, Hallett M 2000: The variability of serial fMRI data: correlation between a visual and a motor task. Neuroreport 11: 3843–3847. [Abstract] [Google Scholar]
Articles from Human Brain Mapping are provided here courtesy of Wiley
Full text links
Read article at publisher's site: https://doi.org/10.1002/hbm.20297
Read article for free, from open access legal sources, via Unpaywall:
https://europepmc.org/articles/pmc6871375?pdf=render
Citations & impact
Impact metrics
Citations of article over time
Smart citations by scite.ai
Explore citation contexts and check if this article has been
supported or disputed.
https://scite.ai/reports/10.1002/hbm.20297
Article citations
Using lickometry to infer differential contributions of salience network regions during compulsion-like alcohol drinking.
Addict Neurosci, 7:100102, 04 May 2023
Cited by: 4 articles | PMID: 38736902 | PMCID: PMC11086682
Parietal Alpha Oscillations: Cognitive Load and Mental Toughness.
Brain Sci, 12(9):1135, 26 Aug 2022
Cited by: 4 articles | PMID: 36138871 | PMCID: PMC9496702
Task effects on functional connectivity measures after stroke.
Exp Brain Res, 240(2):575-590, 02 Dec 2021
Cited by: 3 articles | PMID: 34860257
Losses Motivate Cognitive Effort More Than Gains in Effort-Based Decision Making and Performance.
Front Hum Neurosci, 14:287, 17 Jul 2020
Cited by: 9 articles | PMID: 32765247 | PMCID: PMC7379863
Fatigue in brain tumor patients, towards a neuronal biomarker.
Neuroimage Clin, 28:102406, 01 Sep 2020
Cited by: 5 articles | PMID: 32971465 | PMCID: PMC7509800
Go to all (37) article citations
Similar Articles
To arrive at the top five similar articles we use a word-weighted algorithm to compare words from the Title and Abstract of each citation.
Inefficient executive cognitive control in schizophrenia is preceded by altered functional activation during information encoding: an fMRI study.
Neuropsychologia, 46(1):336-347, 19 Jul 2007
Cited by: 57 articles | PMID: 17707869
The role of the prefrontal cortex in the maintenance of verbal working memory: an event-related FMRI analysis.
Neuropsychology, 19(2):223-232, 01 Mar 2005
Cited by: 108 articles | PMID: 15769206
fMRI abnormalities in dorsolateral prefrontal cortex during a working memory task in manic, euthymic and depressed bipolar subjects.
Psychiatry Res, 182(1):22-29, 15 Mar 2010
Cited by: 80 articles | PMID: 20227857 | PMCID: PMC2918407
Overlapping functional anatomy for working memory and visual search.
Exp Brain Res, 200(1):91-107, 01 Jan 2010
Cited by: 17 articles | PMID: 19756551 | PMCID: PMC2800858
Funding
Funders who supported this work.