Abstract
Free full text

Can genes for mammographic density inform cancer etiology?
Preface
Mammographic density (MD) reflects variation in fat, stromal and epithelial tissues that is thought to be regulated by several genes. High MD is an established risk factor for breast cancer; therefore genes that regulate MD may indirectly influence breast cancer. These genes may also be fewer in number and easier to identify than those for breast cancer risk outside of inherited predisposition syndromes. In this Perspective, we review the limited genetic studies of MD and propose future directions.
Unraveling the genetics of complex diseases can be enhanced by focusing on underlying risk factors. For example, studies on lipoproteins and blood pressure have been shown to correlate well with the risk of vascular disease1, 2. Insights into the pathogenesis of breast cancer may be informed in a similar way using MD because both may be determined by similar genetic variants. In fact, the number of genetic and environmental factors influencing an intermediate such as MD is likely smaller than that influencing the endpoint, namely breast cancer3. Consequently, studies to identify genes for MD could be more powerful and efficient than those for breast cancer.
MD reflects the proportion of stromal and epithelial tissue relative to fat on a mammogram. Dense breast tissue appears light or lucent on radiographs of breast images; in contrast, fat appears dark. Early measures of MD were qualitative, relying on visual scales of morphologic features4, 5. Contemporary methods seek to provide a quantitative and continuous measure of the amount of dense tissue in the mammographic breast image6–9 (Box 2). Results from both measures show that MD is strongly associated with breast cancer risk10. Indeed, the ~30%11 of women who have dense breast tissue in >50% of total breast area have a 3–5 fold increased risk of breast cancer compared to women with no measurably dense breast tissue10, 11. Although the [0]inclusion of MD in breast cancer risk prediction models has minimally improved their overall discrimination among women of mammogram age,12–14 the identification of genes that regulate MD might further enhance the ability to identify women at risk of developing breast cancer. Improved risk prediction will be particularly important for young women, not yet of recommended mammogram screening age for whom prevention efforts will be most beneficial.
Evidence for a Genetic Influence
Evidence for a genetic influence on MD has come from studies on family history, familial aggregation, twin and segregation analyses.
Family History
If the genetic factors that underlie MD increase breast cancer risk, then MD should be associated with family history of breast cancer. Early investigations focused on whether unrelated women with a family history of breast cancer are more likely to have a high MD pattern than women without such a history. Both positive15–17 and negative18–20 results were found. More recently, two large studies of 35,01921 and 6,14622 women from population-based mammography registries have illustrated positive associations between family history of breast cancer and higher MD as determined by BI-RADS21, 22. The odds of a woman having dense breast tissue is 17% greater for a first degree relative with a family history of breast cancer and 22% greater for women with breast cancer that was diagnosed at <50 years of age21.
Familial Aggregation and Twin Studies
Data on related individuals, although based on small sample sizes, show similar mammographic patterns among mother-daughter and sister pairs compared to unrelated pairs23. Familial correlation of percent MD was estimated to be 22% among 275 sister pairs but attenuated to 16% after adjustment for body weight.
Twin studies demonstrated greater concordance of MD patterns among monozygotic compared to dizygotic twin pairs24, 25. A large multi-centered study of 571 monozygotic and 380 dizygotic twin pairs estimated that 60–67% of the variation in MD is attributable to common genetic factors26, 27. Estimates of heritability are stronger when twin pairs are of similar BMI (77% versus 28% when dissimilar on BMI)28. This underscores the importance of accounting for BMI and possibly other covariates when searching for genetic influences on MD.
Segregation Analysis
The above analyses implicate a genetic influence on MD but not the possible mode (Mendelian pattern of inheritance, for example) of transmission of a putative gene(s). In the only reported segregation analysis, the distribution of MD among 1,370 females in 258 independent families was compatible with Mendelian dominant or codominant transmission of a major gene. This was only apparent after adjustment for covariates, including BMI and postmenopausal hormone (PMH) use29. Under the dominant model, 12% of the female population could carry an allele for higher MD, and women carrying at least one dominant allele would have a mean MD that is 27% higher than the rest of the female population29.
The Search for Mammographic Density Genes
Two approaches have been used to identify genes that influence MD: a hypothesis-dependent approach in which knowledge of breast biology guides the selection of candidate genes, and a hypothesis-free approach, in which markers positioned across the genome are evaluated with MD using linkage or genome wide association methodology.
Candidate Gene Analysis
This approach estimates the extent that polymorphisms in a biologically plausible candidate gene influence MD, usually in unrelated individuals. However, our limited understanding of the biology of MD makes selection of candidate genes challenging. Furthermore, MD is a dynamic trait, decreasing with older age and menopause, and even varying transiently during the menstrual cycle. Higher densities are observed among women who are premenopausal, nulliparous, of low BMI or taking combination PMH, and lower densities among those who are postmenopausal, multiparous, of high BMI or on tamoxifen therapy. Because of these hormonal influences the majority of candidate gene studies have focused on evaluation of the pathways that regulate steroid hormone synthesis and metabolism, hormone receptors and proliferative pathways including the insulin-like growth factor (IGF) pathway. However, some studies have focused on genes previously noted to be strongly (BRCA1/BRCA2) or hypothetically (XPD, XRCC3, and ERBB2) associated with breast cancer risk. The results from all of these candidate gene association studies are reviewed in the online Table and some are highlighted below. It should be noted that the size of these studies vary greatly, as do the aims of the studies, the characteristics of the women analyzed and the method of assessing MD, which makes interpretation challenging.
Characteristics of Studies
The majority of studies compared a difference in MD among women with 1 or 2 copies of a polymorphism to women with 0 copies and also estimated tests for trend of a dose-response of 0, 1 or 2 copies. Of 25 identified publications (Table 1), three studies examined several polymorphisms representing genetic variation throughout the entire gene and used haplotype approaches for analyses30–32 while the remainder largely evaluated candidate polymorphisms with putative functional effects that were previously examined with breast cancer. Six studies (24%) had fewer than 300 women33–38, 14 (56%) had between 300–650 women31, 39–51 and five (20%) had between 1,100–2,000 women30, 32, 52–54. All studies except four investigations of BRCA1/2 mutation carriers33–36 and one cross-sectional study 53 estimated MD using the computer-assisted thresholding method.
Table 1
Characteristics of genetic association studies of mammographic density*.
Author, year | Study/ Setting | Design | Subject selection | Participants | Density Assessment | Genes studied | Covariates† |
---|---|---|---|---|---|---|---|
Helvie, 199733 | Michigan, U.S. | Case-series | Clinic-based | 9 mutation + cases | BI-RADS | BRCA1/2 | None; visual assessment only |
Chang, 199934 | Singapore/ Hong Kong | Case-series | Clinic-based | 9 mutation + cases; 19 mutation – cases | Visual assessment, 5-point scale | BRCA1/2 | A |
Huo, 200035 | Chicago, U.S. | Retrospective | Screening mammogram clinic & cancer risk clinic | 15 mutation + cases; 30 “low risk” women | Computer based, 7-point scale | BRCA1/2 | A |
Tilanus-Linthorst, 200236 | Netherlands | Cross-sectional | Clinic breast cancer cases, mammogram at time of diagnosis | 34 mutation + cases; 34 sproadic cancers | BI-RADS | BRCA1/2 | A, U |
Haiman, 200239 | Los Angeles, U.S. | Cross-sectional | Cancer registry cases with pre-diagnosis mammogram | 176 Premenopausal 171 Postmenopausal | Computer based, USC | CYP17A1, COMT, HSD17β1, HSD3β1 | A–H |
Lillie, 200440 | 191 Premenopausal 195 Post menopausal | Computer based, USC | AR | A–C | |||
Haiman, 200341 | U.S. | Cross-sectional | Control subjects in a case-control study of breast cancer | 94 Premenopausal 392 Postmenopausal | Computer based, UT | CYP17A1, CYP19A1, COMT, CYP1A1, CYP1B1, UGT1A1, AR, AIB1 | A, C–F, I |
Tamimi, 200730 | 204 Premenopausal 917 Postmenopausal | Computer based, UT | IGF-1, IGFBP-1, IGFBP-3 | A, C–F, H, I, X | |||
Hong, 200342 | Toronto, Canada | Cross-sectional | Screening mammogram clinic | 181 Premenopausal 171 Postmenopausal | Computer based, UT | COMT | A, B |
Hong, 200443 | 181 Premenopausal 173 Postmenopausal | Computer based, UT | CYP17A1 | A–C, E, G, H, J | |||
Mulhall, 200544 | 177 Premenopausal 171 Postmenopausal | Computer based, UT | GH1, GHRHR | A–D | |||
Lai, 200445 | 206 Premenopausal 206 Postmenopausal | Computer based, UT | IGF-1, IGFBP-3 | A, C, E, I, S | |||
Maskarinec, 200446 | Hawaii, U.S. | Cross-sectional and 2 dietary intervention trials | Screening mammogram clinic | 268 Premenopausal 60 Postmenopausal | Computer based, UT | CYP17A1, COMT, CYP1A1, CYP1A2, CYP1B1 | A–F |
Lord, 200537 | Los Angeles, U.S. | MD changes over time | Subjects in a randomized control trial of PMH & atherosclerosis | 232 Postmenopausal | Computer based, USC | COMT, CYP1B1, UGT1A1, AKR1C4 | A–C, L–P |
Van Duijnhoven, 200547 | Netherlands | Cross-sectional | Large prospective cohort participants | 181 Premenopausal 432 Postmenopausal | Computer based, UT | ESR1 | A, C, D–H, K, I, Q |
Van Duijnhoven, 200652 | Netherlands and U.K. | MD changes over time | 781 non PMH users 795 PMH users | Computer based, UT | ESR1, PGR | A, C–E, G–I, K, N, P–R | |
Verheus, 200732 | Netherlands | Cross-sectional | 1,928 Premenopausal | Computer based, UT | IGF-1 | C (no observed effect from A,C–G, K, Q, R) | |
MD changes over time | 656 Premenopausal women who became Postmenopausal during an average 4.4 years | Computer based, UT | IGF-1 | None (no observed effect from A, D–G, K, M, N, Q, R) | |||
Warren, 200653 | U.K. | Cross-sectional | Large prospective cohort participants | 1,260 Postmenopausal non PMH users | 6-category Boyd scale | CYP17A1, CYP19A1, COMT, CYP1B1, ESR1, PGR, SHBG | B, E, G, K, L |
dos Santos Silva, 200648 | U.K. | Cross-sectional | Large prospective cohort participants | 215 Premenopausal 238 Postmenopausal | Computer based, UK | IGFBP-3 | A, C, F, J, K, L, Q, T |
Mitchell, 200649 | U.K. | Cross-sectional | Large prospective cohort participants | 206 mutation + carriers; 136 mutation – relatives | Computer based, UT | BRCA1/2 | A, C–E |
Takata, 200738 | Hawaii and Los Angeles, U.S. | Average of repeated mammogram assessments at time of breast cancer diagnosis | Nested case-control study, pre-treatment mammograms for cases | Cases: 92 Premenopausal 233 Postmenopausal Controls: 97 Premenopausal 153 Postmenopausal | Computer based, UT | COMT, CYP1A2 | B–H, V, W |
Olson, 200731 | Upper mid-west, U.S. | Cross-sectional | Control subjects in a case-control study of breast cancer | 550 women | Computer based, UT | CYP19A1 | A, C–F,Y |
Olson, 200855 | 402 Postmenopausal 136 Premenopausal | Computer based, UT | COMT | A, C–F,Y | |||
Stone, 200750 | Australia | Cross-sectional and within-sister pair analysis | Twin and sister-pairs (104 MZ, 182 DZ and 171 singletons) | 150 Premenopausal | Computer based, UT | COMT, HSD3β1, IGFBP-3, HER2, XPD, XRCC3 | A, C–E, K |
Lee, 200851 | Los Angeles, U.S. | Cross-sectional | Cancer registry cases with mammogram | 516 women | Computer based, USC | rs889312 – chr5q C>A rs3803662 – chr16q T>C rs3817198 – chr11p LSP1 T>C rs13281615 – chr8q A>G rs13387042 – chr2q A>G rs2981582 – chr10q FGFR2 T>C | A–D |
Diorio, 2008 54 | Quebec, Canada | Cross-sectional | Screening mammogram clinic | 741 Premenopausal | Computer based, UT | IGF-1, IGFBP-3, IGF1R, IRS1, PIK3CB | C, E, J, K, V, Z (no observed effect from F–I, O, Q, R, AA–EE |
Most studies adjusted statistically for age and BMI, and also adjusted or stratified by menopausal status30, 31, 38, 39, 41–48, 50, 55 or PMH use31, 38–48, 55 or restricted analyses to women who did37, 52 or did not47, 53 take PMH, or women who were premenopausal32, 54. Although stratification produces homogeneous subsets of women to examine associations between genetic factors and MD, the resulting sample sizes can be too small to produce any meaningful conclusions. The populations also varied and included women from screening mammogram populations who were42–44 or were not45, 46, 54 selected for extreme MD phenotypes, control subjects in case-control studies of breast cancer30, 31, 41, 55, healthy women participating in cohort studies32, 47, 48, 52, 53, breast cancer cases with pre-diagnosis mammograms39, 40, 51, and a twin study50. The highly-selected sample from a cardiovascular-focused clinical trial37 will not be discussed in the remainder of this Perspective.
Below, we focus our discussion on those polymorphisms evaluated in >1 study of percent MD with similar sample characteristics, and we seek to explain similar or disparate findings. The differences in absolute dense and non-dense area in relation to the polymorphisms will not be discussed.
CYP17A1 +27T>C
This polymorphism is located in the cytochrome P450 17A1 promoter region but its effect on steroid hormone biosynthesis is inconclusive56 (Figure 1). Inappreciable differences in percent MD across genotypes were observed in four studies restricted to 94 to 268 premenopausal women and regardless of age (< 50 years versus > 50 years), study design (mammograms from prospectively enrolled healthy women versus pre-diagnosis mammograms from breast cancer cases), and ethnicity39, 41, 42, 46. Non-significant associations were also observed among samples of 171 to 1,260 postmenopausal women irrespective of current39, 41 or past or never39, 53 PMH use. The data, therefore, provide little support for a role of this polymorphism in MD or in breast cancer57.
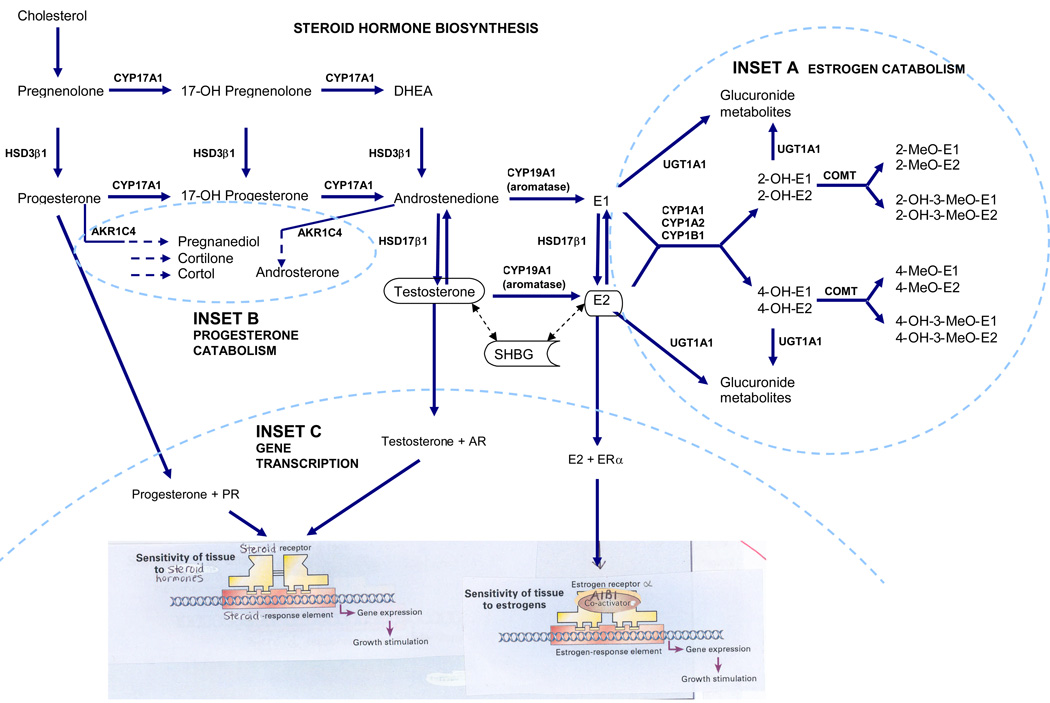
Steroid hormone biosynthesis. The cytochrome p450 (CYP) 17A1 enzyme catalyzes the conversion of pregnenolone and progesterone to the hormones, dehydroepidandrosterone (DHEA) and androstenedione, respectively, which are further metabolized to estrone (E1) and 17β-estradiol (E2) by the CYP19A1 (aromatase) enzyme. Androgen conversion to estrogen in adipose tissue by CYP19A1 is an important source of bioactive endogenous estrogens among postmenopausal women.The hydroxysteroid dehydrogenase (HSD) enzyme HSD3β1 catalyzes the interconversion of pregnenolone and progesterone and of DHEA and androstenedione, while HSD17β1 catalyzes the interconversions of androstenedione and testosterone and of E1 and E2. Testosterone and E2 bind to the serum sex hormone binding globulin (SHBG), which regulates the bioavailability of these hormones in target cells. A. Estrogen catabolism. The CYP1 family of enzymes are phase I enzymes that function in the oxidative metabolic activation and deactivation of compounds including several steroid hormones. E1 and E2 preferentially undergo 2-hydroxylation (2-OH-E1 or -E2) by the CYP1A1 and 1A2 enzymes, whereas E1 and E2 preferentially undergo 4-hydroxylation (4-OH-E1 or -E2) by CYP1B1. Catecholestrogens are deactivated by catechol-O-methyltransferase (COMT). UDP-glucuronosyltransferase (UGT) 1A1 is a detoxifying enzyme, which converts endogenous substrates including E1 or E2 and their metabolites to inactive hydrophilic glucuronides. B. Progesterone catabolism. The aldoketo reductase family 1, member C4 (AKR1C4) enzyme catalyzes the conversion of progesterone and androstenedione to their corresponding alcohols. C. Gene transcription. Progesterone, testosterone and E2 bind to their respective nuclear receptor proteins, progesterone receptor (PR), androgen receptor (AR) and estrogen receptor alpha (ERα), and activate genes with corresponding responsive elements. The steroid receptor coactivator protein, amplified in breast-1 (AIB1) interacts with ERαin a ligand-dependent manner to increase estrogen-dependent transcription.
COMT Val158Met
The COMT enzyme inactivates the bioactive 2- and 4- hydroxy catechol estrogens by methylation58 (Figure 1). The Val158Met polymorphism decreases enzyme activity58 and is the most widely studied polymorphism with MD. Among healthy premenopausal women41, 42, 46, carriers of two copies of the Met allele had higher MD in one study of Caucasians participating in a case-control study of breast cancer41, and lower MD in two studies of women enrolled from a screening mammography clinic42, 46. The latter two studies comprised mixed ethnicities including Asians and, although adjustment was made for ethnicity, this and the younger age distribution compared to the study by Haiman et al41 may account for the differences among relatively healthy premenopausal women. The remaining studies of COMT Val158Met among premenopausal women included breast cancer patients whose screening mammograms were retrieved within the five years preceding diagnosis39 or whose mammograms were performed after breast cancer diagnosis but prior to treatment38 showed only minor differences in MD across COMT genotypes, and the study by Takata et al38 did not evaluate healthy controls separately from cases by menopausal status.
Two studies which enrolled 6739 and 1,26053 postmenopausal women who never used PMH or used PMH in the past showed no particular pattern of percent MD across COMT genotypes. Investigations of postmenopausal women that did not differentiate among hormonal regimens but which adjusted for PMH use reported either no significant differences of MD across COMT genotypes42, 50 or significantly lower MD among carriers of both Met alleles41. The two studies41, 53 closest in comparability due to similar age distributions and study populations (healthy women participating in a nested case-control or prospective cohort study) had disparate findings, which could be attributable to the three fold difference in sample sizes, the statistical adjustment for PMH use41 versus sample restriction to past or never PMH use53, or to method of mammogram assessments (computer assisted39, 41 versus visual assessment by three radiologists using the Boyd six-category scale53). Statistical adjustment for PMH use or menopausal status without evaluation of the assumption that the effect is constant across strata of these variables results in pooled estimates that can conceal important stratum-specific effects. Also, although both MD assessment methods are shown to predict risk of breast cancer10, classification of MD using the Boyd categorical 6-point scale can overestimate risk of breast cancer among women aged >50 years compared to the computer-assisted threshold assessment method 7. Thus, an ideal comparison of results by genotype necessarily relies on similar methodology. In summary, although the COMT Val158Met polymorphism does not appear to be a strong risk factor for breast cancer59 regardless of menopausal status57, the data suggest it may influence premenopausal MD.
ESR1 XbaI and PvuII
Estrogen receptor alpha is a nuclear receptor that mediates estrogen or other steroid hormone action by regulating gene transcription (Figure 1). The two polymorphisms in intron 1 of ESR1 (known as XbaI and PvuII) have no known functional effect on gene expression57, 60, although intronic polymorphisms may affect the post transcriptional processing of gene products and affect receptor binding affinity.
Three studies examined the XbaI polymorphism. The European Prospective Investigation into Cancer and Nutrition (EPIC) cohorts analyzed cross-sectional estimates of MD across XbaI genotypes from 620 postmenopausal women in the Netherlands (EPIC-Prospect) who never used PMH47 and 1,286 postmenopausal women in the United Kingdom (EPIC-Norfolk) who were past or never users of PMH53. While the former study47 reported a significantly higher MD with increasing copies of the x variant allele, the latter study53 reported no appreciable difference in percent MD across genotypes. The greater sample size in the latter study53 is a strength, although MD assessments were subjective and differed from the computer-assisted method used in the EPIC-Prospect study. In a third study52, the computer-assisted method was used for all MD readings among mostly (~70%) postmenopausal women participating in the combined EPIC Prospect and Norfolk cohorts. In this study, the densities of 781 women who never used PMH were compared at baseline and at 5 years of follow-up across XbaI genotypes, thereby reflecting a natural change in MD during this time period. In addition, mammographic densities were compared across XbaI genotypes among 795 women who never used PMH at baseline but who subsequently initiated PMH use during the 5 years of follow-up, thereby reflecting the influence of PMH use during this period. The results suggested that the women who carried at least one x allele had significantly attenuated declines in MD if they took PMH52. The PvuII polymorphism, which is in linkage disequilibrium with the XbaI polymorphism, was also studied in the two aforementioned investigations and shows similar results47, 52. Confirmation of these findings by independent investigators would suggest that PMH use interacts with the XbaI and PvuII polymorphisms to moderate the natural decline in MD associated with the postmenopausal years, possibly to influence breast cancer risk, but this remains to be determined. The PvuII p allele and the XbaI x allele have each been associated with breast cancer61–63.
PGR PROGINS complex
Two (Val660Leu and His770His) of three polymorphisms in the progesterone receptor (PGR) gene are in complete linkage disequilibrium and together all three are called the PROGINS complex64 (Figure 1): they may affect ligand binding and gene transcription65. In the EPIC-Prospect47 and EPIC-Norfolk53 studies, neither Val660Leu or His770His were associated with cross-sectional estimates of MD among postmenopausal women who had not used or who were past users of PMH53, nor with longitudinal change in MD among women who used PMH52. Each copy of the Val660Leu allele was reported to increase breast cancer risk by a modest 8% (P = 0.05)66.
IGFBP-3 −202C>A
This polymorphism is located in the promoter region of the gene and may increase serum IGFBP-3 levels67. It has been investigated by five groups. Examination of 215 healthy premenopausal women participating in a prospective study of breast cancer in the United Kingdom48 and 741 healthy premenopausal women attending a mammography clinic in Quebec, Canada54 showed no influence of the genotype on MD. A third study among 206 healthy premenopausal women attending an outpatient clinic in Toronto, Canada showed a significantly higher MD among women with two copies of the A allele45. Each study used a computer-assisted method to assess MD. However, none of the studies defined the ethnic composition of their sample of women or adjusted for ethnicity. The potential for population stratification among a potentially multi-ethnic sample residing in Toronto45 with the UK48 and French Canadian54 participants warrants further exploration. In addition, postmenopausal women who never used PMH or used PMH in the past45, 48 showed no appreciable difference in percent MD across genotypes of this polymorphism. Also, two additional studies, the Australian twin study of 307 postmenopausal women50 and the Nurses’ Health Study of 917 postmenopausal controls that participated in a case-control study of breast cancer30 found no difference in MD across genotypes following adjustment for PMH use. In summary, the data suggest this polymorphism is not associated with MD regardless of menopausal status, although the C allele has been associated with lower circulating levels of IGFBP-368 and marginally significant decreased breast cancer risk66.
IGF-I rs1520220 C>G and rs6220 A>G
The rs1520220 C>G polymorphism is located in intron 3, while rs6220 A>G is located in the 3′ UTR; neither polymorphism’s function is known. Both were evaluated in two studies of premenopausal women comprising large samples of healthy women attending a mammography clinic (746 in Quebec, Canada54 and 1,928 in the Netherlands32). Only the Canadian study observed a significantly higher percent MD among women who carried two copies of the rs1520220 G allele54 and both studies observed a marginally significantly higher percent MD with two copies of the rs6220 G allele32, 54. Both studies32, 54 also showed higher serum IGF-I with each polymorphism, although significance was attained with two copies of the rs6220 only32. Rs6220 A>G has also been associated with increased breast cancer risk69.
A third study among healthy (204 premenopausal and 917 postmenopausal) women in the Nurses’ Health Study observed significantly lower percent MD among those with both copies of the rs1520220 G allele, but no apparent difference was noted by menopausal status30. The evidence thus far does not support a consistent relation of rs1520220 C>G with MD or with breast cancer risk70, 71, although rs6220 A>G may be a potential candidate for premenopausal MD.
HSD3β1 Asn367Thr
One seemingly consistent finding among genes in the steroid hormone biosynthesis pathway (Figure 1) is the lower level of MD among Caucasian carriers of the Asn367Thr variant of hydroxysteroid dehydrogenase 3β1 (HSD3β1). This polymorphism has no known function. A cross-sectional study of 242 (~50% postmenopausal) Caucasian breast cancer patients with pre-diagnosis mammograms found a significantly lower percent MD among women with one or more copies of the Thr allele compared to no copies39. This finding was confirmed in a study of 457 healthy Caucasian women (~67% postmenopausal) from 207 sisterhoods that also reported a significantly lower MD from one or more copies of the Thr allele50. Analyses restricted to 176 premenopausal women with two copies of the Thr allele displayed a non-significantly higher MD in the former study39, but a marginally significantly lower MD in the latter study of 150 premenopausal women50. Potential reasons for the discrepancy could be variability from small sample sizes within subgroup analyses, the comparison of mammographic densities obtained retrospectively from women who were recruited as breast cancer cases with those of a selected group of twin-pairs, and the slightly lower age among the breast cancer cases compared to the twin-pairs, suggesting an enrichment of younger, higher-risk women in this39 study. Although lower MD is seen for the Thr allele among Caucasian women after adjusting for menopausal status, the associations are inconsistent among premenopausal women, and not significant among postmenopausal women. The relevance of this polymorphism to MD, therefore, remains unclear. The association between the Asn367Thr variant and breast cancer risk has not been studied.
CYP19A1 (TTTA)n
The cytochrome P450 19A1 gene, also known as aromatase, contains a microsatellite repeat polymorphism that may alter the splice site during post-translational protein modification72; however no functional effect of the polymorphism on estrogen biosynthesis is known73 (Figure 1). Two studies of 48641 and 55031 Caucasian women found no statistical association with MD among carriers of the 10 repeat allele compared to carriers without this allele in models that adjusted for age, BMI and menopausal status31, 41. In the same studies, carriers of the 12 repeat allele had marginally significantly lower MD levels than women without this allele in one31 but not in the other41 investigation. Both were case-control studies of breast cancer and used the computer-assisted thresholding method of MD assessment among controls. Differences in SNP associations with MD may be from chance or from subtle ancestral differences among the Caucasian samples. Additional analyses of haplotypes that captured variation across the entire CYP19A1 gene with MD were not statistically significant31. Thus, the association of the CYP19A1 12 repeat allele with MD is inconclusive, and does not appear to be associated with breast cancer57, 74, 75.
CYP19A1 +27(TCT)+/- and +268T>C
CYP19A1 +27(TCT)+/- is an insertion/deletion polymorphism in intron 4 and is in linkage disequilibrium with CYP19A1 (TTTA)n. CYP19A1 +268T>C resides in exon 10 of the 3′ untranslated region (UTR) of the gene, but has no known function (Figure 1). Neither polymorphism was significantly associated with percent MD among 550 Caucasian women participating as controls in a case-control study of breast cancer in the United States regardless of menopausal status or PMH use among postmenopausal women31 or among 1,286 postmenopausal women of unreported ethnicity participating in a prospective cohort study in the United Kingdom who were past or never users of PMH53. A third study among 486 Caucasian controls in the United States also did not observe a significant association of the +268T>C polymorphism following adjustment for age and BMI and irrespective of menopausal status or use of PMH among postmenopausal women41. In the absence of additional data, these polymorphisms do not appear to be associated with MD nor appear to be breast cancer risk factors74, 75.
AR CAG repeat polymorphism
Two studies investigated this polymorphism in the androgen receptor gene (Figure 1). Longer transcripts may produce less active receptor76, 77. MD did not vary by number of AR CAG repeats (≥ 22) among 538 (~73% postmenopausal) healthy Caucasian women participating as controls in a study of breast cancer41 or by ≥ 21 AR CAG repeats among 246 (~50% postmenopausal) Caucasian women with breast cancer whose screening mammograms were retrieved within the five years preceding their cancer diagnosis40. The former study41 did not observe an effect on percent MD from PMH use, although women in the latter study40 with the longer less active AR allele had higher MD if they also took a combination formulation of estrogen plus progesterone PMH. The associations with MD may reflect a large number of comparisons in the data of relatively small numbers of women40. Moreover, the AR polymorphism is inconsistently associated with breast cancer risk78, 79.
Tumor suppressor genes
Over 750 protein-truncating mutations exist in BRCA1 and BRCA280, 81. Findings from early studies were mixed33–36 and relied on varied methods of qualitative assessments of MD in addition to very small sample sizes of 9 to 34 mutation carriers (Table 1). A recent well-designed study using the computer-assisted thresholding assessment found no difference in MD between 206 mutation carriers and 136 noncarriers after adjustment for age at mammography and BMI49 (online Table). Although strong risk factors for heritable breast cancer, these mutations are not associated with MD.
CYP1A2 −154A>C (CYP1A1*1F)
This polymorphism may induce enzyme activity82 to lower bioactive estrogen concentrations and, hypothetically, MD. A marginally significantly lower MD was observed overall among 268 pre- and 60 postmenopausal women screened in a mammography clinic, and particularly among premenopausal women46, whereas a statistically significantly higher MD was reported among control subjects from a case control study of breast cancer in 97 pre- and 153 postmenopausal women38. Interestingly, both studies sampled from among female residents in Hawaii. The former study46 composed healthy women with an entry (baseline) mammogram, while the latter study38 incorporated healthy women participating in two nested case-control studies nested within the Multiethnic Cohort Study who each had an average of 2.5 mammograms in order to reduce intra-individual variability in MD measurements. Given similarities in computerized mammographic assessments and adjustment with similar covariates, the findings from the latter study38 may be more reliable due to increased precision of the measure. With only two studies, the influence of the polymorphism with MD is inconclusive, as is its association with breast cancer in the Shanghai Breast Cancer Study83, although decreased risk has been observed among predominantly postmenopausal women in the Multiethnic Cohort Study59.
CYP1A1 Ile462Val and CYP1B1 Val432Leu
Higher CYP1A1 enzyme expression82 or activity84 and CYP1B1 enzyme activity85, 86 may lower bioactive estrogen concentrations (Figure 1). Neither polymorphism is associated with MD among predominantly premenopausal46 or predominantly postmenopausal41 healthy women. Also, CYP1B1 Val432Leu was not associated with MD among 1,260 cohort members who were past or never users of PMH53. MD among 140 current users of PMH with both copies of the CYP1B1 Leu allele, however, was lower than Val/Val carriers, although of borderline significance only41. This relation among current users was not evaluated in the other studies and requires confirmation. Neither polymorphism is strongly associated with breast cancer 57, 59, 84, 87.
Summary
The hypothesis-dependent approach focusing on candidate genes that may influence breast cancer has produced mostly conflicting results regardless of whether the polymorphisms have known functional effects (Table 2). Indeed, the accumulated evidence indicates that the COMT Val158Met and IGF-I rs6220 3′ UTR polymorphisms (or variants in strong linkage disequilibrium with these polymorphisms) influence percent MD in premenopausal women, and the ESR1 intronic Xba I and Pvu II variants may lead to higher percent MD among women taking PMH. The remaining studies are inconclusive, mostly from too few independent investigations or from between-study heterogeneity in sample characteristics.
Table 2
Summary of association studies between polymorphisms and mammographic density.
No significant effect in >1 study | Mixed results* | Significantly lower density observed in the only study to examine it | Significantly lower density in >1 study | Significantly higher density in the only study to examine it | Significantly higher density in >1 study |
---|---|---|---|---|---|
CYP17A1+27T>C39, 41, 43, 46, 53 | CYP19A1 +268T>C41, 53 | COMT +701A>G 55 | HSD3β1 Asn367Thr among Caucasians adjusted for menopausal status39, 50 | HSD3β1 Asn367Thr among African Americans 39 | ESR1† Xba I47,52Pvu II47,52 among postmenopausal women taking PMH |
CYP1A1 M2A>G 41, 46 | CYP1A2*1FA>C38, 46 | IGF-1 −26989G>A30 | COMT Val158Met among premenopausal women adjusted for ethnicity 42, 46 | AKR1C4† Leu311Val 37 | IGF-1 +1830T>C 31,53 among premenopausal women |
PROGINS complex† 51,52 | CYP1B1† Val432Leu 37, 41, 46, 53 | IGF-1 T>C rs7136446 (change in density from pre to post menopause)32 | PGR † +331G>A52 | ||
IGF-1 −17526T>C30, 32, +4996C>A29,31, C>A rs154959330,54 | COMT Val158Met37–39, 41, 50, 53 among postmenopausal women | PIK3CB T>C rs36107254 | AIB1 (CAG)n repeat 41 | ||
BRCA1/BRCA232,35,48 | UGT1A1† (TA)n TAA repeat 37, 41 | IGF-1 (CA)n repeat 45 | |||
AR (CAG)n repeat40, 41 | IGF-1 A>G rs795654732 | ||||
IGF-1 3′ UTR G>A30, 32, −178C>G30, 32, 54 | IGFBP-1 −574G>A30 | ||||
IGFBP-3 −202C>A45, 48, 50, 54 | GH1 Val26Val 44, Gly30Gly44 |
Linkage Analysis
A major limitation of candidate gene studies is the low prior probability that an association is true88. Selecting candidate genes in a region with prior evidence of genetic linkage directly addresses this weakness. Because the regions identified by linkage analysis are hypothesis-independent, they can identify a gene or genes that are not anticipated. In multifactorial complex traits like MD this is particularly important.
Two linkage analyses of MD have been conducted. Both used a large breast cancer family study as the sampling frame. The first was a small sibpair study of 71 sisterhoods in 22 families and used 147 microsatellite markers placed across the autosomes at a spacing of 30 centiMorgans (cM). The results indicated a possible linkage to chromosome 689. The second larger study included 889 members of 89 multigenerational families and 403 markers spaced an average of 9 cM apart90. The strongest evidence for linkage was identified on chromosome 5p with a maximum log odds for linkage (or LOD score) of 2.9. Finer mapping of this region with a dense set of markers strengthened the evidence for linkage (LOD=4.2). The 1-LOD region which contains 45 genes has not been previously implicated in breast cancer and is currently being pursued. Further, although this locus was not linked to BMI, it was only apparent after adjustment for BMI, and could explain up to 22% of the total variability in MD among women. Two other suggestive regions for linkage were also identified on chromosome 12, one containing the IGF-1 gene. Linkage analysis, then, can result in identification of novel genes that may influence both MD and breast cancer risk.
Genome Wide Association Studies
Genome wide association studies (GWAS) utilize a large number of polymorphisms positioned across the genome. Polymorphisms are not confined to coding or regulatory regions and the function of the majority of these polymorphisms is unknown. No GWAS has been reported for MD, but six polymorphisms identified from three GWASs of breast cancer91–93 have been evaluated by one investigative team in relation to MD (online Table). In this study of 516 Caucasian breast cancer cases, Lee et al.51 observed no association of these variants with MD using the mammogram of the contralateral (non-cancerous) breast, although a positive association with MD of rs3817198 T>C within the lymphocyte-specific protein 1 (LSP1) gene on chromosome 11p was seen specifically in women with ER+/PR+ tumors. One of the LSP1 protein functions is to regulate adhesion to fibrinogen matrix proteins, which may have implications for MD.
Summary and Future Directions
In summary, there is strong evidence for a genetic component to MD. Both hypothesis-dependent and hypothesis-free approaches have provided clues to relevant genes and/or loci. Candidate gene approaches yielded replicated associations between COMT Val158Met and IGF-I rs6220 A>G and premenopausal MD and ESR1 (Xba I and Pvu II) polymorphisms and postmenopausal MD, which possibly translate to breast cancer risk. However, many polymorphisms have only been examined in a few studies, limiting the conclusions that can be drawn (Table 2). Technological advances make genome-wide scans feasible and may reveal novel candidate genes, while haplotype block or tagSNP approaches are advantageous to study all common variation within a gene. Linkage analyses identified a candidate region on chromosome 5p encompassing 45 genes that are also under consideration for their influence on MD.
Future investigations should incorporate well-characterized study populations including specification of age, race and ethnicity, quantify MD and its changes in the most objective manner possible, stratify subjects by design (rather than post-hoc) by menopause and specific type of hormone regimen with mammographic densities reflecting the timing of PMH exposure, and consider assessment of earlier periods in life when MD changes are most dramatic and the inter-individual variation is greatest. Several protocols reviewed in this Perspective drive these recommendations and are strong studies for future comparisons and synthesis32, 48, 52. Also, the reviewed studies underscore the importance of measuring and accounting for non-heritable factors to allow better detection of genetic influences on MD. Several of the polymorphisms examined thus far have inconclusive functional significance, requiring research to understand tissue response to genetic polymorphisms that alter local biosynthesis, metabolism or catabolism of hormones or growth factors. Ultimately, identification of genetic risk factors for early markers of disease may permit better risk prediction and targeted early preventive or therapeutic strategies for breast cancer.
Textbox 1 Glossary terms
Stroma | The supportive framework of a biologic tissue with an extensive extracellular matrix that serves to support cells, separate tissues and regulate intercellular communication. |
Familial aggregation | A tendency for the trait under study to occur or cluster in multiple family members more often than would be expected by chance. |
Parent-offspring/twin studies | Investigates the proportion of variation in the trait under study that is explained by unmeasured additive genetic factors. |
Segregation analysis | Tests hypotheses about whether the existence of major genes account for the observed pattern of familial aggregation of the trait and provides evidence for the mode of inheritance of the genes using pedigree data. Statistical models compare Mendelian inheritance patterns of a trait to a model in which there are no restrictions on mode of inheritance or other model parameters. |
Linkage analysis | The process of determining the approximate chromosomal location of a gene associated with the trait being studied by looking for evidence of cosegregation with other marker genes whose locations are already known. |
Genetic association studies | Tests whether a polymorphism in a specific candidate gene explains the inter-individual variation in the trait being studied. |
Linkage disequilibrium (LD) | The nonrandom association of alleles at two or more loci on chromosomes in a population more than would be expected by chance. |
Haplotype blocks | Configurations of alleles within regions on the chromosome which tend to be inherited together (that is, in high LD). Little genetic variability is observed within this region among individuals in a population. |
tagSNPs | A reduced set of single nucleotide polymorphisms that identify or “tag” other SNPs with which it is in high LD. |
References
Full text links
Read article at publisher's site: https://doi.org/10.1038/nrc2466
Read article for free, from open access legal sources, via Unpaywall:
https://www.ncbi.nlm.nih.gov/pmc/articles/PMC2818036
Citations & impact
Impact metrics
Citations of article over time
Smart citations by scite.ai
Explore citation contexts and check if this article has been
supported or disputed.
https://scite.ai/reports/10.1038/nrc2466
Article citations
Modulation of innate immune response to viruses including SARS-CoV-2 by progesterone.
Signal Transduct Target Ther, 7(1):137, 25 Apr 2022
Cited by: 16 articles | PMID: 35468896 | PMCID: PMC9035769
Mammographic breast density and IGF-1 gene polymorphisms rs1520220, rs2946834 and rs6219 in Polish women.
Contemp Oncol (Pozn), 25(3):191-197, 05 Oct 2021
Cited by: 1 article | PMID: 34729039 | PMCID: PMC8547182
Single nucleotide polymorphisms of insulin-like growth factor gene and mammographic breast density.
Prz Menopauzalny, 19(4):160-170, 01 Dec 2020
Cited by: 0 articles | PMID: 33488326 | PMCID: PMC7812538
Association of Catechol-O-methyltransferase polymorphism Val158Met and mammographic density: A meta-analysis.
Gene, 624:34-42, 01 May 2017
Cited by: 2 articles | PMID: 28473194
Leukocyte telomere length and its association with mammographic density and proliferative diagnosis among women undergoing diagnostic image-guided breast biopsy.
BMC Cancer, 15:823, 30 Oct 2015
Cited by: 3 articles | PMID: 26519084 | PMCID: PMC4628256
Go to all (32) article citations
Data
Data behind the article
This data has been text mined from the article, or deposited into data resources.
BioStudies: supplemental material and supporting data
SNPs (Showing 9 of 9)
- (3 citations) dbSNP - rs6220
- (3 citations) dbSNP - rs1549593
- (2 citations) dbSNP - rs7965399
- (2 citations) dbSNP - rs1019731
- (1 citation) dbSNP - rs2946834
- (1 citation) dbSNP - rs7136446
- (1 citation) dbSNP - rs3817198
- (1 citation) dbSNP - rs361072
- (1 citation) dbSNP - rs7956547
Show less
Similar Articles
To arrive at the top five similar articles we use a word-weighted algorithm to compare words from the Title and Abstract of each citation.
Estrogen pathway polymorphisms and mammographic density.
Anticancer Res, 31(12):4369-4386, 01 Dec 2011
Cited by: 25 articles | PMID: 22199302
Review
Breast cancer risk associated with genotype polymorphism of the catechol estrogen-metabolizing genes: a multigenic study on cancer susceptibility.
Int J Cancer, 113(3):345-353, 01 Jan 2005
Cited by: 81 articles | PMID: 15455371
Common genetic variation and novel loci associated with volumetric mammographic density.
Breast Cancer Res, 20(1):30, 17 Apr 2018
Cited by: 14 articles | PMID: 29665850 | PMCID: PMC5904990
Funding
Funders who supported this work.
NCI NIH HHS (7)
Grant ID: P50 CA116201-04
Grant ID: R01 CA97396
Grant ID: P50 CA116201
Grant ID: R01 CA097396-05
Grant ID: R01 CA097396
Grant ID: R01 CA128931
Grant ID: R01 CA128931-02