Abstract
Free full text

Intervening on risk factors for coronary heart disease: an application of the parametric g-formula
Abstract
Estimating the population risk of disease under hypothetical interventions—such as the population risk of coronary heart disease (CHD) were everyone to quit smoking and start exercising or to start exercising if diagnosed with diabetes—may not be possible using standard analytic techniques. The parametric g-formula, which appropriately adjusts for time-varying confounders affected by prior exposures, is especially well suited to estimating effects when the intervention involves multiple factors (joint interventions) or when the intervention involves decisions that depend on the value of evolving time-dependent factors (dynamic interventions). We describe the parametric g-formula, and use it to estimate the effect of various hypothetical lifestyle interventions on the risk of CHD using data from the Nurses’ Health Study. Over the period 1982–2002, the 20-year risk of CHD in this cohort was 3.50%. Under a joint intervention of no smoking, increased exercise, improved diet, moderate alcohol consumption and reduced body mass index, the estimated risk was 1.89% (95% confidence interval: 1.46–2.41). We discuss whether the assumptions required for the validity of the parametric g-formula hold in the Nurses’ Health Study data. This work represents the first large-scale application of the parametric g-formula in an epidemiologic cohort study.
Epidemiologists often want to estimate the effects of hypothetical interventions to inform policy and clinical decisions. For coronary heart disease (CHD), we seek to answer questions such as: what would be the population risk of CHD if everyone quit smoking? What if everyone started exercising 30min a day? What if everyone quit smoking and started exercising? What if only those diagnosed with diabetes made these changes? Ideally, these questions would be answered by conducting large randomized experiments. In practice, the answers often need to be inferred from observational longitudinal studies. Even in the absence of unmeasured confounding, however, standard analysis techniques cannot appropriately adjust for time-dependent confounders, which are affected by prior components of the intervention. For example, when interested in the effect of an intervention that improves daily diet, diabetes is a time-dependent confounder because1 diabetes is a risk factor for CHD,1 and receiving a diagnosis of diabetes may lead to changes in diet.2 In addition, the risk of diabetes is affected by an individual's prior diet history.3 The most common adjustment methods—either to add both the diabetes status and the lifestyle factor as time-varying covariates in a regression model or to stop updating the lifestyle factor after the diabetes diagnosis—can both lead to bias.4,5
To overcome these problems we use Robins's g-formula.5 The ‘g’ stands for ‘generalized’ because, under assumptions described below, the g-formula allows us to estimate the effect of any form of hypothetical interventions without introducing bias due to inappropriate adjustment for time-dependent confounding. In realistic settings, with high-dimensional data, we use a parametric version of the g-formula, which fits regression models to estimate the complete joint distribution of the outcome and its risk factors. This estimated joint distribution can then be used to simulate the risk of the outcome (e.g. CHD) if everybody were to receive a certain intervention (e.g. improving diet), and to compare it with the observed risk under no intervention. The g-formula can be used to estimate the effects of interventions that involve multiple risk factors (joint interventions) and the effects of interventions that involve decisions which depend on the values of risk factors that may change over time (dynamic interventions). Because the risk of the outcome under each intervention is simulated, comparisons can be made using a wide variety of parameters, including risk ratio, risk difference and number needed to treat.
In this article, we describe the parametric g-formula, apply it to estimate the effect of various hypothetical lifestyle interventions on CHD risk in the Nurses’ Health Study, and discuss whether the assumptions required for the validity of the g-formula hold in the Nurses’ Health Study data.
Materials and methods
Hypothetical interventions on risk factors for CHD
For each of the following interventions, we used the parametric g-formula to estimate the 20-year CHD risk in our population were the entire population to follow the prescribed intervention beginning at start of follow-up in 1982.
Avoid smoking.
Exercise at least 30
min a day.
Keep diet score (described below) in a range corresponding to the top two quintiles of the observed data.
Consume at least 5
g of alcohol per day.
Maintain body mass index (BMI) <25.
Interventions 1–3 combined.
Interventions 1–3 and 5 combined.
Interventions 1–4 combined
Interventions 1–5 combined.
More precisely, in Intervention 2, everyone exercising <30min/day is intervened on to increase their exercise to 30
min/day; all those exercising >30
min/day are not intervened on. Interventions 3–5 are implemented in an analogous fashion. Interventions 5–8 are alternate ‘low-risk’ joint interventions. We exclude alcohol from some combinations because not all subjects are able to consume alcohol. We exclude BMI from some combinations because the intervention on BMI differs from the others in specifying an attribute rather than a behaviour and thus is a more ambiguous intervention (different methods to modify BMI may have different effects on CHD risk).
We chose the interventions listed above based on previous epidemiologic analyses. As discussed elsewhere,6,7,17 all interventions listed above are imprecise and vague to a certain extent, but there is a question of degree and the BMI intervention is especially problematic. Further, our BMI intervention is unrealistic by requiring changes that are rarely observed in the data (e.g. forcing a drop from a BMI of 35–25 in a 2-year period), which leads to problems in estimation. A more realistic intervention, although still vague, might be to reduce BMI by a small percentage, for example 5% among all subjects with BMI >25kg/m2, in any given 2-year period.
Hypothetical Interventions 2–9 are dynamic interventions because different subjects can receive different levels of the exposure under study.8 In contrast, Intervention 1 is static because everyone receives the same exposure level (i.e. no smoking). Interventions 2–9 differ from most dynamic interventions considered in the literature in that we observe the value of the natural value of the exposure and then change it only if it is too low or too high.17,19
We also consider dynamic Interventions 10–18, which are equal to Interventions 1–9, respectively, but are made only following coronary artery bypass graft (CABG) or a diagnosis of high cholesterol, hypertension, diabetes, angina or stroke. We expect that the population effects of Interventions 10–18 are smaller than the effects of Interventions 1–9 as we are intervening on fewer people, but that the targeted interventions may be more effective per intervened-on person.
The Nurses’ Health Study data
We used data from the Nurses’ Health Study, which began in 1976 when 121701 registered US nurses aged 30–55 years responded to a mailed questionnaire. Every 2 years, the nurses received a follow-up questionnaire to update information on potential risk factors and to identify newly diagnosed diseases; validations of many of the assessments have been published elsewhere.9–14 We followed Stampfer and colleagues15 to define a CHD endpoint as confirmed first myocardial infarction (fatal or non-fatal) and to calculate a composite diet score with a higher score indicating a diet low in trans fat and glycaemic load, high in cereal fibre, marine n-3 fatty acids and folate and with a high ratio of polyunsaturated to saturated fat.
We excluded all women with a CHD endpoint before the date of return of their 1982 questionnaire and those with missing values for risk factors on 1978, 1980 or 1982 questionnaires, and incomplete, or implausible diet reported in 1980. Each woman was followed from the time of return of the 1982 questionnaire until a CHD endpoint, death, time of expected return of first skipped questionnaire or June 2002, whichever came first. After exclusions, our cohort included 78746 women. Of these, 2319 had a CHD event, 16
818 were censored when they missed a questionnaire and 5616 died from causes other than CHD during the follow-up. To account for censoring, we calculated the 20-year risk of CHD in this population as 3.50%, using a cumulative-incidence estimator.16
The parametric g-formula
Under the assumptions given below, the g-formula5 can consistently estimate the CHD risk under a hypothetical intervention assuming that all joint predictors of the outcome and of the exposures involved in the intervention are measured at all time points. The CHD risk is estimated by a weighted sum or integral, over all risk factor histories, of the probability of CHD conditional on its risk factors. As a weighted sum over risk-factor histories, the g-formula is the appropriate generalization of standardization for time-varying exposures.
In situations with few possible risk-factor histories, the g-formula can be calculated directly without resorting to statistical models (i.e. non-parametrically); the more typical situation with high-dimensional data requires (i) a combination of parametric models to estimate the components of the integral, and (ii) Monte Carlo simulation to approximately compute the integral under each intervention of interest. The benefits of the parametric g-formula compared with standard regression models are summarized in the first column of Table 1; costs and caveats are in the second column. Like standard regression models, the parametric g-formula requires the assumptions of no unmeasured or residual confounding, no measurement error and no model misspecification. Unlike standard methods, the parametric g-formula can deliver consistent estimates of risk even when time-dependent confounders are affected by prior components of the intervention; although, in this setting, the parametric g-formula is subject to the ‘g-null paradox’ theorem, which implies it can be essentially impossible to correctly specify the needed parametric models under the causal null hypothesis. As a consequence, the method will reject the causal null, even when true, in sufficiently large samples.5
Table 1
Advantages and disadvantages of the g-formula compared with conventional methods
Advantages | Disadvantages |
---|---|
Appropriately adjusts for time-varying confounding affected by prior exposures although subject to the g-null paradox. | Requires models for covariates as well as outcome. |
Naturally handles interventions on multiple risk factors (joint interventions) and interventions dependent on evolving risk factor values (dynamic interventions). | May be more sensitive to violations of assumptions of no unmeasured confounding, no measurement error and no model misspecification. |
Estimates multiple parameters (including risk ratios and risk differences) and yields population estimates. | Not implemented in packaged statistical software. |
The algorithm for our application of the parametric g-formula is outlined below (see Appendix 1 for details):
For each 2-year period between 1982 and 2002, model on the whole sample the following as a function of prior risk factor history.
Each risk factor.
Risk of non-CHD death.
CHD risk.
Simulate a cohort followed between 1982 and 2002 under the intervention of interest. First, select a random sample (n
=
10
000) from the study population. Then repeat the following steps for each individual and for each 2-year period t from 1982 to 2000 (see Figure 1 for schematic).
Predict time t covariates by applying the coefficients estimated in Step 1a to data from times t−1 and t−2.
Change time t covariate data as indicated by the intervention of interest.
Predict the probability of non-CHD death between t and t
+
1 by applying the coefficients estimated in Step 1b to data from times t and t−1.
Predict the probability of CHD between t and t
+
1 by applying the coefficients estimated in Step 1c to data from times t and t–1.
Estimate the population CHD risk under the intervention as the average of the subject-specific risks.
Repeat Steps 2 and 3 for each intervention of interest.
Repeat Steps 1–4 on 200 bootstrap samples to obtain 95% confidence intervals (CIs) for the estimated CHD risks and measures of comparison between two interventions.
An SAS macro that we have developed to implement the parametric g-formula is available on our website (http://www.hsph.harvard.edu/causal).
Modelling the joint distribution
To implement Step 1 of the above algorithm, we used separate regressions to model CHD, death and each of the following time-varying risk factors: cigarette smoking, diet, alcohol consumption, physical activity, BMI, hypertension, high cholesterol, diabetes, angina, stroke, CABG, cancer, osteoporosis, menopausal status, post-menopausal hormone use, multivitamin use, regular aspirin use and statin use. We used pooled logistic regression to model the probability of CHD and the probability of death in each 2-year period. Each time-varying risk factor was classed as binary, history-binary, full continuous or zero continuous, and then modelled in each period as follows. Binary-dependent variables, like aspirin use, and history-binary-dependent variables, like diagnosis of diabetes, were modelled using logistic regression. The models for history-binary variables (indicators that move only from zero to one, like history of diabetes) were limited to those with no history at the beginning of the 2-year period. Full continuous-dependent variables, like BMI, were estimated using linear or log-linear regression. Zero continuous-dependent variables, like cigarettes per day, had large numbers of zero values and were modelled in two stages—first as a logistic regression of zero vs non-zero values and then as a log-linear regression of the non-zero values. All models were pooled over all 2-year periods but, if a given risk factor was not assessed in a particular period, the data from that period were not included in the model of that risk factor (for example, the 1988 data were not used in the pooled model for diet score).
All models included, as predictors, the two previous values of all time-varying risk factors, age, 2-year period and the non-time-varying covariates of parental history of myocardial infarction before age 60 years, oral contraceptive use before 1980, BMI at the age of 18 years, smoking history before 1980, baseline smoking, baseline physical activity, baseline diet score, baseline alcohol consumption and baseline BMI. When a time-varying covariate was not assessed in all periods, only the most recent measurement was used, and an interaction between the most recent measurement and the time since that measurement was added to the model. Binary predictors were entered into the models as indicators; continuous predictors were categorized in the primary analyses, and entered as polynomials (linear, quadratic and cubic) and restricted cubic splines in sensitivity analyses. Table 2 summarizes the information on the covariates included in the primary analysis.
Table 2
Summary of covariates
Variablea | Years assessed | Type of model when used as dependent variable | Functional form when used as predictor |
---|---|---|---|
Non-modifiable | |||
![]() ![]() ![]() ![]() | – | Not predicted | 5-year categories |
![]() ![]() ![]() ![]() | – | Not predicted | Period indicators |
![]() ![]() ![]() ![]() | – | Not predicted | Indicator |
![]() ![]() ![]() ![]() | – | Not predicted | Indicator |
![]() ![]() ![]() ![]() | – | Not predicted | Indicator |
![]() ![]() ![]() ![]() | – | Not predicted | Six categoriesb |
![]() ![]() ![]() ![]() | 1982 | Not predicted | Five categoriesb |
![]() ![]() ![]() ![]() | 1982 | Not predicted | Six categoriesb |
![]() ![]() ![]() ![]() | 1980 | Not predicted | Quintile indicators |
![]() ![]() ![]() ![]() | 1980 | Not predicted | Four categoriesb |
![]() ![]() ![]() ![]() | 1982 | Not predicted | Six categoriesb |
Directly modifiable | |||
![]() ![]() ![]() ![]() | Starting in 80 | Logistic | Indicator |
![]() ![]() ![]() ![]() | 80, 82, 84, 88-on | Logistic | Indicator |
![]() ![]() ![]() ![]() | 88, 94-on | Logistic | Indicator |
![]() ![]() ![]() ![]() | All | Logistic | Indicator |
![]() ![]() ![]() ![]() | All | Logistic, log-linear | Five categoriesb |
![]() ![]() ![]() ![]() | 80, 82, 86, 88, 92-on | Linear | Six categoriesb |
![]() ![]() ![]() ![]() | 80, 84, 86, 90, 94, 98 | Linear | Quintile indicators |
![]() ![]() ![]() ![]() | 80, 84, 86, 90, 94, 98 | Logistic, log-linear | Four categoriesb |
Indirectly modifiable | |||
![]() ![]() ![]() ![]() | All | Linear (on log scale) | Six categoriesb |
![]() ![]() ![]() ![]() | All | Logistic to failure | Indicator |
![]() ![]() ![]() ![]() | All | Logistic to failure | Indicator |
![]() ![]() ![]() ![]() | All | Logistic to failure | Indicator |
![]() ![]() ![]() ![]() | All | Logistic to failure | Indicator |
![]() ![]() ![]() ![]() | All | Logistic to failure | Indicator |
![]() ![]() ![]() ![]() | Starting in 86 | Logistic to failure | Indicator |
![]() ![]() ![]() ![]() | All | Logistic to failure | Indicator |
![]() ![]() ![]() ![]() | All | Logistic to failure | Indicator |
![]() ![]() ![]() ![]() | Starting in 82 | Logistic to failure | Indicator |
aAll covariate values are self reported by participants unless otherwise specified.
bBMI, and BMI at the age of 18 years, categories are <18.0, 18.0–21.9, 22.0–24.9, 25.0–29.9, 30.0–34.9 and ≥35.0. Physical activity categories, in min/week, are <30, 30–89, 90–149, 150–209, 210–269, 270–329 and ≥330. Smoking categories, in cigarettes per day, are 0, 1–4, 5–14, 15–29 and ≥30. Alcohol categories, in g/day, are 0, 1–4, 5–9 and ≥10.
To model the joint distribution of the time-varying covariates reported in the same questionnaire, we chose an arbitrary ordering [such as (i) physical activity, (ii) cigarette smoking and (iii) alcohol consumption, etc.]. We first modelled the first covariate in the order (physical activity), then modelled each subsequent covariate as a function of the concurrent, previously modelled covariates (cigarette smoking as a function of concurrent physical activity, alcohol consumption as a function of both concurrent physical activity and concurrent smoking, etc). Under the strong assumptions that no risk factor causes any other concurrent risk factor, that there is no unmeasured confounding for the effect on CHD and survival of the combined intervention9 on Risk Factors 1–5, and that any common causes of risk factors at one time point are independent of any common causes of the risk factors at all other time points, the g-formula has a causal interpretation regardless of the intervention under consideration or the choice of covariate order.17 We explored the influence of covariate order in a sensitivity analyses. Cross-validation was used to improve the prediction models by checking the predicted risk under no intervention against the observed risk as a test of model fit. We conducted multiple secondary analyses to assess the sensitivity of our estimates to our assumptions regarding eligibility criteria, censoring and modelling.
Simulations
To implement Step 2 of the above algorithm, we used Monte Carlo simulation on random samples of 10000. Using the total sample size (78
746) instead had practically no effect on the variance estimates, indicating that the Monte Carlo contribution to the variance was small. The risk factors predicted by a logistic model were assigned a value of one if the predicted probability was greater than a random number from a uniform distribution; risk factors predicted by a linear model were assigned a value equal to the predicted value plus the standard error multiplied by a random number from a Normal (0, 1) distribution. Thus, two subjects with the same risk factor history were not necessarily predicted to have exactly the same risk factors at the next time point. Additionally, we truncated the simulated values of continuous risk factors so that they would not fall outside of the observed range. It was still possible for us, under this process, to simulate risk factor histories in which every risk-factor value was observed in the data, but the combination of risk-factor values was not.
Results
CHD risk under interventions
We used the parametric g-formula to simulate the 20-year risk of CHD under no intervention, in the presence of death from other causes and in the absence of censoring due to skipped questionnaires. The simulated risk was 3.68% (95% CI: 3.56–4.09).
We then compared the estimated 20-year risk under no intervention with the estimated risks under the hypothetical interventions (Tables 3and and4).4). We present 20-year risk, risk ratios and risk differences, all with 95% confidence intervals, along with the number needed to treat and the average percent of the entire population requiring an intervention in each follow-up period. For all results the bootstrap estimate of bias, calculated as the percent difference between the point estimate of risk and the mean of the bootstrap estimates, was <5%. The average percent intervened on in each 2-year time period is a measure of how much behaviour change is required by the intervention and facilitates comparisons across interventions. For example, exercising at least 30min/day and quitting smoking appear to have similar effects on population risk of CHD: ~20% reduction in risk. The first, however, required that an average of 53% of the population change behaviour in a given time period compared with only 5% for the second. This reflects the prevalence of adherence to the intervention in the population, the strength of the risk factor and the correlation between time periods. Starting smoking, or even restarting smoking after quitting, was relatively rare, so that 27% of the population changed behaviour for the smoking intervention at the first time point and then far fewer at subsequent times. For the other behaviours, there was less correlation between time periods so that the numbers required to change behaviour at least once during follow-up were much higher than the number required to change at the average 2-year time period.
Table 3
Simulated population risk estimates using the g-formula. Hypothetical interventions on entire cohort
Intervention | 20-year risk | Population risk ratio | Population risk difference | Number needed to Intervene | Average percent intervened upona | Cumulative percent intervened upona |
---|---|---|---|---|---|---|
(0) No intervention | 3.68 (3.56 to 4.09) | 1 | 0 | – | 0 | 0 |
(1) Quit smoking | 3.01 (2.86 to 3.38) | 0.82 (0.78 to 0.85) | −0.67 (−0.88 to −0.56) | 150 | 5 | 30 |
(2) Exercise at least 30![]() | 2.90 (2.47 to 3.60) | 0.79 (0.64 to 0.92) | −0.77 (−1.41 to −0.32) | 129 | 53 | 99 |
(3) Keep diet score in the top 2 quintiles | 3.27 (3.08 to 3.68) | 0.89 (0.82 to 0.95) | −0.41 (−0.70 to −0.19) | 247 | 55 | 99 |
(4) Consume at least 5g alcohol per day | 3.19 (2.84 to 3.72) | 0.87 (0.75 to 0.98) | −0.48 (−0.97 to −0.08) | 206 | 56 | 89 |
(5) Maintain BMI <25 | 3.62 (3.45 to 4.11) | 0.98 (0.93 to 1.04) | −0.06 (−0.28 to 0.14) | 1632 | 51 | 73 |
(6) ‘Low-risk’ lifestyle (1–3 combined) | 2.22 (1.85 to 2.74) | 0.60 (0.48 to 0.70) | −1.45 (−2.02 to −1.13) | 69 | 74 | 100 |
(7) ‘Low-risk’ lifestyle (1–3 and 5 combined) | 2.17 (1.78 to 2.69) | 0.59 (0.47 to 0.70) | −1.51 (−2.06 to −1.13) | 66 | 87 | 100 |
(8) ‘Low-risk’ lifestyle (1–3 and 4 combined) | 1.88 (1.51 to 2.38) | 0.51 (0.40 to 0.63) | −1.80 (−2.29 to −1.40) | 56 | 86 | 100 |
(9) ‘Low-risk’ lifestyle (1–5 combined) | 1.89 (1.46 to 2.41) | 0.51 (0.39 to 0.64) | −1.79 (−2.34 to −1.41) | 56 | 92 | 100 |
aThe average percent intervened on is the average, across all 2-year time periods, of the percent of the population required to change behaviour in a given 2-year time period. The cumulative percent intervened on is the percent of the population required to change behaviour in at least one 2-year time period.
Table 4
Simulated population risk estimates using the g-formula. Hypothetical interventions only following high cholesterol, hypertension, diabetes, angina, stroke or CABG
Intervention | 20-year risk | Population risk ratio | Population risk difference | Number needed to assessa | Average percent intervened uponb | Cumulative percent intervened uponb |
---|---|---|---|---|---|---|
(0) No intervention | 3.68 (3.56 to 4.09) | 1 | 0 | – | 0 | 0 |
(10) Quit smoking | 3.17 (3.04 to 3.56) | 0.86 (0.83 to 0.89) | −0.50 (−0.68 to −0.40) | 199 | 3 | 17 |
(11) Exercise at least 30![]() | 3.11 (2.68 to 3.65) | 0.84 (0.71 to 0.94) | −0.57 (−1.13 to −0.24) | 175 | 29 | 72 |
(12) Keep diet score in the top 2 quintiles | 3.37 (3.22 to 3.73) | 0.92 (0.85 to 0.96) | −0.31 (−0.57 to −0.16) | 323 | 29 | 69 |
(13) Consume at least 5g alcohol per day | 3.29 (2.98 to 3.81) | 0.89 (0.79 to 0.98) | −0.39 (−0.82 to −0.07) | 259 | 33 | 67 |
(14) Maintain BMI <25 | 3.72 (3.47 to 4.13) | 1.01 (0.94 to 1.04) | 0.04 (−0.24 to 0.15) | N/A | 32 | 58 |
(15) ‘Low-risk’ lifestyle (10–12 combined) | 2.55 (2.19 to 2.96) | 0.69 (0.58 to 0.77) | −1.12 (−1.65 to −0.88) | 89 | 40 | 76 |
(16) ‘Low-risk’ lifestyle (10–12, 14 combined) | 2.47 (2.15 to 2.97) | 0.67 (0.56 to 0.77) | −1.20 (−1.66 to −0.87) | 83 | 48 | 77 |
(17) ‘Low-risk’ lifestyle (10–13 combined) | 2.25 (1.88 to 2.69) | 0.61 (0.50 to 0.70) | −1.43 (−1.90 to −1.11) | 70 | 47 | 77 |
(18) ‘Low-risk’ lifestyle (10–14 combined) | 2.25 (1.88 to 2.70) | 0.61 (0.50 to 0.71) | −1.43 (−1.91 to −1.12) | 70 | 51 | 78 |
aOnly those meeting the criteria of having a history of high cholesterol, hypertension, diabetes, angina, stroke or CABG, are intervened upon. We are, however, looking at the 20-year risk in the full population. Thus, this version of the ‘number needed to treat’ represents the number that need to be assessed, and intervened upon if the criteria are met, in order to prevent one event. Because the estimated risk for the BMI intervention was greater than the estimated risk under no intervention, the concept of number needed to assess is not applicable.
bThe average percent intervened on is the average, across all 2-year time periods, of the percent of the population required to change behaviour in a given 2-year time period. The cumulative percent intervened on is the percent of the population required to change behaviour in at least one 2-year time period.
Interventions 10–18 are done only following an intermediate event or diagnosis, so only affect part of the population, yet we are estimating their effect on the 20-year risk in the entire population. Thus, Interventions 10–18 have a smaller overall effect on the population-wide risk than the corresponding Interventions 1–9, but may have a larger effect per person. For exercising 30min/day, Intervention 2 (on the entire population) reduced risk by 21% compared with a reduction of 16% for Intervention 11 (only on those at highest risk), but the first involved 53% of the people changing their behaviour and the second involved only 29% doing so. In other words, Intervention 10 provided 76% of the benefit of Intervention 1, but required <55% of the effort (calculated as 16/21
=
61% and 29/53
=
55). Thus, we can consider not only the overall effect of interventions, but also the feasibility of such interventions. If coupled with data on the per-person per-year cost of making each change, they could also allow approximate cost-effectiveness analysis.
In addition to the lifestyle interventions in the tables, we also evaluated pharmacologic interventions in which everyone would take multivitamins, aspirin or statins. Rather than the expected protective effect, we instead found null or close to null effects for these interventions. These findings are likely explained by our inability to adjust for confounding by indication, given the coarse measurement of the indicating conditions in the variables used in this analysis (such as self-reported binary high cholesterol rather than measured low- and high-density lipoprotein levels).
Sensitivity analyses
Restricting the study population and entire analysis to only ‘healthy’ women, defined as those without diabetes, angina, stroke, CABG or cancer at baseline, reduced the overall estimated incidence of CHD, but had little effect on the estimates of relative risk. Alternate model specifications using linear, quadratic or cubic polynomials overestimated the observed risk (estimating the risk under no intervention as 3.89, 4.48 and 3.84, respectively) and had mixed effects on the estimates of relative risk. Using restricted cubic splines with three, four and five knots for each of the continuous covariates strengthened the estimated effect for the smoking intervention, but otherwise did not consistently change the estimates. Using one or three lagged measurements (instead of two) and adding two-way interactions with high blood pressure, high cholesterol and diabetes had little effect on any estimates. Measures of ethnicity and education were not included in the primary analysis because they were measured after baseline and thus are missing for some women. Adding ethnicity, education and employment status at baseline also had little effect. In addition to the arbitrary order used in our base specification, we replicated the analysis under 10 other random orderings of covariates. We found that the estimation of the risk under no intervention ranged from 3.64 to 3.75%; the estimated risk ratios and risk differences were not substantially altered.
For the interventions specifying a threshold (e.g. exercising at least 30min/day) our primary analysis assigned those not meeting the criteria just to the threshold (e.g. to exactly 30
min/day). In a secondary analysis, we explored the sensitivity to this by assigning them well past the threshold (e.g. to 60
min/day). We also conducted similar secondary analyses for diet (to top quintile), for alcohol (to 15
g/day), for BMI (to 22) and for the joint interventions. There was little difference in the estimates (results not shown).
Discussion
The g-formula provides a framework to estimate the effects of hypothetical interventions, including joint and dynamic ones, from complex longitudinal data. Although the g-formula was originally published by Robins in 1986,5 its applications have been hindered by the lack of available software and of rich longitudinal epidemiologic data. We present an application of the g-formula to the Nurses’ Health Study in which we estimated the CHD risk reduction under various hypothetical lifestyle interventions, including the most effective interventions—joint interventions on multiple risk factors and dynamic interventions that vary over time to maximize the health benefits.
Estimates of the effect of lifestyle and BMI on the risk of CHD in the Nurses’ Health Study have been previously obtained using conventional statistical methods.15 Those estimates, however, require additional assumptions to be interpreted as the effect of hypothetical interventions, and thus cannot be directly compared with the estimates presented in this article. Yet, those estimates from standard methods and our g-formula estimates are qualitatively similar. The most noticeable difference is that the g-formula effect estimate for the BMI intervention is null, compared with the apparently harmful effect of high BMI estimated by the standard methods.18 The lack of clearly beneficial effect estimate for BMI in our anlaysis might be explained by the fact that, compared with the other interventions, the BMI intervention may be particularly subject to residual confounding by undiagnosed preclinical illness (often referred to as ‘reverse causation’),18 it is less well defined, especially when partly controlling for diet and physical activity,7 and the impact of measurement error may differ between the g-formula and standard methods. A methodological approach specifically designed to appropriately adjust for reverse causation may be needed to correctly estimate the effect of BMI on mortality.19
The parametric g-formula relies on the same assumptions (no unmeasured confounding, no measurement error and no model misspecification) as alternative methods such as standard methods, marginal structural models or structural nested models. It may, however, be more sensitive to violations of these assumptions because a violation in one of the multiple models may reverberate throughout the others. Even though we adjusted for as many potential confounders as possible, we cannot rule out bias due to unmeasured or mismeasured confounders. Likewise, despite cross-validation and sensitivity analyses, we cannot rule out bias due to model misspecification. If the assumptions are met, however, the g-formula, unlike standard methods, correctly adjusts for time-varying confounders affected by prior exposure.
A necessary, although insufficient, condition for the absence of gross model misspecification is being able to predict accurately CHD risk under no intervention. The observed 20-year risk of CHD in this population was estimated as 3.50%. Using the g-formula to simulate data completely analogous to the observed data (by not adjusting for either censoring due to skipped questionnaires or death from other causes), we estimated a risk of 3.64%, which is consistent with a reasonable prediction model. The estimated risk of 3.68% under no intervention reported in Tables 3and 4 differs from 3.64% because in our primary analysis we simulated a cohort without censoring due to skipped questionnaires.
Our sensitivity analysis shows that, although our estimates of relative risk are reasonably robust, our estimates of the absolute risks are quite sensitive to small changes in the models. Different covariate orderings result in estimated risks under no intervention that differ by >10%. That our primary analysis yielded a CHD risk under no intervention so close to the observed risk may be due to the cross validation process we used to fine-tune our primary models. When using the g-formula, it may be important to fine-tune the model in order to closely predict the risk under no intervention; in our analysis, this fine-tuning did not appear to bias estimated intervention-specific risk differences.
To avoid the ‘g-null paradox’, we only considered interventions for which we did not a priori believe that the null is true. To check against this limitation, as well as the others, we could replicate our findings using methods such as inverse probability weighting of marginal structural models and g-estimation of nested structural models. Both of these methods model different aspects of the joint distribution, impose fewer parametric restrictions and are free of the g-null paradox. This is beyond the scope of the current work, but will be an important next step.
Although it has limitations, we believe the parametric g-formula is a powerful and useful tool for epidemiologic analysis. By applying this method to the Nurses’ Health Study, we have aimed to show that it is feasible as an analytic approach for longitudinal cohort data, and by making software available, we intended to facilitate future use.
Funding
National Institutes of Health (R01 HL080644).
Acknowledgements
The authors thank Roger Logan and Jessica Young for their technical assistance.
Conflict of interest: None declared.
Appendix 1
In this appendix, we describe how we estimate the proportion of the Nurses’ Health Study population that would have developed CHD between 1982 and 2002 for the interventions of interest under the assumption of no unmeasured confounders.
Definitions and notation
Let k denote questionnaire period with k taking on integer values from 0 to 10 representing the 1980, 1982, 1984,…, 2000 questionnaire periods. Let Zk be the vector of covariates reported by each individual in questionnaire period k. We include the following variables in the vector Z: age, questionnaire period, cigarette smoking, physical activity, diet score, alcohol consumption, BMI, hypertension, multivitamin use, regular aspirin use, use of statins, use of post-menopausal hormones, high cholesterol, diabetes, angina, stroke, CABG, cancer, menopausal status and osteoporosis. We do not index by individual because we assume that the random variable for each subject is drawn independently from a distribution common to all subjects. We use overbars to indicate the history for any time-dependent variable. For example,
=
(Z0,
Z1,
…,
Zk) represents a subject's covariate history from questionnaire 0 to k. Let Ck and Nk represent the event that the subject is censored in the period (k−1, k], due to loss-to-follow-up or death to other causes, respectively. We consider both as part of the covariate vector Z. Let V be a vector of baseline variables measured at questionnaire zero that do not vary with time. This includes parental history of myocardial infarction before age 60 years, BMI at age 18 years, smoking history prior to 1980, oral contraceptive use prior to 1980, baseline smoking, baseline physical activity, baseline diet score, baseline alcohol consumption and baseline BMI. Let Dk represent our outcome (CHD) with Dk
=
1 if CHD is diagnosed in the period (k−1, k] and Dk
=
0 otherwise.
Let f(z) and F(z) represent the density and distribution function of the observed covariate data Z. Let Zk* be the vector of covariates that would have been observed if interventions occurred through questionnaire k−1 but no intervention is made at time k. Define an intervention d to be a collection of functions d=
(d0,
…,
d10) where dk: (
,Zk*)
→
Zk maps the covariate history and the covariates that would have been observed at time k to a new set of covariates for time k. Associated with each intervention d is the distribution Fd(z) with density fd(z), which represents the distribution of the data had, contrary to fact, all subjects in the population followed intervention d. In our analysis, this is a deterministic function, but the notation is intended to allow interventions that are not deterministic. Then, under the same framework, we could consider interventions such as ‘A randomly selected 10% of smokers quit smoking’ or ‘Exercise levels are drawn from a pre-specified distribution.’
The parametric g-formula
The g-formula5,17 to compute the cumulative risk of CHD between 1982 and 2002 is given below as the sum of the incidences in each 2-year period.
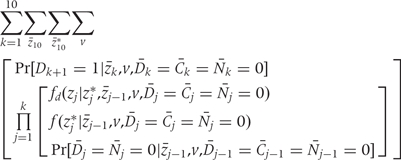
where k=
1, …,10 denotes 2-year time period starting in 1982; zk is the vector of intervention values of the risk factors Zk at time k;
is the vector of values that would be observed without time k intervention; v is the vector of time-independent baseline covariates; Dk+1
=
1 is the event that CHD is diagnosed between exams k and k
+
1;
=
0 is the event that a subject remains free of CHD through exam k;
=
0 is the event that a subject remains uncensored through exam k; and Nk
=
0 is the event that a subject has not died from other causes through exam k.
The sum is over all possible z10, z10* histories. Because each is a high-dimensional vector of covariates, a direct calculation based on (A.1) is computationally infeasible. Rather, we approximate the result of the g-formula under a given intervention by Monte Carlo simulation. To see how to conduct the simulation, first note that the g-formula (A.1) gives the probability of developing CHD between 1982 and 2002 based on an intervention-specific joint distribution of CHD and risk factors. Under the assumption of no unmeasured confounders, this is the joint distribution had all subjects followed the intervention. Therefore we generate, for each intervention, a simulated population in which the joint distribution of CHD and risk factors is approximately equal to the joint distribution implied by the g-formula. Then the CHD risk in the simulated population (i.e. the expected fraction of subjects in the simulated population who develop CHD between 1982 and 2002) estimates the desired probability.
Both and
, are estimated from the observed data using maximum likelihood. To estimate
, we chose an arbitrary ordering of risk factors at exam k: such as physical activity, cigarette smoking, alcohol consumption, history of diabetes, etc. We then estimate: (i) the conditional probability of physical activity at k given the past variables through k−1, (ii) the conditional probability of smoking given physical activity at k and past variables through k−1, (iii) the conditional probability of alcohol at k given physical activity, smoking at k and past variables through k−1, and so on. We estimate
as the product of these estimated conditional densities. We assume that no risk factor measured at k causes any other risk factor measured at k, that there is no unmeasured confounding for the set of risk factors Z and the outcome D, and that any unmeasured common causes of Zr and any unmeasured common causes of Zs are marginally independent for r
≠
s. Under these assumptions, we have a causal interpretation for all regimes d regardless of the ordering of the variables in Z.
Because the density of f(z) is invariant to the ordering of the risk factors in Z, we can alternately write the g-formula as follows. This formulation separates Z into (A, L) where A represents the set of risk factors undergoing intervention and L represents all other time-varying risk factors.
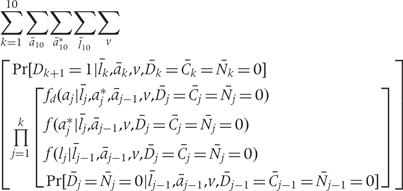
where k=
1, …,10 denotes 2-year time period starting in 1982; ak is the vector of intervention values of the risk factors Ak at time k; ak* is the vector of values that would be observed without time k intervention; lk is the vector of the risk factors that are not intervened on Lk at time k; v is the vector of time-independent baseline covariates; Dk+1
=
1 is the event that CHD is diagnosed between exams k and k
+
1; Ck
=
0 is the event that a subject remains uncensored through exam k; and Nk
=
0 is the event that a subject has not died from other causes through exam k.
This formulation is consistent with previously published versions of the g-formula17 and may be easier to conceptualize for some readers. Note that this formulation implies an ordering of the risk factors in Z, with risk factors to be intervened upon appearing after all other risk factors. For convenience in our estimation, we do not impose this implied ordering of risk factors and thus are able to consider multiple interventions on different risk factors using the same base models. As explained earlier, under the assumption that no risk factor at time k causes another at that same time, the interpretation of the g-formula is invariant to the ordering of risk factors.
Steps of the parametric g-formula
Step 1a: Estimating 
We fit pooled regression models to predict each risk factor given past risk factor history, among those with no prior diagnosis of CHD. Risk factors at k are the dependent variables in models that include other risk factors at exam k (according to the arbitrary ordering explained above) plus all risk factors measured in questionnaires k−1 and k−2 as covariates. As described in the main paper, we use a model structure (e.g. linear, logistic, etc.) appropriate for the distribution of the risk factor dependent variable. The parameters of these models define the estimated conditional distributions of each risk factor. The model is restricted to those with no diagnosis of CHD at or before exam k and who have been neither lost-to-follow-up nor died from other causes.
Step 1b: Censoring variables, Ck and Nk
In our primary analysis, we fit a pooled logistic regression to predict Nk+
1, the probability that a subject dies of a non-CHD cause in the subsequent 2-year period, as a function of risk factor history. The model is restricted to those with no diagnosis of CHD at or before exam k and who have been neither lost-to-follow-up nor died from other causes. In the primary analysis, we do not simulate censoring due to skipped questionnaires, which is to say that we intervene to force Ck
=
0 for all subjects at all time points. Thus we do not need a model for the risk of Ck. If, as in secondary analysis, we do not wish to intervene on Ck, we then also fit a model for Ck+1 with the same characteristics as the model for Nk+1.
Step 1c:
We fit a pooled (over persons and time) logistic regression model to predict the risk of CHD as a function of risk factor history. The outcome is CHD diagnosis between questionnaires k and k+
1, and the covariates in the model are risk factors measures in exams k and k−1. The model is restricted to those with no diagnosis of CHD at or before exam k and who have been neither lost-to-follow-up nor died from other causes. The parameters of this model define the estimated conditional probability of CHD risk given the entire past, and thus implicitly assume that time-dependent risk factors measured more than two time periods previously do not predict CHD risk given time-dependent risk factors in the past two periods.
Step 2: Monte Carlo simulation
The following is repeated at each time point k from 1982 to 2000 on a random sample (n=
10
000) from the study population. Follow-up starts at k
=
1 (1982) in our analyses because two prior questionnaires (k
=
0 and k
=
1) are used to predict CHD risk between k and k
+
1. Thus, the CHD risk we estimate refers to the 20-year period between 1982 and 2002. The risk factor values from questionnaire zero of these simulated individuals are those actually observed (interventions beginning at k
=
1 could not affect these distributions so we are able to use the empirical distribution of the data).
Step 2a: Simulation of covariates, zk*
Using the conditional distribution estimates in Step 1a above, the risk factor values at subsequent questionnaires are generated non-deterministically. For linear models, a predicted value of the conditional mean of the covariate is calculated from regression parameters and the risk factor history, and a randomly drawn error term is added to the prediction. For logistic models, a predicted probability is calculated from the regression parameters and the risk factor history, and the prediction is compared with a randomly drawn uniform value. The covariate is assigned value one if the predicted probability is less than the random term and zero otherwise.
Step 2b: Intervention on covariates, zk
To simulate a counterfactual population subject to a given intervention d under the assumption of no unmeasured confounders, we take zk for k>0 from the intervention density.

Note that, if we simulate no intervention
[i.e. with =
1 if zk* = zk for all k],
the expected CHD risk in the simulated population should equal that of the actual study population without censoring, because the joint distribution implied by the g-formula for the simulated population is precisely that of the study population.
Step 2c: Censoring
In the primary analysis Ck is set to zero for all subjects at all time points (i.e. there is no censoring due to skipped questionnaires). Nk is simulated by estimating the predicted probability of non-CHD death at each time point, based on the simulated and intervened on risk-factor values and the conditional distribution estimates in Step 1b above. In a secondary analysis, Nk is simulated in this way, and Ck is simulated by estimating the predicted probability of censoring due to skipped questionnaire at each time point, and using that plus a randomly drawn uniform value. If the predicted probability is less than the random term, the subject is assigned to be censored.
Step 2d: Simulations of CHD risk,
For each simulated individual the predicted probability of CHD at each time point is estimated, based on her simulated and intervened on risk factor values, using the conditional distribution estimated in Step 1c above.
Step 3: Calculating the cumulative risk
For each intervention d, the 20-year CHD risk is calculated using a method, similar to Kaplan–Meier, which adjusts for competing risks:
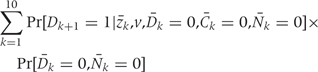
By averaging this estimated risk over the simulated population we are summing over all risk factor histories, weighting by the frequency of risk factor history. The average risk in the simulated populations gives the g-formula estimate.
Step 4: Intervention repetitions
Steps 2 and 3 are repeated to give cumulative incidence estimates for each intervention. The estimated cumulative risks under different interventions d and d′ are compared to give a risk ratio and risk difference.
Step 5: Bootstrap repetitions
We use non-parametric bootstrap methods (sampling the observed study population with replacement 200 times) to estimate approximate confidence intervals of the counterfactual CHD risks and risk ratios. The size of each bootstrap sample is that of the original sample. To obtain CIs for the risks, risk ratios and risk differences, we re-apply Steps 1–4 to each of the bootstrap samples. The bounds for the confidence intervals are set at the 2.5th and 97.5th percentiles of the distribution of the bootstrap estimates.
References
Articles from International Journal of Epidemiology are provided here courtesy of Oxford University Press
Full text links
Read article at publisher's site: https://doi.org/10.1093/ije/dyp192
Read article for free, from open access legal sources, via Unpaywall:
https://academic.oup.com/ije/article-pdf/38/6/1599/18480155/dyp192.pdf
Free after 24 months at ije.oxfordjournals.org
http://ije.oxfordjournals.org/cgi/reprint/38/6/1599.pdf
Free after 24 months at ije.oxfordjournals.org
http://ije.oxfordjournals.org/cgi/content/full/38/6/1599
Free to read at ije.oxfordjournals.org
http://ije.oxfordjournals.org/cgi/content/abstract/38/6/1599
Citations & impact
Impact metrics
Article citations
Impact of New York City's 4-year multi-component natural experiment to improve elementary school physical education on student cardiorespiratory fitness.
BMC Public Health, 24(1):3161, 14 Nov 2024
Cited by: 0 articles | PMID: 39543591 | PMCID: PMC11562091
Health disparities in cirrhosis care and liver transplantation.
Nat Rev Gastroenterol Hepatol, 31 Oct 2024
Cited by: 0 articles | PMID: 39482363
Review
Contribution of Involuntary Job Loss to the Burden of Depressive Symptoms Over Two Decades in a National Study of Aging Adults.
J Gerontol B Psychol Sci Soc Sci, 79(10):gbae135, 01 Oct 2024
Cited by: 0 articles | PMID: 39110128
Racial and ethnic differences in the risk of dementia diagnosis under hypothetical blood pressure-lowering interventions: The Multi-Ethnic Study of Atherosclerosis.
Alzheimers Dement, 20(8):5271-5280, 10 Jul 2024
Cited by: 1 article | PMID: 38984649 | PMCID: PMC11350054
Counterfactual Sepsis Outcome Prediction Under Dynamic and Time-Varying Treatment Regimes.
AMIA Jt Summits Transl Sci Proc, 2024:285-294, 31 May 2024
Cited by: 0 articles | PMID: 38827103 | PMCID: PMC11141800
Go to all (174) article citations
Similar Articles
To arrive at the top five similar articles we use a word-weighted algorithm to compare words from the Title and Abstract of each citation.
Changes in fish consumption in midlife and the risk of coronary heart disease in men and women.
Am J Epidemiol, 178(3):382-391, 27 Jun 2013
Cited by: 42 articles | PMID: 23813701 | PMCID: PMC3727335
Hypothetical midlife interventions in women and risk of type 2 diabetes.
Epidemiology, 24(1):122-128, 01 Jan 2013
Cited by: 46 articles | PMID: 23222556 | PMCID: PMC3526834
Hypothetical interventions to prevent stroke: an application of the parametric g-formula to a healthy middle-aged population.
Eur J Epidemiol, 33(6):557-566, 02 Jan 2018
Cited by: 13 articles | PMID: 29294206
Risk factors for coronary heart disease in women.
Cardiol Clin, 16(1):1-8, 01 Feb 1998
Cited by: 19 articles | PMID: 9507775
Review
Funding
Funders who supported this work.
NHLBI NIH HHS (1)
Grant ID: R01 HL080644
NIAID NIH HHS (1)
Grant ID: R37 AI032475