Abstract
Free full text

Adenovirus VA RNA-derived miRNAs target cellular genes involved in cell growth, gene expression and DNA repair
Abstract
Adenovirus virus-associated (VA) RNAs are processed to functional viral miRNAs or mivaRNAs. mivaRNAs are important for virus production, suggesting that they may target cellular or viral genes that affect the virus cell cycle. To look for cellular targets of mivaRNAs, we first identified genes downregulated in the presence of VA RNAs by microarray analysis. These genes were then screened for mivaRNA target sites using several bioinformatic tools. The combination of microarray analysis and bioinformatics allowed us to select the splicing and translation regulator TIA-1 as a putative mivaRNA target. We show that TIA-1 is downregulated at mRNA and protein levels in infected cells expressing functional mivaRNAs and in transfected cells that express mivaRNAI-138, one of the most abundant adenoviral miRNAs. Also, reporter assays show that TIA-1 is downregulated directly by mivaRNAI-138. To determine whether mivaRNAs could target other cellular genes we analyzed 50 additional putative targets. Thirty of them were downregulated in infected or transfected cells expressing mivaRNAs. Some of these genes are important for cell growth, transcription, RNA metabolism and DNA repair. We believe that a mivaRNA-mediated fine tune of the expression of some of these genes could be important in adenovirus cell cycle.
INTRODUCTION
RNA interference (RNAi) is a posttranscriptional gene silencing process that affects from Saccharomyces pombe to humans. RNAi-mediated regulation of gene expression is achieved by inducing gene deletion, DNA heterochromatinization, mRNA decay or translation inhibition (1). Several small non-coding RNAs have been described that guide the RNAi machinery in controlling the expression of specific genes. In mammals, small RNAs include small interfering RNAs (siRNAs) and microRNAs (miRNAs) (2). siRNAs, with perfect complementarity to their targets, activate RNAi-mediated cleavage of the target mRNAs, while miRNAs generally induce RNA decay and/or translation inhibition of target genes (3–6).
MicroRNAs (miRNAs) are ~22-nt long RNAs processed from long primary transcripts, called pri-miRNAs. pri-miRNAs are cleaved in the nucleus by an RNAse III called Drosha, into imperfectly pairing stem-loop precursors of ~70 nt called pre-miRNAs (1,7). The pre-miRNAs are then exported by Exportin 5 (Exp5) to the cytoplasm, where Dicer processing renders mature double-stranded miRNAs that interact with the RNA-induced silencing complex (RISC) (1,8,9). The antisense strand of the miRNA must be incorporated into RISC, to guide the complex to the 3′UTR of the target gene (9). There, recognition of only 6 nt that base pair with the seed sequence of the miRNA are enough to induce functional inhibition of the target gene (10).
miRNA-regulated genes are not easy to identify. Searching for mRNAs that contain a given 6-nt long sequence in their 3′UTR, retrieves few real targets scattered among thousands of other mRNAs. Prediction programs with good rates of identification of real miRNA targets have incorporated into their algorithms other features that may influence miRNA targeting. These benefit (i) AU-rich sequences near the target, which may be an indirect measurement for target accessibility, (ii) proximity of the target to residues pairing to miRNA nucleotides 13–16, (iii) proximity of the target to other miRNA targets which may act cooperatively and (iv) target location away from the center of long 3′UTRs and relatively close to the stop codon (11). Biochemical approaches have also been used to identified miRNA targets. As miRNAs induce RNA decay and/or translation inhibition of target genes, both proteomics and genomics have been employed (3,4,6). Comparison of the proteome between control cells and cells expressing a given miRNA, should result in identification of proteins whose expression is downregulated by the miRNA. Microarray technology allows analysis of complete genomes and can be used for identification of all mRNAs with target sequences that decrease in the presence of a given miRNA. However, this approach does not identify targets affected exclusively by translation inhibition.
It has been calculated that RNAi controls the expression of 30% of human genes, some involved in development, differentiation, apoptosis and proliferation (10,12). Moreover, a clear connection between cancer and RNAi has been shown (13). In plant and insect cells, RNAi also works as an alternative immune mechanism against viral infections (14). Several mammalian viruses are also inhibited by siRNAs or certain cellular miRNAs, suggesting that RNAi could play an antiviral role also in vertebrates (15,16). Plant viruses have evolved to escape antiviral RNAi with the development of silencing suppressors. Several animal viruses have been also described as encoding silencing suppressors, such as PFV-1 Tas, HIV Tat, influenza NS1, vaccinia E3L, Ebola VP35, HCV core and adenovirus virus-associated (VA) RNA (15,17–22). Controversy exists because viruses have also been described as using the cellular silencing machinery to control gene expression. Thus, viral miRNAs that could regulate expression of both host and viral genes have been described in several viruses (17,23–27). Surprisingly, adenovirus VA RNAs can act both as silencing suppressors by inhibiting Dicer and RISC and as precursors of viral miRNAs (17,21,23,28).
Most human adenovirus, including serotypes 2 and 5, express two VA RNAs, VAI and VAII (29). These VA RNAs are non-coding polymerase III-transcribed ~160-nt long RNAs that fold into a dsRNA with a well-conserved structure (29). VA RNAs are very abundant during viral infection. VA RNAI accumulates to 108 molecules per cell (30), and therefore, may saturate or block several cellular VA interacting factors. After nuclear transcription, VA RNAs have been described to saturate Exp5 on their way to the cytoplasm (21). There, VAI is involved in the blockade of RNA-dependent protein kinase (PKR), an interferon-inducible serine-threonine protein kinase activated in infected cells as part of the antiviral response (31). Also, as VA RNAs and pre-miRNAs have a similar structure, VA RNAs bind and block Dicer (17,21). However, 2–5% of the VA RNAs can be processed by Dicer in their terminal stem to adenoviral miRNAs named svaRNAs or mivaRNAs (17,23). Even if Dicer processing is not efficient, over 106 mivaRNAs are produced that can bind the RISC complex (17,23,28). mivaRNAs with VA RNA 3′-terminal sequences are preferentially incorporated into RISC (17,23,28). The most abundant are two mivaRNAs from VAI, where Dicer may cut at nucleotide 137 or 138 (mivaRNAI-137, mivaRNAI-138), and a single mivaRNA from VAII, where Dicer most favorably cuts at position 138 (mivaRNAII-138) (28). Analysis of RISC-bound miRNAs in adenovirus-infected cells have showed that 80% correspond to mivaRNAs, indicating saturation of RISC and decrease in regulation by cellular miRNAs in infected cells (28). However, RISC-bound mivaRNAs can inhibit the expression of genes with complementary sequences (23,28). Also, inhibition of mivaRNAI with antagomirs affects efficient adenovirus production, suggesting that mivaRNAs could regulate the expression of cellular or viral genes that may affect virus viability (23).
We believe that mivaRNAs may function as viral miRNAs, as we have not found cellular or viral genes with perfect complementary sequences to mivaRNAs. The combination of bioinformatics and genomics has allowed us to identify several putative mivaRNA targets. Thirty-one out of the 50 genes analyzed are downregulated in cells expressing mivaRNAs. Further, we have validated TIA-1 as a direct target of mivaRNAI-138.
MATERIALS AND METHODS
Cell lines and viruses
Human 293 and HeLa cells were obtained from the ATCC. All cell lines were cultured in DMEM medium, supplemented with 10% FBS, 1% penicillin–streptomycin and 2 mM l-glutamine. Cell lines were cultured at 37°C in a 5% CO2 atmosphere. All cell culture reagents were obtained from Gibco BRL/Life Technologies. Plasmids were transfected using calcium phosphate as described (32). Transfection efficiency was >90%. Transfection with 2′-O-methyl oligonucleotides (Sigma) was performed using Lipofectamine 2000 (Invitrogen) following the recommendations of the supplier as described (23). In brief, 100 pmol of oligo were added to 3.5 × 105 cells in 1 ml of Opti-MEM (Gibco). 2′-O-methyl oligonucleotides used were 2omeMut (CGACGUCAGACAACGGGGGAGCGCUCCUUUU), and 2omeAS3′ (AAAAGGAGCGCUCCCCCGUUGUCUGACGUCG).
Adenoviruses were amplified in 293 cells, purified by double cesium chloride gradient ultra-centrifugation and desalted in a G50 sephadex column (33). The Adenovirus titer was calculated with AdenoX rapid titer kit (BD Biosciences) or by limiting dilution. 293 cells were infected in DMEM 2% FBS with 1 moi (multiplicity of infection) of wild-type adenovirus type 5, or mutant ΔVA (34). Transfected cells were infected one day after transfection and analyzed when indicated.
Plasmids
pAdvantage (Promega) contains VAI and VAII genes from adenovirus type 2 with their own promoters. pSuper (35) plasmid is widely used to express shRNAs from a H1 promoter. To construct pVAI, VAI sequences were amplified with oligo pSUPVAI5′ (GCGCGAGATCTCCCGGGCACTCTTCCGTGGTCTGG) and pSUPVAI3′ (GCGCGAAGCTTTCCAAAAAGGAGCGCTCCCCCGTT). The amplified fragment was digested with BglII and HindIII, gel purified and cloned in the same sites of pSuper. pmivaRNAI-138 was constructed by cloning the hybridized oligonucleotides shVAI-15′ (GATCCCCGGGCACTCTTCCGTGGTCTTT CAAGAGAGACAACGGGGGAGCGCTCCTTTTTGGAAA) and shVAI-13′ (AGCTTTTCCAAAAAGGAGCGCTCCCC CGTTGTCTCTCTTGAAAGACCACGGAAGAGTGCCCGGG) into the BglII and HindIII sites of pSuper. pHA-TIA-1 was obtained from Juan Valcárcel and expresses TIA-1 protein fused to a HA peptide. pGL3-Promoter (Promega) expressing Firefly luciferase was used as a transfection control. pRL-SV40 (Promega) was used to construct the plasmids that express TIA-1 target sequences in the 3′UTR of renilla mRNA. Proper oligonucleotide sequences were cloned in the Xba I–Not I site of pRL-SV40 to obtain pRL-TIA-1 (CAGGGAGUGUAGUAAAGCCGUUGUUUACUUAAAG) and pRL-TIA-1Mut (CAGGGAGUGUAGUAAAGGGCAACAUUACUUAAAG). Positive clones were verified by DNA sequencing (ABI Prism 310 genetic analyzer from Perkin-Elmer).
Protein analysis
Renilla and firefly luciferase activity was quantified using the Dual Luciferase System (Promega) as previously described (36) in a Berthold Luminometer (Lumat LB 9507). Western blots were hybridized to TIA-1 (ML-29) monoclonal antibody (a kind gift from J. Valcárcel), or goat anti-TIA-1 (C-20, Santa Cruz) diluted 1 : 1000. Secondary antibodies were anti-mouse, or anti-goat coupled to peroxidase diluted 1 : 5000 (Sigma). Western blots were developed with ECL (Perkin-Elmer).
Preparation and analysis of RNA
Total RNA was purified using guanidium thiocyanate as described (37) or the RNeasy kit (Qiagen) following the recommendations of the supplier. Quantitative RT–PCRs were performed with the protocol and oligonucleotides recommended by Applied Biosystems. To detect TIA-1 we used Hs 00234977_m1 and Hs 00254561_m1, and Hs 99999905_m1 for GAPDH, used as a control. Primers used for the other genes analyzed are listed in Table 1.
Table 1.
Expression analysis in cells expressing mivaRNAs
Probe | Name | Putative targets | 12 hpi | 24 hpi | 48 hpi | 72 hpi | pVA | pVAI | pmiva RNAI-138 | Category |
---|---|---|---|---|---|---|---|---|---|---|
Hs00181599_m1 | ADCY9 | I-138; I-137; II-138 | 0.98 | 1.80 | 2.37 | 4.14 | 1.50 | 2.87 | 1.82 | Signalling |
Hs00388776_m1 | ARHGEF7 | I-138; I-137; II-138 | 1.05 | 1.39 | 2.81 | 3.31 | 1.72 | 1.77 | 1.92 | Signalling |
Hs00176747_m1 | MAP3K3 | I-138; I-137; II-138 | 1.46 | 2.31 | 3.63 | 5.51 | 2.71 | 3.91 | 1.93 | Signalling |
Hs00187940_m1 | DUSP3 | I-138(TS); I-137 | 1.60 | 2.33 | 3.53 | 6.10 | 2.02 | 4.70 | 1.65 | Signalling |
Hs00193642_m1 | PPP1R3C | I-138(TS); I-137 | 1.53 | 6.61 | 11.81 | 15.44 | 1.55 | 3.64 | 1.87 | Signalling |
Hs00743856_s1 | PTP4A1 | I-138; II-138 | 1.24 | 1.46 | 3.51 | 5.03 | 2.09 | 2.66 | 1.15 | Signalling, growth |
Hs00234259_m1 | TGFBR3 | I-138(TS); I-137; II-138 | 1.42 | 1.79 | 3.87 | 9.44 | 1.67 | 3.43 | 2.05 | Growth, signalling |
Hs00382690_m1 | LY6K | I-138; I-137 | 3.61 | 2.91 | ND | ND | 3.08 | 8.76 | 2.69 | Growth |
Hs00277039_m1 | CCND1 | I-138; II-138 | 1.20 | 1.96 | 2.18 | 3.83 | 1.86 | 1.93 | 2.30 | Growth |
Hs00188949_m1 | BNIP3L | I-138; II-138 | 1.04 | 2.08 | 2.77 | 3.15 | 1.79 | 1.95 | 1.19 | Proapoptotic |
Hs00969291_m1 | BNIP3 | I-137 | 0.98 | 1.64 | 2.99 | 3.68 | 1.71 | 3.00 | 1.82 | Proapoptotic |
Hs00901425_m1 | ETS1 | I-138(TS); I-137 | 2.19 | 2.96 | 9.55 | 5.46 | 1.52 | 2.72 | 1.95 | Transcription, growth |
Hs00176209_m1 | CDK8 | I-138; I-137 | 1.49 | 1.99 | 3.12 | 7.77 | 1.58 | 1.94 | 1.67 | Transcription, growth |
Hs00794653_m1 | RBPSUH | I-138(TS); I-137; II-138 | 1.36 | 1.79 | 3.69 | 6.51 | 2.35 | 2.69 | 2.82 | Transcription, recombination |
Hs00363134_m1 | PHF20 | I-138; I-137; II-138 | 1.10 | 1.46 | 1.82 | 2.45 | 1.63 | 2.35 | 2.68 | Transcription |
Hs00212624_m1 | TH1L | I-138(TS) | 1.03 | 1.32 | 2.46 | 6.04 | 1.58 | 2.27 | 2.56 | Transcription |
Hs00429643_m1 | DAZAP2 | I-138; I-137 | 1.11 | 1.58 | 2.60 | 5.15 | 2.68 | 7.71 | 2.06 | RNA |
Hs00389488_m1 | MDN1 | I-138; I-137; II-138 | 1.30 | 1.29 | 2.05 | 3.40 | 1.51 | 2.68 | 1.83 | RNA |
Hs00198069_m1 | CUGBP1 | I-137; II-138 | 1.45 | 2.27 | 4.14 | 5.50 | 1.85 | 3.47 | 2.14 | RNA |
Hs00287641_m1 | QKI | I-138; I-137; II-138 | 1.05 | 1.24 | 1.67 | 2.54 | 1.75 | 3.11 | 1.91 | RNA |
Hs00173159_m1 | POLS | I-138(TS); I-137 | 1.14 | 1.95 | 2.23 | 4.55 | 1.53 | 1.92 | 1.68 | DNA repair |
Hs00243513_m1 | EXO1 | I-137 | 1.35 | 1.42 | 3.40 | 6.14 | 2.32 | 5.17 | 3.17 | DNA repair |
Hs00854751_s1 | UBE2N | I-137 | 1.34 | 1.05 | 3.27 | 2.00 | 2.06 | 1.54 | 0.92 | DNA repair |
Hs00189460_m1 | PHC2 | I-138(TS); I-137; II-138 | 1.58 | 2.66 | 3.75 | 11.73 | 1.84 | 3.94 | 2.33 | DNA |
Hs00293359_m1 | DOLPP1 | I-138; II-138 | 1.33 | 1.43 | 2.17 | 2.72 | 1.66 | 2.03 | 1.51 | Modification |
Hs00390363_m1 | KIAA0528 | I-138(TS) | 1.03 | 1.51 | 5.59 | 4.12 | 1.90 | 2.28 | 1.53 | UK |
Hs00412280_m1 | KIAA0182 | I-138(TS); I-137; II-138 | 1.19 | 2.17 | 2.53 | 5.16 | 2.23 | 3.65 | 2.23 | UK |
Hs00376032_m1 | C16orf63 | I-138(TS) | 1.12 | 1.23 | 2.80 | 3.74 | 2.23 | 2.15 | 1.57 | UK |
Hs00367486_m1 | C1orf43 | I-138(TS); I-137; II-138 | 1.17 | 2.37 | 3.55 | 7.00 | 1.98 | 2.67 | 1.36 | UK |
Hs00230651_m1 | C9orf5 | I-138(TS); II-138 | 1.09 | 1.38 | 2.19 | 3.25 | 1.76 | 3.71 | 1.90 | UK |
Hs00181213_m1 | IGFBP5 | I-138; I-137; II-138 | 0.39 | 0.65 | 0.61 | 1.37 | 1.62 | 3.85 | 1.83 | |
Hs00272413_m1 | RAD51AP1 | I-138; I-137 | 0.96 | 1.24 | 2.41 | 1.57 | 2.37 | 2.53 | 1.91 | |
Hs00757386_s1 | ARL5A | II-138 | 1.06 | 1.18 | 1.76 | 1.97 | 2.47 | 1.93 | 1.52 | |
Hs00209047_m1 | CEP164 | I-138; II-138 | 1.28 | 1.65 | 1.35 | 0.44 | 1.80 | 3.25 | 2.53 | |
Hs00216375_m1 | IPO9 | I-137; II-138 | 1.27 | 1.41 | 1.10 | 1.97 | 1.61 | 3.03 | 1.80 | |
Hs00757716_m1 | CUL4A | I-138; II-138 | 1.06 | 1.92 | 1.60 | 1.74 | 1.67 | 2.08 | 1.21 | |
Hs00245657_m1 | HNRPDL | I-137 | 1.15 | 1.22 | 1.52 | 1.60 | 1.20 | 1.99 | 1.25 | |
Hs00830558_g1 | CHES1 | I-138; I-137; II-138 | 1.33 | 1.13 | 1.01 | 1.69 | 1.80 | 2.23 | 1.21 | |
Hs00392822_m1 | SETD1B | I-138; I-137; II-138 | 1.71 | 1.58 | 3.94 | 10.20 | 1.15 | 0.93 | 1.26 | Chrom remodeling |
Hs01030716_m1 | PRDM2 | I-138(TS); I-137; II-138 | 1.58 | 1.40 | 3.28 | 2.46 | 1.08 | 1.08 | 1.13 | Chrom remodeling |
Hs00428515_g1 | MAT2A | II-138 | 0.61 | 1.32 | 2.33 | 2.80 | 1.06 | 0.94 | 1.05 | Metabolism |
Hs00265885_g1 | EEF1A1 | II-138 | 0.86 | 0.97 | 0.86 | 0.84 | 1.35 | 1.06 | 1.07 | |
Hs00228436_m1 | CHD9 | II-138 | 0.87 | 1.34 | 2.06 | 1.09 | 1.25 | 0.84 | 1.16 | |
Hs00542737_m1 | SHPRH | II-138 | 0.72 | 1.23 | 2.12 | 1.90 | 1.04 | 1.04 | 1.11 | |
Hs99999906_m1 | PGK1 | II-138 | 0.73 | 0.78 | 0.98 | 1.45 | 0.65 | 1.18 | 0.90 | |
Hs00325656_m1 | NFIA | II-138 | 1.08 | 1.00 | 1.14 | 1.77 | 0.98 | 1.05 | 1.16 | |
Hs00187953_m1 | EIF2S1 | I-137; II-138 | 0.75 | 0.74 | 1.39 | 1.67 | 1.02 | 0.75 | 1.11 | |
Hs00831673_gH | EIF2S3 | I-138 | 0.94 | 0.86 | 1.10 | 1.13 | 0.66 | 0.91 | 1.20 | |
Hs00199471_m1 | SFRS1 | 1.01 | 1.08 | 1.56 | 1.70 | 1.16 | 1.00 | 1.22 |
Expression of each gene was evaluated by quantitative RT–PCR in cells transfected with a control plasmid, pVA, pVA1 or pmivaRNAI-138 and in cells infected with wild-type or a ΔVA mutant adenovirus at 12, 24, 48 and 72 hpi. Fold inhibition is indicated for each condition and was calculated by the ratio of expression levels in control cells versus levels in mivaRNA expressing cells. The probe used in the PCR, the putative targets and a functional category are also indicated for each gene. When fold inhibition was higher than 1.5 at 48 hpi and higher than 2.0 at 72 hpi (marked in bold), the gene was considered to show decreased expression in cells infected with the mivaRNA expressing virus. ND indicates that the expression of the gene was undetectable in infected cells expressing VA RNAs. TS indicates that the gene was also identified by TargetScan. UK denotes unknown function.
Microarray analysis
RNA isolated from cells mock transfected or transfected with pAdvantage and collected 3 days posttransfection was processed as recommended by Affymetrix. The affymetrix Test 2 Array was used to determine the quality of the RNA. After column cleanup of the RNA with Qiagen Rneasy, cDNA was synthesized by using an oligo(dT) primer attached to a sequence of the T7 promoter region. This cDNA was then used to perform in vitro transcription with bacteriophage T7 RNA polymerase and biotin-labeled nucleotides from the IVT Labeling kit (Affymetrix). Labeled RNA was purified, quantified spectrophotometrically, fragmented and hybridized to the Affymetrix Human Genome U133 Plus 2.0. The arrays were washed, stained, and scanned by using a confocal scanner (Affymetrix). Finally, the results were analyzed with GeneChip Operating Software (GCOS, Affymetrix).
Both background correction and normalization were carried out using RMA (Robust Multichip Average) algorithm (38). We first restricted the data analysis to probe sets in which percentage of present calls in at least one of the experimental conditions was >20% (Affymetrix Microarray suite user guide, version 5). This filter reduced the number of targets included in the experiment to 39 284 probe sets, which were considered for the statistical analysis. The selection of those genes differentially expressed in cells transfected with pAdvantage with respect to the mock transfected cells was performed using a t-test statistics (39). A cut-off P-value according to a FDR (40) of 5% was established as the selection criterion, resulting in a set of 1384 probe sets.
Functional categories enriched by Gene Ontology (GO) analysis were detected using a standard hypergeometric test (41). The results were complemented with Ingenuity Pathway Analysis (Ingenuity Systems, www.ingenuity.com), as this database includes manually curated and fully traceable data retrieved from literature. A proximal promoter analysis of the selected genes was performed with FactorY. This web application facilitates the identification of common regulatory mechanisms involved in the transcriptional control of co-expressed genes (42). Briefly, FactorY calculates the enrichment of transcription factor binding sites from the public versions of Jaspar (43) and Transfac (44) databases with a hypergeometric distribution. The proximal promoter of each differentially expressed gene is defined as 1000 bp upstream of the transcription start site and has been extracted from Ensembl database release 35 (45).
Sequence analysis for microRNA target prediction
MotifLocator can be used to screen DNA sequences with precompiled motifs (46). Motifs described in Figure 2C were scanned with MotifLocator against the 3′UTR sequence of each differentially expressed gene extracted from the Ensembl database release 35. Putative targets for adenoviral miRNAs and putative cellular miRNAs that may bind TIA-1 mRNA were searched for using TargetScan, MiRanda and PicTar (10,47,48). Targets for adenoviral miRNAs obtained from TargetScan were combined to differentially expressed genes identified in the VA microarray analysis. As a result, putative microRNA target genes with altered expression were identified.
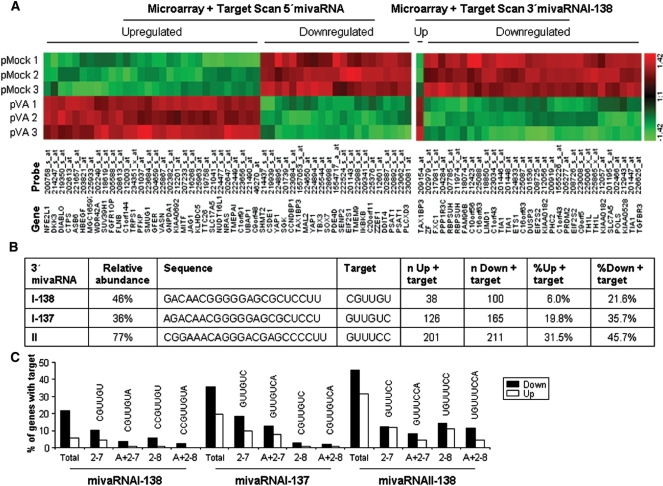
Analysis of genes with putative mivaRNA target sequences whose expression is altered in the presence of VA RNAs. (A) Color code of the VA RNA microarray expression of the genes identified by TargetScan using VAI miRNAs as input sequences. Expression of the genes listed at the bottom of the table was detected with the indicated probes. The probes were spotted on microarrays hybridized with RNA isolated from mock transfected cells (pMock1 to 3) or cells transfected with pVA (pVA1-3), as indicated to the left. Expression levels range from red (higher expression) to green (lower expression), as gradiented to the right. The genes are upregulated (Up) or downregulated in pVA transfected cells, as indicated at the top of the figure. The genes shown are also identified by TargetScan with 5′mivaRNAI sequences (left) or 3′ mivaRNAI-138 sequences (right). (B) Summary of the analysis of 3′mivaRNA seed-complementary sequences in the genes with altered expression in the VA RNA microarray. The sequence, target seed-complementary sequence and relative abundance of major 3′mivaRNAs from VAI and VAII are shown. The table also indicates the number (n) and percentage (%) of genes with seed complementary sequences which are upregulated (up) or downregulated (down) in the presence of VA RNAs. (C) Bar graph showing the percentage of genes with altered expression in the pVA microarray and with seed complementary sequences. Seeds chosen are either total or complementary to positions 2–7 or 2–8 of the seed with or without an extra A. Seed target sequence from mivaRNAI-138, mivaRNAI-137 and mivaRNAII-138 is indicated at the top of the graphs. Plotted genes are either downregulated (down) or upregulated (up) in the presence of VA RNA.
RESULTS
High-throughput analysis of genes altered upon expression of VA RNAs
Several approaches were used to identify cellular targets of mivaRNAs. As miRNAs induce mRNA decay and/or translational repression of target mRNAs, we first looked for proteins decreased in the presence of mivaRNAs. Thus, we compared the proteome of mock-transfected cells and cells transfected for three days with pAdvantage (pVA), which expresses RNA VAI and VAII. Several proteins were identified by mass-spectrometry that were decreased or increased in the presence of VA RNA (data not shown). However, none of the genes that encode for these proteins contained mivaRNA seed complementary sequences, neither in their 3′UTR nor in their coding region (data not shown). Therefore, we decided to use a transcriptomic approach to identify mivaRNA targets. RNA isolated from mock-transfected HeLa cells or HeLa cells transfected for three days with pVA, was hybridized to HG-U133-Plus2 microarrays (Figure 1A). The experiment was done three times to increase the significance of the statistical analysis (see Materials and methods section for details). The analysis of the microarrays indicated that 39 284 probes showed expression out of the 54 675 probes spotted in the array. Altered expression was significant for 4095 probes (P < 0.01) but a more stringent statistical analysis showed significant differences in 1384 probes (FDR < 0.05). We found that 730 probes, which correspond to 637 genes, were upregulated in pVA-transfected cells (Figure 1A; Supplementary Table S1). 654 probes, which correspond to 462 genes, were downregulated in the presence of pVA (Supplementary Table S1).
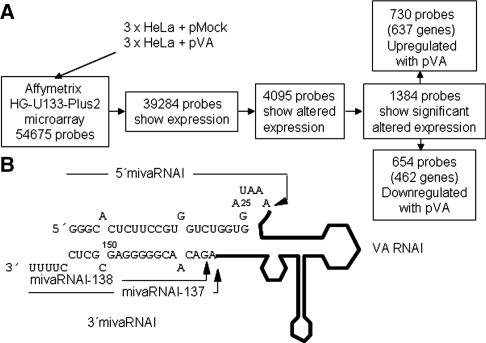
(A) Work flow of the microarray analysis. RNA isolated from triplicates of HeLa cells mock transfected or transfected with pVA was hybridized to Affymetrix HG-U133-Plus2 microarrays containing 54 675 probes. Out of the 39 284 probes that showed expression, 4095 showed different expression between mock and pVA transfected cells. Expression differences were significant for 1384 probes which corresponded to 637 upregulated genes (730 probes) and 462 downregulated genes (654 probes) in the presence of VA RNAs. (B). Schematic of VAI RNA processing to viral miRNAs. The terminal nucleotide sequence of VA RNAI processed to viral miRNAs is indicated. The structure of the remaining VA RNAI is depicted with a black line. VA RNAI is processed to a minor 5′ miRNA and two major 3′ miRNAs (mivaRNAI-137 and -138). The cleavage site for each miRNA is indicated with an arrow.
Upregulated genes showed a clear signature of NFκß activation (Supplementary Figure S1). We used FactorY to analyze the promoters of all upregulated genes and identify common transcription factors that regulate the expression of many of these genes. The results showed a significant enrichment of genes activated by NFκß. We believe that polymerase III-transcribed VA RNA, with 3p-5′-ends, activated RIG-I and therefore NFκß. NFκß activation leads to an increase of inflammatory cytokines such as IL6 and IL1, both found in the list of upregulated genes. We scanned upregulated genes with Ingenuity to find interaction networks. This is done by searching for links between genes into a database of curated information derived from the literature. This analysis showed that the network with the highest score contained NFκß-related genes (Supplementary Figure S1). Functional analysis of downregulated genes showed a single network exclusively related to RNA metabolism. Thus, 50 genes out of the 462 downregulated genes are involved in splicing, translation or encode for RNA binding proteins (Supplementary Table S2). Only few downregulated genes function in RNA polyadenylation, stability or transport. Besides, the transcription factor binding sites of Ets-1 and Elk were found statistically enriched upon FactorY analysis of downregulated genes. Indeed, Ets-1 and Elk were downregulated in the presence of pVA and this may account for the decreased expression of several genes.
Identification of putative mivaRNA-targeted genes altered in the presence of VA RNAs
VAI RNA is processed at the terminal stem to 5′ and 3′mivaRNAIs that could inhibit the expression of target genes (Figure 1B). To search for putative targets of these mivaRNAs, we performed a bioinformatic analysis using TargetScan (23). TargetScan is an algorithm that predicts targets of miRNAs by searching for conserved sites that match the seed region of a given miRNA. TargetScan analysis with 5′and 3′ mivaRNAIs as input sequences yielded 863 and 198 putative targets, respectively. Then, we looked for genes identified by TargetScan that were also downregulated in the microarray analysis. When we compared all the genes altered in the microarray analysis with the genes identified by TargetScan with the 5′mivaRNAI, we found 15 common genes downregulated, but also 30 common genes upregulated (Figure 2A, left). This result is discouraging, as it has been described in the analysis of other microarrays that study the effect of miRNAs, that few upregulated genes with target sequence should be found. This effect has been described as ‘antitarget signature’. In fact, the antitarget effect was found when we compared the genes altered in the microarray analysis with the genes identified by TargetScan with the 3′mivaRNAI. In this case, we found 23 common genes downregulated and only 1 common gene upregulated (Figure 2A, right). This result leads us to propose that the 3′mivaRNAI could inhibit gene expression at the level of mRNA stability of one or several of these 23 common genes.
TargetScan searches for seed complementary sequences in conserved regions. However, adenovirus is non-replicative in rodents, so sequence conservation should not be taken into consideration to define putative targets of mivaRNAs. Thus, we decided to use Motif Locator, which searches for a given sequence in all the genes included in a database. Motif Locator was used to identify seed complementary target sequences in the 3′UTR of all the genes with altered expression in the microarray analysis. The number of VA RNA upregulated genes with seed complementary sequences was calculated to gauge the specificity of the analysis. We defined the best antitarget effect as the highest ratio of the relative number of seeds in downregulated genes versus the relative number of seeds in upregulated genes. Three different sequences were used as input for the Motif Locator analysis. These sequences correspond to the seeds of the two major 3′mivaRNAs of VAI (137 and 138) (Figure 1B) and the major 3′mivaRNA of VAII (Figure 2B). Also, seeds contained nucleotides 2–7 or 2–8 of the mivaRNA and may have an Adenine at the end of the sequence (Figure 2C) (10). The results of these analyses show that in all cases the number of seed complementary sequences in downregulated genes is higher than in upregulated genes, indicating a certain antitarget effect (Figure 2B and and2C).2C). As expected, the antitarget effect increased when the analysis was performed with longer seeds (Figure 2C). The best antitarget effect was obtained with the seed of the mivaRNAI-138. Analysis with the other seeds identified a higher number of putative targets, even if the GCU content of the three sequences tested was almost identical (Figure 2B).
Analysis of TIA-1 mRNA as a mivaRNAI-138 putative target
A set of rules was defined to choose a putative target of mivaRNAs that could be studied further. We concentrated on putative targets of mivaRNAI-138, as they showed the best antitarget effect (Figure 2B and Figure 2C). Also, we decided to choose a VA RNA downregulated gene that was also identified by TargetScan. These conditions limited the possibilities to 23 genes. Finally, as VA RNA downregulated genes showed a significant increase for genes related to splicing and translation (Supplementary Table S2), we arbitrarily decided to choose the only candidate involved in both processes. Thus, we decided to analyze the effect of mivaRNAI-138 in the expression of the T-cell intracellular antigen 1 gene (TIA-1).
The putative target of the mivaRNAI-138 found in TIA-1 mRNA fulfils several requirements to be considered a good target (11). First, the target locates in the 3′UTR close to the stop codon of TIA-1 mRNA (Figure 3A). Second, the target can base-pair to a seed of 7 nt in the mivaRNAI-138 and pairing can be extended to nucleotides 12–20 (Figure 3A). Furthermore, the seed complementary sequence is located between a region particularly enriched for A and U nucleotides (Figure 3A). Finally, ClustalX analysis has shown that the target is well-conserved in Pan troglodytes and the seed complementary sequence has a single C to U change in Mus musculus, which could still lead to a G : U pairing (Figure 3A).
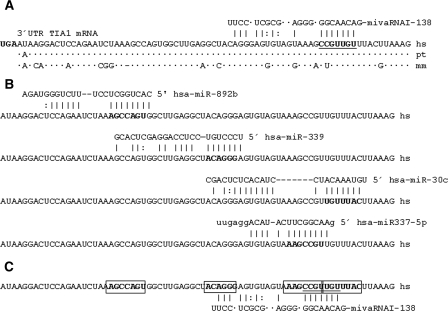
Analysis of the putative miRNA target sequence in TIA-1 3′UTR. (A) mivaRNAI-138 target sequence in TIA-1 mRNA. Human (hs) TIA-1 3′UTR sequence close to the stop codon (bold) is shown. Non-conserved nucleotides of the same sequence in chimpanzee (pt) and mice (mm) are indicated. The putative binding showed between the mivaRNAI-138 and TIA-1 mRNA has been predicted by TargetScan. Base pairing is indicated with a line and GU wobbles are connected with a colon. The seed target is highlighted in bold and underlined. (B and C) Target sequences in TIA-1 3′UTR from cellular miRNAs. (B) TargetScan predicted binding between TIA-1 3′UTR and hsa-miR-892b, hsa-miR-339, hsa-miR-30c and hsa-miR-337-5p. (C) Seed complementary sequences to the miRNAs shown in (B) are boxed. mivaRNAI-138 seed binds to the seed target sequence of hsa-miR-30 and hsa-miR-337-5p.
The 3′UTR of TIA-1 mRNA was scanned with PicTar, TargetScan and miRanda, which predict miRNA targets. These programs revealed that several cellular miRNAs could bind to TIA-1 mRNA close to the putative mivaRNAI-138 target site, so they could theoretically act cooperatively (Figure 3B). These include hsa-miR-892b, hsa-miR-339, hsa-miR-337-5p and several hsa-miR-30 (Figure 3B; note that only one hsa-miR-30 is shown). Interestingly, two cellular miRNAs, hsa-miR337-5p and hsa-miR-30, could bind to adjacent seed complementary sequences that could, probably, define an accessible region targeted by mivaRNAI-138. We could not find any cellular human miRNA with the same seed sequence as the mivaRNAI-138.
TIA-1 is downregulated in the presence of mivaRNAI-138
We wanted to validate the downregulation of TIA-1 mRNA upon VA RNA expression observed in the microarray analysis. To this aim, we used different plasmids that express the mivaRNAI-138. The plasmids chosen were pVA, pVAI, which only expresses VAI RNA from a polymerase III histone promoter, and pmivaRNAI-138, which uses the same promoter to express a shRNA processed to accumulate the mivaRNAI-138. Expression of mivaRNAI-138 was confirmed by primer extension in cells transfected with these plasmids (Supplementary Figure S2). RNA isolated from HeLa or 293 cells mock-transfected or transfected with pVA, pVAI or pmivaRNAI-138, was also used to perform quantitative RT–PCR analysis of TIA-1 mRNA. The result shows that TIA-1 mRNA is decreased to similar levels in HeLa or 293 cells expressing VA RNAs, only VAI RNA or solely the mivaRNAI-138 (Figure 4A and B).
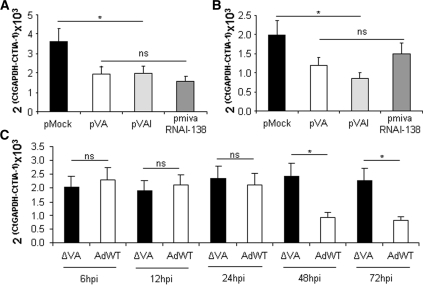
TIA-1 mRNA is downregulated in the presence of VA RNA. (A and B) TIA-1 mRNA is downregulated in cells transfected with pVA. RNA was isolated from 293 (A) or HeLa (B) cells mock transfected or transfected with pVA, pVAI or pmivaRNAI-138. Then, GAPDH and TIA-1 mRNAs were quantified by RT–PCR and plotted for comparison. (C) TIA-1 mRNA is downregulated at late times postinfection with wild-type adenovirus. The 293 cells were infected with wild-type (AdWT) or a ΔVA mutant adenovirus and RNA was isolated at 6, 12, 24, 48 and 72 hpi. Quantitative RT–PCR was performed as indicated in (A). Error bars show standard deviations of three different experiments. Asterisk highlights significant differences while ns indicates non-significant differences.
TIA-1 mRNA levels were also evaluated in 293 cells infected with wild-type adenovirus or an adenovirus mutant that does not express VA RNAs. The quantitative RT–PCR analysis of TIA-1 mRNA was evaluated at 6, 12, 24, 48 and 72 h postinfection (hpi). The results show that similar levels of TIA-1 mRNA are observed in cells infected with the adenovirus mutant or in cells infected with wild-type adenovirus at early times postinfection (Figure 4C). However, TIA-1 mRNA levels decrease in cells infected with wild-type adenovirus for 48 or 72 h, whereas no changes were observed in cells infected with the mutant adenovirus (Figure 4C).
Similar results are observed when TIA-1 protein is evaluated by western blot analysis (Figure 5). TIA-1 is decreased to similar levels in cells expressing VA RNAs, VAI RNA or mivaRNAI-138 (Figure 5A). Quantification of different experiments indicates that TIA-1 decreases 2-fold in the presence of mivaRNAI-138 (data not shown). Two-fold is an expected miRNA-mediated repression. A similar decrease was observed in cells infected with wild-type adenovirus for 24 h, when, in our experimental settings, the initiation of the late phase of adenovirus infection occurs (Figure 5B). To determine whether TIA-1 is also downregulated by mivaRNAI-138 in adenovirus-infected cells, we blocked 3′mivaRNAI functionality with antagomirs. We found that TIA-1 expression is not altered in adeno-infected cells transfected with an antagomir complementary to the mivaRNAI-138, while TIA-1 is decreased 40% in infected cells transfected with a mutant antagomir (Figure 5B).
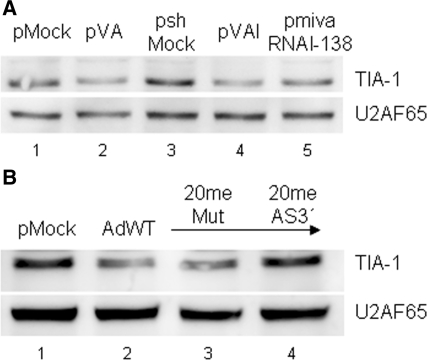
TIA-1 protein is downregulated in the presence of VA RNA. (A) TIA-1 protein is downregulated in cells transfected with pVA. HeLa cells were transfected with control plasmids expressing a non-related gene (pMock) or a non-related shRNA (pshMock) or with pVA, pVAI or pmivaRNAI-138 plasmids. Extracts were collected at 48 h posttransfection and TIA-1 and U2AF65, used as a loading control, were evaluated by western blot analysis. (B) TIA-1 protein is downregulated in cells infected with wild-type adenovirus with functional 3′mivaRNAIs. 293 cells were transfected with antagomiRs that block 3′mivaRNAI function (2OmeAS3′) or with a mutant control (2OmetMut). These cells were infected with AdWT at 24 h posttransfection. Untransfected cells were also infected or mock infected, as a control. Extracts were collected at 24 hpi and TIA-1 and U2AF65 proteins were analyzed as in (A).
TIA-1 mRNA is targeted directly by 3′mivaRNA
mivaRNAI-138 could downregulate TIA-1 expression directly or indirectly, as a secondary effect of mivaRNAI-138 targeting a gene involved in TIA-1 expression. To discriminate between these two possibilities, we performed a reporter assay. The putative target sequence of mivaRNAI-138 in TIA-1 was cloned in the 3′UTR of a Renilla luciferase reporter (Figure 6A). A mutant version in the seed-complementary sequence was also cloned as a negative control (Figure 6A). Renilla luciferase activity was measured in cells transfected with either of these plasmids and a control plasmid or plasmids that express VAI and VAII RNAs, only VAI or mivaRNAI-138. A plasmid expressing Firefly luciferase was also co-transfected in all cases and Firefly luciferase activity was measured as a transfection control. The result shows that mivaRNAI-138 expression decreases 2-fold Renilla luciferase expression from the Renilla luciferase mRNA with target sequences (Figure 6B). Renilla luciferase expression from the construct that lacks a seed complementary sequence was not altered, suggesting that pairing between the seed sequence of mivaRNAI-138 and the target mRNA is required for inhibition. These results allow us to suggest that TIA-1 has a target sequence that could interact directly with mivaRNAI-138, which could induce miRNA-mediated downregulation of TIA-1 mRNA.
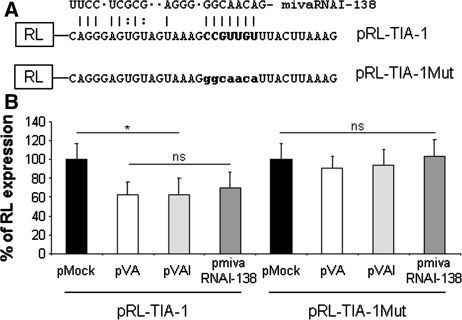
TIA-1 mRNA is a direct target of mivaRNAI-138. (A) Schematic of Renilla luciferase mRNA with TIA-1 mivaRNAI-138 target sequence (pRL-TIA-1) or with a matching seed complementary mutated sequence (pRL-TIA-1Mut). mivaRNAI-138 binding to the RNA is indicated for clarity. (B) mivaRNAI-138 decreases expression of a Renilla luciferase reporter mRNA with TIA-1 mivaRNAI-138 target sequences. HeLa cells were transfected with the plasmids expressing the Renilla constructs shown in (A), and a control plasmid or pVA, pVAI and pmivaRNAI-138 plasmids. A plasmid expressing a Firefly luciferase reporter was also co-transfected as a control. Luciferase activity was evaluated at 48 h posttransfection. Renilla luciferase expression in control cells was set to 100% and the percentage of Renilla expression in all cases was calculated accordingly. Error bars show standard deviations of three different experiments. Asterisk highlights significant differences while ns indicates non-significant differences.
Several genes are downregulated in the presence of mivaRNAs
The results obtained with TIA-1 were encouraging, as TIA-1 was chosen arbitrarily from a list of 462 genes downregulated in the VA RNA microarray. Thus, we wondered whether the expression of other genes from the same list could also be controlled by mivaRNAs. Motif Scanner searches using the major adenoviral mivaRNAs as input sequences, found seed complementarity in the 3′UTR of 305 genes out of the 462 downregulated in the VA RNA microarray (Supplementary Table S3). We decided to study 50 of these genes in more detail. We also analyzed as a control SFRS1, which was found downregulated in the VA RNA microarray but lacked seed complementarity to major mivaRNAs. Out of the 50 genes chosen, 22 have good seed complementarity to mivaRNAI-138. In fact, 14 of them were also identified by TargetScan. We also included genes with good seed complementarity (A + 2-8, see Figure 2C) to mivaRNAI-137 (n = 4) or to mivaRNAII-138 (n = 12). Twelve random genes of well-known function were also chosen.
We decided to use quantitative RT–PCR as a simple method to test whether the expression of these genes decreases in cells expressing mivaRNAs. This technique was able to detect expression of all genes except EIF2S2 and USP6, which were eliminated from the study. First, we quantified RNA levels of the remaining 49 genes and GAPDH, used as a control, in 293 cells infected for 12, 24, 48 or 72 h with a moi of 1 of wild-type adenovirus (WT) or the adenovirus mutant that does not express VA RNAs (ΔVA). The results were used to calculate the fold inhibition (FI) for each gene and time postinfection, defined as the ratio of the expression levels obtained in cells infected with ΔVA versus the levels in cells infected with WT (Table 1). We consider that genes strongly inhibited by VA RNA expression should show FI higher than 1.5 at 48 hpi and higher than 2.0 at 72 hpi. Sixteen genes did not fit these criteria. However, the FI of the remaining 33 genes fit the criteria and their FI tended to increase at later times postinfection. In these cases, expression levels in ΔVA-infected cells were higher than in WT virus-infected cells, although the mRNA content could actually decrease in the course of infection (Figure 7A).
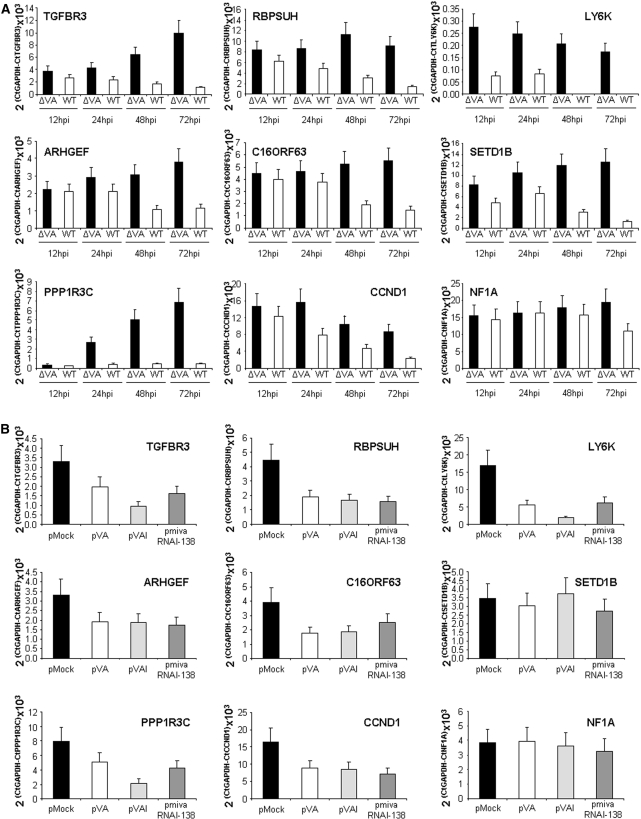
mivaRNAs downregulate the expression of several genes. Expression of each gene was evaluated by quantitative RT–PCR in cells infected with wild-type or a ΔVA mutant adenovirus at 12, 24, 48 and 72 hpi (A) and in cells transfected with a control plasmid, pVA, pVAI or pmivaRNAI-138 (B). Error bars show standard deviations of three different measurements. Only representative genes are shown.
To test whether mivaRNAs were involved in the observed inhibition of gene expression, we evaluated RNA levels of the 49 genes in HeLa cells transfected with pVA, pVAI, pmivaRNAI-138 or with a control plasmid. The results are shown in Figure 7B and expressed as FI in Table 1. Out of the 33 genes downregulated in infected cells expressing VA RNAs, 30 genes were also downregulated after transfection with pVAI and 26 were inhibited after transfection with pmivaRNAI-138, suggesting that mivaRNAI-138 could control the expression of these genes also in infected cells. Besides, eight genes were downregulated in pVA transfected cells but not in WT adenovirus infected cells, indicating that they could escape mivaRNA control during infection. Noticeably, inhibitions were normally higher in infected cells than in transfected cells expressing VA RNAs or mivaRNAs.
DISCUSSION
We have employed several methods to identify cellular targets of adenoviral miRNAs: bioinformatics, proteomics and genomics. Computer predictions using as input 5′ mivaRNAI and 3′ mivaRNAI-138, mivaRNAI-137 and mivaRNAII-138 retrieved a long list of putative targets. Note that a difference in Dicer cleavage of only 1 nt yields two different mivaRNAs, mivaRNAI-137 and 138, with a 1 nt shift in the seed sequence. This results in different targets being regulated by miRNAs very similar in sequence. This could also be observed for cellular miRNAs and could theoretically be regulated.
Proteomic analysis of factors decreased in the presence of mivaRNAs did not allow identification of target genes. The differences observed in the proteome may have resulted from secondary effects of VA RNA expression. Real targets may have been difficult to identify by proteomics due to charge, low abundance or few differences in expression in the presence and absence of the miRNA regulator. Modifications to the proteomics protocol could have been applied to increase the number of proteins detected and ease quantification (51). Alternatively, we decided to analyze the impact of VA RNA expression on mRNA accumulation with human whole genome microarrays. The combination of the results obtained with the microarray analysis and bioinformatic searches allowed us to obtain a short list of VA RNA-downregulated genes with putative target sequences (Figure 2, Supplementary Table S3).
VA RNA overexpression can block Dicer and Exp5 (17,21). Dicer and Exp5 inhibition should lead to a decrease in cellular miRNAs and, therefore, an increase in miRNA-targets. However, completion of this cascade should take several days, as cellular miRNAs are very stable (33). Also, overexpression of adenoviral miRNAs should saturate RISC (17). However, we found no upregulation of any well-characterized miRNA targets, such as BCL2, PDCD4, CDKN2A or PTEN, which are regulated by miRNAs highly expressed in HeLa cells (52–55). It may be that in our experimental conditions, viral miRNA production was not high enough to saturate RISC.
VA RNA decreases mRNA levels of 462 genes. This could be caused by decreased transcription and/or increased decay. Transcription of several downregulated genes is controlled by Ets-1 and Elk, which decrease in the presence of VA RNA. VA RNAs are processed to several viral miRNAs that could activate decay of target mRNAs. The major miRNAs are mivaRNAI-137, mivaRNAI-138 and mivaRNAII-138 (28). Other viral miRNAs are also produced from VA RNAs to lower levels, and therefore should have a lower capacity for gene regulation (23,28). This is the case of 5′ mivaRNAI, which has been shown to have a lower impact than 3′mivaRNAI in the inhibition of genes with complementary sequences and on virus functionality (23). Also, we found no ‘antitarget signature’, a relative decrease in mRNAs upregulated by VA RNA with 5′ mivaRNAI targets (Figure 2A). The antitarget signature is nicely observed for the major mivaRNAs (Figure 2).
The results show that out of 462 downregulated genes, 305 have seed complementary sequences to major mivaRNAs and 172 have more than 1 putative target for major mivaRNAs in all possible combinations (Supplementary Table S3). Fifty genes were further evaluated and eight showed decreased expression only in cells expressing VA RNA upon transfection, indicating that they could escape VA RNA or mivaRNA control during infection. Regulation of miRNA-mediated inhibition has been already described for some cellular miRNA targets. Thirty-one of the evaluated genes showed decreased expression when VA RNA expression was achieved by transfection or infection (Figure 7, Table 1). Five of them have unknown functions. Seven are related to cell signalling (ADCY9, ARHGEF7, MAP3K3, DUSP3, PPP1R3C, PTP4A1 and TGFBR3) and nine to cell growth and apoptosis (PTP4A1, TGFBR3, LY6K, CCND1, BNIP3L, BNIP3, TIA-1, ETS1 and CDK8). Nine are related to DNA and involved in transcription or DNA repair (ETS1, CDK8, RBPSUH, PHF20, TH1L, PHC2, POLS, EXO1 and UBE2N), and five are involved in RNA metabolism (DAZAP2, MDN1, CUGBP1, QKI and TIA1) (Table 1). Interestingly, other authors have already shown that DNA repair and apoptosis are inhibited in adenovirus-infected cells. Moreover, miRNAs derived from other viruses, such as KSHV and EBV, have been shown to target proapoptotic genes. Inhibitions are normally higher in infected cells than in transfected cells expressing VA RNAs or mivaRNAs. This could reflect that infected cells saturate the RISC machinery with viral mivaRNAs so the RISC complex could be more efficient in silencing mivaRNA target genes. Alternatively, there could be other mechanisms that cause decreased expression of these genes in infected cells, as seems to be the case for SETD1B, PRDM2 and MAT2A. Some genes show inhibitions higher than average also in transfected cells expressing mivaRNAI-138, indicating that those genes could have several target sites which act cooperatively. Thirty-two out of the 50 genes evaluated are downregulated in cells expressing mivaRNAI-138. Out of the 15 genes identified by TargetScan and analyzed further, 13 were downregulated in cells expressing mivaRNAI-138. This highlights the efficacy of the prediction program. Small scale studies will be required to determine whether the genes downregulated in mivaRNAI-138 expressing cells are direct targets of this miRNA and to evaluate their effects in adenoviral infections.
The list of genes downregulated by VA RNA shows a statistically significantly increase of genes related to RNA binding, splicing and translation (Supplementary Table S2). It is unclear to us why VA RNA expression decreases many genes related to RNA metabolism. Also, most of these genes do not contain seed-complementary sequences to major mivaRNAs. One exception is TIA-1, an RNA binding protein related to splicing and translation. TIA-1 has a good mivaRNAI-138 target sequence according to several published parameters (Figure 3 and see the Results section) (11). We were surprised to see that mivaRNAI-138 targets a region which is also predicted to be seed-complementary to two other cellular miRNAs (Figure 3B). We speculate that 3′UTR sequences capable of being regulated by miRNAs could be rare, as they need to be accessible. Therefore, when a virus develops functional miRNAs to control cellular gene expression, there could be a high possibility that they target similar sequences than cellular miRNAs. In fact, a viral miRNA with the same seed sequence as a cellular miRNA has been described (56,57). Thus, the presence of cellular miRNA targets could be a benefit in an algorithm for the prediction of viral miRNA targets. A disadvantage of sharing target sequences is that there could be competition between cellular and viral miRNAs. This should not be a problem in the case of adenovirus as major mivaRNAs are expressed to high levels.
TIA-1 mRNA is a direct target of mivaRNAI-138. TIA-1 protein and mRNA are downregulated in transfected cells that express mivaRNAI-138 or in cells infected with an adenovirus that expresses functional mivaRNAI (v 4 and 5). Our results suggest that mivaRNAI-138 inhibits translation and activates RNA decay of TIA-1 mRNA. mivaRNAI-138 is detected at 12 hpi and accumulates as infection proceeds (23). A decrease in TIA-1 protein was first detected 24 hpi (v 5) and later, at 48 hpi, we observe a downregulation of TIA-1 mRNA (Figure 4). We also used a reporter assay to define mivaRNAI-138 target in TIA-1 and to prove that mivaRNAI-138 binds directly to this target. Note that this region is also a putative target for hsa-miR337-5p and hsa-miR-30, but these miRNAs are poorly expressed in 293 and HeLa cells, so they should not control the expression of the Renilla reporters (compare Mock bars in Figure 6B).
mivaRNAI-138 has been only characterized in adenovirus family C (Adenovirus 2 and 5). Members from other subgenera (A, B, D, E or F) also express VA RNAs with a terminal stem that could be cleaved by Dicer. However, the sequence and the structure of the terminal stem is different in different families, including bulges and G:U wobbles at different positions that could affect Dicer cleavage. Therefore it is difficult to predict whether Dicer cleavage could occur at the same position in VA RNAs from all families. Assuming Dicer cleaves at identical position or allowing a difference in cleavage of 1 nucleotide, we have found seed complementary sequences in human TIA-1 mRNA from putative mivaRNA-138s derived from all human adenovirus subgenera (data not shown). Interestingly, several seed complementary sequences from putative mivaRNA-138s derived from simian adenovirus have been found in the 3′UTR of TIA-1 mRNA from macaca mulatta (data not shown). This suggests that VA RNAs from different adenovirus could produce mivaRNA-138s with different sequences that also target TIA-1.
TIA-1 is a well-characterized factor that activates apoptosis by controlling the RNA metabolism of some mRNAs that encode for proapoptotic molecules (58). We hypothesized that adenovirus infection could proceed favorably with decreased TIA-1 and, therefore, in a less apoptotic environment. However, none of the experiments performed so far have demonstrated this hypothesis (data not shown). TIA-1 binds to U rich sequences to control RNA metabolism by increasing splicing or by blocking translation (49, 59–61). Several of the adenoviral mRNAs contain putative U rich TIA-1 binding sites in introns or 3′UTRs. Further studies will be required to address whether TIA-1 can control expression of adenoviral genes. In fact, functional mivaRNAIs are important for adenovirus production, as cells with antagomir-blocked mivaRNAIs produce lower viral titers (23). Therefore inhibition of TIA-1 or other mivaRNAI targets could be relevant for virus production. We cannot exclude that mivaRNA control of many of these genes at the same time could fine tune the expression pattern in the cell to one that is more advantageous for viral growth.
FUNDING
Ministerio de Ciencia e Innovación (SAF2003-01804, BIO2006-13225, FPI to O.A.); Instituto Carlos III (C03/02); DIGNA Biotech and through the agreement between FIMA and the ‘UTE project CIMA’.
Conflict of interest statement. None declared.
ACKNOWLEDGEMENTS
We are grateful to J. Valcárcel and N. Majos for antibodies to TIA-1 and U2AF65 and pHA-TIA-1 plasmid, R. Alemany for providing AdΔVA, to R. Hernández-Alcoceba for help in adenovirus amplification and titration, and E. Casales for technical assistance.
REFERENCES
Articles from Nucleic Acids Research are provided here courtesy of Oxford University Press
Full text links
Read article at publisher's site: https://doi.org/10.1093/nar/gkp1028
Read article for free, from open access legal sources, via Unpaywall:
https://academic.oup.com/nar/article-pdf/38/3/750/16768118/gkp1028.pdf
Free to read at nar.oxfordjournals.org
http://nar.oxfordjournals.org/cgi/reprint/38/3/750.pdf
Free to read at nar.oxfordjournals.org
http://nar.oxfordjournals.org/cgi/content/abstract/38/3/750
Free to read at nar.oxfordjournals.org
http://nar.oxfordjournals.org/cgi/content/full/38/3/750
Citations & impact
Impact metrics
Citations of article over time
Alternative metrics
Smart citations by scite.ai
Explore citation contexts and check if this article has been
supported or disputed.
https://scite.ai/reports/10.1093/nar/gkp1028
Article citations
Quantitative Virus-Associated RNA Detection to Monitor Oncolytic Adenovirus Replication.
Int J Mol Sci, 25(12):6551, 14 Jun 2024
Cited by: 0 articles | PMID: 38928259
Anti-Adenoviral Effect of Human Argonaute 2 Alone and in Combination with Artificial microRNAs.
Cells, 13(13):1117, 28 Jun 2024
Cited by: 0 articles | PMID: 38994969 | PMCID: PMC11240694
miRNA Pathway Alteration in Response to Non-Coding RNA Delivery in Viral Vector-Based Gene Therapy.
Int J Mol Sci, 23(23):14954, 29 Nov 2022
Cited by: 4 articles | PMID: 36499289 | PMCID: PMC9741442
Review Free full text in Europe PMC
Sequence-Specific Features of Short Double-Strand, Blunt-End RNAs Have RIG-I- and Type 1 Interferon-Dependent or -Independent Anti-Viral Effects.
Viruses, 14(7):1407, 28 Jun 2022
Cited by: 1 article | PMID: 35891387 | PMCID: PMC9322957
Viral long non-coding RNA regulates virus life-cycle and pathogenicity.
Mol Biol Rep, 49(7):6693-6700, 17 Mar 2022
Cited by: 9 articles | PMID: 35301646 | PMCID: PMC8929458
Review Free full text in Europe PMC
Go to all (57) article citations
Data
Data behind the article
This data has been text mined from the article, or deposited into data resources.
BioStudies: supplemental material and supporting data
Similar Articles
To arrive at the top five similar articles we use a word-weighted algorithm to compare words from the Title and Abstract of each citation.
Identification of RISC-associated adenoviral microRNAs, a subset of their direct targets, and global changes in the targetome upon lytic adenovirus 5 infection.
J Virol, 89(3):1608-1627, 19 Nov 2014
Cited by: 21 articles | PMID: 25410853 | PMCID: PMC4300742
Adenovirus-encoding virus-associated RNAs suppress HDGF gene expression to support efficient viral replication.
PLoS One, 9(9):e108627, 02 Oct 2014
Cited by: 4 articles | PMID: 25275311 | PMCID: PMC4183520
Small RNA sequence analysis of adenovirus VA RNA-derived miRNAs reveals an unexpected serotype-specific difference in structure and abundance.
PLoS One, 9(8):e105746, 21 Aug 2014
Cited by: 15 articles | PMID: 25144466 | PMCID: PMC4140831
Adenovirus and miRNAs.
Biochim Biophys Acta, 1809(11-12):660-667, 17 May 2011
Cited by: 13 articles | PMID: 21621026 | PMCID: PMC7102710
Review Free full text in Europe PMC