Abstract
Free full text

Factors affecting Aβ plasma levels and their utility as biomarkers in ADNI
Abstract
Previous studies of Aβ plasma as a biomarker for Alzheimer’s disease (AD) obtained conflicting results. We here included 715 subjects with baseline Aβ1–40 and Aβ1–42 plasma measurement (50% with 4 serial annual measurements): 205 cognitively normal controls (CN), 348 patients mild cognitive impairment (MCI) and 162 with AD. We assessed the factors that modified their concentrations and correlated these values with PIB PET, MRI and tau and Aβ1–42 measures in cerebrospinal fluid (CSF). Association between Aβ and diagnosis (baseline and prospective) was assessed. A number of health conditions were associated with altered concentrations of plasma Aβ. The effect of age differed according to AD stage. Plasma Aβ1–42 showed mild correlation with other biomarkers of Aβ pathology and were associated with infarctions in MRI. Longitudinal measurements of Aβ1–40 and Aβ1–42 plasma levels showed modest value as a prognostic factor for clinical progression. Our longitudinal study of complementary measures of Aβ pathology (PIB, CSF and plasma Aβ) and other biomarkers in a cohort with an extensive neuropsychological battery is significant because it shows that plasma Aβ measurements have limited value for disease classification and modest value as prognostic factors over the 3-year follow-up. However, with longer follow-up, within subject plasma Aβ measurements could be used as a simple and minimally invasive screen to identify those at increased risk for AD. Our study emphasizes the need for a better understanding of the biology and dynamics of plasma Aβ as well as the need for longer term studies to determine the clinical utility of measuring plasma Aβ.
Introduction
Alzheimer’s disease (AD) is neuropathologically defined by the deposition of intracellular tau aggregates, extracellular amyloid beta (Aβ) deposits and synapse loss. These findings, coupled with other data, led to the amyloid cascade hypothesis and thus to the development of biomarkers to measure Aβ pathology including Aβ imaging and measurements of Aβ peptides in cerebrospinal fluid (CSF) and plasma [22, 49]. In turn, biomarkers have advanced our understanding of the temporal profile of the pathophysiological events that occur during the progression of AD [24]. For example, measurement of CSF Aβ has been shown to be accurate and informative diagnostically for distinguishing cognitively normal subjects from AD subjects [49], predicting conversion of MCI subjects [10, 50] and in the differential diagnosis with frontotemporal degeneration [11]. CSF Aβ values also show a strong negative correlation with Pittsburgh Compound B (PIB) PET values [13, 14, 57]. But there is a compelling need for minimally invasive plasma biomarkers [1].
However, studies of plasma Aβ have been contradictory and cross-sectional analyses have reported higher Aβ1–42 levels [36–38], higher Aβ1–40 [36], lower levels of Aβ1–42 [31, 34] as well as lower Aβ1–42/1–40 ratio (AβR) values [5, 31, 34] in AD patients. Further, when other studies adjusted for different factors, they only found a mild effect of baseline Aβ1–40 as a predictor for developing MCI, while the other associations with plasma Aβ disappeared after multivariate adjustment [17, 33, 54].
In cohort studies, baseline higher Aβ1–40 concentration [8, 52, 55], higher levels of Aβ1–42 [8, 30, 36, 37, 46], high AβR [36, 37] or low AβR [20, 28, 55, 59] were associated with risk for AD [36]. Studies that measured plasma Aβ concentrations at two time points in cohort studies report that a decrease of plasma Aβ1–42 [8, 37, 46–48] and a decrease of the AβR [46–48] were associated with progression to AD or with cognitive decline. Two studies measured plasma Aβ levels at several time intervals but did not describe the temporal profile of changes in their concentrations [32, 47, 48].
Plasma Aβ concentrations have also been linked to vascular brain pathology in the case of Aβ40 [21] and in longitudinal studies of plasma Aβ concentrations were related to progression to mixed and vascular dementia [28] and hypertension [29].
Factors that lead to the discrepancies in the literature on the diagnostic and prognostic utility of measuring plasma Aβ are not well understood, but could reflect many clinical and methodological factors that affect plasma levels [3, 4, 9, 37], while age may act as a confounding factor [17, 20, 33, 52] (plasma Aβ concentrations could have different values at different stages of disease).
To address all these conflicting results, we analyzed different factors affecting Aβ concentration in order to be able to adjust the statistical models used to test the considered hypotheses. The relationship of the Aβ burden measured by biomarkers in different biological compartments was tested. We then tested the association with cerebrovascular lesions and vascular risk factors followed by testing the utility for baseline classification. Finally, we tested the longitudinal changes of Aβ plasma concentrations across time at different stages of the disease and the utility of Aβ plasma as a prognostic factor in AD.
Materials and methods
Subjects
The ADNI is a multicenter longitudinal neuroimaging study, launched in 2004 by the National Institute on Aging, the National Institute of Biomedical Imaging and Bioengineering, the Food and Drug Administration, private pharmaceutical companies and non-profit organizations. ADNI includes 819 adult subjects, 55–90 years old, who meet entry criteria for a clinical diagnosis of amnestic mild cognitive impairment (MCI), probable AD or cognitively normal (CN), who are prospectively followed, gathering clinical information, neuroimaging studies and biological samples for molecular biomarker measurement as previously described [23, 25, 39, 49] (for more details, see http://www.adni-info.org/index and supplementary material). We further classified subjects as stable if they did not change their diagnostic category from CN to MCI/AD or from MCI to AD, having a follow-up of at least 36 months. Patients who progressed were classified as CN progressors and MCI progressors.
Plasma and CSF Aβ1–42 and Aβ1–40
Plasma concentrations of Aβ1–42 and Aβ1–40 were measured using Module A of the INNO-BIA plasma Aβ forms immunoassay kit (Innogenetics, Ghent, Belgium, for research use-only reagents) on the Luminex 100 immunoassay platform and IS v.2.3 software (Luminex, Austin, TX, USA) using a fully automated sample preparation approach and lowest calibrator concentrations of 3.75 pg/mL and 1.25 pg/mL for Aβ1–40 and Aβ1–42, respectively (Figurski et al., manuscript in preparation) and other operating conditions as previously described [5, 27]. The same reagents were used for all samples. The inter-assay %CV obtained from daily measurement of aliquots prepared from two human subject plasma samples was 4.1% (n = 75) and 5.2% (n = 42) for Aβ1–42 and 7.0 and 5.5% for Aβ1–40. After each of 75 analytical runs, 2–3 samples were randomly selected for re-testing of a second never previously thawed aliquot. The average %CV obtained for 195 test–retest pairs was: 4.5%CV for Aβ1–42 and 7.2%CV for Aβ1–40. The qualification of this assay has been described in studies by Vanderstichele, Shaw and colleagues and the data are available at http://www.adni-info.org/index.
Details of the CSF collection, measurements and the formula to classify subjects by normal and pathological CSF signature can be found in Shaw et al. [49].
Pittsburgh Compound B PET imaging
ADNI PIB PET studies were performed at 14 different sites, where the production and radiolabeling of PIB were performed as outlined previously by Mathis et al. [35]. The ADNI PIB PET images undergo several quality control and standardization steps. Regional assessment of the PIB-PET data involves sampling 13 different brain areas using an automated region of interest (ROI) template method and standardized uptake value ratios were calculated as reviewed in Jagust et al. [25], using a cerebellar gray matter reference region. A PIB retention summary measure was formed by combining anterior cingulate cortex, lateral temporal cortex, precuneus, parietal and frontal cortex ROI values for each subject.
MRI and white matter hyperintensity volume
Acquisition of 1.5-T MRI data at each performance site followed a previously described standardized protocol that was rigorously validated across sites [23]. Then, for each fast-spin echo scan, the proton density and T2 images were linearly combined to form a “pseudo-T1”, which was aligned to the T1 scan and non-brain tissues were removed from the T1 scan. White matter hyperintensities (WMH) were detected in minimum deformation template space at each voxel based on corresponding PD, T1, and T2 intensities, the prior probability of WMH, and the conditional probability of WMH based on the presence of WMH at neighboring voxels. The resulting map of WMH voxels across the brain is summarized by an estimate of total WMH volume (WMHV) [7]. Infarctions were ascertained through qualitative review by experts in Helen Wills Institute on the PD/T2 images.
Cognitive testing
Neuropsychological evaluation and criteria for the clinical diagnosis of MCI and AD have been described [39]. Briefly, the battery consists of global assessment scales (ADAS-Cog [44], MMSE [16]) and a neuropsychological battery that assesses different cognitive domains: story A from the Logical Memory Test [56], Rey Auditory Verbal Learning Test [43], the Boston Naming Test [26], Category Fluency Test [6], Trail Making Test [41], Digit Symbol Substitution Test [56] and Digit Span Test [19, 56].
Measures of cognitive reserve
The errors in the American National Adult Reading test (e-AMNART) [45] were used as an estimate of cognitive reserve, which has shown to have greater variation than education [42]. However, AMNART scores are affected with the progression of cognitive decline [53], therefore a correction of the score was used linearly regressing e-AMNART (re-AMNART) on MMSE score [2].
Statistics
To study the effect of different factors on plasma Aβ1–42, Aβ1–40 and AβR, continuous variables were classified in quartiles except bilirubin, creatinine and liver enzyme levels, which were classified according to the laboratory reference levels as normal or abnormal. For comparisons of normally distributed numerical variables, a t test or ANOVA was used. In the absence of a normal distribution, log transformation was applied (individual Aβ measures were normally distributed but the ratio was log transformed), but if this failed to normalize the data, the non-parametric Kruskal–Wallis test was applied. Variables with a p value lower than 0.20 were selected to enter a forward stepwise selection multivariate regression model. For correlations between biomarker levels Pearson r coefficients were calculated (rP), but in the presence of bivariate outliers in the relplot representation [18] a percentage bend correlation (rPB) was used. For dependent categorical variables binary logistic regression models were applied. ROC curves and their respective area under the curve (AUC) were estimated using values predicted by the logistic regression. To assess MCI conversion to AD, Cox proportional hazards models were used. For repeated measures analysis of quantitative variables, mixed-effect models were applied. The obtained p values were corrected for multiple comparisons using Bonferroni’s method.
Z-scores were obtained calculating an α-winsorized mean and standard deviation (SD) (α = 0.10). We obtained an averaged z-score for five cognitive domains (learning and delayed verbal memory, language, executive function and processing) using the baseline scores of the 167 subjects who continued to be classified as CN at the third year follow-up, and a global composite cognitive measure was obtained averaging these domains. The mean and SD for Aβ plasma levels were obtained using the baseline values of CN subjects with normal CSF signature. A change in Aβ measures was classified as a decrease or increase if at 36 months there was a decrease greater than 0.5 SD or an increase greater than 0.5 SD. Statistical analyses were performed using SPSS 19.0 and R 2.12.1 [40, 58].
Results
We included 715 subjects who had a baseline Aβ measurement, whose follow-up information is presented in Fig. 1 and whose characteristics are summarized in Table 1.
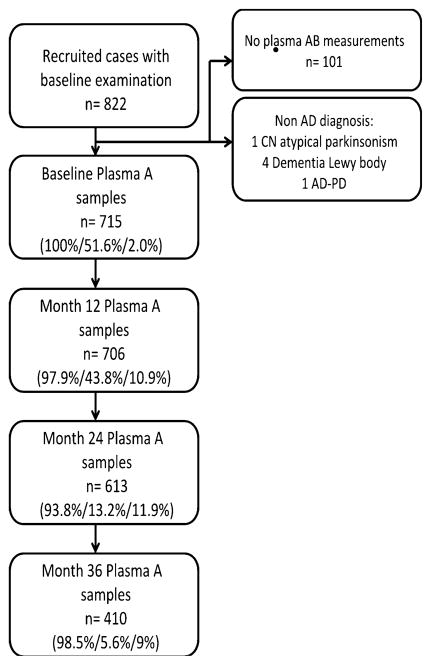
Flow chart with follow-up data of the cohort. The percentages in brackets represent proportion of subjects with plasma Aβ, CSF Aβ and PIB measures at each follow-up, respectively. Aβ amyloid beta, AD Alzheimer’s disease, CN cognitively normal, PD Parkinson’s disease
Table 1
Demographics, baseline cognitive measurements and blood analytes
CN stable (n = 187) | CN progression (n = 10) | CN dropouts (n = 8) | MCI stable (n = 162) | MCI progression (n = 145) | MCI dropouts (n = 41) | AD (n = 162) | p value | |
---|---|---|---|---|---|---|---|---|
Age (years) | 75.80 (5.02) | 78.40 (4.06) | 75.63 (4.41) | 74.69 (7.57) | 74.61 (7.20) | 76.63 (7.26) | 75.27 (7.60) | 0.866a 0.106b 0.265c 0.723d |
Gender (% male) | 52.4 | 50.0 | 50.0 | 68.5 | 62.1 | 61.0 | 53.1 | |
Ethnicity (% caucasian) | 92.0 | 90.0 | 75.0 | 91.4 | 90.3 | 90.2 | 93.8 | |
Education (years) | 16.08 (2.81) | 16.10 (3.00) | 16.38 (2.07) | 15.98 (2.86) | 15.65 (2.96) | 15.22 (3.45) | 14.83 (3.06) | 0.973a 0.249b 0.990c 0.004d |
e-AMNART | 9.04 (8.18) | 8.70 (4.90) | 15.12 (9.58) | 13.05 (10.28) | 13.76 (9.56) | 14.76 (10.58) | 14.76 (10.58) | 0.033a 0.787b 0.026c < 0.003d |
MMSE | 29.17 (0.99) | 29.10 (0.74) | 28.25 (1.67) | 27.52 (1.71) | 26.72 (1.70) | 26.59 (1.73) | 23.44 (1.99) | 0.106a 0.060b 0.127c <0.001d |
ADAS-Cog | 5.74 (2.75) | 8.17 (3.43) | 8.75 (4.38) | 9.57 (4.19) | 13.14 (4.11) | 12.03 (4.03) | 18.31 (6.03) | 0.049a 0.084b 0.003c <0.001d |
BMI (kg/m2) | 26.73 (4.34) | 29.61 (5.07) | 25.14 (3.81) | 26.38 (3.95) | 25.84 (4.13) | 25.82 (3.54) | 25.67 (3.79) | 0.276a 0.650b 0.410c 0.030d |
Creatinine (mg/dL) | 0.96 (0.23) | 1.04 (0.34)) | 0.98 (0.21) | 1.00 (0.25) | 1.03 (0.27) | 0.93 (0.24) | 1.03 (0.264) | 0.794a 0.052b 0.399c 0.540d |
Cholesterol (mg/dL) | 192.72 | 176.22 (35.61) | 194.40 (23.46) | 195.31 (38.95) | 196.86 (38.45) | 201.93 (34.04) | 203.29 (42.26) | 0.758a 0.362b 0.430c 0.154d |
Platelets (cells/mm3) | 234,950 (61,850) | 231,000 (56,460) | 250,250 (40,294) | 227,900 (49.44) | 245,110 (70,523) | 241,720 (71,178) | 241,070 (64,549) | 0.356a 0.719b 0.648c 0.030d |
WMH (Volume %) | 2.65 (2.60) | 2.21 (1.67) | 2.87 (0.98) | 2.33 (1.87) | 2.80 (2.93) | 3.22 (2.63) | 3.35 (3.27) | 0.419a 0.094b 0.883c 0.006d |
Plasma Aβ40 (pg/ml) | 152.52 (49.45) | 145.53 (52.76) | 133.09 (25.50) | 153.70 (54.42) | 150.65 (57.15) | 143.35 (35.65) | 152.46 (46.80) | 0.281a 0.162b 0.301c 0.590d |
Plasma Aβ42 (pg/ml) | 38.14 (12.33) | 32.12 (10.78) | 36.56 (9.29) | 36.89 (12.85) | 35.72 (11.39) | 35.46 (9.45) | 36.41 (10.40) | 0.773a 0.657b 0.179c 0.597d |
Plasma Aβ42/40 ratio | 0.27 (0.08) | 0.23 (0.07) | 0.27 (0.03) | 0.26 (0.11) | 0.26 (0.11) | 0.25 (0.05) | 0.25 (0.06) | 0.547a 0.964b 0.462c 0.971d |
CSF Aβ42 (pg/ml) | 207.38 (53.73) | 172.67 (56.88) | 263.50 (7.78) | 175.92 (61.64) | 146.68 (40.04) | 180.32 (47.81) | 143.97 (42.02) | 0.175a 0.036b 0.490c 0.001d |
CSF TTau (pg/ml) | 69.51 (28.74) | 63.83 (31.20) | 65.00 (19.80) | 84.40 (46.57) | 113.72 (11.40) | 84.45 (57.03) | 119.66 (60.20) | 0.974a 0.748b 0.593c <0.001d |
Aβ amyloid beta, ADAS-Cog Alzheimer’s Disease Assessment Scale-cognitive subscale, BMI body mass index, CSF cerebrospinal fluid, e-AMNART errors in the American National Adult Reading test, MMSE mini-mental state examination, TTAU total tau, WMH White matter hyperintensitiy
Factors affecting Aβ plasma concentration
Increased concentrations of baseline plasma Aβ1–42 and Aβ1–40 were associated with increasing age, low levels of total proteins, decreasing platelet count, impaired kidney function and with gender (males > females), while Aβ1–40 levels decreased with increasing cholesterol levels. Aβ1–42 decreased with increasing copies of APOE4 allele. However, Aβ1–42/Aβ1–40 ratio values were only affected by glucose (Table 2).
Table 2
Factors affecting plasma AB measurements
Factor | Aβ1–40 | Aβ1–42 | AβR |
---|---|---|---|
p | p | p | |
Platelets | 0.001 | 0.006 | 0.419 |
Gender | 0.004 | 0.003 | 0.228 |
Ethnic | 0.558 | 0.595 | 0.954 |
Hematocrit | 0.573 | 0.256 | 0.509 |
Red blood cells | 0.402 | 0.633 | 0.372 |
White Blood cells | 0.510 | 0.885 | 0.314 |
Glucose | 0.053 | 0.159 | 0.030 |
Creatin kinase | 0.747 | 0.251 | 0.560 |
Cholesterol | 0.127 | 0.025 | 0.911 |
Bilirubin | 0.832 | 0.558 | 0.244 |
DirectBil | 0.637 | 0.567 | 0.332 |
GGT | 0.477 | 0.394 | 0.469 |
Creatinine | <0.0001 | <0.0001 | 0.059 |
GPT | 0.673 | 0.473 | 0.300 |
Total protein | 0.012 | <0.0001 | 0.652 |
Albumin | 0.307 | 0.155 | 0.851 |
Calcium | 0.819 | 0.861 | 0.641 |
Triglycerides | 0.445 | 0.866 | 0.187 |
APOE4a | 0.093 | 0.006 | 0.169 |
Age | <0.0001 | <0.0001 | 0.419 |
BMI | 0.859 | 0.239 | 0.492 |
p values are not corrected for multiple comparisons (see methods)
BMI body mass index, GGT gamma glutamyl transpeptidase, GPT alanine transaminase
Age, platelet count, total protein and creatinine concentration were independent predictors for Aβ1–40 and Aβ1–42 and explained 12.1 and 12.9% of the variability of their respective concentrations. These variables were included in the multivariate models to adjust for possible confounders.
Aβ levels measured in plasma and CSF and the PIB Aβ burden in brain
Correlation between Aβ1–40 and Aβ1–42 plasma concentrations was 0.834 (p < 0.001), while the correlation between Aβ1–42 in plasma and CSF in the 368 available subjects was low (rPB = 0.155, p = 0.017). Adjusting for factors affecting Aβ1–42 plasma levels did not improve the ability to predict CSF Aβ1–42 values. There was a mild inverse correlation between Aβ1–42 plasma and CSF tau (rPB = −0.143, p = 0.037) and p-taup181 (rPB = −0.171, p = 0.006) (Supplementary Fig. 1). Similar results were obtained for the following groups: CN and MCI with normal CSF and MCI stable, MCI progressors and AD with pathological CSF.
Ninety-five subjects had at least one PIB and plasma Aβ measures and 44 subjects had Aβ1–42 CSF and PIB measures obtained at the same follow-up visit. Mean PIB values showed a high inverse correlation with CSF Aβ1–42 levels (rP = −0.759, p < 0.001), a mild inverse correlation with Aβ1–42 plasma levels (rPB = −0.234, p = 0.044) and no correlation with plasma Aβ1–40 (Fig. 2).
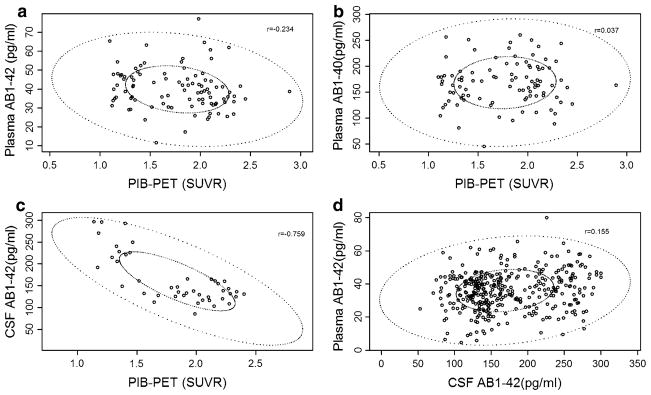
Relplots of the biomarker data (The small ellipse represents the bivariate interquartile range and the outer ellipse delimits the data points not classified as outliers). a PIB PET and plasma Aβ1–42. b PIB PET and plasma Aβ1–40. c PIB PET and cerebrospinal Aβ1–42. d Plasma Aβ1–42 and cerebrospinal Aβ1–42. Aβ amyloid beta, PIB-PET Pittsburgh Compound B positron emission tomography, SUVR standardized uptake value ratio
Aβ plasma levels, vascular risk factors and WMHV
There was no correlation between WMHV and plasma Aβ1–42 (rPB = 0.069, p = 0.17) and Aβ1–40 (rPB = 0.083, p = 0.82). We adjusted in a multiple regression model with a two-category variable that divided the population into CN and MCI subjects with normal CSF tau and Aβ signature and MCI and AD with the pathological CSF tau and Aβ signature and the factors that affected plasma levels. In this model, the partial correlation value of Aβ1–42 was 0.129 with a p value of 0.058 while there was no interaction between the diagnostic groups and plasma Aβ1–42.
Subjects with infarctions revealed by MRI had higher plasma Aβ1–42 levels [p = 0.024, mean Aβ1–42 difference 7.10 pg/ml (95%CI 1.90–12.29)] in the model adjusted for factors that affected plasma Aβ1–42 concentration. There was no correlation between the three plasma Aβ measurements and the studied vascular risk factors (BMI, systolic and diastolic blood pressure). Plasma Aβ1–42 levels, adjusted for age and BMI, predicted if a subject was or was not hypertensive (p = 0.03), however the AUC of the model was 0.639.
Baseline plasma Aβ levels, changes in cognitive status and CSF tau and Aβ levels
There were no differences in plasma Aβ measures comparing CN stable, MCI stable, MCI progressors and AD. We then compared in a two factor ANOVA, adjusting for age groups (split by median age, 75 years) and the following diagnostic categories based on CSF signature: CN stable with normal CSF (n = 71), MCI stable with normal (n = 42) and pathological CSF (n = 32), and MCI progressors (n = 58) and AD cases with pathological CSF (n = 86). In this model, there were no differences based on diagnostic categories (p = 0.333) but the term for age (p = 0.003) as well as the interaction between diagnostic category and age (p = 0.018) was significant in the ANOVA studying Aβ1–42 (Fig. 3). The latter was only significant in the groups with pathological CSF signature. The results for Aβ1–40 were similar, with an interaction between age and diagnostic group (p = 0.015), that was only significant in both MCI groups with a pathological CSF signature. When studying AβR, there was neither a difference between the diagnostic groups (p = 0.234), nor an interaction between the diagnostic groups and age groups (p = 0.249).
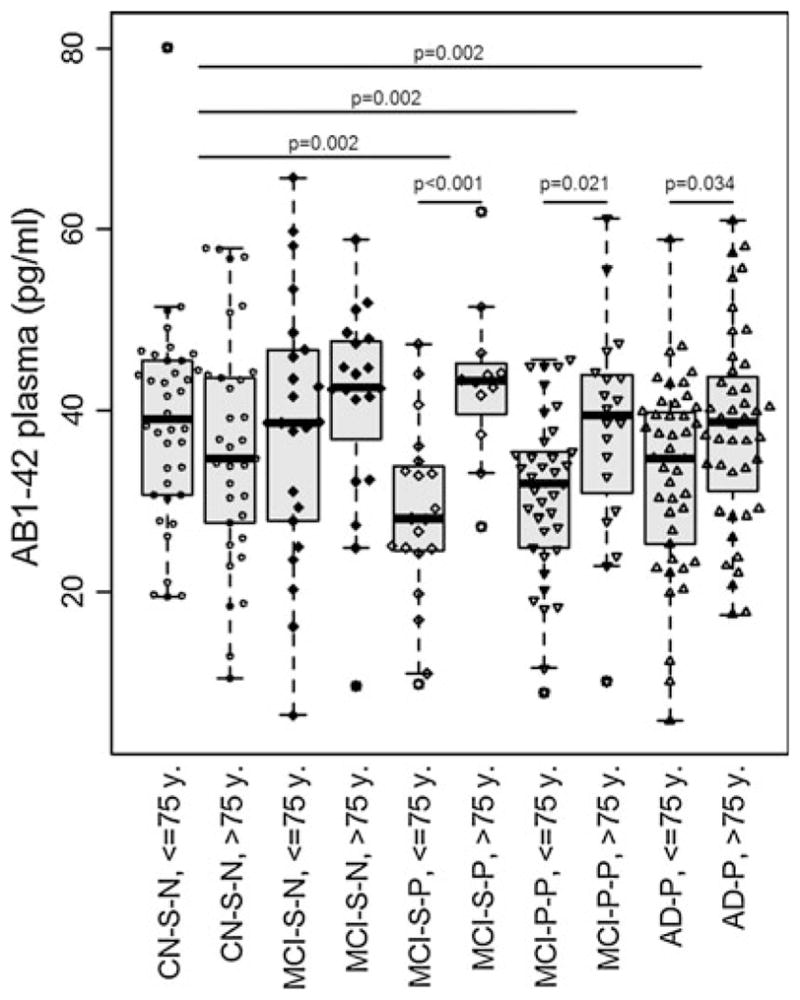
Amyloid beta (Aβ) 1–42 levels in the different cognitive groups stratified by CSF signature and age. Aβ1–42 levels in cognitively normal (CN) stable, mild cognitive impairment (MCI) stable and progressors and Alzheimer’s disease (AD) subjects, stratified by normal and pathological cerebrospinal fluid signature (CSF). There was an interaction between age and diagnostic group in the three groups with pathological CSF signature: MCI stables (p < 0.001), MCI progressors (p = 0.021) and AD (p = 0.034). Only the groups with younger subjects and pathological CSF had a difference in Aβ1–42 levels than the pooled cognitively normals with normal CSF signature
Longitudinal analysis of Aβ plasma levels
For the analysis of the temporal profile of changes in Aβ plasma levels, we studied the following groups: CN stable subjects with normal CSF (n = 71), MCI stable subjects (n = 31) and MCI progressors with pathological CSF (n = 35). In the mixed-effect models studying Aβ1–40 and Aβ1–42 plasma measures there was a statistically significant effect of time, with values increasing in the successive follow-ups and a difference between groups in the Aβ1–42 model (Fig. 4). The AβR decreased along time and there was no difference between diagnostic groups. AD subjects could only be studied up to 2-year follow-up. There was no difference between baseline and 24-month follow-up in the AD group.
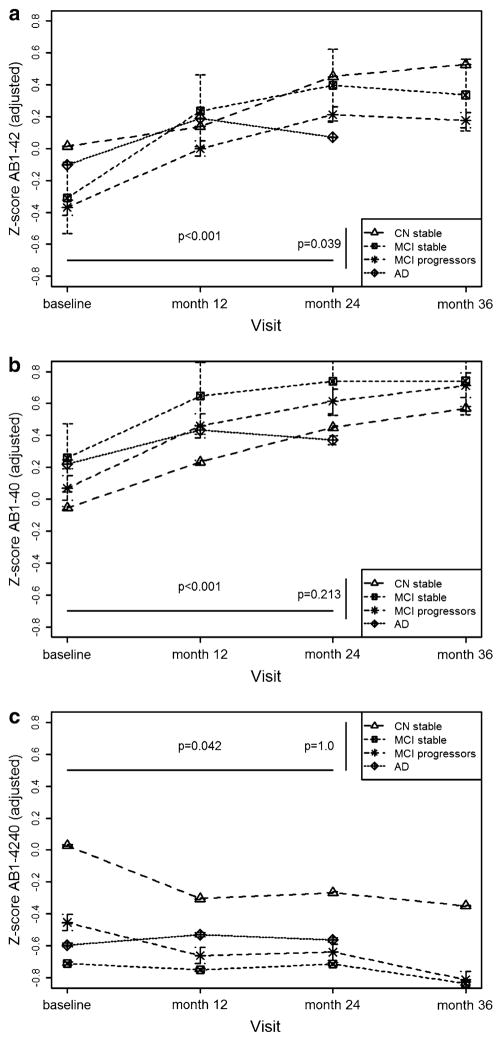
Amyloid beta (Aβ) plasma biomarker changes along time in cognitive normal subjects with normal cerebrospinal fluid (CSF), mild cognitive impairment stable and progressor subjects with pathological CSF and Alzheimer’s disease (AD) subjects with pathological CSF with 95% CI based on SE. All the models were adjusted for age and took into consideration the interaction between age and diagnostic category. a Aβ1–42 along time: There is an increase in the concentration across time (p <0.001), except in AD cases (p = 0.067), and mild cognitive impairment (MCI) progressors have lower levels than MCI stables and cognitive normals (CN) (p = 0.039). b Aβ1–40 along time: There is an increase in the concentration across time (p < 0.001), except in AD cases (p = 0.372), and no difference between diagnostic groups (p = 0.213). c AβR along time: There was a decrease of the ratio across time (p = 0.042) except in AD cases (p = 0.902), but no difference between diagnostic groups (p = 1.0). Aβ amyloid beta
Utility of Aβ plasma measure to predict cognitive decline
We calculated tertiles of baseline Aβ plasma measures in the CN group. None of the three plasma Aβ measurements classified according to the previously described tertiles showed an increased risk for conversion from MCI to AD in the adjusted Cox proportional hazards models (pAβ1–40 = 0.633, pAβ1–42 = 1.0, pAβR = 1.0). On the other hand, a pathological CSF signature heralded a higher risk of conversion [HR 2.12 (95%CI 1.20–3.75)], as previously reported. The percentage of dropouts with a follow-up of 36 months was 23.6%. Results were similar with a follow-up of 24 months and 13.8% of dropouts.
We further studied the cognitive evolution of our sample in a mixed-effect model that included age at baseline, re-AMNART, the different annual visits, Aβ tertiles at baseline and the following diagnostic categories: CN stable with normal CSF and MCI stable and MCI progressors with a pathological CSF signature. None of the Aβ plasma measures had a statistical effect on change in the composite cognitive measure. However, there was a significant interaction between re-AMNART and Aβ1–42 tertiles (Fig. 5). When analyzing the different cognitive domains, the interaction was present in the language and executive domains (Table 3). Subjects with highest re-AMNART and in the lowest Aβ42 tertile had worse cognitive performance than those in the highest Aβ42 tertile and highest re-AMNART. The interaction was not significant when using the years of education. But education did not predict cognitive scores in two of the models and the association was milder in the model with general cognition (p = 0.031).

Changes in global cognitive score according to errors in the in American National Adult Reading test and amyloid beta (Aβ) measures, with 95% CI based on SE. a Aβ1–40 baseline level tertiles. b Aβ1–42 baseline level tertiles. c AβR baseline level tertiles. d Change in Aβ1–40 measures at 36 months. e Change in Aβ1–42 measures at 36 months. f Change in AβR measures at 36 months. Aβ amyloid beta
Table 3
Mixed effect models for cognitive outcome measures
Outcome and baseline BM | Age | Tertile Aβ | Diagnostic evolution | Visit | re-AMNART | Interaction re-AMNART and Tertile Aβ | Interaction diagnostic evolution and visit | Interaction tertile Aβ and visit |
---|---|---|---|---|---|---|---|---|
GC (Aβ1–42) | 0.264 | 0.501 | < 0.0001 | < 0.0001 | 0.005 | 0.001 | < 0.0001 | 0.346 |
GC (Aβ1–40) | 0.207 | 0.891 | < 0.0001 | < 0.0001 | 0.006 | 0.314 | < 0.0001 | 0.348 |
GC (AβR) | 0.078 | 0.753 | < 0.0001 | < 0.0001 | 0.075 | 0.753 | < 0.0001 | 0.467 |
Language (Aβ1–42) | 0.380 | 0.263 | < 0.0001 | < 0.0001 | 0.005 | 0.0002 | < 0.0001 | 0.778 |
Executive (Aβ1–42) | 0.161 | 0.163 | < 0.0001 | < 0.0001 | 0.003 | 0.013 | < 0.0001 | 0.129 |
Outcome and change in BM | Age | Change Aβ | Diagnostic evolution | Visit | re-AMNART | Interaction re-AMNART and Change Aβ | Interaction diagnostic evolution and Visit | Interaction change Aβ and visit |
---|---|---|---|---|---|---|---|---|
GC (Aβ1–42) | 0.043 | 0.676 | < 0.0001 | < 0.0001 | 0.246 | 0.987 | < 0.0001 | 0.577 |
GC (Aβ1–40) | 0.017 | 0.054 | < 0.0001 | < 0.0001 | 0.087 | 0.044 | < 0.0001 | 0.874 |
GC (AβR) | 0.070 | 0.044 | < 0.0001 | < 0.0001 | 0.042 | 0.0.65 | < 0.0001 | 0.505 |
p values are not corrected for multiple comparisons
GC global cognitive Z score, Aβ amyloid beta, re-AMNART regressed errors on the American National Adult Reading test
We then studied the previous statistically significant models in the whole sample without classifying subjects according to their CSF signature. There was no effect on Aβ1–42 tertiles or an interaction of Aβ1–42 tertiles and re-AMNART in any of the models.
Finally, in a mixed-effect model with CN stable (n = 168) and all MCI (n = 276) subjects, there was no interaction between re-AMNART and the Aβ categories, but patients who had an increase in AβR (p = 0.027) or a decrease of Aβ1–40 (p = 0.045) had worse performance on the composite cognitive measure, without a significant effect of the change of Aβ1–42 (p = 0.778; Fig. 5).
Discussion
In our study we confirmed the poor performance of measuring plasma Aβ levels for classification at different stages of AD in cross-sectional analyses, but we found a different trends in the repeated plasma measurements with levels being stable in the AD group. Thus, it is possible that longitudinal measures of plasma Aβ could be informative as a biomarker for the response to Aβ therapies or as an indicator of increased risk for conversion from normal or MCI to AD. We also quantified systemic factors that account for 12–13% of the variability of plasma levels. Finally, we confirmed the interaction between cognitive reserve and Aβ plasma measurements, finding an effect in the executive and language domains, and described a new association with infarctions.
The results of this study show that patients with infarctions documented by MRI have higher Aβ1–42 levels than subjects without such lesions, and there was a trend for an association between WMHV and Aβ1–42 plasma. In addition to the well-described association between renal function and plasma Aβ [3, 36], we also found that total proteins and platelets affected plasma Aβ levels as suggested earlier for blood levels [9]. Plasma Aβ1–42 and Aβ1–40 concentrations were associated with aging, but this association was only present in MCI subjects with a pathological CSF signature who showed increasing levels with increasing age. When repeated measures of plasma Aβ1–40 and Aβ1–42 were analyzed, there was an increase in both biomarker concentrations with time in the three studied groups although levels were lower in MCI patients with a pathological CSF signature. AβR decreased with time and the ratio was lower in MCI patients with pathological CSF. Concentration did not change in the AD group which would favor the hypothesis that levels stay stable or decrease in advanced stages of the disease. We found that in early stages a decrease in Aβ1–40 and an increase in AβR were associated with a worse cognitive performance. Subjects with lower cognitive reserve, who had lower Aβ1–42 plasma concentrations at baseline scored worse on cognitive testing, indicating that they may only exert a mild effect that is counteracted by cognitive reserve as reported recently [59]. In our study, we had a cognitive battery which allowed us to study different domains, finding that the effects were driven by the executive and language domain. Results for years of education were negative. We think that this is due to the skewed representation of mainly highly educated subjects that precludes a wide range and normal distribution of values. Aβ plasma levels had a better correlation with Aβ amyloid brain deposits than with CSF Aβ values across all the studied groups, thereby confirming previous results [12, 13, 34]. We did not find a significant association between vascular risk factors and Aβ plasma levels.
Our results are consistent with the idea that the conflicting results of previous studies can, in part, be caused by age and disease stage effects on plasma Aβ concentrations as these factors differed across the studies. Our data also underline the importance of having well-defined subjects and the use of biomarkers that recognize the underlying pathology in AD (i.e. CSF tau and Aβ biomarkers) to evaluate the utility of other potential AD biomarkers (i.e. plasma Aβ) in the absence of neuropathological diagnosis. Our results show that given our current understanding Aβ plasma levels, they are not useful for cross-sectional classification purposes in agreement with a recent metanalysis [51]. Although our study did not find a prognostic utility studies with longer follow-up periods and their joined results have shown predictive value [15, 46, 51]. Thus, it is plausible that measures of plasma Aβ within subjects over periods of greater duration could become simple and minimally invasive screens to identify individuals at increased risk for AD dementia. Notably, our study provides insight into factors that affect Aβ plasma levels and better understanding of these factors will be critical if we are to be able to exploit measures of plasma Aβ as a screening assay along the lines described above. Indeed plasma Aβ levels increase with aging, but changes in plasma Aβ are more stable as AD progresses to more advanced stages of disease.
One of the major strengths of our study is that it combines imaging, CSF and plasma Aβ data from the same cohort, i.e. ADNI subjects, and our study included an analysis of the data according to underlying AD neuropathology (assessed with CSF tau and Aβ signature of AD) with a comprehensive neuropsychological battery to assess the effects of plasma levels of Aβ on different cognitive domains.
Our study also has some weaknesses. The inclusion criteria excluded subjects with important vascular pathology, so our results on vascular risk factors and vascular dementia are to be taken cautiously. The selection criteria used in the ADNI study were designed to enroll late MCI subjects, and this has been confirmed by the high progression rate to AD, and therefore we may not have captured important plasma Aβ changes that could have occurred in earlier stages of the disease. Last, the follow-up and biomarker sampling were not complete in AD cases and we did not have available data for the whole cohort which decreases the statistical power. These weaknesses notwithstanding, our study advances understanding of the significance of plasma Aβ measurements in normal aging, MCI and AD.
Acknowledgments
We thank our ADNI colleagues for their contributions to the work summarized here which has been supported mainly by the ADNI U01 AG024904. ADNI is funded by the National Institute of Aging, the National Institute of Biomedical Imaging and Bioengineering (NIBIB), and the Foundation for the National Institutes of Health, through generous contributions from the following companies and organizations: Pfizer Inc., Wyeth Research, Bristol-Myers Squibb, Eli Lilly and Company, GlaxoSmithKline, Merck & Co. Inc., AstraZeneca AB, Novartis Pharmaceuticals Corporation, the Alzheimer’s Association, Eisai Global Clinical Development, Elan Corporation plc, Forest Laboratories, and the Institute for the Study of Aging (ISOA), with participation from the U.S. Food and Drug Administration. Other support has come from AG10124 and the Marian S. Ware Alzheimer Program. VMYL is the John H. Ware 3rd Professor for Alzheimer’s Disease Research and JQT is the William Maul Measy-Truman G. Schnabel Jr. M.D. Professor of Geriatric Medicine and Gerontology. We thank the ADNI Biomarker Core for the analyses. We thank Donald Baldwin and the Molecular Diagnosis Genotyping Facility at the University of Pennsylvania Medical Center for provision of the APOε genotyping data. J.B.T.’s work was supported by a grant from the Alfonso Martín Escudero foundation. L.S.’s, C.J.’s and M.W.’s work is partially supported by NIH.
Footnotes
For the Alzheimer’s Disease Neuroimaging Initiative: Data used here were produced by the ADNI Biomarker Core or obtained from the ADNI database (www.loni.ucla.edu/ADNI). Many ADNI investigators contributed to ADNI but did not participate in the analysis of the data presented here or in the writing of this report. ADNI investigators are listed at www.loni.ucla.edu\ADNI\Collaboration\ADNI_Manuscript_Citations.pdf).
Electronic supplementary material The online version of this article (10.1007/s00401-011-0861-8) contains supplementary material, which is available to authorized users.
Conflict of interest J.Q.T., V.M.Y.L., A.W.T., S.X.X., E.T., M.F., M.W., C.J., P.A., W.J. and J.B.T. have no conflicts of interest. L.S. belongs to the advisory board of Innogenetics Technical. H.V. works at INNX-Fujirebio.
Contributor Information
Jon B. Toledo, Department of Pathology and Laboratory Medicine, Institute on Aging, Center for Neurodegenerative Disease Research, University of Pennsylvania School of Medicine, HUP, Maloney, 3rd (JQT) or 7th (LMS) Floor, 36th and Spruce Streets, Philadelphia, PA 19104-4283, USA.
Hugo Vanderstichele, Department of Diagnostic Development, Innogenetics NV, Ghent, Belgium.
Michal Figurski, Department of Pathology and Laboratory Medicine, Institute on Aging, Center for Neurodegenerative Disease Research, University of Pennsylvania School of Medicine, HUP, Maloney, 3rd (JQT) or 7th (LMS) Floor, 36th and Spruce Streets, Philadelphia, PA 19104-4283, USA.
Paul S. Aisen, University of California at San Diego, San Diego, CA 92093, USA.
Ronald C. Petersen, Mayo Clinic College of Medicine, Rochester, MN 55905, USA.
Michael W. Weiner, Department of Radiology Medicine and Psychiatry, University of California at San Francisco, San Francisco, CA, USA.
Clifford R. Jack, Jr, Mayo Clinic, Rochester, MN, USA.
William Jagust, Helen Wills Neuroscience Institute University of California, Berkeley, CA, USA.
Charles Decarli, Department of Neurology, University of California, Sacramento, CA, USA.
Arthur W. Toga, Laboratory of Neuro Imaging, Department of Neurology, University of California at Los Angeles School of Medicine, Los Angeles, CA, USA.
Estefanía Toledo, Department of Preventive Medicine and Public Health, Medical School, Clinica Universidad de Navarra, University of Navarra, Pamplona, Spain.
Sharon X. Xie, Department of Biostatistics and Epidemiology, University of Pennsylvania School of Medicine, Philadelphia, PA 19104, USA.
Virginia M.-Y. Lee, Department of Pathology and Laboratory Medicine, Institute on Aging, Center for Neurodegenerative Disease Research, University of Pennsylvania School of Medicine, HUP, Maloney, 3rd (JQT) or 7th (LMS) Floor, 36th and Spruce Streets, Philadelphia, PA 19104-4283, USA.
John Q. Trojanowski, Department of Pathology and Laboratory Medicine, Institute on Aging, Center for Neurodegenerative Disease Research, University of Pennsylvania School of Medicine, HUP, Maloney, 3rd (JQT) or 7th (LMS) Floor, 36th and Spruce Streets, Philadelphia, PA 19104-4283, USA.
Leslie M. Shaw, Department of Pathology and Laboratory Medicine, Institute on Aging, Center for Neurodegenerative Disease Research, University of Pennsylvania School of Medicine, HUP, Maloney, 3rd (JQT) or 7th (LMS) Floor, 36th and Spruce Streets, Philadelphia, PA 19104-4283, USA.
References
Full text links
Read article at publisher's site: https://doi.org/10.1007/s00401-011-0861-8
Read article for free, from open access legal sources, via Unpaywall:
https://europepmc.org/articles/pmc3299300?pdf=render
Citations & impact
Impact metrics
Citations of article over time
Alternative metrics
Article citations
Clearance and transport of amyloid β by peripheral monocytes correlate with Alzheimer's disease progression.
Nat Commun, 15(1):7998, 12 Sep 2024
Cited by: 1 article | PMID: 39266542 | PMCID: PMC11393069
Research Progress on the Pathogenesis, Diagnosis, and Drug Therapy of Alzheimer's Disease.
Brain Sci, 14(6):590, 09 Jun 2024
Cited by: 1 article | PMID: 38928590
Review
Associations among plasma, MRI, and amyloid PET biomarkers of Alzheimer's disease and related dementias and the impact of health-related comorbidities in a community-dwelling cohort.
Alzheimers Dement, 20(6):4159-4173, 15 May 2024
Cited by: 1 article | PMID: 38747525 | PMCID: PMC11180870
Alzheimer blood biomarkers: practical guidelines for study design, sample collection, processing, biobanking, measurement and result reporting.
Mol Neurodegener, 19(1):40, 15 May 2024
Cited by: 5 articles | PMID: 38750570 | PMCID: PMC11095038
Review Free full text in Europe PMC
Biomarkers associated with the pathogenesis of Alzheimer's disease.
Front Cell Neurosci, 17:1279046, 07 Dec 2023
Cited by: 4 articles | PMID: 38130871 | PMCID: PMC10733517
Review Free full text in Europe PMC
Go to all (115) article citations
Data
Data behind the article
This data has been text mined from the article, or deposited into data resources.
BioStudies: supplemental material and supporting data
Similar Articles
To arrive at the top five similar articles we use a word-weighted algorithm to compare words from the Title and Abstract of each citation.
Oligomeric forms of amyloid-β protein in plasma as a potential blood-based biomarker for Alzheimer's disease.
Alzheimers Res Ther, 9(1):98, 15 Dec 2017
Cited by: 78 articles | PMID: 29246249 | PMCID: PMC5732503
Plasma Aβ42/40 Ratio Detects Early Stages of Alzheimer's Disease and Correlates with CSF and Neuroimaging Biomarkers in the AB255 Study.
J Prev Alzheimers Dis, 6(1):34-41, 01 Jan 2019
Cited by: 44 articles | PMID: 30569084
Association Between Longitudinal Plasma Neurofilament Light and Neurodegeneration in Patients With Alzheimer Disease.
JAMA Neurol, 76(7):791-799, 01 Jul 2019
Cited by: 315 articles | PMID: 31009028 | PMCID: PMC6583067
The Alzheimer's Disease Neuroimaging Initiative: a review of papers published since its inception.
Alzheimers Dement, 8(1 suppl):S1-68, 02 Nov 2011
Cited by: 265 articles | PMID: 22047634 | PMCID: PMC3329969
Review Free full text in Europe PMC
Funding
Funders who supported this work.
NIA NIH HHS (6)
Grant ID: P30 AG010124
Grant ID: U01 AG024904-07
Grant ID: P30 AG010124-19
Grant ID: AG10124
Grant ID: U01 AG024904
Grant ID: U19 AG010483