Abstract
Free full text

Genome-Wide Methylation Analysis Identifies Genes Specific to Breast Cancer Hormone Receptor Status and Risk of Recurrence
Abstract
To better understand the biology of hormone receptor-positive and negative breast cancer and to identify methylated gene markers of disease progression, we performed a genome-wide methylation array analysis on 103 primary invasive breast cancers and 21 normal breast samples using the Illumina Infinium HumanMethylation27 array that queried 27,578 CpG loci. Estrogen and/or progesterone receptor-positive tumors displayed more hypermethylated loci than ER-negative tumors. However, the hypermethylated loci in ER-negative tumors were clustered closer to the transcriptional start site compared to ER-positive tumors. An ER-classifier set of CpG loci was identified, which independently partitioned primary tumors into ER-subtypes. Forty (32 novel, 8 previously known) CpG loci showed differential methylation specific to either ER-positive or ER-negative tumors. Each of the 40 ER-subtype-specific loci was validated in silico using an independent, publicly available methylome dataset from The Cancer Genome Atlas (TCGA). In addition, we identified 100 methylated CpG loci that were significantly associated with disease progression; the majority of these loci were informative particularly in ER-negative breast cancer. Overall, the set was highly enriched in homeobox containing genes. This pilot study demonstrates the robustness of the breast cancer methylome and illustrates its potential to stratify and reveal biological differences between ER-subtypes of breast cancer. Further, it defines candidate ER-specific markers and identifies potential markers predictive of outcome within ER subgroups.
Introduction
Approximately 200,000 women are diagnosed each year in the US with breast cancer, and nearly 50,000 die of their metastatic disease. Significant improvement made in both early detection and local/systemic therapy in the last few decades has significantly improved patient outcomes, especially survival. Breast cancers are characterized by their estrogen and progesterone receptor status (hereon termed ER), and it is established that ER expression (ER-positive) identifies a tumor phenotype with improved near/mid-term prognosis and likely benefit from adjuvant endocrine therapy when compared to ER-negative tumors. Yet, little is known about the genomic features within each ER-subtype of breast cancer that could explain why some patients with the same ER status have a good outcome while others do poorly regardless of treatment.
Current decision algorithms based on standard clinicopathologic factors (1) stratify ER-negative disease as having a high-risk for recurrence. (2-4) Although patients are now routinely offered adjuvant chemotherapy, most patients with node-negative, ER-negative disease remain disease-free after local therapy alone, including approximately 80% of ER-negative patients with tumors ≤ 1 cm (5) and up to 60% of all with stage 1 disease. (6) Consequently, there are patients with ER-negative disease that might do well without adjuvant chemotherapy and could avoid its potential toxicities, while others with a high residual risk despite it might be offered trials of novel therapies. Unfortunately, existing markers routinely used in clinical practice are of limited or no use in ER-negative patients (7). For example, commonly used gene expression tests by RT-PCR have no clear prognostic/predictive utility in ER-negative disease (8, 9) and microarray assays developed so far appear to identify essentially all such patients as high risk (10, 11), while other markers are still in development. Consequently, there is a critical need to develop better prognostic factors to improve assessment of residual risk and better predictive markers to optimize patient selection for standard and investigational systemic therapies.
Established clinically-annotated tissue banks from prospective randomized clinical trials and extensive databases on expression profiling in breast cancer in the last decade have allowed the prospective-retrospective development of the several prognostic and predictive tests. For instance, clinicians have come to accept foregoing adjuvant chemotherapy in patients with ER-positive, node-negative disease with a low-risk risk of distant recurrence of <10% at 10 years according to the 21-gene expression profile Oncotype DX assay, while strongly recommending it in those with a residual risk > 20% despite 5 years of adjuvant tamoxifen (9). Similarly useful tests are urgently needed for ER-negative breast cancer.
Multiple published studies using candidate gene approaches have suggested the utility of analyzing genes that undergo tumor-specific and promoter-specific hypermethylation as biomarkers for early detection and for prediction of outcome in multiple types of cancer (reviewed in 12). Methylated genes are particularly robust as biomarkers. In past studies, we developed a cancer detection panel using a quantitative cumulative methylation assay (QM-MSP) wherein the methylation status of multiple genes could be determined individually and cumulatively from picograms of input DNA, such as is retrieved from ductal lavage or ductoscopy (13, 14) and pathologic nipple discharge fluid (15). We and others have found that methylated genes are frequently detected in the pre-invasive stage of DCIS (16-18). Further, histopathologically normal ducts in the vicinity of tumor tissue display detectable hypermethylation of genes that are present in the adjacent DCIS or invasive cancer, while normal ducts present farther away do not (18-21). However, using the candidate marker approach it has been difficult to identify markers informative of the biology specifically of ER-positive or negative breast cancer or those that predict response to therapy, disease progression and survival. Therefore, we tested whether a genome-wide discovery platform would identify gene loci in tumors that better predict clinical outcomes (22-26).
As the first step towards studies with clinical trial samples, we performed methylation array analyses on a discovery set of 103 primary invasive tumors and 21 normal samples. We found that distinctly different gene CpG loci typify the methylome of ER-positive and ER-negative breast cancers. Forty gene loci were identified that stratified tumors according to ER-status. We also identified a putative “prognostic signature” of 100 CpG loci that are individually and collectively associated with outcome in patients with breast cancer. This feasibility study demonstrates that CpG locus methylation levels could reveal important biological differences in the epigenome between breast cancer subtypes and provide ancillary clinical diagnostic, prognostic, and predictive tools.
Materials and Methods
Tissues
Frozen breast cancer tissues that were excised from patients with Stage 1-3 disease prior to treatment (n=103) were retrieved from Surgical Pathology at Johns Hopkins Hospital (Baltimore, Maryland) and confirmed to contain > 50% epithelial cells. Normal breast organoids were prepared by enzymatic digestion of reduction mammoplasty specimens (n=15; median patient age = 52 years, range 47 to 71). Normal ducts from breast tissue > 2 cm away from the tumor (n=6) were isolated from cryosections using laser-capture micro-dissection (PALM MicroBeam, Carl Zeiss Microimaging, North America). The studies were done with institutional review board approval. Tumor characteristics are provided in Table 1 and Supplemental Table 1.
Table 1
Characteristics* | ER+ (N=44) | ER- (N=38) |
---|---|---|
Recurrences | 7 | 11 |
| ||
DFS at 5yrs (Estimated by Kaplan-Meier) | 87% | 71% |
| ||
HER2+/# cases annotated | 4/20 | 10/25 |
| ||
Median % Ki67 | 20 | 50 |
| ||
AJCC Stage | ||
![]() | 7 | 4 |
![]() | 22 | 19 |
![]() | 15 | 15 |
| ||
Median Tumor size (mm) | 28 | 59 |
| ||
Having <1mm margin | 10 | 7 |
| ||
Therapy** | ||
![]() | 3 | 11 |
![]() | 0 | 2 |
![]() | 34 | 0 |
![]() | 21 | 27 |
A total of 21 additional samples were arrayed and used for ER classification, but not for outcome analyses. These 21 cases were excluded from the outcome analysis for the following reasons: Neoadjuvant treatment (n = 8); Samples obtained 6 months after the initial diagnosis (n=10); progression within 6 month after diagnosis (n=10).
Genomic DNA extraction, sodium bisulfite conversion and quality assurance
DNA extraction and quality assurance were performed as described previously (27, 28) and in Supplementary Materials and Methods (Supplemental Figure 1A).
Methylome analysis
Bisulfite-converted DNA was analyzed using Illumina Infinium Human Methylation27 BeadChip Kit (WG-311-1202) in the JHU DNA Microarray Core. Locus methylation was calculated as a β-value within GenomeStudio software, low to high ranging from 0-1, respectively.
Data analysis
Data was analyzed using GenomeStudio software (Illumina, Inc., San Diego, CA) and Bioconductor in R (http://www.bioconductor.org). Unsupervised cluster analysis was used to visualize and characterize broad methylation patterns in the data. All tests were two tailed and p values of <0.05 were considered significant. Cox regression and Kaplan-Meier plots were used to model associations between methylation levels and time to recurrence, with and without adjustment for relevant clinical covariates, and to identify potential predictive markers. Covariates used were patients' age at diagnosis, tumor grade, pathological T stage, lymph node status, estrogen receptor, progesterone receptor, type of primary surgery (with or without radiotherapy), and adjuvant therapy (chemotherapy and/or endocrine therapy).To identify methylated genes associated with ER status and their biology, a different approach was taken, emphasizing genes in which methylation changed dramatically between ER-positive and ER-negative samples. To achieve this, the initial selection was based on large fold changes. To evaluate the predictive capability of a panel of loci associated with ER status, we used independent samples to perform ROC analysis of a summary score of methylation derived as follows: 1) Methylation at each locus was standardized to have a common scale by subtracting the mean methylation level and dividing by the standard deviation for that locus, so that low methylation resulted in negative values, while high methylation gave positive values. 2) high methylation was associated with ER-positive status at some loci, and with ER-negative status at others, so standardized methylation scores for these latter loci were multiplied by -1, such that a high score uniformly indicated ER-positive samples; and 3) Genes were combined by averaging the standardized methylation scores for each patient, and the average score used in ROC analyses. The same procedure was used to summarize multi-locus homeobox panels associated with recurrence.
Validation in TCGA samples
To verify that patterns of methylation observed in association with ER status and risk of recurrence within the JHU cohort were characteristic of breast cancer, we downloaded and analyzed data publicly available from the Cancer Genome Atlas Project (TCGA, http://tcga-data.nci.nih.gov/). We selected TCGA to perform this analysis since Illumina Meth27K was used, enabling direct comparisons for the same 50 bp CpG locus probes. In total, 185 samples were available on the Illumina 27k Human Methylation platform, and 465 samples were available on the Agilent G4502A expression array. Time to recurrence was not available at the time of download, but time to death was obtained for 342 of the samples queried on expression array and 182 samples queried on methylation array. Probe level data (TCGA level 2) was obtained for the methylation platform while gene-level summaries (TCGA level 3) were used for RNA expression. Rank-based Spearman correlations were calculated between methylation and expression using the 182 samples. Each methylation probe was mapped to the nearest gene using the open source Illumina methylation platform annotation package available from Bioconductor (http://www.bioconductor.org/packages/2.6/data/annotation/html/IlluminaHumanMethylation27k.db.html), and correlations calculated for probes mapping to genes found on the expression array. Benjamini-Hochberg adjusted p-values are reported for each probe, alongside the correlation coefficient. Association between overall survival and methylation or expression was evaluated by Cox regression. The ability of molecular markers to predict ER status was measured by performing an ROC analysis using the methylation and expression levels of individual genes as predictors and reporting the area under the ROC curve. For expression, the ROC analysis was based on the expectation of an inverse relationship between methylation and expression, so that in some cases, where a significant, positive association is observed between the two platforms, the area under the ROC may be substantially less than 0.5.
Quantitative-Multiplex Methylation-Specific PCR (QM-MSP)
The two-step multiplexed methylation-specific PCR method was previously described (15, 27, 28). For AKR1B1 primers/probes see Supplemental Materials and Methods.
Results
Methylation Profiling of Primary Invasive Breast Cancer Tumors
Whole-genome methylation array analysis was performed using the Illumina Infinium HumanMethylation27 BeadChip with primary invasive carcinoma samples (n=103), samples from microdissected normal breast tissue distant from the primary tumor (n=6), and epithelium enriched organoids isolated from normal breast (n=15). The array quantifies the proportion of methylated cytosines (5mC) to total cytosines at each of 27,578 different CpG dinucleotides. The steps followed for our analysis is shown as a flowchart in Figure 1.
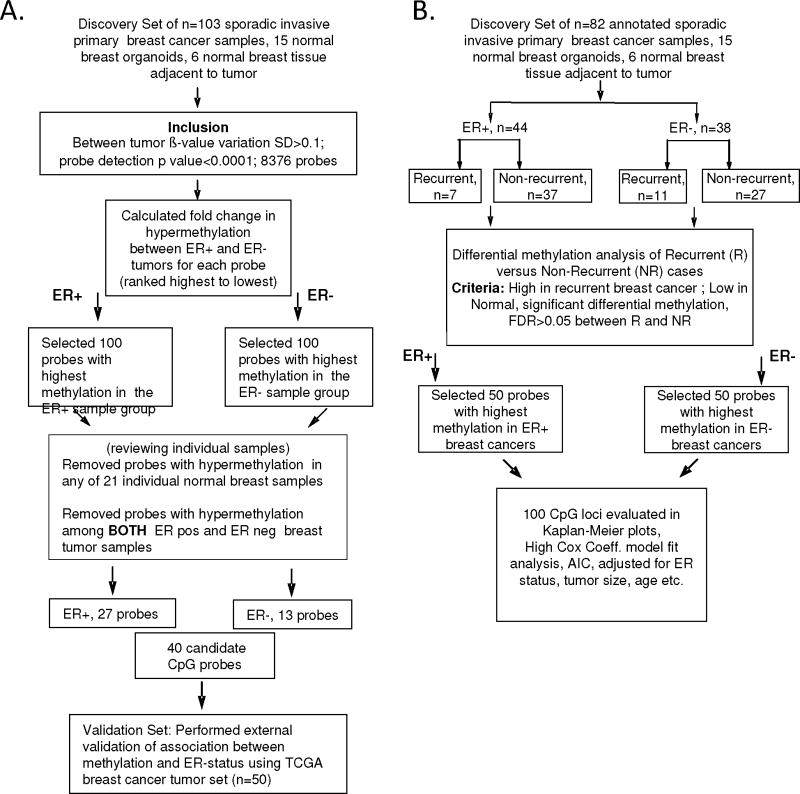
Schema outlining study design for analysis of association of methylation with (A) ER-status and (B) disease outcome.
To characterize the overall methylation profile of primary invasive breast tumors, unsupervised hierarchical cluster analysis using the Manhattan distance was performed on the most varied probes across tumors (1378 gene loci, SD>1.60) (Figure 2A). Two distinct clusters of tumors were observed. Cluster 1 was enriched for ER-positive breast cancer (21/28; 75%), while Cluster 2 contained 85% of the ER-negative tumors (41/75; 55% of total). Given the importance of ER in breast cancer, it is not surprising to observe a strong association between predominant methylation patterns and ER status (odds ratio = 3.57, 95% C.I = 1.27-11.20, p-value = 0.082), but the result also highlights the importance of gene methylation in the disease process. The data also suggested additional subgroups within Clusters 1 and 2 with distinct methylation profiles such as Cluster 2B, which contains all ER-PR+ tumor samples.
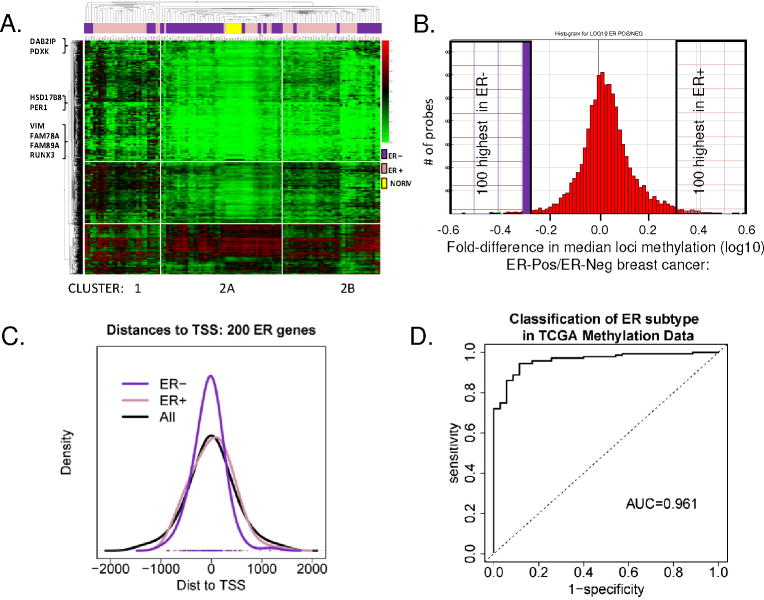
A. Unsupervised hierarchical cluster analysis of the most varied 5% of CpG loci probes among tumors (1378 loci; SD > 0.160). 2D-hierachial cluster analysis was performed using the Manhattan distance on 103 tumors, and 1378 loci showed two distinct clusters. Cluster 1 is enriched for ER+ tumors (pink bars); Regions of Cluster 2 appear enriched for ER-negative tumors (purple bars). Normal organoids cluster together (yellow bars). Examples of breast cancer related gene loci are indicated at left. B. Histogram plot showing the frequency of loci differentially methylated in ER-positive and ER-negative tumors. Plotted on the X-axis is the fold-difference in the mean locus methylation of ER-positive tumors/ER-negative tumors, among 8376 loci with (SD>0.1 across all tumors). The 200 (left and right shaded boxes) are CpG loci most differentially methylated between ER-positive and ER-negative tumors. C. Distance to the transcriptional start site (TSS) of the top CpG loci identified in ER-positive versus ER-negative tumors. Location of 100 ER-positive (pink line), 100-ER negative (purple line) and all 8376 (black line) CpG loci was plotted relative to the TSS indicated as 0 on the X axis. D. Validation of ER Subtype Markers. An ROC plot shows AUC 0.961 for detection of ER-subtype in 50 independent tumors within TCGA, using the ER-specific 40 loci marker set (data in Figure 3, Supp Table 2, Supp Fig 2).
Distinct groups of genes are specifically and recriprocally hypermethylated in ER-positive versus ER-negative breast cancer
Very little is known about the genomic features within each ER subtype of breast cancer that could explain why some patients have a good outcome while others will do poorly regardless of treatment. To determine the differences in breast cancer biology/behavior between ER subtypes, we characterized methylation patterns at 8376 selected CpG loci according to ER status. These loci met two criteria: 1) showed the most variation across primary tumors (SD >0.100) and 2) had probe detection p-values <0.0001 (indicating that DNA from that locus was present above background levels and that probe intensities were consistently measured across replicate beads; the distribution of methylation among these loci is shown in Supplemental Figure 1B). A substantial number of loci were observed with median methylation levels ≥ 0.15 in both groups of tumor and normal breast organoids. However, the majority of loci were more highly methylated in tumor than in normal organoid samples; 1744 loci in tumors had median methylation more than 2-fold higher compared to normal organoids (Supplemental Figure 1C).
ER-positive tumors were found to have a higher frequency of hypermethylated gene loci compared to ER-negative tumors (Figure 2B). Methylation at 5264 loci was higher (ratio >1) in ER-positive tumors samples, compared to methylation of 3112 loci (ratio <1) in ER-negative tumors. The top 100 hypermethylated CpG loci in each group of ER-positive and ER-negative tumors were selected (Figure 2B; ER-negative loci = ratio 0.52 − 0.15 and ER positive loci = ratio 3.98 − 2.23; Supplemental Table 2). Interestingly, ER-negative tumors had a higher number of hypermethylated loci located closer to the transcriptional start site (TSS), compared to ER-positive tumors, or to the 8376 array loci as a whole (Figure 2C). This finding suggested a more rigorous suppression of gene expression by methylation in the ER-negative subtype, since methylated regions overlapping the TSS have been shown to most tightly negatively regulate transcription.
To further refine this set to identify ER subtype-specific biological/molecular functions most driven by the epigenome in breast cancer, we selected a subgroup of 40 hypermethylated loci of the the 200 CpG locus set that individually showed the highest subtype specifity in individual tumor samples. Each individual locus was selected whose methylation profile demonstrated 1) robust reciprocal methylation between the two ER subtypes, 2) an incidence >20% of methylation within the breast cancer subtype and 3) low methylation in normal breast epithelium/stroma and leukocytes (β-value <0.15; Figure 3). Using these selection criteria, in the discovery set, we identified 27 loci/probes aberrantly and reciprocally hypermethylated in ER-positive tumors and 13 loci/probes aberrantly hypermethylated in ER-negative tumors. The majority of these were at loci newly identified as hypermethylated in breast cancer, and some never observed before as hypermethylated in cancer (Table 2). As shown in Figure 3A, Supp Figure 2 and Supp Table 3, ACADL, ADAMTSL1, ARFGAP3, B3GAT1, CDCA7, FAM78A, FAM89A, FLJ31951 (RNF145), FLJ34922 (SLFN11), GAS6, HAAO, HEY2, HOXB9, ITGA11, NETO, PROX1, PSAT1, RECK, SMOC1, SND1, and TNFSF9 were found hypermethylated almost exclusively in ER-positive tumors while, ADHFE1, DYNLRB2, HSD17B8, PDXK, PISD and WNK4 were found hypermethylated in ER-negative tumors. A number of genes previously reported as having subtype-specific methylation were also identified. EVI1, ETS1, IRF7, LYN, PTGS2 (COX2), RUNX3, and VIM were found to be hypermethylated in ER-positive tumors, while DAB2IP, HSD17B4, and PER1 were reported to be hypermethylated in ER-negative breast cancers (detailed information and references in Table 3). A second distinct CpG locus of PDXK was previously found hypermethylated in ER-positive breast cancers (29) We did not find any gene that was preferentially methylated in ER-positive or ER-negative tumors where the literature conflicted with our data. The concordance between current and published data is shown for two of of these gene CpG loci, EVI1 (23-25) and DAB2IP (23), in Figure 3B. Thus, many novel and some published gene loci were discovered that showed tumor-specific and ER-subtype specific hypermethylation. Existing literature provided further validity to our current observations.
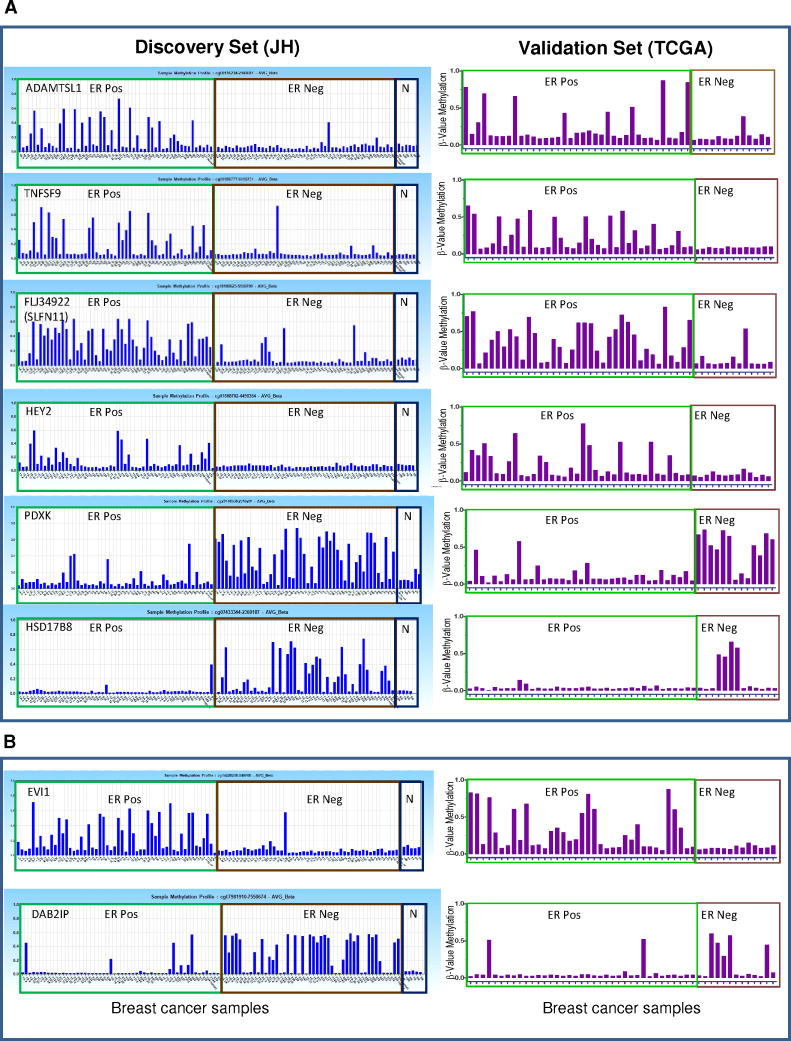
CpG Methylation Biomarkers of ER-positive versus ER-negative subtypes in primary tumors. In the left panel (Discovery set, JH) CpG loci were evaluated using 103 primary tumors. The Y-axis: β-methylation, X-axis: Breast cancer samples, and N: normal breast organoids. The ER-status of the tumors is denoted on top of each box. Right panel (TCGA validation set), shows validation on an independent dataset (n=50) from TCGA. Shown are representative novel (A) and previously known (B) CpG loci specifically hypermethylated in ER+ and ER− breast cancers.
Table 2
Gene symbol | Identified in this study | Hyper-Methylated in other cancers | Known aberrant expression | Gene | Location | Ref |
---|---|---|---|---|---|---|
ACADL | ER-POS | NO | NO | acyl-CoA dehydrogenase, long chain | Cytoplasm | |
ADAMTSL1 | ER-POS | NO | NO | ADAMTS-like 1 | Extracellular | |
ARFGAP3 | ER-POS | NO | NO | ADP-ribosylation factor GTPase activating protein 3 | Cytoplasm | |
B3GAT1 | ER-POS | NO | NO | beta-1,3-glucuronyltransferase 1 | Cytoplasm | |
CDCA7 | ER-POS | NO | ER-NEG (36) | cell division cycle associated 7 | Nucleus | (36) |
FAM78A | ER-POS | NO | NO | family with sequence similarity 78, member A | unknown | |
FAM89A | ER-POS | NO | NO | family with sequence similarity 89, member A | unknown | |
FLJ31951 (RNF145) | ER-POS | NO | BASAL (37) | unknown | unknown | (37) |
FLJ34922 (SLFN11) | ER-POS | NO | NO | schlafen family member 11 | Nucleus | |
GAS6 | ER-POS | NO | NO | growth arrest-specific 6 | Extracellular | |
HAAO | ER-POS | NO | NO | 3-hydroxyanthranilate 3,4-dioxygenase | Cytoplasm | |
HEY2 | ER-POS | NO | NO | hairy/enhancer-of-split related with YRPW motif 2 | Nucleus | |
HOXB9 | ER-POS | NO | NO | homeobox B9 | Nucleus | |
ITGA11 | ER-POS | NO | NO | integrin, alpha 11 | Plasma Membrane | |
NETO | ER-POS | NO | NO | neuropilin (NRP) and tolloid (TLL)-like 2 | unknown | |
PROX1 | ER-POS | NO | NO | prospero homeobox 1 | Nucleus | |
PSAT1 | ER-POS | NO | BASAL (37) | phosphoserine aminotransferase 1 | Cytoplasm | (37) |
RECK | ER-POS | YES | NO | reversion-inducing-cysteine-rich protein with kazal motifs | Plasma Membrane | |
SMOC1 | ER-POS | NO | NO | SPARC related modular calcium binding 1 | Extracellular | |
SND1 | ER-POS | NO | NO | staphylococcal nuclease and tudor domain containing 1 | Nucleus | |
TNFSF9 | ER-POS | YES | NO | tumor necrosis factor (ligand) superfamily, member 9 | Extracellular | |
ADHFE1 | ER-NEG | YES | NO | alcohol dehydrogenase, iron containing, 1 | unknown | |
DYNLRB2 | ER-NEG | NO | NO | dynein, light chain, roadblock-type 2 | Cytoplasm | |
HSD17B8 | ER-NEG | NO | NO | hydroxysteroid (17-beta) dehydrogenase 8 | Cytoplasm | |
PISD | ER-NEG | NO | NO | phosphatidylserine decarboxylase | Cytoplasm | |
PDXK (C21orf124) | ER-NEG | NO | NO | Pyridoxal kinase (vitamin B6 kinase) | Cytoplasm | |
WNK4 | ER-NEG | NO | NO | WNK lysine deficient protein kinase 4 | Plasma Membrane |
Table 3
Gene symbol | Published | Hyper methylated in other cancers | Known aberrant expression | Gene | Location | Ref |
---|---|---|---|---|---|---|
EVI1 (MECOM) | ER-POS (25) | NO | BASAL (38) | MDS1 and EVI1 complex locus | Nucleus | (25, 38, 39) |
ETS1 | ER-POS (25) | BASAL (37) | v-ets erythroblastosis virus E26 oncogene homolog 1 | Nucleus | (37) | |
IRF7 | ER-POS (25) | YES | interferon regulatory factor 7 | Nucleus | ||
LYN | ER-POS (25) | YES | BASAL (37, 40, 41) | v-yes-1 Yamaguchi sarcoma viral related oncogene homolog | Cytoplasm | (25, 37, 40, 41) |
PDXK | ER-POS | NO | NO | pyridoxal kinase (vitamin B6 kinase) | Cytoplasm | (29) |
PTGS2 (COX2) | ER-POS (25) | YES | BASAL (37) | prostaglandin-endoperoxide synthase 2 | Cytoplasm | (25, 37) |
RUNX3 | ER-POS (42) | YES | BASAL (37) | runt-related transcription factor 3 | Nucleus | (37, 42) |
VIM | ER-POS | YES | BASAL (40) | vimentin | Cytoplasm | (40) |
DAB2IP (4 LOCI) | ER-NEG (25) | YES | DAB2 interacting protein | Plasma Membrane | (25) | |
HSD17B4 | ER-NEG (43) | YES | ER POS (43) | hydroxysteroid (17-beta) dehydrogenase 4 | Cytoplasm | (43) |
PER1 | ER-NEG (23) | period homolog 1 (Drosophila) | Nucleus | (23) |
External validation of methylation array findings in an independent test set of primary tumors
Next we validated these findings in publicly available data on the breast cancer samples in TCGA (http://tcga-data.nci.nih.gov/) using an ROC analysis to evaluate predictive ability (Supplemental Table 2A). The median area under the ROC curve for the 200 loci was 0.7; and one gene, SERPINA12, had an AUC of 0.95. In all, 156/200 ER probes yielded AUCs higher than 0.563, a range in which we expect only 5% of CpG loci by chance alone. Interestingly, expression of most of these same genes is also a very strong predictor of ER status. Here, 121 of the 175 unique genes from our ER panel and available on the expression array had areas under the curve exceeding the same 5% threshold. This is consistent with the high degree of correlation observed between expression and methylation measurements of these genes in the TCGA data. At an FDR of 0.05, 142 of 200 CpG loci are significantly inversely correlated with expression. And, as seen in Figure 3, the TCGA data provided support for the existence of ER-subtype-specific methylation in breast cancer. To evaluate the predictive performance of the 40 locus panel (Figure 2D), we derived an average methylation score for the entire set as described in the Methods. Using this score ROC analysis demonstrated a high classification accuracy for the ER-subtype in TCGA data with an area under the ROC curve of 0.961, with a specificity of 89% at a sensitivity of 90% (Figure 2D; details in Supplemental Materials and Methods). A similar composite score derived from expression probes for the same genes showed some discriminatory ability in the TCGA data, albeit reduced, with an area under the ROC of 0.667 (data not shown).
CpG loci associated with disease progression in patients with newly diagnosed invasive breast cancer
To develop an epigenomic signature that predicts outcome in patients with breast cancer, we conducted differential methylation analysis on primary tumors from recurrent versus non-recurrent breast cancers. We used a subgroup of 82 well-annotated, invasive breast tumors derived from the discovery set of 103 tumors that included 44 ER-positive (7 recurrences) and 38 ER-negative (11 recurrences) breast cancers and independently queried the ER-positive and ER-negative tumor groups (Table 1, Supplementary Table 1) as follows. Differential methylation analysis was performed in GenomeStudio, using the DiffScore algorithm to compare tumors which later recurred to those which did not recur. The analysis was performed separately on the ER-positive and ER-negative tumor groups. Candidate loci (50 per ER subtype) were selected meeting 3 criteria: 1) more highly methylated in recurrent tumors than in non-recurrent tumors, 2) relatively unmethylated in normal samples (β < 0.15), and 3) significantly differentially methylated above the false discovery rate cutoff (5%). Next we performed a multivariate Cox regression analysis for each of these candidate loci and generated Kaplan-Meier plots, showing the interrelationships between ER status and methylation and depicted in these plots as high/low with respect to the median methylation level for each CpG locus. From these 100 candidate CpG loci, a set of 32, selected for high Cox coefficients (Supplemental Table 4A), and visually striking Kaplan-Meier plots (Figure 4, Supplemental Figure 3) were followed up most closely, including with an extensive literature search to identify previous associations with outcome in breast cancer (Supplemental Table 4C). Novel associations with poor outcome were identified for 1) TMEM179, CRMP1 and SCNN1B in ER-positive breast cancer, 2) ALX1, COL14A1, EPHA5, EYA4, FLRT2, GPX7, KCNB2, LAMA1, LHX1, NEUROG1, POU3F2, AND STMN3 in ER-negative breast cancer, and 3) AKR1B1, COL6A2, EYA4, GPX7, HOXA13, HOXB13, NKX6-2, NRP2, POU4F2, REM1, and SLITRK2, in both ER-positive and ER-negative tumors. Cox regression p-values for each member of the 100 CpG loci set is presented in Supp Table 4A. Since the differential methylation analysis was designed in such a way to find loci most highly methylated in recurrent tumors, we did not observe hypomethylated loci associating with recurrence.
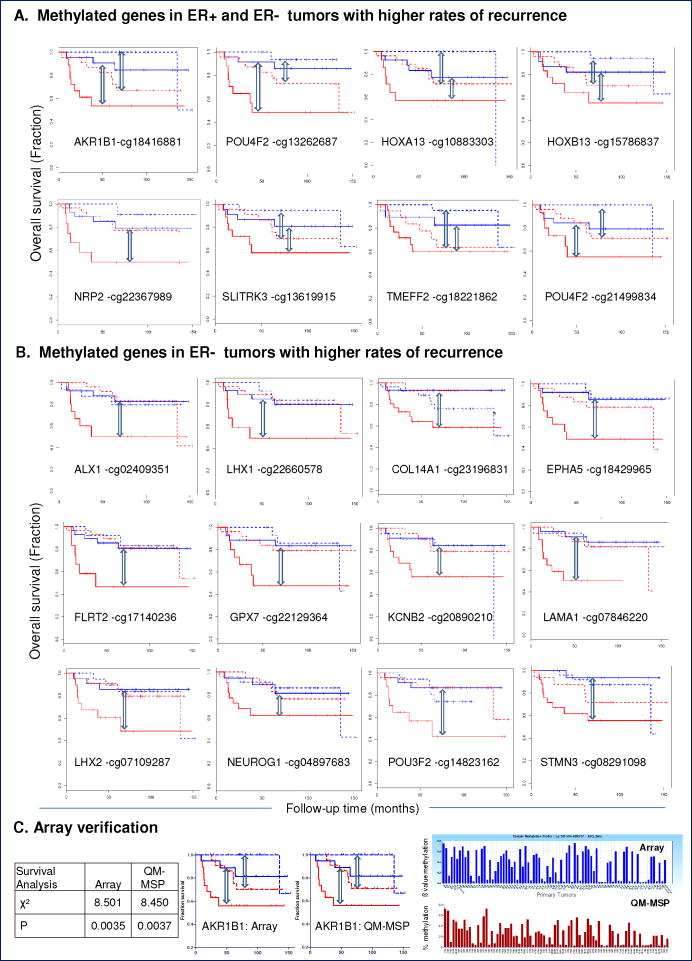
Methylated CpG Loci Associated with Disease Progression.
Kaplan-Meier plots show CpG loci with strong associations between hypermethylation and high rate of disease progression among 82 tumors. Recurrence is shown for ER+ (dashed line), and ER-negative tumors (solid line). High (red line) and low (blue line) methylation was defined relative to the median methylation level for a given CpG loci within ER subtype. CpG loci associated with a high rate of recurrence in (A) ER+ and ER-negative breast tumors. (B) ER-negative breast tumors. Cox regression p-values for each of the 100 CpG loci set is presented in Supp Table 4A. (C) Verification of HumanMethylation27 array data (top panel, blue bars) by QM-MSP analysis (bottom panel) for AKR1B1. X-axis: Tumors; Y-axis: methylation levels (β-value for array, % methylation for QM-MSP). Kaplan-Meier curves using array and QM-MSP values.
Figure 4A: CpG loci associated with high rate of recurrence in ER+ and ER− breast cancer
Figure 4B: CpG loci associated with high rate of recurrence in ER-negative breast cancer
Figure 4C: Translation from array to QM-MSP for AKR1B1
To verify array data using an independent assay, and to ensure future technical translation of the HumanMethylation27 array data to laboratory assays, we tested several methylated genes, such as EVI1, DAB2IP and AKR1B1 by performing Quantitative Multiplex-Methylation Specific PCR (QM-MSP). In each case, we observed an excellent correlation between the levels of gene methylation assessed by both assays. A comparison of level of methylation in AKR1B1 assessed by the array and by QM-MSP in individual primary tumors, and both data plotted as Kaplan-Meier plots in shown in Figure 4C.
A striking observation was that nearly 20% of the recurrence loci (18/100 loci; 15/91 unique genes) were from homeobox-containing genes including the HOX, LHX, POU, ALX and NK6 gene families (Figure 5, Supplemental Table 4A). With only 375 homeobox loci (189 genes) present in the 27,578 loci (14,495 genes) array, this represented a dramatic enrichment of homeobox genes in our 100 loci recurrence related set (odds ratio = 16.17, p = 6.515e-13). These data clearly implicate methylated homeobox genes as key factors in tumor progression. To determine if the other homeobox loci on the array exhibited similar methylation patterns, we extended our analysis to 60 homeobox loci which showed high variance (SD above the 95th percentile for the array) among the tumors, excluding the 18 loci represented in the recurrence sets. 2D-hierarchial cluster analysis (using the Manhattan distance) was performed to characterize these loci. As shown in the heatmap in Figure 5 (panel A), the 18 homeobox gene loci derived from the 100 recurrence locus set have distinctive methylation patterns, showing significant co-methylation within the first cluster, with highly methylated samples tending to be methylated for all the loci. Interestingly, a similar clustering profile was observed with the 60 homeobox loci (Figure 5, panel B), suggesting that the homeobox genes as a group have a common methylation signature. To evaluate correlation with recurrence, we derived an average methylation score for the panel as described in Methods. In a multivariate analysis that included age, stage, treatment and ER status, there was clear evidence of a significant additional and independent contribution to the model where the Cox coefficient was 1.74, with a p-value of 0.0042. Kaplan-Meier plots for the 18 and the 60 homeobox loci (but not for all 1378 CpG loci that showed differential methylation across all the tumors) illustrate their predictive value. These results support the notion that highly methylated homeobox loci and loss of their expression may likely contribute to poor outcome in breast cancer.
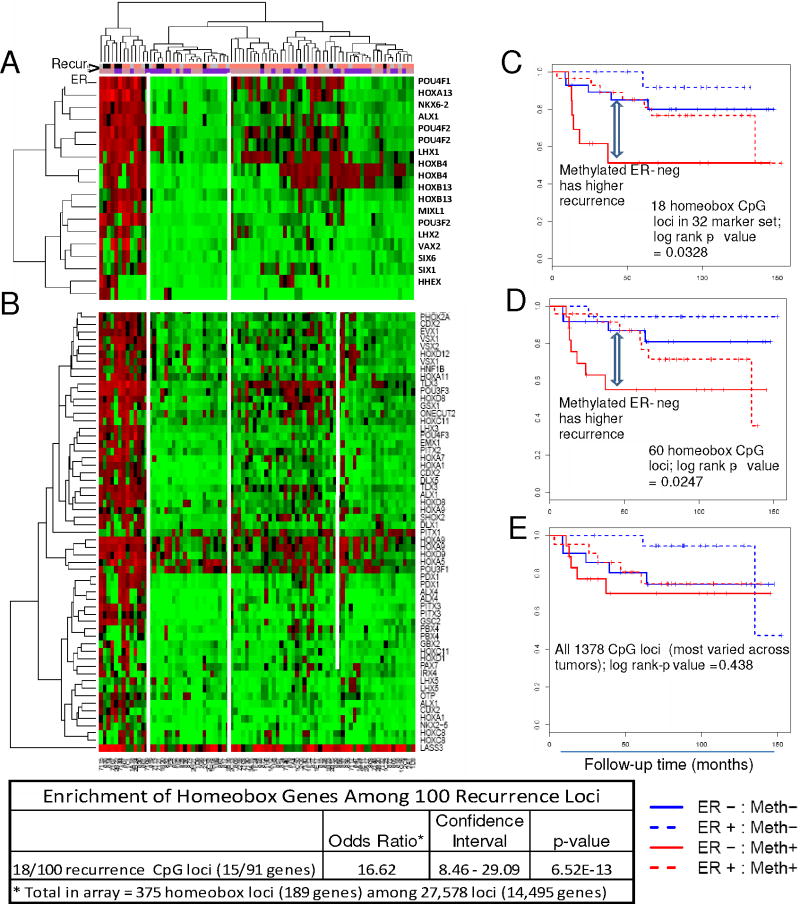
2-D Hierarchical cluster analysis of CpG loci in homeobox genes. Methylation levels of homeobox (A) 18 loci from the 100 recurrence markers; and (B) 60 additional unique loci in 82 primary invasive tumors. Kaplan-Meier plots of homeobox (C) 18 loci set, log rank p-value=0.033, (D) 60 loci set, p-value=0.025, and (E) unsupervised top 5% most varied array probes across tumor samples, p-value=0.438. Strong enrichment of homeobox genes was observed within the 100 recurrence loci set (table). The bars under the case dendrogram indicate recurrence (black, recurred < 60 mo; salmon, did not recur by 60 mo; gray, censored by 60 mo) and ER (pink, ER-positive; purple ER-negative) status.
Analysis of methylation array homeobox gene loci in primary invasive tumors (n=82)
External validation of associations with outcome, in an independent test set of primary tumors
Next we sought to validate these findings in publicly available TCGA breast cancer samples (http://tcga-data.nci.nih.gov/), using Cox regression to evaluate association between methylation and overall survival; progression free survival was not available for these samples at the time of download. In total, survival information was available for 342 of the samples available on expression array, of which 182 were also available on methylation array. Despite the change of outcome variable and moderate sample sizes, results in TCGA data as a set confirmed the findings that these genes are significantly associated with outcome. An overwhelming majority of our recurrence marker loci (78/100) have positive Cox regression coefficients, indicating that hypermethylation of these loci is associated with a worse outcome in these samples as well. By comparison, we would expect only half of these loci to have positive Cox coefficients by chance alone, giving a composite p-value of 2.2e-09, in support of the association. Additional confirmation for the panel is provided by the fact that for more than 2/3 of these genes, Cox regression analysis of TCGA expression data shows that low expression correlates with worse outcome. This result is wholly consistent with the observed methylation results, and statistically significant in its own right, with a p-value of 0.00022. This is consistent with the high degree of correlation observed between expression and methylation measurements of these genes in the TCGA data. At an FDR of 0.05, 43 of 100 CpG loci are significantly inversely correlated with expression. We also performed a multivariate Cox regression analysis for each of these candidate loci and generated Kaplan-Meier plots for the sets of 18 loci (log rank test p-value 0.00027) and 60 loci (log rank test p-value 0.00036), compared to the top 5% of varied probes (1378 probes, p-value 0.112), demonstrating significant interrelationships between homeobox gene methylation and survival (data not shown).
Discussion
In this study, we report the results of a genome wide array analysis of primary invasive breast cancers of 27,578 CpG loci. This screen identified hypermethylated genes that specifically segregate with ER-positive or ER-negative tumor subtypes, which were then validated in silico using the newly populated TCGA breast cancer database. The array analysis also identified 100 gene loci that were enriched for homeobox-containing genes and predicted recurrence in breast cancers. Many novel hypermethylated loci were identified. In summary, we demonstrate that the methylome is a rich source of genes whose hypermethylation has the potential to significantly contribute to the understanding of ER-subgroups of breast cancer and predict recurrence in ER-positive and in ER-negative breast cancers.
We observed a significantly higher frequency of hypermethyation in ER-positive compared to ER-negative tumors (p<0.0001). The reason for the hypermethylated phenotype of ER-positive tumors is not yet clear. The simplest explanation could be that the ER-positive markers are drivers that enhance the expression of the DNA methyltransferases, or inhibit the repair processes that remove methyl groups from DNA. On the other hand, reduced methylation in ER-negative tumors might offer an explanation for their relative aggressiveness since the uncontrolled expression of growth factors and their receptors may be facilitated by removing the protective imposition of methylation-mediated silencing. The observation of a higher frequency of hypermethylated genes in ER-positive tumors is substantiated by five recent studies describing the breast cancer methylome (22, 24-26, 29). In a study examining 44 primary tumors, Hill et al (22) confirmed a highly significant difference (ANOVA, p value=0.001) in hypermethylation depending on hormone receptor status; in their study. Similarly, in the Fang study (26), ER/PR-positive tumors displayed high level of methylation across the top 5% variant loci in the 27K Illumina array. Our study of 103 primary breast cancers identified many novel loci that have the ability to impressively segregate ER-positive and ER-negative tumors (Table 2, Figure 3A, B), shedding light on many novel pathways and constituent genes that may be involved in the genesis of these subgroups. We tested the strength of these observations using an independent dataset from TCGA for both methylation and expression. All 40 CpG loci showed reproducible associations with ER-subtype and these markers classified essentially all tumors into the correct ER subtype (AUC 0.961). Interestingly, expression of the same genes was also found to be a very strong predictor of ER status. Thus, independent TCGA data strongly validated our findings. With the caveat that both the discovery and validation cohorts represent small sample sets, the reproducibility of the findings supports the strength of this platform to reveal differences that can now be studied in detail.
A major goal of our work is to find markers that can prognosticate recurrence and predict benefit from therapy. Expression array-based analyses have proven to be useful for ER-positive breast cancers (30, 31). Their utility, however, has been limited in ER-negative breast cancer. Also, DNA mutation and copy number studies have been found less useful in breast cancer compared to other cancers (eg. lung and colon), probably reflecting the greater diversity of breast cancer subtypes (32-34). Epigenetically mediated gene silencing through DNA methylation occurs extremely frequently and has now been accepted as a major driver in neoplastic transformation, especially in the breast (12). Genome-wide methylation analysis allowed us to identify a tumor recurrence marker set of 100 gene loci; a few specific to ER-positive, many loci specific to ER-negative tumors and many common to both (Figure 4, Supplemental Table 4C). The emergence of a homeobox gene-methylation signature predictive of recurrence among the 100 recurrence loci and also among all homeobox loci on the array is notable. Substantial inverse correlation was seen between methylation and expression of the genes, both of the ER-stratifying set and the recurrence set of CpG loci, suggesting functional relevance to the effects of methylated genes observed in our study. These genes play critical roles in differentiation and development, growth factor receptor signaling, angiogenesis and more recently an unequivocal role in stem cell function [reviewed in (35)] At the same time, particularly within the recurrence panel, expression alone could not duplicate the level of performance achieved with methylation probes.
The tissues used for the current analysis were from an institutional cohort of frozen specimens and are therefore, samples of convenience with their inherent drawbacks. Additional studies will need to address the question of the precise role of methylation signatures in prognosticating outcome and predicting response to therapy. More discovery and validation will need to be performed with annotated samples from controlled studies, with more uniform standards of sample collection, such as in the context of large mature randomized clinical trials. To allow investigation on archival specimens, the rapid development of methods to retrieve high quality DNA from paraffin embedded tissues is imperative. Our recent success in standardizing restoration of DNA retrieved from FFPE tissues, in collaboration with Illumina (our unpublished data, AACR Abstract LB 178, 2011), bodes well for the future of these investigations.
In summary, this study has demonstrated the feasibility of distinguishing ER-subtype in breast cancers and possibly predicting outcome based on CpG DNA methylation. The study suggests pathways that may explain distinctive behaviors among ER-positive and ER-negative tumors. In conclusion, the data strongly support upcoming planned studies that will use existing clinically-annotated tissues from previously conducted prospective randomized trials to examine the prognostic outcome and predictive therapeutic information offered by methylation markers in a prospective-retrospective fashion.
Supplementary Material
Supplementary Figure 1
Supplementary Figure 2
Supplementary Figure 3
Supplementary Table 1
Supplementary Table 2
Supplementary Table 3
Supplementary Table 4
Supplementary Text
Acknowledgments
Grant support: This work was supported by grants from the Rubenstein and Cohen families and the Breast Cancer SPORE: P50-CA-88843. We thank Dr. Wayne Yu for performing the methylation microarray, Drs. Gedge Rosen and Michelle Manahan for providing the reduction mammoplasty tissues, and Ms. Areli Lopez for excellent technical assistance.
References
Full text links
Read article at publisher's site: https://doi.org/10.1158/0008-5472.can-11-1630
Read article for free, from open access legal sources, via Unpaywall:
https://aacrjournals.org/cancerres/article-pdf/71/19/6195/2657007/6195.pdf
Citations & impact
Impact metrics
Citations of article over time
Alternative metrics

Discover the attention surrounding your research
https://www.altmetric.com/details/102130035
Article citations
Hypomethylation of ATP1A1 Is Associated with Poor Prognosis and Cancer Progression in Triple-Negative Breast Cancer.
Cancers (Basel), 16(9):1666, 25 Apr 2024
Cited by: 0 articles | PMID: 38730618 | PMCID: PMC11083557
Alteration of DNA methyltransferases by eribulin elicits broad DNA methylation changes with potential therapeutic implications for triple-negative breast cancer.
Epigenomics, 16(5):293-308, 15 Feb 2024
Cited by: 1 article | PMID: 38356412
Discovery of novel DNA methylation biomarker panels for the diagnosis and differentiation between common adenocarcinomas and their liver metastases.
Sci Rep, 14(1):3095, 07 Feb 2024
Cited by: 1 article | PMID: 38326602 | PMCID: PMC10850119
Estrogen Receptor Signaling in Breast Cancer.
Cancers (Basel), 15(19):4689, 23 Sep 2023
Cited by: 10 articles | PMID: 37835383 | PMCID: PMC10572081
Review Free full text in Europe PMC
Notch, SUMOylation, and ESR-Mediated Signalling Are the Main Molecular Pathways Showing Significantly Different Epimutation Scores between Expressing or Not Oestrogen Receptor Breast Cancer in Three Public EWAS Datasets.
Cancers (Basel), 15(16):4109, 15 Aug 2023
Cited by: 1 article | PMID: 37627137 | PMCID: PMC10452656
Go to all (132) article citations
Data
Data behind the article
This data has been text mined from the article, or deposited into data resources.
BioStudies: supplemental material and supporting data
Similar Articles
To arrive at the top five similar articles we use a word-weighted algorithm to compare words from the Title and Abstract of each citation.
Methylome and transcriptome analyses reveal insights into the epigenetic basis for the good survival of hypomethylated ER-positive breast cancer subtype.
Clin Epigenetics, 12(1):16, 20 Jan 2020
Cited by: 2 articles | PMID: 31959227 | PMCID: PMC6971951
Genome-wide DNA methylation profiling of CpG islands in breast cancer identifies novel genes associated with tumorigenicity.
Cancer Res, 71(8):2988-2999, 01 Mar 2011
Cited by: 108 articles | PMID: 21363912
DNA methylation and hormone receptor status in breast cancer.
Clin Epigenetics, 8:17, 16 Feb 2016
Cited by: 31 articles | PMID: 26884818 | PMCID: PMC4754852
Prognostic and Predictive Biomarkers of Endocrine Responsiveness for Estrogen Receptor Positive Breast Cancer.
Adv Exp Med Biol, 882:125-154, 01 Jan 2016
Cited by: 21 articles | PMID: 26987533
Review
Funding
Funders who supported this work.
NCI NIH HHS (5)
Grant ID: P50 CA088843-10
Grant ID: P50 CA088843
Grant ID: P50-CA-88843
Grant ID: R01 CA140311
Grant ID: U54 CA112970