Abstract
Free full text

Challenges and Opportunities in Genome-Wide Environmental Interaction (GWEI) studies
Abstract
The interest in performing gene-environment interaction studies has seen a significant increase with the increase of advanced molecular genetics techniques. Practically, it became possible to investigate the role of environmental factors in disease risk and hence to investigate their role as genetic effect modifiers. The understanding that genetics is important in the uptake and metabolism of toxic substances is an example of how genetic profiles can modify important environmental risk factors to disease. Several rationales exist to set up gene-environment interaction studies and the technical challenges related to these studies – when the number of environmental or genetic risk factors is relatively small – has been described before.
In the post-genomic era, it is now possible to study thousands of genes and their interaction with the environment. This brings along a whole range of new challenges and opportunities. Despite a continuing effort in developing efficient methods and optimal bioinformatics infrastructures to deal with the available wealth of data, the challenge remains how to best present and analyze Genome-Wide Environmental Interaction (GWEI) studies involving multiple genetic and environmental factors. Since GWEIs are performed at the intersection of statistical genetics, bioinformatics and epidemiology, usually similar problems need to be dealt with as for Genome-Wide Association gene-gene Interaction (GWAI) studies. However, additional complexities need to be considered which are typical for large-scale epidemiological studies, but are also related to “joining” two heterogeneous types of data in explaining complex disease trait variation or for prediction purposes.
Introduction
Experimental studies in model organisms have provided several evidences of interactions between genes and exposures. For a review about the utility of mouse models in the detection of gene-environment interaction effects and the limitations on their application, we refer to Willis-Owen and Vade (2009). These animal models may be helpful in suggesting candidate gene-environment interactions, but epidemiological studies – although more complicated – are needed if we ever want to have a complete understanding of the genetic architecture of complex human diseases. Most common complex diseases are believed to be the result of the combined effect of genes, environmental factors and their interactions. Throughout this document, we will use the terms exposure and environment interchangeably.
The term “gene-environment interaction” is often loosely used as referring to the interplay of gene and environment in some way. A first clear reporting of different categories of gene-environment interactions dates back from 1938 as referred to in Smith and colleagues (2008). Here, we define it via “biological” or “statistical” interaction. A biological gene-environment interaction occurs when one or more genetic and one or more environmental factors participate in the same causal mechanism in the same individual (Rothman et al. 2008; Yang and Khoury 1997). One popular and appealing formal definition of “biological interaction” invokes the sufficient component cause model of causation. In this setting, there is one sufficient component cause that involves both the genetic and environmental exposure (Rothman and Greenland 1998; Tchetgen Tchetgen and VanderWeele 2012). (We note that this definition of “biological interaction” does not imply anything about the biochemical mechanism of how genes and environment combine to cause disease.)
In contrast, the statistical interactions, which are typically defined as modifications of the effect on one factor by the levels of the other factor in some underlying scale (Bhattacharjee et al. 2010; Greenland 2009; Siemiatycki and Thomas 1981; Thompson 1991), do not imply any inference about a particular biological mode of action. Statistical interactions can be clustered variously based on the specificity of the underlying statistical models. The common classification distinguishes between “quantitative interaction” and “qualitative interaction”. Quantitative interaction refers to the presence of a factor (e.g. an exposure) that modified the magnitude of the effect of a second factor (e.g. a mutation) without changing the direction of the effect. On the other hand qualitative interaction refers to situation where a factor will either cancel or reverse the effect of another factor. For additional details on these definitions see Clayton D. (Clayton 2009) or Thomas D. (Thomas 2010a). For example of statistical models of interactions see for example Wright et al (2002) or Dempfle et al. (2008).
Gene-environment interaction effects have been investigated for a wide range of candidate genes and exposures for many complex traits, such as cancer, depression, Type 2 Diabetes, and asthma (Franks 2011; Hunter 2005; Lesch 2004; Stern et al. 2002; Vercelli 2010; Wu et al. 2011). However, only a handful of the large number of reported statistically significant interactions has been replicated, despite well-powered replication efforts for some influential preliminary reports (Cornelis et al. 2011; Dunn et al. 2011; Risch et al. 2009). The candidate gene interaction literature suffers from many of the same problems that plagued the literature on marginal effects of candidate genes, including small sample sizes and inappropriate (or lack of) adjustment for multiple testing. Moreover, replication in the context of gene-environment interaction effects faces additional challenges, including differences in exposure measurement protocols across studies, differences in the scale of reported gene-environment interaction effects, and differences in the distribution of exposures across studies. The candidate gene interaction literature can therefore only provide limited guidance on the number and size of gene-environment interaction effects expected to truly exist in human populations, although it does suggest that large and pervasive interaction effects are unlikely.
Genome-wide approaches to identify loci involved in gene-environment interactions have just begun to appear in the peer-reviewed literature (Ege et al. 2011; Hamza et al. 2011; Pare et al. 2010). For example, Ege et al. (2011) recently completed a Genome-Wide Environment Interaction (GWEI) study for childhood asthma and farming exposures in the context of GABRIEL (A Multidisciplinary Study to Identify the Genetic and Environmental Causes of Asthma in the European Community). Although this study was well-powered to detect gene-environment interactions for common alleles, no interactions were statistically significant, not even those interactions involving genetic markers in genes previously reported to show interactions (Ober and Vercelli 2011). Developing methods to overcome the conceptual, technical, and methodological hurdles GWEI studies involve is the focus of much ongoing methodological work.
Gene-environment interaction at the age of genome-wide data has been recently discussed in several reviews (Dempfle et al. 2008; Hunter 2005; Khoury and Wacholder 2009; Thomas 2010a, 2010b). In this review, we focus on strategies and methodological aspects of genome-wide association study of gene-environment interactions. In particular, we provide an overview of possible analytical choices in relation to researchers’ aims and beliefs. Simply stated, what are the main advantages and disadvantages of the existing approaches based on the goal: identifying new genetic variants involved in interactions, identifying gene-environment interaction per se or screening for potential interactions without testing?
The quest for gene-environment interactions
The interest in studying the combine effect of genes and environmental factors in the etiology of common multifactorial disease has grown up in parallel with the study of their genetic component only. Among the past ten years large investments have been done trying to elucidate some of these mechanisms. The UK Medical Research Council, the Wellcome Trust and the Department of Health for example have launched in 2002 the BioBank UK study, a prospective cohort study of 500,000 individuals, which attempt to integrate the genetic and environmental components of disease risk (Wright et al. 2002). The National Institutes of Health (NIH) has initiated the Genes, Environment and Health Initiative (GEI). It includes the Gene Environment Association Studies (GENEVA) consortium which was established to facilitate the identification of variations in gene-trait associations related to environmental exposures (Cornelis et al. 2010). More recently the Kaiser Permanente Research Program on Genes, Environment, and Health (RPGEH) and the University of California San Francisco have launched a new resource for studying disease, health and aging. In this project, DNA and exposure to environmental factors are collected for more than 100,000 samples.
Besides pharmacogenomics, which represent a particular (and promising) field of study for gene-environment interaction (Meyer 2000; Wright et al. 2002), there are three common arguments that have been emphasized for searching for the presence of gene-environment interactions in common multifactorial diseases. First, for most of the identified genetic variants in GWAs, the mechanisms through which genetic variants contribute to the associated complex phenotypes remains largely unknown. Second, the predictive potential of common genetic variants that have been extensively study in genome-wide scan appears to be limited (Gibson 2010; Visscher et al.; Yang et al. 2010). Third, the common SNPs that have been identified so far only explain a small proportion of the variance of complex traits. Overall, interaction effects with environmental factors are considered one possible key to a better understanding of the genetic architecture of complex traits (Manolio and Collins 2007; Zuk et al. 2012). Gene-environment interactions might also be further translated into improvement in our ability to predict disease risk and be of utility for various personalized medicine applications, such as targeting individuals that may need costly intervention (Rothman et al. 1980)
However this ideal picture needs to be balanced by our current knowledge of statistical interaction effect in epidemiology. First, it is notoriously difficult to make inference regarding biological mechanisms from epidemiologic data, and interaction reflect a level of complexity that makes such inference even harder (Clayton 2009; Greenland 2009; Siemiatycki and Thomas 1981; Thompson 1991). Second, interactions are unlikely to dramatically improve risk prediction if they have only moderate effects or if the number of interactions is low (Aschard et al. 2012). Third, the identification of any interaction effect is recognized as an extremely challenging task and the lack of discoveries clearly confirms this issue. Hence a reasonable consensus is that gene-environment interaction studies may at least help in the discovery of new genetic variants and new environmental risk factors, (Gauderman and Thomas 2001; Kraft et al. 2007; Manolio and Collins 2007), which remains an important step toward our understanding of complex diseases.
Our ability to attain some of these goal increases with the growing number of rich heterogeneous data resources, with data available on genetics, family history, physical and behavioral characteristics, life-style, intra-individual changes over time, etc. However it also comes with some caveats. Despite the fact that these data allow the investigation of more complex, possible nonlinear relationships between genetic and non-genetic factors, it remains the question whether the toolbox that is available to date contains sufficiently refined tools and methodologies to be applied in a genome-wide context. Compared to the total number of paper published on gene-environment interactions, GWEI studies only represent a handful of studies (Figure 1). While we believe gene-environment interaction are more and more studied at the genome-wide scale, the low number of publications may be partially explained by the non-publication of negative results. It may indicate that there is still room for novel approaches and rigorous strategies, that can overcome some of the hurdles scientists are facing when performing a GWEI study.
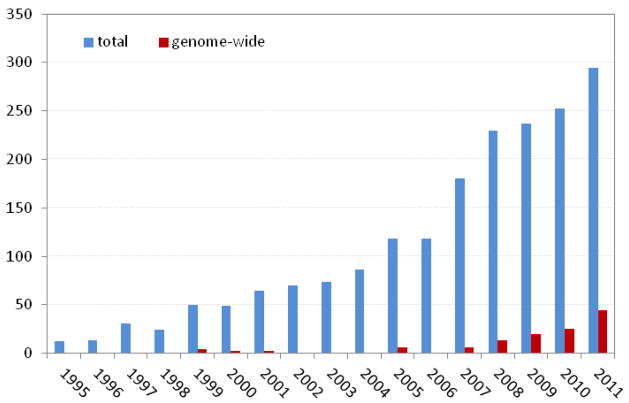
Number of papers in PubMed with (“gene-environment” or “gene-by-environment” or “gene x environment”) and “interaction” in the title or abstract (in blue). Furthermore, the number of papers is shown which additionally to the previous search term also contain (“genome-wide” or genomewide) in the title or abstract (in red). It should be noted that this search only retrieves “potential” GWEI studies and that the real numbers of GWEI studies are probably even lower than the reported counts.
What are possible complicating factors in GWEI studies?
Confounding
Confounding may occur when independent variables are associated with one another and with the outcome of interest. In epidemiology it refers to situation when an extraneous variable that cause the phenotype under study is also associated with a predictor of interest that is not causal (i.e. that is not on the “causal pathway” of the phenotype). The existence of confounding variables can make it difficult to establish a clear causal link between the studied predictor and the outcome unless appropriate methods are used to adjust for the effect of the confounders. However dealing with known confounders is relatively easy. It can be minimized or controlled by a study design or by employing appropriate data analysis methods such as multiple regression or stratification analyses (Demissie and Cupples 2011; Rothman et al. 2008). Dealing with unknown confounders is obviously much trickier, although recent work has shown that unknown confounders of the interacting factors may not necessarily bias the estimation of interaction effect per se (Tchetgen Tchetgen and VanderWeele 2012). It should also be noted that the case-only technique is more likely to be subject to confounding. For example, when analyzing related individuals, family-history, which is related to genetic susceptibility as well as life-style exposures, may create artificial dependencies between a mutation and an exposure. Such confounding effects may invalidate the case-only test while it may be easily handled by using family-data methods (Thomas 2000). Confounding due to latent population substructures, when unintentionally including groups of different ethnicity, is also known to have a larger impact on the validity of the case-only test of interaction than on the case-control interaction test (Wang and Lee 2008).
Exposure measurement error and misclassification
The detection of G-E interactions can be severely hampered by unreliability in the assessments of exposures. Measurement challenges for underlying key exposures (e.g., diet, physical activity, air pollution parameters) present important barriers to interaction identification, but equally the assessment of their marginal impact on disease trait (Prentice 2011). Measurement error (or misclassification when explanatory variables in regression models are categorical) is a well known issue in association studies that can both bias point estimates and generate invalid association. In general, conventional parametric and non-parametric regression techniques are no longer valid when errors in the predictors are expected. Improved study design and methods for corrections have been widely discussed in studies of a single factor (Bashir and Duffy 1995). More recently, attention has been given to the impact of exposure measurement error in G-E interaction studies (Carroll et al. 2006; Wong et al. 2004). Despite the fact that various solutions are around to handle measurement error during the statistical analysis (Garcia-Closas et al. 1999; Garcia-Closas et al. 1998; Lindstrom et al. 2009; Lobach et al. 2011; Thomas 2010b), these methods are not widely used in practice, even for smaller-scaled G-E interaction studies. Another consideration about exposure measurement error is that the error structures of environmental exposures may differ across populations and this could have implications for how interactions are detected and interpreted.
In practice, misclassification is usually addressed from two perspectives: a) how to correct for misclassification in statistical test and b) how to define the trade-off between sample size and measurement precision to maximize statistical power. The common approach to account for misclassification in statistical test is to use validation studies. It consists in measuring repeatedly a fraction of the sampled subjects with the same error-prone instrument to obtain estimates of misclassification probabilities. Various statistical techniques can be built on this framework. Some of them have been recently described by Zhang et al. (2008) who also introduce simple and practically useful concepts to minimize the biases of all parameters of interest in the presence of both genotyping and exposure misclassification errors. Unfortunately, validation or repeated measurement data that is required to apply such methods in practice are not available in typical studies. When the misclassification issue is considered at the design stage, the perspective is slightly different. Since improving the measurement can be achieved by taking repeated measurements for all individuals (provided the error in repeated measures is uncorrelated), the question is how to balance quantity and quality. Obviously, for a fixed total number of subject evaluations, the use of multiple measurements per subject would result in a halving of sample size. Wong et al. (2003) provide arguments for this strategy by showing that smaller studies with reasonably accurate measurement might be more efficient than larger studies with poor assessment of exposure and outcome when the goal is testing for interaction per se. However this result does not necessarily hold when the goal is rather to identify genetic variants while allowing for potential interaction effect. In this case, testing for global genetic effect over multiple exposure strata may conserve reasonable power when misclassification remain low, while the standard test of interaction can suffer a dramatic loss of power (Lindstrom et al. 2009).
Population stratification and population dependencies
Concerns about the widespread of population stratification or the bias it may induce have been raised before (Kraft 2011). Several approaches to population stratification in main effects GWA studies are available and commonly in use (Price et al. 2010). Population stratification also becomes an issue in G-E interaction studies if subpopulation membership based on genetics is associated with the outcome, the genetic effect and the environmental exposure. If the strength of the linkage disequilibrium between the marker and the causal variant varies across preferentially-mating subpopulations, and the distribution of the exposure also differs across subpopulations, then differences in the genetic effect across subpopulations due solely to difference in linkage disequilibrium will appear to be due to G-E interaction (Kraft 2011). In contrast to GWA studies, it is less clear how to correct for population stratification and cryptic relatedness in GWEI studies, since strata or degrees of relatedness may be related to the environmental exposure under investigation. It was recently shown that principal component methods, that have been popular for correction of population stratification in GWA studies, can be used for adjustment of gene-gene or gene-environment dependence due to population stratification in interaction studies (Bhattacharjee et al. 2010).
Alternatively, one can use family-based methods that condition on parental genotypes, which are thought to be robust against population stratification (Laird and Lange 2006). However, recent work by Shi et al. (2011) showed that the standard family-based tests of gene-environment interaction can be biased when the tested genetic variant is not itself the causal variant but a proxy for it (i.e. in linkage disequilibrium with the causal) and the studied exposure does participate in population structure (i.e. when the exposure is correlated with the genotypic strata). They present a solution to correct for such bias when exposure is binary which consists in adjusting for a family-based measure of the exposure distribution. Explicitly they fit a saturated model for the genetic main effect within strata defined by the siblings’ exposure profile (exposure need to be collected for an unaffected sibling). Although the empirical extent of the example presented by Shi and colleagues is unknown, there are realistic scenarios where such bias may occur; especially when analyzing recently admixed population such as African-American or Latino (Kraft 2011).
Dynamics of gene-environment interactions
Many exposures change over time and may be prevalent in one population and rare or absent in another. Thus, the amount of population variation in a disease that can be explained by one or more exposures may not be generalized from one population to another, or from one time period to another (Pearce 2011). The dynamic “behavior” of an exposure is a function of its prevalence over time in an individual and in a population of interest. The nature of the exposure may also be relevant in terms of G-E interaction effects (e.g., the dose and route of exposure, when exposure first or last occurred, or whether exposures were periodic, continuous, intermittent, or single events). Furthermore, there could be critical windows of exposure (etiologically relevant exposure periods), when the exposure is more or less likely to contribute to, or may even have opposing effects on, a disease process. This includes for example conception, fetal development, early childhood, adulthood, before or after the menopause. Several studies have already been successful in identifying such effects (Balansky et al. 2012; Bouzigon et al. 2008; Doherty et al. 2009; Lo et al. 2009). As mentioned above, the calendar time period may also be important since many exposures and exposure opportunities change over time (e.g., environmental tobacco smoke, environmental pollution, processed foods, and pharmaceutical drugs).
To the extent that this is possible in ongoing and future prospective cohort studies, exposure should be periodically re-assessed over the course of a study. The ideal design would be a life course approach in which exposure information is collected at different time points throughout an individual’s life. Such a study would be cost-prohibitive for most investigators, but very large cohorts of individuals that include extended measurement to a range of exposures and genetic data are now in progress. The aformentionned RPGEH project for example includes comprehensive longitudinal health information over long period and will offer the opportunity to explore some of these aspects. Finally, gene-by-‘timing of exposure’ effects might also be amenable to study in animal model systems (models from conception to death). Such model systems may help to inform the potential critical windows of exposure and relevant mechanisms in humans.
Power and sample size
Perhaps one of the greatest challenges in GWEI studies is that of power (Bookman et al. 2011; Murcray et al. 2011; Thomas 2010a). Inadequate sample sizes give rise to underpowered studies and increase the occurrence of false positive and false negative findings. Only a handful of software packages or programs are available to compute sample size and power for G-E interaction studies (Dempfle et al. 2008). For a simple interaction model between a single genetic variant and binary or continuous exposure, (Murcray et al. 2011) derived the sample size required to achieve 80% power, for a variety of G-E interaction tests, while correcting for multiple testing at the genome-wide level. Their study clearly shows that for moderate to low effects, the required sample size for classical tests is likely to be extremely large, larger than for similar tests of marginal effects with the same amplitude. Obviously, the improved efficiency (increasing power while keeping the same sample size) by using one methodology over another, will highly depend on the mode of interaction. Simulation strategies such as the one developed by Amato et al. (2010), accommodating non-linear interactions, may further help in elucidating the scenario’s in which a particular method performs best. Unfortunately, most studies deriving sample size and power calculations in simulated data assume no error in the assessment of genetic factors nor environmental factors, whereas these are known to induce power loss (Garcia-Closas et al. 1999; Tung et al. 2007). It leaves no doubt that there is still room for additional simulation strategies of G-E interactions, allowing for differential modes of interaction, that are flexible to incorporate some of the complicating factors mentioned above.
Methods
Defining aims and fitting the context
We have compiled a list of papers which define or explore (via simulation or theoretic development) the properties of methods for investigation of gene environment interactions (Table 1). The methods papers listed cover a range of study designs from family-based to case-control to case-only methods. While not exhaustive, the list covers the majority of such research papers published prior to development of this review. In particular, the entries of Table 1 address whether the method is applicable to gene-gene interactions, whether the method is tailored to GWA studies or candidate gene studies, and for which type of outcome the method is tailored (i.e, binary, continuous, etc). While many of the methods can be extended beyond what has currently been described, we limited our categorization to those situations explicitly discussed in the research paper. The table demonstrates the sheer number of methods that are available and illustrates the difficulty in determining which method is appropriate for a given study/situation. For many methods, there is no clear point of comparison or clear choice as to which method is superior.
Table 1
Overview of methods papers for studying of gene-environment interactions
Manuscript | Manuscript type1 | Marker Design2 | Subject Design3 | Outcome Type4 | Aim5 | Analysis Approach6 | Population Stratification Addressed | Applicable to Gene-Gene Interaction |
---|---|---|---|---|---|---|---|---|
Albrechtsen et al. (2007) | 2,3,4 | 1 | 3 | 1,2 | 1 | 2 | no | yes |
Andrieu et al. (2004) | 2,3 | 2 | 2,5 | 1 | 1 | 1 | no | no |
Aschard et al. (2011) | 2,3 | 1,2 | 2,3,4 | 1,2 | 1 | 1 | no | no |
Bureau et al. (2005) | 2,3,4 | 2 | 2 | 1 | 1,5 | 2 | no | yes |
BůŽková et al. (2011) | 2,3 | 2 | 2 | 1,2 | 1 | 1,2 | no | yes |
Cattaert et al. (2010) | 2,3,4 | 2 | 4,5 | 2 | 1,3,5 | 2 | no | yes |
Chanda et al. (2009a) | 2,3,4 | 2 | 1,2 | 2 | 1,3,5 | 2 | no | yes |
Chanda et al. (2009b) | 2,3,4 | 2 | 2 | 1, 5 | 1,3 | 2 | no | yes |
Chanda et al. (2008) | 2,3,4 | 2 | 2 | 1, 5 | 1,5 | 2 | no | yes |
Chanda et al. (2007) | 2,3,4 | 2 | 2 | 1,5 | 1,3 | 2 | no | yes |
Chatterjee et al. (2006) | 2,3,4 | 2 | 1,2 | 1,2 | 1,2 | 2 | no | yes |
Chatterjee et al. (2005) | 2,3 | 1,2 | 5 | 1 | 1,2 | 1 | yes | no |
Chen et al. (2009b) | 2,3 | 2 | 1,5 | 1 | 1,2 | 1 | yes | no |
Chen et al. (2008) | 2,3 | 2 | 2 | 1 | 1 | 1 | yes | no |
Chen et al. (2007) | 2,3,4 | 2 | 2 | 1 | 1,2,5 | 2 | no | yes |
Cheng (2006) | 2,3,4 | 1,2 | 4 | 1 | 1,2 | 1 | no | 2 |
Cordell et al. (2004) | 2,3 | 1,2 | 5 | 1 | 1 | 1,2 | no | yes |
Culverhouse et al. (2004) | 2,3,4 | 2 | 4 | 2 | 1,3,5 | 2 | no | yes |
Dai et al. (2010) | 2,3 | 1,2 | 2,3 | 1,2 | 1 | 1 | no | no |
Efird (2005) | 2,3 | 2 | 2 | 1 | 1,2 | 1 | no | no |
Fan et al. (2011) | 2,3,4 | 2 | 2 | 1 | 1,3,5 | 2 | no | yes |
Fardo et al. (2011) | 2,3 | 2 | 5 | 1,2 | 1 | 1 | yes | no |
Gauderman et al. (2010) | 2,3 | 1,2 | 5 | 1 | 1 | 2 | no | no |
Gauderman et al. (1997) | 2,3 | 3 | 5 | 2 | 1 | 2 | no | no |
Geneletti et al. (2011) | 5 | 2 | 4 | 1 | 1 | 1 | no | no |
Gu et al. (2009) | 2,3,4 | 1,2 | 4 | 1 | 2 | 1 | yes | no |
Hoffmann et al. (2009) | 2,3 | 1,2 | 5 | 1 | 1 | 1 | yes | no |
Kazma et al. (2011) | 2,3 | 1,2 | 4 | 1 | 1,2 | 1 | no | no |
Kraft et al. (2007) | 2,3 | 1,2 | 2,4 | 1,2 | 1,2 | 1 | no | no |
Hothorn et al. (2006) | 2,4 | 3 | 1,2 | 1,2,3,4 | 1,3 | 2 | no | yes |
Lake et al. (2004) | 2,3 | 1,2 | 5 | 1 | 1 | 1 | yes | no |
Lee et al. (2006) | 2 | 2 | 1,4 | 1 | 1 | 1 | no | no |
Li et al. (2009) | 2,3,4 | 1,2 | 2 | 1 | 1,2 | 1 | no | no |
Lim et al. (2005) | 2,3 | 2 | 5 | 1 | 1 | 1 | yes | no |
Lobach et al. (2011) | 2,3,4 | 1,2 | 2 | 1 | 1 | 2 | no | no |
Lou et al. (2008) | 2,3,4 | 2 | 5 | 1,2 | 1,3,5 | 2 | no | yes |
Mahachie John et al. (2011) | 2,3 | 2 | 4 | 2 | 3, 5 | 2 | no | yes |
Maity et al. (2009) | 2,3,4 | 2 | 2 | 1 | 1,2 | 1 | no | no |
Manning et al. (2011) | 2,3 | 1,2 | 2,3,5 | 1,2 | 1 | 1 | no | no |
Mi et al. (2011) | 2,4 | 2 | 5 | 1,2 | 2 | 1 | no | no |
Moerkerke et al. (2010) | 2,3 | 2 | 5 | 1 | 1 | 1 | yes | no |
Mukherjee et al. (2007) | 2,3,4 | 2 | 2 | 1 | 1,2 | 1 | yes | no |
Mukherjee et al. (2008) | 2,3,4 | 1,2 | 2 | 1 | 1,2 | 1 | yes | no |
Mukherjee et al. (2010) | 2,3,4 | 2 | 2 | 1 | 1,2 | 1 | no | no |
Paré et al. (2007) | 2,3,4 | 1,2 | 1,3 | 2 | 1 | 2 | no | yes |
Ritchie et al. (2007) | 2,3 | 2 | 2 | 1 | 1,3 | 2 | no | yes |
Schaid (1999) | 1,2 | 2 | 2,5 | 1 | 1,3 | 1 | yes | no |
Struchalin et al. (2010) | 1,2,3 | 1,2 | 3,4 | 2 | 1 | 1 | no | no |
Tan et al. (2006) | 2,3 | 2 | 5 | 1 | 1,3 | 1 | no | yes |
Tanck et al. (2006) | 2,3,4 | 2 | 5 | 2 | 1,2 | 2 | no | yes |
Tchetgen Tchetgen et al. (2010) | 2,3 | 2 | 1 | 1 | 1 | 1 | no | yes |
Tzeng et al. (2011) | 2,3,4 | 2 | 4 | 2 | 1,3,5 | 2 | yes | yes |
Umbach et al. (2000) | 3,5 | 2 | 5 | 1 | 1,5 | 1 | yes/no | no |
Van Der Sluis et al. (2008) | 2,3 | 2 | 5 | 2 | 1,3 | 1 | yes | no |
Tweel et al. (2004) | 2,3,4 | 2 | 2 | 1 | 1,2 | 1 | no | yes |
Vansteelandt et al. (2008) | 2,3 | 2 | 5 | 1,2 | 1 | 1 | yes | no |
Wakefield et al. (2010) | 2,3,4 | 1,2 | 2 | 1 | 1 | 2 | no | yes |
Wang et al. (2008) | 2,3,4 | 2 | 2 | 1 | 1 | 1 | no | yes |
Witte et al. (1999) | 3 | 2 | 2,5 | 1 | 1,3 | 1 | yes/no | no |
Wu et al. (2009) | 2,3 | 2 | 2 | 1 | 1 | 1 | no | no |
Wyszynski et al. (2001) | 5 | 2 | 1,5 | 1 | 5 | 1 | no | no |
Yoshida et al. (2011) | 2,3,4 | 2 | 2 | 1 | 1 | 2 | no | yes |
Yu et al. (2012) | 2,3,4 | 2 | 2 | 1 | 1,2 | 2 | no | yes |
Zhang et al. (2011) | 2,3,4 | 1,2 | 2 | 1,2 | 1 | 2 | no | yes |
We have categorized the methods in terms of several features related to the type of studies or data to which the methods are meant to be applied. Some features of some methods would benefit from slightly different categorizations; we chose these as they allow the vast majority of methods to described using similar terms. While many of the methods can or have been extended beyond what has currently been described, we limited our categorization to those situations explicitly discussed in each research paper. For example, some methods may be easily applicable to gene by gene interactions, but unless it was clear based on first principles or explicitly described in the paper, we labeled that paper “no.”
Explanation coding:
Naively, any data analysis can be decomposed in three tightly linked cornerstones: 1) the analysis type which is in a one-to-one correspondence with the problem type or research question, 2) the sampling design which aims to maximize the efficiency for a fixed number of individual, and 3) the (statistical) model or methodology which summarizes the (statistical) answer to the research question.
We do not address specifically the measurement type of the variables included, which is related in GWEI studies to traits, genetic markers and exposures. A discussion of the types of genetic markers (e.g., SNPs or CNVs) or measurement scales of exposure variables falls outside the scope of this work. We merely want to highlight that the most commonly used genetic markers used in GWEI studies are SNPs and that the most popular coding is additive, while other type of genetic variations such as CNV (e.g. Karageorgi et al. (2011)) or epigenetic markers are barely used. Related to the popularity of the case-control design, traits are often quantified via a binary variable (see also Table 1), although many quantitative traits have also been studied at the genome-wide scale. We discuss below study designs and statistical models that allow handling either binary or quantitative outcome or both.
Cornerstone 1: Research problem
Methodological requirements for identifying G-E interactions are largely driven by the research question and the viewpoint. From a public health perspective, the objective will usually be testing for genetic variant while allowing for interaction or testing for public health interactions (Siemiatycki and Thomas 1981). In such a situation one may use analytic methods making assumptions about the functional form of models and/or effects being modeled and derive an appropriate test to derive effect size estimates and test the hypothesis of interest. In human genetics, two popular analysis types are linkage and association studies. G-E interaction studies in linkage studies may involve performing exposure stratified analyses (e.g., Colilla et al. (2003)) or G-E interaction testing strategies using sib-pairs (e.g., Dizier et al. (2003) for a review). Here, we will restrict attention to genetic association problems.
It is less clear what test of interaction is most appropriate when the goal of the study is to draw inference about biological mechanism. A significant test for interaction—whether from a multiplicative odds ratio model or additive absolute risk model for disease traits, or from additivity for log-transformed or untransformed continuous traits—need not imply biological interaction, just as biological interaction need not imply statistical interaction (Greenland 2009; Siemiatycki and Thomas 1981; Thompson 1991). The observed distribution of traits across the strata defined by genotype and exposure may be suggestive of underlying biological mechanism, but it is suggestive at most. Formally testing whether a hypothesized null interaction model is contradicted by observed epidemiologic data requires careful mathematical modeling of how the proposed biological mechanism would affect the observed trait distributions—and such modeling will always require untestable assumptions (Thompson 1991).
Cornerstone 2: Design
Similar to other epidemiologic studies, the success of G-E interaction studies largely depends on the selection of an optimal study design. Most common designs used for genetic association studies of main effect can be used to search for interactions. It includes family-based designs, such as nuclear families (parents and offspring) and sib designs (case and siblings), as well as common population-based designs, such as prospective cohorts and case-control data. Particular G-E interaction designs such as case-only designs have obtained increased popularity due to their properties and/or easy adoption. Randomized clinical trials are being curtailed to address the pharmacogenetic aspects of G-E interactions. However, the requirement of large sample sizes to achieve reasonable statistical power in genome-wide G-E interaction studies has catalyzed the development of more efficient designs over the last few years (Bookman et al. 2011). In the sequel, we briefly discuss some of the most popular designs. For a detailed summary of advantages and disadvantages of some of these designs in the context of complex trait gene-environment interaction studies we refer to Weinberg and Umbach (2000), Dempfle et al. (2008) and Thomas et al. (2010a).
Family-based designs can be of great interest for GWEI studies, since they usually require weaker assumptions on distributions of genetic and environmental factors than population-based designs (Liu et al. 2004). They can be robust against population stratification and can be more efficient when rare mutations are involved, although as aforementioned they still may subject to bias in some situations (Shi et al. 2011). Moerkerke et al. (2010) extended FBAT-I and established a test that is doubly robust. The approach is valid if either the model for the main genetic effect holds or if the model for the expected environmental exposure holds, but not necessarily both. Vansteelandt et al. (2008) used causal inference methodology to establish a family-based test for G-E interaction that is robust against unmeasured confounding due to population stratification and Fardo et al. (2011) extended that methodology to test for G-E interaction in family based studies with phenotypically ascertained samples.
Bias and efficiency of several family designs (e.g., using parents, siblings, cousins or “pseudo-sibs”) have been studied under a range of situations by many authors (Chatterjee et al. 2005; Cordell 2009; Schaid 1999; Whittemore 2007; Witte et al. 1999). However, there is no single design that fits all purposes or is optimal for all scenario’s, since utility and performance depend on disease prevalence, frequency of risk allele and risk exposure, underlying genetic model and modes of interactions, and on the goal of the study. For example, Chatterjee et al. (2005) showed some efficiency advantage of case-sibling designs compared to case-parent designs in a variety of settings. But the latter remains of interest for the estimation of the genetic association parameter (i.e. the odds ratio associated with the gene variant among subjects with environmental exposure).
Despite the advantages of family-based design, population-based design has been often preferred for genetic association studies. Ascertainment of non-relatives is logistically more convenient and potential population stratification can easily be estimated and controlled for in population-based data using genotype data from markers that are unlinked to the loci under study. Among possible population-based designs, cohort studies have long been recommended for G-E interaction studies (Clayton and McKeigue 2001). However, these remain extremely expensive and time consuming.
Moreover, cohort studies are of limited use for the investigation of very rare diseases, which may require unrealizable large sample sizes. Because of this drawback, the standard case–control design (either nested in a cohort design or derived from a retrospective study) rose as the gold standard for association studies of genetic main effects (Clayton and McKeigue 2001) and is widely used in gene-environment interaction studies. Case-control designs are also often preferred to partial-collection designs (e.g., case-only, case-parents), since they might offer a better compromise between cost and efficiency (Liu et al. 2004). Statistical tests that are built within this framework are robust to a range of assumptions, such as G-E independence (although see Lindstrom et al. (2009)). They generally allow unbiased estimation of all parameters that are of interest in the G-E study, although dealing with bias due to exposure misclassification remains challenging (see works from Garcia-Closas et al. for examples of impact on multiplicative interactions (Garcia-Closas et al. 1998) and impact on additive interactions (Garcia-Closas et al. 1999)).
The case-only design is probably the most discussed alternative to case-control data. It has been proposed as a less expensive design when the goal is to assess interaction effects only (Piegorsch et al. 1994; Umbach and Weinberg 1997). It relies on the assumption of independence between the genetic and environmental factor in the population. When this assumption is valid, departures from a multiplicative relative risk model can be evaluated by testing the association between G and E in cases only. This test (as well as other approaches that rely on G-E independence) has repeatedly been shown to be more efficient than other approaches. The flip side is that when the assumption does not hold, statistical tests based on cases only give rise to inflated type I error rates. Whether or not the aforementioned independence assumption is a reasonable one in GWEI settings is debatable. Artificial G-E dependencies can be created in multiple situations. Population stratification for example can create correlation between genotypes and environmental exposures in the study population (Chatterjee et al. 2005; Umbach and Weinberg 1997). Elbaz and Alperovitch (2002) have also shown that substantial correlation may appear between genetic risk factors and risk exposure of late-onset diseases in the presence of competing risks and interaction effects. Although bias in case-only designs is likely to be uncommon in practice (Dennis et al. 2011; Liu et al. 2004), using this particular design remains controversial (Albert et al. 2001). Moreover, several studies have shown that interactions opposite to the main genetic effect might not be captured within case-only data (Liu et al. 2004; Mukherjee et al. 2011).
Apart from the somewhat more traditional designs from the previous paragraphs, a range of alternative ascertainment schemes have been proposed in the literature, all with the aim to identify gene-environment interactions. Some of these designs include both related and unrelated controls (Andrieu and Goldstein 2004; Chen et al. 2009b) to increase power while others have addressed specific gene-environment interaction patterns. For example Chen et al. (2009b) proposed a two-stage study design where a case-only study is performed at the first stage, and a case-parent/case-sibling study is performed at the second stage on a random subsample of the first-stage case sample as well as their parents/unaffected siblings. Whittemore (2007) on the other hand, discussed potential designs in studies that attempt to assess associations between lifestyle or environmental exposures and disease risk in carriers of rare mutations. Andrieu et al.(2001) also addressed the issue of rare risk factors, considering either rare mutations or rare environmental exposure. They proposed the counter-matching design which consists in increasing the number of subjects with the rare factor without increasing the number of measurements that must be performed.
Cornerstone 3: Methodology
In the context of GWEI analyses, several analytical routes can be followed (Figure 2). Some of these roads to travel by are more “natural” with specific study designs (Table 1).
Parametric and semi-parametric approaches modeling approaches
Many researchers have built upon the comforting regression framework in developing customized approaches to detect G-E interactions, including ordinary regression, penalized regression (Park and Hastie 2008) and logic regression (Schwender and Ruczinski 2010). In general, the joint effect of a genetic variant G and a given exposure E on a phenotype Y is often defined with the simple model:
where G is the number of allele (coded 0,1,2), E is continuous or categorical, Z represent a set of covariates one may adjust for, β are the linear effects of each component and g() the link function is the logit for dichotomous Y and the identity for quantitative Y. This model is a simplification, in that it ignores possible dominance effects. Still, just as the additive model has good power over a wide range of possible dominance models and has become the primary test statistic used in most GWAS (Lettre et al. 2007), the additive main and interaction effects will be detectably non-zero for a wide range of true dominance models, and the proportion of variance explained by the missing dominance effects will be quite small for most models.
Simplification is common in classical frequentist approaches, where adding degree of freedom can reduce statistical power. Or to quote the parallel from Kooperberg and Leblanc (Kooperberg and Leblanc 2008) with a cake: “if we want to divide the power over all possible interactions, nobody will get more than a crumb, and no-one will taste how good the cake is; we are better off dividing the cake among those people we believe to enjoy it.” For example a saturated linear model for a trichotomous E will have nine degree of freedom (df) compared to four df for the equation (1). In fact the same strategy has been used in most GWAs of marginal effect for the same reason.
It is important to note that even a simple model as equation (1) may encounter statistical issues. Especially, recent works from Tchetgen Tchetgen and Kraft (Tchetgen and Kraft 2011) have shown that when the main effect of continuous E, βE in equation (1), is mis-specified, the likelihood ratio test, score test, and Wald test statistics of the main effect of G and the interaction effect can have incorrect type-1 error rates. This issue, which has been shown to be due to underestimation of the variance of βGE, can be solved using different techniques (Cornelis et al. 2011): a) using a more flexible model for the environmental main effect (e.g., adding quadratic and cubic term for the exposure); b) using a robust “sandwich” estimator of the variance and c) modeling a continuous exposure by using general categorical variables.
A Bayesian framework gives the opportunity to make a step further in modeling the complexity of interaction effects. It provides a rational and quantitative way to consider a range of hypothesis in a single analysis. For example, Bayesian methods can be used to consider simultaneously multiple genetic models, some of them including diverse interaction effects, and to evaluate the posterior probability of each of these models (e.g., Crainiceanu et al. (2009) and Zhang and Liu (2007)). They also allow for multiple assumptions, which can be used to build composite estimators. If one wants to quantify the relevance of the G-E independence assumption (discussed in further sections), they offer solutions to trade off between bias and efficiency in a data adaptive way (Li and Conti 2009; Mukherjee et al. 2010). Finally, they allow incorporating biological information and knowledge accumulated in previous association studies, so that interaction effects can be weighted by their plausibility. However, despite their potential advantages, Bayesian approaches have been only sparsely used in genetic association studies and their advantages and limits from a modeling point of view need to be studied further. In particular, many hypothesized models are likely to be roughly equally consistent with the observed data for realistic sample sizes, making it difficult to infer which model provides the best fit: the cake will be split among so many people that nobody will get more than a crumb.
Screening for variants involved in interaction when interacting factor are unknown
Most genetic variants having effect through interactions with other risk factors are also likely to display marginal linear effect. For example, using random parameters for model (1) to simulate data —specifically, generating main effects and interaction effects independently of each other— will produce genetic variants with marginal effect almost 100% of time. This suggests one can simply test for marginal effect with power being almost only related to sample size, unless (as discussed below) the state of nature is such that most true models include interaction effects, but these are offset by the main effects so that the marginal genetic effects are quite small. This is especially useful if potential interacting factor are unmeasured or when interaction effects are expected to be difficult to assess.
Interaction models with small or no marginal genetic effects are theoretically possible(Culverhouse et al. 2002; Song et al. 2010). If such interactions are common then this will have significant consequences for how we go about searching for the genetic basis of complex phenotypes and will obviously limit the interest of screening for marginal effect. However such models have not yet been observed and confirmed in real data. This has led some to suggest that increasing sample size and testing for the marginal linear effect in agnostic GWAs scans might be the most powerful approach in most cases, while using more complex models might have only limited advantages (Clayton and McKeigue 2001; Hirschhorn and Daly 2005; Wang et al. 2005). The large success of this strategy in detecting genetic variants in GWAs has provided arguments in this direction, but the small amount of heritability explained by the “GWAs variants” is a potential rebuttal to the efficiency of this strategy.
When searching for quantitative trait loci (QTLs) an alternative for screening for the presence of interactions without using potential interacting factors is to test for homogeneity of variances across genotypic classes (Pare et al. 2010). The rationale is that, if the magnitude and the direction of the effect of a QTL differ depending on other genetic or non-genetic factors, the variability of the phenotypic outcome among individuals carrying the risk allele is likely to be larger than among the non-carrier. Hence, under the assumption that the main effect of the QTL affect neither the within genotype variance nor the between genotype variance, testing for heteroscedasticity will test for the presence of potential interactions. Note that heterogeneity of variances may be explained not only by the presence of interactions, but also by other biological mechanisms or other association patterns such as linkage disequilibrium with variants with large effect size (Takeuchi et al. 2010). Simulation studies have shown that the power of the test was limited when applying genome-wide significance threshold (Pare et al. 2010; Struchalin et al. 2010). It is also highly dependent on the main effect of the unknown interacting factors, having an optimal power for specific magnitude of main effect of E (Struchalin et al. 2010). Despite these limitations, testing for homogeneity of variance can be built in a two step approach. Since the power of the test, which highly depends on the main effect of the unknown interacting factors, is limited when applying genome-wide significance threshold, testing for homogeneity of variance has been proposed to preselect variants of interest that can be tested further for interactions. The potential of this approach has been recently demonstrated in a genome-wide association study of C-reactin and soluble ICAM-1 conducted in the Women’s Genome Health Study (Pare et al. 2010). Interestingly one of the identified GxE interaction was replicated in an independent study (Dehghan et al. 2011).
Leveraging interaction effect to improve detection of marginal effect
When a locus is expected to have residual marginal effects conditional on others factors tested for interaction, an efficient strategy is to use composite null hypothesis where both main effect and interaction effects are tested jointly (Kraft et al. 2007). Explicitly, testing the null hypothesis that the genetic variant has no effect in any strata or based on equation (1) H0: βG =0 or βGE =0. This can be done by using a multivariate Wald test or a likelihood ratio test comparing a model including effect of E and Z only versus a model including effects of G, GE, E and Z. A simple alternative when exposure is binary or categorical is to test for marginal genetic effect in strata defined by exposure E. The joint test can then be computed as the sum of chi-squared for association derived from each stratum. Since the samples are independents, the sum follows a chi-square with the degree being equal to the of strata for E.
For case-control studies, the test for such joint effect can be performed using standard logistic regression, the more powerful retrospective likelihood approach (Chatterjee and Carroll 2005; Cornelis et al. 2011) can exploit an underlying gene-environment independence assumption or using the empirical Bayes approach (Chen et al. 2009a; Mukherjee and Chatterjee 2008) that can data adaptively relax the independence assumption. An extension from the family-based test for the joint test of gene main effect and G-E interaction (FBAT-J) has been recently proposed for dichotomous traits in trios and sibships (Hoffmann et al. 2009). The test assumes the genotype and the environment are independent conditional on the parental mating type. If the assumption does not hold, the test will have an inflated type I error rate (Weinberg and Umbach 2000).
By allowing for heterogeneous genetic effect among genetic or environmental strata one can maximize the statistical power to detect the locus while minimizing the loss of power when genetic effect is homogeneous. Simulation studies have shown that a joint test for a main genetic effect and interaction effect is likely to have higher statistical power than the marginal test or the standard one degree of freedom test in presence of moderate interaction effect or when interaction effect are in opposite direction to the main effect (Kraft et al. 2007). Conversely, in the presence of a small interaction effect, the marginal test may conserve the highest power.
Methods for meta-analysis of multiple parameters have been recently described so that estimates of effects from the joint test can also be combined across independent sample. In particular, Manning et al (2011) have described a general approach, while Aschard and colleagues (2011) have extended the aforementioned principle of analyzing sample stratified by environmental factors. The first approach should be used when analyzing quantitative exposures and in situations where the samples within each cohort have to be analyzed as a whole (e.g. in family data where one has to account for correlation among individuals). The second approach essentially offers practical advantage and it can be more flexible in situations where environmental categories may differ among the cohort analyzed. The first genome-wide application of the joint test has been published recently by Hazma et al (2011). They identified a new genetic variant associated with Parkinson’s disease and replicated the signal in independent samples.
As any test modeling interaction effect per se, the joint test is limited by the multiple testing issues in large scale data. Hence, it is only applicable in situations where there is a measured factor that might interact with the tested locus. Nevertheless some have shown that the joint test can be built in framework where multiple potential effect modifiers can be considered for a single locus. Strategy for testing can then be defined by averaging the effect of a given locus over other factors (Ferreira et al. 2007) or by testing the maximum joint test over a range of possible model (Chapman and Clayton 2007). It has been also suggested that degree-of-freedom for such joint tests can be reduced using Tukey style one-degrees-of-freedom model for interaction between groups of related genetic or/and environmental variables (Chapman and Clayton 2007; Chatterjee et al. 2006; Ciampa et al. 2011).
Testing for interaction per se
Besides TDT-like extension for G-E interaction as FBAT-I and its extension (Hoffmann et al. 2009; Lake and Laird 2004; Moerkerke et al. 2010) that are applicable to nuclear families data only, the traditional test for interaction consists in evaluating the term βGE from equation (1). This test is relatively robust compared to many other approaches, although as described previously, misspecification of the main effect of a continuous E may increase type I error rate. The main concern when applying this simple test in GWAs data is its limited power (see “power” section above). Two types of strategies have been discussed to increase detection: a) to use multi-stage approaches to reduce multiple testing burden; and b) to leverage additional assumption on the data analyzed to improve efficiency.
Since the seminal paper from Marchini et al. (2005), multi-stage approaches using sequential test are considered as realistic approaches in GWAs. Even if not demonstrated, their work suggests that such strategy may improve the power of identifying interaction effects in GWAs. Since then diverse analysis strategies have been proposed, most of them focusing on the gene-gene interaction which face a strong multiple testing issues in GWAs. However these approaches can also be applied in the context of G-E. Examples include screening on genetic marginal effects (Kooperberg and Leblanc 2008; Macgregor and Khan 2006), or screening on a test that models the G-E association induced by an interaction in the combined case-control sample (Murcray et al. 2009). Simulation studies suggest that such approaches can be more powerful than traditional single-stage approach in which a huge penalty needs to be paid for multiple testing. Using a two-step strategy allows for less stringent thresholds of significance in the second step, since genetic markers have been prioritized in step one for their likely involvement in G-E interactions. While these methods became popular, questions have risen on how power and type 1 error are influenced by the correction among the two steps. While the two stages have been shown to be virtually independent in simulation study when screening on marginal effect (Kooperberg and Leblanc 2008; Marchini et al. 2005), recent work from Dai et al. (2010), provides proof of asymptotic independence of marginal association statistics and interaction statistics in linear regression, logistic regression, and Cox proportional hazard models when analyzing rare disease. Hence, in many situations the family-wise type I error rate might be controlled using classical Bonferroni correction for number of interaction tested at the second step only or by using permutation when markers considered at the second step are correlated.
Making assumption about the data analyzed to increase power of statistical test is a common principle. For binary trait such as disease status, the most popular one is the G-E independence assumption that allows testing for interaction in case-only data by testing for association between G and E among the cases using
Under the assumption of G-E independence in the whole population or G-E independence in controls for rare disease, testing for H0: γE = 0 is equivalent to testing for H0: βGE = 0 from equation (1). When the assumption holds this method has the maximum power compared to most other approaches that leverage the G-E independence, except in the situation where the main effect of G or E is in opposite direction to the interaction effect (Mukherjee et al. 2011; Murcray et al. 2011). However it has also disadvantages: the main effect of G and E cannot be estimated and the type I error can be highly inflated when the assumption does not hold.
A range of other approaches have been proposed to leverage this assumption while providing a trade-off between increased power and controlled type I error rate (Chatterjee et al. 2005; Chen et al. 2009a; Cheng 2006; Mukherjee and Chatterjee 2008; Mukherjee et al. 2007). For example, when data on both cases and controls are available in a study, then one can be much more flexible than case-only analysis in studies of gene-environment interaction whether or not the independence assumption is valid. One can use a retrospective likelihood approach (Chatterjee et al. 2005) under the gene-environment independence assumption to obtain very efficient estimate all of the parameters of a general logistic regression model. On the other hand, if violation of the gene-environment independence assumption is suspected, one can perform data adaptive methods such as an empirical Bayes technique (Chen et al. 2009a; Mukherjee and Chatterjee 2008), which can be robust to violation of the independence assumption and yet can be more powerful than traditional case-control analysis when the independence assumption is valid. Other alternatives to the case-only test include multi-step approaches in a single sample (Gauderman et al. 2010; Murcray et al. 2009), multi-sample design (Chen et al. 2009b), and approaches that use Bayesian framework (Li and Conti 2009; Mukherjee et al. 2010). One should note that, based on recent reports, differences in performances between these methods only exist at the margin and they always depend on the type of model simulated (see Mukherjee and Chatterjee (2008) for a detailed comparison of several of these methods).
Exploratory or agnostic approaches
Traditional statistical methods such as multivariable linear or logistic regression are ill-equipped to incorporate all possible pairwise interactions among a large number of markers and exposures, let alone higher-order interactions. However, for complex diseases or traits the influence of non-linear or higher-order gene-gene and G-E interactions may be appreciable. Therefore, researchers are faced with difficult decisions to make their analysis practically feasible within computational and modeling restrictions (Maenner et al. 2009). The common alternative is to move away from the classical hypothesis testing framework and estimation of statistical significance level, and to use “model free” approaches or to adopt an agnostic approach to identify gene-environment interactions. Different analysis approaches from machine learning or data mining are needed to manage the high dimensionality of genome wide analysis studies and large scale data collections.
Interdisciplinary collaborations have led to the adoption of approaches from one community to another, especially in the field of gene-gene interactions. These include data segmentation methods (Tryon 1939), tree-based methods (Breiman et al. 1984), pattern recognition methods (Ripley 1996) and (non-)linear dimension reduction methods (Fodor 2002). A list of examples of these in the context of gene-gene interactions is given in Van Steen (Van Steen 2012). Unfortunately, the adoption of these methods in genome-wide based G-E interaction detection is not as “frequent” as it is genome-wide epistasis studies. In the following, we elaborate on two techniques that deserve more attention in the context of GWEI studies: Tree-based and Multifactor Dimensionality Reduction derived techniques.
Because the number of possible genetic model can be quite large, exploratory methods are often built on a trade-off and assume or favor some specific interaction models. Recursive partitioning approaches, such as Random Forests (Breiman et al. 1984; Schwarz et al. 2010) – a flexible and efficient data mining method based on regression or classification trees – also face such issues. Random Forests do not model interaction variables per se but they allow for interactions (or complex non-linear relationships) in the sense that they evaluate classification ability of particular combination of values taken by sets of predictor variables. Because of the independence assumption used during node splitting of “trees” these methods have been shown to have limited ability to detect pure interaction effects (McKinney et al. 2009). Notably, the recent SNPInterForest approach (Yoshida and Koike 2011) performed very well in successfully identifying pure epistatic interactions with high precision and was still more than capable of concurrently identifying multiple interactions under the existence of genetic heterogeneity. Hence, extensions that relax the independence assumption within a conditional inference framework (Hothorn et al. 2006) and improved procedures to extract interaction patterns from Random Forest (Yoshida and Koike 2011) make the Random Forest methodology particularly attractive for GWEI studies. Different variable importance measures have been proposed in the literature, including a joint importance measure which extends the idea of single importance to multiple importance and can be useful especially for interactions (Bureau et al. 2005). Note that correlated predictors and varying predictor categories or measurement scales are likely to exist in G-E studies and that care needs to be taken in the selection of the importance criterion. For instance, Strobl et al. (2008) identified the mechanisms causing the bias for permutation importance scores and developed a conditional variable importance which reflects the true impact of each predictor variable more reliable than the original permutation variable importance measure.
As an application example, Maenner et al. (Maenner et al. 2009) analyzed coronary heart disease cases from the Framingham Heart Study by firstly identifying influential SNPs for age of onset of early coronary heart disease using a random forest approach. Variable importance scores from a RF analysis provide measures to determine important SNPs and environmental exposures taking into account interactions without specifying a genetic model (Lunetta et al. 2004). Secondly, generalized estimating equations were used to evaluate the statistical significance of main effects and interactions of previously detected SNPs and smoking status (Maenner et al. 2009) (however note that such significance level should be take with caution since the selection at the “mining step” potentially overfits the data). The authors used a simple solution to handle family structure within their data by considering a binary family indicator as covariate for building the random forest. Similarly, Zhai et al. (2011) performed a 2-step approach with initial screening for SNPs associated to environmental measures by random forest and further analysis based on case-only logistic regression to obtain parameter estimates for the selected variables.
Tree-based methods might be an relevant alternative to logistic regression methods for identifying genes without strong marginal effects and of robustness to genetic heterogeneity where different subsets of genes can lead to a phenotype of interest (Lunetta et al. 2004). Random forests outperformed Fisher’s exact test when several risk SNPs interact (Lunetta et al. 2004) and behaved more robust when a high number of unassociated noise SNPs is present (Bureau et al. 2005). Another interesting approach combining regression models and tree-based methodology is a semi-parametric regression model, named partially linear tree-based regression model (PLTR) (Chen et al. 2007). The linear regression part of their model can control efficiently for confounders and provides the possibility to correct for linear main effects of variables so that a parsimonious summary of the joint effect of genetic and environmental variables is obtained.
Also non-parametric data mining methods such as Multifactor Dimensionality Reduction (MDR) (Ritchie et al. 2001), are the subjects of a trade-off. In contrast to logistic regression and random forests, MDR can be used to detect G-E interactions in the absence of any main effects. MDR can be applied to smaller sample sizes than logistic regression which needs enough observations to model all main and interactions effects. However, the “reduction” step consists in splitting the different combination of two variables (defined by E and G) in two groups of high risk versus low risk. This allows a range of model to be tested. But the interaction is summarized in a single binary parameter and is therefore unlikely to capture the full complexity of interactions (e.g., a gradient of effect across different combinations). Several extensions and variations of the MDR method have been proposed to address initial shortcomings of MDR (including the lack of correction for lower order effects, and the too stringent reduction into two risk groups). Model-Based MDR (MB-MDR) (Calle et al. 2010) and its extension to family data, family MDR (FAM-MDR) (Cattaert et al. 2010), enables adjustments for possible confounders and the handling of various phenotypes, e.g., continuous, categorical or censored. In particular, MB-MDR uses a reduction into a one-dimensional variable with three levels, i.e. high risk, no evidence, low risk, and potentially a continuum of risk groups (Calle et al. 2010; Cattaert et al. 2010). While comparing MB-MDR to MDR in the presence of noise, i.e. genotyping error, phenocopy and genetic heterogeneity, MB-MDR was found to have increased power in most situations, especially for genetic heterogeneity, phenocopies and minor allele frequencies. Previous to applying the MB-MDR method, FAM-MDR uses a preparation step where familial correlation free traits are obtained as residuals from a polygenic model (hence, hereby adjusting for potential population stratification). FAM-MDR outperformed Pedigree-based MDR (PGMDR) (Lou et al. 2008) in terms of handling multiple testing, empirical power and efficient use of available information from complex and extended pedigrees (Cattaert et al. 2010) and is therefore a promising alternative to the classical MDR derivatives to explore gene-environment interactions. One disadvantage of MDR is that its computational burden increases with the number of SNPs and the order of considered interactions. A parallel algorithm of MDR and MB-MDR has been implemented by Bush et al. (2006) and Van Lishout et al. (2011), respectively. Despite these efforts, filtering methods to preselect a subset of candidate factors and stochastic search algorithms (e.g., simulated annealing and evolutionary algorithms) are needed to assist researchers in the exhaustive search for interactions in genome-wide association studies. Knowledge about the pros and cons of these filtering approaches (as applied to genome-wide epistasis settings) will be most beneficial for GWEI studies and the availability of an entire exposeome.
Duell et al. (2008) compared MDR to focused interaction testing framework and logistic regression for identification of higher-order interaction effects in a case-control study using 26 polymorphisms and smoking as possible environmental risk factor. Little concordance existed between MDR and interaction testing framework with regard to the interaction factors. This finding may be caused by the different interaction modeling methodologies behind the approaches. The authors recommend using multiple approaches for data screening and analysis to detect potentially new risk factor combinations. More comparative studies are actually needed, examining differences between traditional (often regression-based) approaches with untraditional (often data-mining) methods in the context of GWEI studies. The study from Duell et al.(2008) also highlight the difficulties in computing a comprehensive significance level for exploratory methods. Overall, one should remind that there is no straightforward way to define a null hypothesis and to test it in these exploratory approaches. However strategies to statistically evaluate the significance of models obtained through data mining procedures are now discussed in the literature (e.g. (Pattin et al. 2009)) and more might be developed in the future.
Out-of-the-box approaches
Information theoretic metrics allow for complex interactions between genetic variations and environmental factors without any modeling but have not yet been widely applied. Based on the Total Correlation Information (TCI) (Chanda et al. 2007), Chanda and colleagues (Chanda et al. 2008) developed the Phenotype-Associated Information (PAI), which is robust against dependencies between environmental and genetic factors. Furthermore, these authors suggest a greedy search algorithm (AMBIENCE) where potential variable combinations associated with a phenotype of interest are selected based on lower order PAI values and the interaction between the determined relevant variable subsets is re-evaluated using the more parsimonious k-way interaction information. This approach is particularly suitable for large scale data sets. The method was extended to quantitative traits (Chanda et al. 2009a), when normally distributed within each strata of the gene-environmental variable combination. Wu et al. (2009) and Fan et al. (2011) used test-statistics developed from information theoretic metrics to detect G-E interactions associated with discrete phenotypes. While the mutual information based test statistic of Wu et al. (2009) is applicable to two-way interactions, Fan et al. (2011) also consider higher order interactions. An extension of their computationally efficient approaches to quantitative traits and family data would increase the applicability and flexibility of information theoretic metrics further.
To prioritize genetic and environmental variables for follow-up sequencing studies, Chanda et al. (2009b) proposed to calculate the interaction index (defined as the sum of the average interaction contribution of each considered k-th order interaction for the given variable) for each variable. Comparing their approach to the Restricted Partitioning Method (RPM) (Culverhouse et al. 2004), Chanda et al. (2009b) find high concordance between the two methods for one-variable combinations but not for two-variable combinations. In contrast to for instance MDR and RPM, the greedy search algorithm AMBIENCE (Chanda et al. 2008) allows for higher dimensional datasets but disables the detection of pure epistasis effects. An alternative approach to the search algorithm might be to use an information-theoretic metrics as objective function in a dimensionality reduction method as MDR for which variables could be pooled into high-risk and low-risk sets based on their PAI value (Fodor 2002).
Recently, rule based classifier algorithms have been introduced in the context of genetic interaction studies, whereas they had proven their utility non-genetic datasets in the past (Tan et al. 2006). Rule based classifiers generate classification models using a collection of “if … then …” rules. The algorithms are computationally feasible, and allow the inclusion of both categorical and continuous variables. For a comparison of rule based classifiers in the context of G-E interactions, we refer to Lehr et al. (Lehr et al. 2011).
Alternatively, GWEI studies may benefit from neural networks (NN) (Gunther et al. 2009) and their modifications, e.g., genetic programming neural networks (GPNN) (Ritchie et al. 2007) and grammatical evolution neural networks (GENN) (Motsinger et al. 2006).
Unlike logistic regressions, Neural Networks do not explicitly use interaction terms for modeling data. There is no easy way to assess whether interaction is present using a neural network, nor to derive clear interpretations of estimated weights (Gunther et al. 2009). The GPNN algorithm attempts to generate optimal neural network architecture for a given data set, and – in contrast to classical NN – does not rely on the pre-specification of inputs and architecture (Ritchie et al. 2007). Although these types of approaches are often regarded as a black box, the flexibility of Neural Network- based approaches in model development clearly is a major advantage, especially when highly complex data structures with challenging gene-gene or G-E interaction structures need to be modeled.
GWEI and GWAI studies
Large-scale G-E interaction studies and large-scale gene-gene interaction studies, via the common genetic component they involve, share quite a number of challenges: high-dimensionality, computational capability, the absence/presence of marginal effects, the multiple testing problem, and genetic heterogeneity. These challenges, and possible solutions in the context of GWAI studies have been discussed elsewhere (Van Steen 2012).
When environmental risks are investigated, usually the focus is on a single exposure or several exposures from particular category, for instance involving air and water pollution, occupation, diet, stress and behavior, or types of infection. However, in the context of a genome-wide screen for loci involved in interactions, a marker may interact with an exposure from any category, or multiple exposures within or across categories. The effect of a marker may differ across strata defined by more than one exposure (e.g. the effect of a breast cancer marker might be different among women with a high Gail score, which summarizes several non-genetic breast-cancer risk factors, and women with a low Gail score). Along those lines, it is believed to be crucial to combine the genome with an entire “exposome” (i.e., the totality of environmental exposures from conception onwards) (Wild 2005). This idea is similar to evaluating the effects of genetic variants in a particular genetic background, as summarized by high-dimensional genetic data (Phillips 2008; Tzeng et al. 2011; Van Steen 2012). Methods for the measurement of the “expososome” are lagging far behind methods for measuring genomic variation. However, instead of characterizing the entire exposome, it should be feasible to identify at least critical components at several stages in an individual’s life and consider these in the G-E analysis (Rappaport and Smith 2010). The Bayesian paradigm is promising in this sense, since latent variables can potentially be used to capture genetic variation and models can be developed allowing environment effects to vary across different genetic profile categories (Yu et al. 2012).
GWEI studies may benefit from the abundance of methodologies that are available in the context of large-scale genetic association or epistasis screenings (Khoury and Wacholder 2009). We believe that there are several reasons for the limited translation of GWAI to GWEI methodologies. First, genome-wide G-E interaction studies have only recently become possible through several organized large-scale data collections (Davis and Khoury 2007) containing both genetic and good quality environmental measurements. Still, germline variation are static and can be captured at any time point, while exposures can change over time and are not always measured at the relevant time period (measurement at baseline or at interview may not reflect the relevant windows of exposure and will not reflect lifetime exposure). Hence some GWAI methods are likely to be underpowered since they are not designed to account for such variations. Second, GWEI studies involve factors that are measured on different scales. GWAI studies usually involve one type of genetic markers that have been pre-processed and underwent high quality control procedures. The measurement type (coding) is regarded to be the same for all SNPs in the analysis. An environmental factor can be continuous, categorical, or binary, whatever reflects the true underlying nature best. Combining different measurement scales within one approach, and inclusion of factors with differential degrees of accuracy, measurement error or variability poses additional complications (e.g., in Random Forests approaches (Strobl et al. 2008; Strobl et al. 2007)). Third, for GWAI studies there is a consensus on how to deal with missing genotypes. Several procedures have been developed to “impute” missing data in this context, for instance using HapMap reference data. Clearly, the taxonomy of Little and Rubin (Little and Rubin 1987) and bio-statistical knowledge about missing data handling in epidemiology, now needs to be combined with missing data handling techniques commonly adopted in statistical genetics. We refer to recent work of Lobach et al. (2011), that discusses exposure measurement error and genotype missing data in the context of a small-scaled gene-environment interaction analysis. Fourth, GWEI studies may face additional methodological challenges when the original GWA study is based on shared publicly available controls. It has been now well established that use of shared controls, after appropriate adjust for population stratification using principal component and related methods, produce valid inference for detection of genetic main effects. For studies of gene-environment interaction, however, one needs more caution as the exposure distribution for the underlying population of the controls may be quite different from the exposure distribution for the underlying population from which the cases were drawn. Further, data on relevant environmental exposures of interest may not often be available on publicly available studies. In such situation, while one can use a case-only analysis to examine multiplicative gene-environment interaction, but such inference is inherently limited as we have noted earlier. Fifth, meta-analytic approaches to boost power of GWEI studies are usually limited to parametric G-E detection methods that result in estimable effect sizes (Aschard et al. 2011; Manning et al. 2011). Model misspecification is one of the major concerns in meta-analysis contexts (Pereira et al. 2011; Pereira et al. 2009). General approaches are needed that require no assumption on modes of action in the meta-analytical context of GWEI studies. Finally meta-GWEI studies will further benefit from continuing efforts to improve the accuracy of epidemiological questionnaires of, medical records, occupational records, and other proxy measurements of environmental factors, as well as the development of low-cost, validated, and standardized environmental measures, (Bookman et al. 2011; Khoury and Wacholder 2009).
Future perspectives
The detection of G-E interactions is usually based on making inferences from statistical interactions that are observed at a population level, the most popular methodologies being based on regression paradigms. The most interesting types of G-E interactions are those that are coined “non-removable”, in the sense that the evidence of (statistical) interaction exists when no obvious monotone transformation of the trait exists (i.e., rescaling of the trait) that removes the interaction. Uher (2008) argued that concerns about statistical models and scaling can be addressed by integration of observed and experimental data. However, the aforementioned assumes that we already have identified “interesting” environmental risk factors. Most of these risk factors for common complex diseases have not yet been identified, and for those that have been identified, the mode of action is not well known. Moving from a hypothesis-driven to a hypothesis generating viewpoint (i.e., from a limited selection of candidate environmental risk factors to an exposome) magnifies some of the issues involved in interaction detection, with agents that may be highly structured or inter-connected in epidemiological or biological networks. Fortunately, lessons can be learnt from similar settings, such as those generated by GWAI data. Several efforts are being made to tackle some of the identified hurdles in this manuscript (Engelman et al. 2009) and a steady increase in GWEI studies is observed (refer to Figure 1). Although most of the identified interactions have not yet been confirmed, the first GWEI results suggest the importance of testing for G-E interactions. Adopting an interdisciplinary attitude and a systems biology view, using out-of-the-box strategies and non-linear mathematics that are less known in epidemiology (Knox 2010) may help identify interacting factors and better understand gene-environment interplay.
A G-E interaction effect in a population is dependent upon the distribution of genetic and environmental factors in the population of interest. Obviously, the distribution of environmental and genetic factors can be quite different between individuals and across populations. Thus, some observed G-E interaction effects, including those involving epigenetic phenomena, might be detected in one population but be absent in another. We wish to emphasize that in valid epidemiologic comparisons, controls should be a random sample of the population from which the cases arise. If a control were to become a case, would he or she be selected as a case in your study?
The availability of the entire sequence of the human genome offers enormous opportunities. It is now possible to obtain data on rare variants as well as common variants, for complex disease association studies. The effects on dimensionality are enormous, but Wray et al. (Wray et al. 2011) have argued that genes identified via GWA studies harboring common variants are likely to be good candidates for the identification of rare variants, which can then (theoretically) be investigated for their relationship with a disease trait. The role of rare variants (relative to more common variants) in complex disease etiology is still unclear. It has been proposed that multiple rare variants through LD may be responsible for some of the common variant hits from recent GWAS (so called synthetic associations); however, this has been deemed unlikely (Anderson et al. 2011). Large-scale sequencing efforts will be required to fully investigate the genetic architecture of complex disease etiology. Understanding how one or more rare variants may interact with each other and with environmental exposures will be an extremely difficult task to accomplish. Many thousands of participants will be required even to evaluate main effects of rare variants. The analysis of interactions between rare variants and environmental exposures will be very challenging for the same reasons it is difficult for common variants. Moreover, if we are willing to believe that most chronic diseases are a result of numerous subtle perturbations in exogenous and endogenous exposures and variation at the epigenomic level, then each individual may indeed have their own ‘personalized interactome’. This could have tremendous implications for the study of G-E and G-G interactions, and might help to explain why even very large consortium efforts have been unsuccessful at identifying more than a minor fraction of the heritability of disease.
Acknowledgments
H. Aschard and P. Kraft were supported by grant NIH/NIDDK - R21 DK084529. B. Maus and K. Van Steen acknowledge research opportunities offered by the Belgian Network BioMAGNet (Bioinformatics and Modeling: from Genomes to Networks), funded by the Interuniversity Attraction Poles Program (Phase VI/4), initiated by the Belgian State, Science Policy Office. Their work was also supported in part by the IST Program of the European Community, under the PASCAL2 Network of Excellence (Pattern Analysis, Statistical Modeling and Computational Learning), IST-2007-216886. E.J. Duell was supported by the Spanish Ministry of Health (ISCIII RETICC RD06/0020). The scientific responsibility for this work rests with its authors.
Footnotes
The authors declare that they have no conflict of interest.
References
- Albert PS, Ratnasinghe D, Tangrea J, Wacholder S. Limitations of the case-only design for identifying gene-environment interactions. Am J Epidemiol. 2001;154:687–93. [Abstract] [Google Scholar]
- Albrechtsen A, Castella S, Andersen G, Hansen T, Pedersen O, Nielsen R. A Bayesian multilocus association method: allowing for higher-order interaction in association studies. Genetics. 2007;176:1197–208. [Europe PMC free article] [Abstract] [Google Scholar]
- Amato R, Pinelli M, D’Andrea D, Miele G, Nicodemi M, Raiconi G, Cocozza S. A novel approach to simulate gene-environment interactions in complex diseases. Bmc Bioinformatics. 2010;11:8. [Europe PMC free article] [Abstract] [Google Scholar]
- Anderson CA, Soranzo N, Zeggini E, Barrett JC. Synthetic Associations Are Unlikely to Account for Many Common Disease Genome-Wide Association Signals. Plos Biology. 2011:9. [Europe PMC free article] [Abstract] [Google Scholar]
- Andrieu N, Goldstein AM. The case-combined-control design was efficient in detecting gene-environment interactions. J Clin Epidemiol. 2004;57:662–71. [Abstract] [Google Scholar]
- Andrieu N, Goldstein AM, Thomas DC, Langholz B. Counter-matching in studies of gene-environment interaction: efficiency and feasibility. Am J Epidemiol. 2001;153:265–74. [Abstract] [Google Scholar]
- Aschard H, Chen J, Cornelis M, Chibnik L, Karlson E, Kraft P. Inclusion of gene-gene and gene-environment interactions unlikely to dramatically improve risk prediction for complex diseases. Am J Hum Genet. 2012 Epub. [Europe PMC free article] [Abstract] [Google Scholar]
- Aschard H, Hancock DB, London SJ, Kraft P. Genome-wide meta-analysis of joint tests for genetic and gene-environment interaction effects. Hum Hered. 2011;70:292–300. [Europe PMC free article] [Abstract] [Google Scholar]
- Balansky R, Ganchev G, Iltcheva M, Nikolov M, Steele VE, De Flora S. Differential carcinogenicity of cigarette smoke in mice exposed either transplacentally, early in life or in adulthood. Int J Cancer. 2012;130:1001–10. [Abstract] [Google Scholar]
- Bashir SA, Duffy SW. Correction of risk estimates for measurement error in epidemiology. Methods Inf Med. 1995;34:503–10. [Abstract] [Google Scholar]
- Bhattacharjee S, Wang Z, Ciampa J, Kraft P, Chanock S, Yu K, Chatterjee N. Using principal components of genetic variation for robust and powerful detection of gene-gene interactions in case-control and case-only studies. Am J Hum Genet. 2010;86:331–42. [Europe PMC free article] [Abstract] [Google Scholar]
- Bookman EB, McAllister K, Gillanders E, Wanke K, Balshaw D, Rutter J, Reedy J, Shaughnessy D, Agurs-Collins T, Paltoo D, Atienza A, Bierut L, Kraft P, Fallin MD, Perera F, Turkheimer E, Boardman J, Marazita ML, Rappaport SM, Boerwinkle E, Suomi SJ, Caporaso NE, Hertz-Picciotto I, Jacobson KC, Lowe WL, Goldman LR, Duggal P, Gunnar MR, Manolio TA, Green ED, Olster DH, Birnbaum LS. Gene-environment interplay in common complex diseases: forging an integrative model-recommendations from an NIH workshop. Genet Epidemiol 2011 [Europe PMC free article] [Abstract] [Google Scholar]
- Bouzigon E, Corda E, Aschard H, Dizier MH, Boland A, Bousquet J, Chateigner N, Gormand F, Just J, Le Moual N, Scheinmann P, Siroux V, Vervloet D, Zelenika D, Pin I, Kauffmann F, Lathrop M, Demenais F. Effect of 17q21 variants and smoking exposure in early-onset asthma. N Engl J Med. 2008;359:1985–94. [Abstract] [Google Scholar]
- Breiman L, Friedman J, Olshen R, Stone C. Classification and Regression Trees. Chapman and Hall/CRC; 1984. [Google Scholar]
- Bureau A, Dupuis J, Falls K, Lunetta KL, Hayward B, Keith TP, Van Eerdewegh P. Identifying SNPs predictive of phenotype using random forests. Genet Epidemiol. 2005;28:171–82. [Abstract] [Google Scholar]
- Bush WS, Dudek SM, Ritchie MD. Parallel multifactor dimensionality reduction: a tool for the large-scale analysis of gene-gene interactions. Bioinformatics. 2006;22:2173–2174. [Europe PMC free article] [Abstract] [Google Scholar]
- Calle ML, Urrea V, Malats N, Van Steen K. mbmdr: an R package for exploring gene-gene interactions associated with binary or quantitative traits. Bioinformatics. 2010;26:2198–9. [Abstract] [Google Scholar]
- Carroll RJ, Ruppert D, Stefanski LA, Crainiceanu CM. Measurement Error in Nonlinear Models. Vol. 2. Chapman & Hall CRC Press; 2006. [Google Scholar]
- Cattaert T, Urrea V, Naj AC, De Lobel L, De Wit V, Fu M, Mahachie John JM, Shen H, Calle ML, Ritchie MD, Edwards TL, Van Steen K. FAM-MDR: a flexible family-based multifactor dimensionality reduction technique to detect epistasis using related individuals. PLoS One. 2010;5:e10304. [Europe PMC free article] [Abstract] [Google Scholar]
- Chanda P, Sucheston L, Liu S, Zhang A, Ramanathan M. Information-theoretic gene-gene and gene-environment interaction analysis of quantitative traits. BMC Genomics. 2009a;10:509. [Europe PMC free article] [Abstract] [Google Scholar]
- Chanda P, Sucheston L, Zhang A, Brazeau D, Freudenheim JL, Ambrosone C, Ramanathan M. AMBIENCE: a novel approach and efficient algorithm for identifying informative genetic and environmental associations with complex phenotypes. Genetics. 2008;180:1191–210. [Europe PMC free article] [Abstract] [Google Scholar]
- Chanda P, Sucheston L, Zhang A, Ramanathan M. The interaction index, a novel information-theoretic metric for prioritizing interacting genetic variations and environmental factors. Eur J Hum Genet. 2009b;17:1274–86. [Europe PMC free article] [Abstract] [Google Scholar]
- Chanda P, Zhang A, Brazeau D, Sucheston L, Freudenheim JL, Ambrosone C, Ramanathan M. Information-theoretic metrics for visualizing gene-environment interactions. Am J Hum Genet. 2007;81:939–63. [Europe PMC free article] [Abstract] [Google Scholar]
- Chapman J, Clayton D. Detecting association using epistatic information. Genetic Epidemiology. 2007;31:894–909. [Abstract] [Google Scholar]
- Chatterjee N, Carroll RJ. Semiparametric maximum likelihood estimation exploiting gene-environment independence in case-control studies. Biometrika. 2005;92:399–418. [Google Scholar]
- Chatterjee N, Kalaylioglu Z, Carroll RJ. Exploiting gene-environment independence in family-based case-control studies: increased power for detecting associations, interactions and joint effects. Genet Epidemiol. 2005;28:138–56. [Abstract] [Google Scholar]
- Chatterjee N, Kalaylioglu Z, Moslehi R, Peters U, Wacholder S. Powerful multilocus tests of genetic association in the presence of gene-gene and gene-environment interactions. American Journal of Human Genetics. 2006;79:1002–1016. [Europe PMC free article] [Abstract] [Google Scholar]
- Chen J, Yu K, Hsing A, Therneau TM. A partially linear tree-based regression model for assessing complex joint gene-gene and gene-environment effects. Genet Epidemiol. 2007;31:238–51. [Abstract] [Google Scholar]
- Chen YH, Chatterjee N, Carroll RJ. Retrospective analysis of haplotype-based case control studies under a flexible model for gene environment association. Biostatistics. 2008;9:81–99. [Europe PMC free article] [Abstract] [Google Scholar]
- Chen YH, Chatterjee N, Carroll RJ. Shrinkage Estimators for Robust and Efficient Inference in Haplotype-Based Case-Control Studies. Journal of the American Statistical Association. 2009a;104:220–233. [Europe PMC free article] [Abstract] [Google Scholar]
- Chen YH, Lin HW, Liu HM. Two-stage Analysis for Gene-Environment Interaction Utilizing Both Case-Only and Family-Based Analysis. Genetic Epidemiology. 2009b;33:95–104. [Abstract] [Google Scholar]
- Cheng KF. A maximum likelihood method for studying gene-environment interactions under conditional independence of genotype and exposure. Stat Med. 2006;25:3093–109. [Abstract] [Google Scholar]
- Ciampa J, Yeager M, Jacobs K, Thun MJ, Gapstur S, Albanes D, Virtamo J, Weinstein SJ, Giovannucci E, Willett WC, Cancel-Tassin G, Cussenot O, Valeri A, Hunter D, Hoover R, Thomas G, Chanock S, Holmes C, Chatterjee N. Application of a Novel Score Test for Genetic Association Incorporating Gene-Gene Interaction Suggests Functionality for Prostate Cancer Susceptibility Regions. Human Heredity. 2011;72:182–193. [Europe PMC free article] [Abstract] [Google Scholar]
- Clayton D, McKeigue PM. Epidemiological methods for studying genes and environmental factors in complex diseases. Lancet. 2001;358:1356–1360. [Abstract] [Google Scholar]
- Clayton DG. Prediction and interaction in complex disease genetics: experience in type 1 diabetes. PLoS Genet. 2009;5:e1000540. [Europe PMC free article] [Abstract] [Google Scholar]
- Colilla S, Nicolae D, Pluzhnikov A, Blumenthal MN, Beaty TH, Bleecker ER, Lange EM, Rich SS, Meyers DA, Ober C, Cox NJ, Asthm CSG. Evidence for gene-environment interactions in a linkage study of asthma and smoking exposure. Journal of Allergy and Clinical Immunology. 2003;111:840–846. [Abstract] [Google Scholar]
- Cordell HJ. Estimation and testing of gene-environment interactions in family-based association studies. Genomics. 2009;93:5–9. [Europe PMC free article] [Abstract] [Google Scholar]
- Cordell HJ, Barratt BJ, Clayton DG. Case/pseudocontrol analysis in genetic association studies: A unified framework for detection of genotype and haplotype associations, gene-gene and gene-environment interactions, and parent-of-origin effects. Genet Epidemiol. 2004;26:167–85. [Abstract] [Google Scholar]
- Cornelis MC, Agrawal A, Cole JW, Hansel NN, Barnes KC, Beaty TH, Bennett SN, Bierut LJ, Boerwinkle E, Doheny KF, Feenstra B, Feingold E, Fornage M, Haiman CA, Harris EL, Hayes MG, Heit JA, Hu FB, Kang JH, Laurie CC, Ling H, Manolio TA, Marazita ML, Mathias RA, Mirel DB, Paschall J, Pasquale LR, Pugh EW, Rice JP, Udren J, van Dam RM, Wang X, Wiggs JL, Williams K, Yu K. The Gene, Environment Association Studies consortium (GENEVA): maximizing the knowledge obtained from GWAS by collaboration across studies of multiple conditions. Genet Epidemiol. 2010;34:364–72. [Europe PMC free article] [Abstract] [Google Scholar]
- Cornelis MC, Tchetgen Tchetgen EJ, Liang L, Qi L, Chatterjee N, Hu FB, Kraft P. Gene-environment interactions in genome-wide association studies: a comparative study of tests applied to empirical studies of type 2 diabetes. Am J Epidemiol. 2011;175:191–202. [Europe PMC free article] [Abstract] [Google Scholar]
- Crainiceanu A, Liang KY, Crainiceanu CM. Bootstrap Bayesian Analysis with Applications to Gene-Environment Interaction. 2009; 24th International Symposium on Computer and Information Sciences; 2009. pp. 649–654. [Google Scholar]
- Culverhouse R, Klein T, Shannon W. Detecting epistatic interactions contributing to quantitative traits. Genet Epidemiol. 2004;27:141–52. [Abstract] [Google Scholar]
- Culverhouse R, Suarez BK, Lin J, Reich T. A perspective on epistasis: limits of models displaying no main effect. Am J Hum Genet. 2002;70:461–71. [Europe PMC free article] [Abstract] [Google Scholar]
- Dai JY, Kooperberg C, LeBlanc M, Prentice RL. UW Biostatistics Working Paper Series Working Paper. 2010. On two-stage hypothesis testing procedures via asymptotically independent statistics; p. 367. [Google Scholar]
- Davis RL, Khoury MJ. The emergence of biobanks: Practical design considerations for large population-based studies of gene-environiment interactions. Community Genetics. 2007;10:181–185. [Abstract] [Google Scholar]
- Dehghan A, Dupuis J, Barbalic M, Bis JC, Eiriksdottir G, Lu C, Pellikka N, Wallaschofski H, Kettunen J, Henneman P, Baumert J, Strachan DP, Fuchsberger C, Vitart V, Wilson JF, Pare G, Naitza S, Rudock ME, Surakka I, de Geus EJ, Alizadeh BZ, Guralnik J, Shuldiner A, Tanaka T, Zee RY, Schnabel RB, Nambi V, Kavousi M, Ripatti S, Nauck M, Smith NL, Smith AV, Sundvall J, Scheet P, Liu Y, Ruokonen A, Rose LM, Larson MG, Hoogeveen RC, Freimer NB, Teumer A, Tracy RP, Launer LJ, Buring JE, Yamamoto JF, Folsom AR, Sijbrands EJ, Pankow J, Elliott P, Keaney JF, Sun W, Sarin AP, Fontes JD, Badola S, Astor BC, Hofman A, Pouta A, Werdan K, Greiser KH, Kuss O, Meyer zu Schwabedissen HE, Thiery J, Jamshidi Y, Nolte IM, Soranzo N, Spector TD, Volzke H, Parker AN, Aspelund T, Bates D, Young L, Tsui K, Siscovick DS, Guo X, Rotter JI, Uda M, Schlessinger D, Rudan I, Hicks AA, Penninx BW, Thorand B, Gieger C, Coresh J, Willemsen G, Harris TB, Uitterlinden AG, Jarvelin MR, Rice K, Radke D, Salomaa V, Willems van Dijk K, Boerwinkle E, Vasan RS, Ferrucci L, Gibson QD, Bandinelli S, Snieder H, Boomsma DI, Xiao X, Campbell H, et al. Meta-analysis of genome-wide association studies in >80 000 subjects identifies multiple loci for C-reactive protein levels. Circulation. 2011;123:731–8. [Europe PMC free article] [Abstract] [Google Scholar]
- Demissie S, Cupples LA. Bias due to two-stage residual-outcome regression analysis in genetic association studies. Genet Epidemiol. 2011;35:592–6. [Europe PMC free article] [Abstract] [Google Scholar]
- Dempfle A, Scherag A, Hein R, Beckmann L, Chang-Claude J, Schafer H. Gene-environment interactions for complex traits: definitions, methodological requirements and challenges. European Journal of Human Genetics. 2008;16:1164–1172. [Abstract] [Google Scholar]
- Dennis J, Hawken S, Krewski D, Birkett N, Gheorghe M, Frei J, McKeown-Eyssen G, Little J. Bias in the case-only design applied to studies of gene-environment and gene-gene interaction: a systematic review and meta-analysis. Int J Epidemiol. 2011;40:1329–41. [Abstract] [Google Scholar]
- Dizier MH, Selinger-Leneman H, Genin E. Testing linkage and gene x environment interaction: Comparison of different affected sib-pair methods. Genetic Epidemiology. 2003;25:73–79. [Abstract] [Google Scholar]
- Doherty SP, Grabowski J, Hoffman C, Ng SP, Zelikoff JT. Early life insult from cigarette smoke may be predictive of chronic diseases later in life. Biomarkers. 2009;14(Suppl 1):97–101. [Abstract] [Google Scholar]
- Duell EJ, Bracci PM, Moore JH, Burk RD, Kelsey KT, Holly EA. Detecting pathway-based gene-gene and gene-environment interactions in pancreatic cancer. Cancer Epidemiol Biomarkers Prev. 2008;17:1470–9. [Europe PMC free article] [Abstract] [Google Scholar]
- Dunn EC, Uddin M, Subramanian SV, Smoller JW, Galea S, Koenen KC. Research review: gene-environment interaction research in youth depression - a systematic review with recommendations for future research. J Child Psychol Psychiatry. 2011;52:1223–38. [Europe PMC free article] [Abstract] [Google Scholar]
- Efird JT. Method for indirectly estimating gene-environment effect modification and power given only genotype frequency and odds ratio of environmental exposure. Eur J Epidemiol. 2005;20:389–93. [Abstract] [Google Scholar]
- Ege MJ, Strachan DP, Cookson WO, Moffatt MF, Gut I, Lathrop M, Kabesch M, Genuneit J, Buchele G, Sozanska B, Boznanski A, Cullinan P, Horak E, Bieli C, Braun-Fahrlander C, Heederik D, von Mutius E. Gene-environment interaction for childhood asthma and exposure to farming in Central Europe. J Allergy Clin Immunol. 2011;127:138–44. 144, e1–4. [Abstract] [Google Scholar]
- Elbaz A, Alperovitch A. Bias in association studies resulting from gene-environment interactions and competing risks. Am J Epidemiol. 2002;155:265–72. [Abstract] [Google Scholar]
- Engelman CD, Baurley JW, Chiu YF, Joubert BR, Lewinger JP, Maenner MJ, Murcray CE, Shi G, Gauderman WJ. Detecting Gene-Environment Interactions in Genome-Wide Association Data. Genetic Epidemiology. 2009;33:S68–S73. [Europe PMC free article] [Abstract] [Google Scholar]
- Fan R, Zhong M, Wang S, Zhang Y, Andrew A, Karagas M, Chen H, Amos CI, Xiong M, Moore JH. Entropy-based information gain approaches to detect and to characterize gene-gene and gene-environment interactions/correlations of complex diseases. Genet Epidemiol. 2011;35:706–21. [Europe PMC free article] [Abstract] [Google Scholar]
- Fardo DW, Liu J, DeMeo DL, Silverman EK, Vansteelandt S. Gene-environment Interaction Testing in Family-based Association Studies With Phenotypically Ascertained Samples: A Causal Inference Approach. Biostatistics 2011 [Europe PMC free article] [Abstract] [Google Scholar]
- Ferreira T, Donnelly P, Marchini J. Powerful Bayesian gene–gene interaction analysis. Am J Hum Genet. 2007;81(Suppl) [Google Scholar]
- Fodor I. LLNL technical report. 2002. A survey of dimension reduction techniques. [Google Scholar]
- Franks PW. Gene x environment interactions in type 2 diabetes. Curr Diab Rep. 2011;11:552–61. [Abstract] [Google Scholar]
- Garcia-Closas M, Rothman N, Lubin J. Misclassification in case-control studies of gene-environment interactions: assessment of bias and sample size. Cancer Epidemiol Biomarkers Prev. 1999;8:1043–50. [Abstract] [Google Scholar]
- Garcia-Closas M, Thompson WD, Robins JM. Differential misclassification and the assessment of gene-environment interactions in case-control studies. American Journal of Epidemiology. 1998;147:426–433. [Abstract] [Google Scholar]
- Gauderman WJ, Faucett CL. Detection of gene-environment interactions in joint segregation and linkage analysis. Am J Hum Genet. 1997;61:1189–99. [Europe PMC free article] [Abstract] [Google Scholar]
- Gauderman WJ, Thomas DC. The role of interacting determinants in the localization of genes. Genetic Dissection of Complex Traits. 2001;42:393–412. [Abstract] [Google Scholar]
- Gauderman WJ, Thomas DC, Murcray CE, Conti D, Li D, Lewinger JP. Efficient genome-wide association testing of gene-environment interaction in case-parent trios. Am J Epidemiol. 2010;172:116–22. [Europe PMC free article] [Abstract] [Google Scholar]
- Geneletti S, Gallo V, Porta M, Khoury MJ, Vineis P. Assessing causal relationships in genomics: From Bradford-Hill criteria to complex gene-environment interactions and directed acyclic graphs. Emerg Themes Epidemiol. 2011;8:5. [Europe PMC free article] [Abstract] [Google Scholar]
- Gibson G. Hints of hidden heritability in GWAS. Nature Genetics. 2010;42:558–560. [Abstract] [Google Scholar]
- Greenland S. Interactions in epidemiology: relevance, identification, and estimation. Epidemiology. 2009;20:14–7. [Abstract] [Google Scholar]
- Gu CC, Yang WW, Kraja AT, de Las Fuentes L, Davila-Roman VG. Genetic association analysis of coronary heart disease by profiling gene-environment interaction based on latent components in longitudinal endophenotypes. BMC Proc. 2009;3(Suppl 7):S86. [Europe PMC free article] [Abstract] [Google Scholar]
- Gunther F, Wawro N, Bammann K. Neural networks for modeling gene-gene interactions in association studies. BMC Genet. 2009;10:87. [Europe PMC free article] [Abstract] [Google Scholar]
- Hamza TH, Chen H, Hill-Burns EM, Rhodes SL, Montimurro J, Kay DM, Tenesa A, Kusel VI, Sheehan P, Eaaswarkhanth M, Yearout D, Samii A, Roberts JW, Agarwal P, Bordelon Y, Park Y, Wang L, Gao J, Vance JM, Kendler KS, Bacanu SA, Scott WK, Ritz B, Nutt J, Factor SA, Zabetian CP, Payami H. Genome-wide gene-environment study identifies glutamate receptor gene GRIN2A as a Parkinson’s disease modifier gene via interaction with coffee. PLoS Genet. 2011;7:e1002237. [Europe PMC free article] [Abstract] [Google Scholar]
- Hirschhorn JN, Daly MJ. Genome-wide association studies for common diseases and complex traits. Nat Rev Genet. 2005;6:95–108. [Abstract] [Google Scholar]
- Hoffmann TJ, Lange C, Vansteelandt S, Laird NM. Gene-environment interaction tests for dichotomous traits in trios and sibships. Genet Epidemiol. 2009;33:691–9. [Europe PMC free article] [Abstract] [Google Scholar]
- Hothorn T, Hornik K, Zeileis A. Unbiased recursive partitioning: A conditional inference framework. Journal of Computational and Graphical Statistics. 2006;15:651–674. [Google Scholar]
- Hunter DJ. Gene-environment interactions in human diseases. Nat Rev Genet. 2005;6:287–98. [Abstract] [Google Scholar]
- Karageorgi S, Prescott J, Wong JY, Lee IM, Buring JE, De Vivo I. GSTM1 and GSTT1 copy number variation in population-based studies of endometrial cancer risk. Cancer Epidemiol Biomarkers Prev. 2011;20:1447–52. [Europe PMC free article] [Abstract] [Google Scholar]
- Kazma R, Babron MC, Genin E. Genetic association and gene-environment interaction: a new method for overcoming the lack of exposure information in controls. Am J Epidemiol. 2011;173:225–35. [Abstract] [Google Scholar]
- Khoury MJ, Wacholder S. Invited commentary: from genome-wide association studies to gene-environment-wide interaction studies--challenges and opportunities. Am J Epidemiol. 2009;169:227–30. discussion 234–5. [Europe PMC free article] [Abstract] [Google Scholar]
- Knox SS. From ‘omics’ to complex disease: a systems biology approach to gene-environment interactions in cancer. Cancer Cell International. 2010:10. [Europe PMC free article] [Abstract] [Google Scholar]
- Kooperberg C, Leblanc M. Increasing the power of identifying gene x gene interactions in genome-wide association studies. Genet Epidemiol. 2008;32:255–63. [Europe PMC free article] [Abstract] [Google Scholar]
- Kraft P. Population stratification bias more widespread than previously thought. Epidemiology. 2011;22:408–409. [Abstract] [Google Scholar]
- Kraft P, Yen YC, Stram DO, Morrison J, Gauderman WJ. Exploiting gene-environment interaction to detect genetic associations. Hum Hered. 2007;63:111–9. [Abstract] [Google Scholar]
- Laird NM, Lange C. Family-based designs in the age of large-scale gene-association studies. Nat Rev Genet. 2006;7:385–94. [Abstract] [Google Scholar]
- Lake SL, Laird NM. Tests of gene-environment interaction for case-parent triads with general environmental exposures. Ann Hum Genet. 2004;68:55–64. [Abstract] [Google Scholar]
- Lee WC, Chang CH. Assessing effects of disease genes and gene-environment interactions: the case-spouse design and the counterfactual-control analysis. J Epidemiol Community Health. 2006;60:683–5. [Europe PMC free article] [Abstract] [Google Scholar]
- Lehr T, Yuan J, Zeumer D, Jayadev S, Ritchie MD. Rule based classifier for the analysis of gene-gene and gene-environment interactions in genetic association studies. BioData Min. 2011;4:4. [Europe PMC free article] [Abstract] [Google Scholar]
- Lesch KP. Gene-environment interaction and the genetics of depression. J Psychiatry Neurosci. 2004;29:174–84. [Europe PMC free article] [Abstract] [Google Scholar]
- Lettre G, Lange C, Hirschhorn JN. Genetic model testing and statistical power in population-based association studies of quantitative traits. Genet Epidemiol. 2007;31:358–62. [Abstract] [Google Scholar]
- Li D, Conti DV. Detecting gene-environment interactions using a combined case-only and case-control approach. Am J Epidemiol. 2009;169:497–504. [Europe PMC free article] [Abstract] [Google Scholar]
- Lim S, Beyene J, Greenwood CM. Continuous covariates in genetic association studies of case-parent triads: gene and gene-environment interaction effects, population stratification, and power analysis. Stat Appl Genet Mol Biol. 2005;4:Article20. [Abstract] [Google Scholar]
- Lindstrom S, Yen YC, Spiegelman D, Kraft P. The impact of gene-environment dependence and misclassification in genetic association studies incorporating gene-environment interactions. Hum Hered. 2009;68:171–81. [Europe PMC free article] [Abstract] [Google Scholar]
- Little R, Rubin D. Statistical analysis with missing data. John Wiley and Sons; New York: 1987. [Google Scholar]
- Liu X, Fallin MD, Kao WH. Genetic dissection methods: designs used for tests of gene-environment interaction. Curr Opin Genet Dev. 2004;14:241–5. [Abstract] [Google Scholar]
- Lo CY, Hsieh PH, Chen HF, Su HM. A maternal high-fat diet during pregnancy in rats results in a greater risk of carcinogen-induced mammary tumors in the female offspring than exposure to a high-fat diet in postnatal life. Int J Cancer. 2009;125:767–73. [Abstract] [Google Scholar]
- Lobach I, Mallick B, Carroll RJ. Semiparametric Bayesian analysis of gene-environment interactions with error in measurement of environmental covariates and missing genetic data. Stat Interface. 2011;4:305–316. [Europe PMC free article] [Abstract] [Google Scholar]
- Lou XY, Chen GB, Yan L, Ma JZ, Mangold JE, Zhu J, Elston RC, Li MD. A combinatorial approach to detecting gene-gene and gene-environment interactions in family studies. Am J Hum Genet. 2008;83:457–67. [Europe PMC free article] [Abstract] [Google Scholar]
- Lunetta KL, Hayward LB, Segal J, Van Eerdewegh P. Screening large-scale association study data: exploiting interactions using random forests. Bmc Genetics. 2004:5. [Europe PMC free article] [Abstract] [Google Scholar]
- Macgregor S, Khan IA. GAIA: an easy-to-use web-based application for interaction analysis of case-control data. BMC Med Genet. 2006;7:34. [Europe PMC free article] [Abstract] [Google Scholar]
- Maenner MJ, Denlinger LC, Langton A, Meyers KJ, Engelman CD, Skinner HG. Detecting gene-by-smoking interactions in a genome-wide association study of early-onset coronary heart disease using random forests. BMC Proc. 2009;3(Suppl 7):S88. [Europe PMC free article] [Abstract] [Google Scholar]
- Mahachie John JM, Van Lishout F, Van Steen K. Model-Based Multifactor Dimensionality Reduction to detect epistasis for quantitative traits in the presence of error-free and noisy data. Eur J Hum Genet. 2011;19:696–703. [Europe PMC free article] [Abstract] [Google Scholar]
- Maity A, Carroll RJ, Mammen E, Chatterjee N. Testing in semiparametric models with interaction, with applications to gene-environment interactions. J R Stat Soc Series B Stat Methodol. 2009;71:75–96. [Europe PMC free article] [Abstract] [Google Scholar]
- Manning AK, LaValley M, Liu CT, Rice K, An P, Liu Y, Miljkovic I, Rasmussen-Torvik L, Harris TB, Province MA, Borecki IB, Florez JC, Meigs JB, Cupples LA, Dupuis J. Meta-analysis of gene-environment interaction: joint estimation of SNP and SNP x environment regression coefficients. Genet Epidemiol. 2011;35:11–8. [Europe PMC free article] [Abstract] [Google Scholar]
- Manolio TA, Collins FS. Genes, environment, health, and disease: facing up to complexity. Hum Hered. 2007;63:63–6. [Abstract] [Google Scholar]
- Marchini J, Donnelly P, Cardon LR. Genome-wide strategies for detecting multiple loci that influence complex diseases. Nat Genet. 2005;37:413–7. [Abstract] [Google Scholar]
- McKinney BA, Crowe JE, Guo J, Tian D. Capturing the spectrum of interaction effects in genetic association studies by simulated evaporative cooling network analysis. PLoS Genet. 2009;5:e1000432. [Europe PMC free article] [Abstract] [Google Scholar]
- Meyer UA. Pharmacogenetics and adverse drug reactions. Lancet. 2000;356:1667–71. [Abstract] [Google Scholar]
- Mi X, Eskridge KM, George V, Wang D. Structural equation modeling of gene-environment interactions in coronary heart disease. Ann Hum Genet. 2011;75:255–65. [Abstract] [Google Scholar]
- Moerkerke B, Vansteelandt S, Lange C. A doubly robust test for gene-environment interaction in family-based studies of affected offspring. Biostatistics. 2010;11:213–225. [Europe PMC free article] [Abstract] [Google Scholar]
- Motsinger AA, Dudek SM, Hahn LW, Ritchie MD. Comparison of Neural Network Optimization Approaches for Studies of Human Genetics. Lect Notes Comput Sci. 2006;3907:103–114. [Google Scholar]
- Mukherjee B, Ahn J, Gruber SB, Chatterjee N. Testing gene-environment interaction in large-scale case-control association studies: possible choices and comparisons. Am J Epidemiol. 2011;175:177–90. [Europe PMC free article] [Abstract] [Google Scholar]
- Mukherjee B, Ahn J, Gruber SB, Ghosh M, Chatterjee N. Case-control studies of gene-environment interaction: Bayesian design and analysis. Biometrics. 2010;66:934–48. [Europe PMC free article] [Abstract] [Google Scholar]
- Mukherjee B, Chatterjee N. Exploiting gene-environment independence for analysis of case-control studies: An empirical bayes-type shrinkage estimator to trade-off between bias and efficiency. Biometrics. 2008;64:685–694. [Abstract] [Google Scholar]
- Mukherjee B, Zhang L, Ghosh M, Sinha S. Semiparametric Bayesian analysis of case-control data under conditional gene-environment independence. Biometrics. 2007;63:834–44. [Abstract] [Google Scholar]
- Murcray CE, Lewinger JP, Conti DV, Thomas DC, Gauderman WJ. Sample Size Requirements to Detect Gene-Environment Interactions in Genome-Wide Association Studies. Genetic Epidemiology. 2011;35:201–210. [Europe PMC free article] [Abstract] [Google Scholar]
- Murcray CE, Lewinger JP, Gauderman WJ. Gene-environment interaction in genome-wide association studies. Am J Epidemiol. 2009;169:219–26. [Europe PMC free article] [Abstract] [Google Scholar]
- Ober C, Vercelli D. Gene-environment interactions in human disease: nuisance or opportunity? Trends Genet. 2011;27:107–15. [Europe PMC free article] [Abstract] [Google Scholar]
- Pare G, Cook NR, Ridker PM, Chasman DI. On the use of variance per genotype as a tool to identify quantitative trait interaction effects: a report from the Women’s Genome Health Study. PLoS Genet. 2010;6:e1000981. [Europe PMC free article] [Abstract] [Google Scholar]
- Park MY, Hastie T. Penalized logistic regression for detecting gene interactions. Biostatistics. 2008;9:30–50. [Abstract] [Google Scholar]
- Pattin KA, White BC, Barney N, Gui J, Nelson HH, Kelsey KT, Andrew AS, Karagas MR, Moore JH. A computationally efficient hypothesis testing method for epistasis analysis using multifactor dimensionality reduction. Genet Epidemiol. 2009;33:87–94. [Europe PMC free article] [Abstract] [Google Scholar]
- Pearce N. Epidemiology in a changing world: variation, causation and ubiquitous risk factors. International Journal of Epidemiology. 2011;40:503–512. [Abstract] [Google Scholar]
- Pereira TV, Patsopoulos NA, Pereira AC, Krieger JE. Strategies for genetic model specification in the screening of genome-wide meta-analysis signals for further replication. Int J Epidemiol. 2011;40:457–69. [Abstract] [Google Scholar]
- Pereira TV, Patsopoulos NA, Salanti G, Ioannidis JP. Discovery properties of genome-wide association signals from cumulatively combined data sets. Am J Epidemiol. 2009;170:1197–206. [Europe PMC free article] [Abstract] [Google Scholar]
- Phillips PC. Epistasis - the essential role of gene interactions in the structure and evolution of genetic systems. Nature Reviews Genetics. 2008;9:855–867. [Europe PMC free article] [Abstract] [Google Scholar]
- Piegorsch WW, Weinberg CR, Taylor JA. Non-hierarchical logistic models and case-only designs for assessing susceptibility in population-based case-control studies. Stat Med. 1994;13:153–62. [Abstract] [Google Scholar]
- Prentice RL. Empirical evaluation of gene and environment interactions: methods and potential. J Natl Cancer Inst. 2011;103:1209–10. [Europe PMC free article] [Abstract] [Google Scholar]
- Price AL, Zaitlen NA, Reich D, Patterson N. New approaches to population stratification in genome-wide association studies. Nature Reviews Genetics. 2010;11:459–463. [Europe PMC free article] [Abstract] [Google Scholar]
- Rappaport SM, Smith MT. Environment and Disease Risks. Science. 2010;330:460–461. [Europe PMC free article] [Abstract] [Google Scholar]
- Ripley B. Pattern Recognition and Neural Networks. Cambridge University Press; Cambridge: 1996. [Google Scholar]
- Risch N, Herrell R, Lehner T, Liang KY, Eaves L, Hoh J, Griem A, Kovacs M, Ott J, Merikangas KR. Interaction between the serotonin transporter gene (5-HTTLPR), stressful life events, and risk of depression: a meta-analysis. JAMA. 2009;301:2462–71. [Europe PMC free article] [Abstract] [Google Scholar]
- Ritchie MD, Hahn LW, Roodi N, Bailey LR, Dupont WD, Parl FF, Moore JH. Multifactor-dimensionality reduction reveals high-order interactions among estrogen-metabolism genes in sporadic breast cancer. American Journal of Human Genetics. 2001;69:138–147. [Europe PMC free article] [Abstract] [Google Scholar]
- Ritchie MD, Motsinger AA, Bush WS, Coffey CS, Moore JH. Genetic Programming Neural Networks: A Powerful Bioinformatics Tool for Human Genetics. Appl Soft Comput. 2007;7:471–479. [Europe PMC free article] [Abstract] [Google Scholar]
- Rothman K, Greenland S. Modern epidemiology. Lippencott-Raven; Philadelphia: 1998. [Google Scholar]
- Rothman K, Greenland S, Lash T. Modern epidemiology. 3. Lippincott Williams & Wilkins; Philadephia: 2008. [Google Scholar]
- Rothman KJ, Greenland S, Walker AM. Concepts of Interaction. American Journal of Epidemiology. 1980;112:467–470. [Abstract] [Google Scholar]
- Schaid DJ. Case-parents design for gene-environment interaction. Genetic Epidemiology. 1999;16:261–273. [Abstract] [Google Scholar]
- Schwarz DF, Konig IR, Ziegler A. On safari to Random Jungle: a fast implementation of Random Forests for high-dimensional data. Bioinformatics. 2010;26:1752–1758. [Europe PMC free article] [Abstract] [Google Scholar]
- Schwender H, Ruczinski I. Logic regression and its extensions. Adv Genet. 2010;72:25–45. [Abstract] [Google Scholar]
- Shi M, Umbach DM, Weinberg CR. Family-based Gene-by-environment Interaction Studies Revelations and Remedies. Epidemiology. 2011;22:400–407. [Europe PMC free article] [Abstract] [Google Scholar]
- Siemiatycki J, Thomas DC. Biological models and statistical interactions: an example from multistage carcinogenesis. Int J Epidemiol. 1981;10:383–7. [Abstract] [Google Scholar]
- Smith GD, Timpson N, Ebrahim S. Strengthening causal inference in cardiovascular epidemiology through Mendelian randomization. Ann Med. 2008;40:524–41. [Abstract] [Google Scholar]
- Song YS, Wang F, Slatkin M. General epistatic models of the risk of complex diseases. Genetics. 2010;186:1467–73. [Europe PMC free article] [Abstract] [Google Scholar]
- Stern MC, Johnson LR, Bell DA, Taylor JA. XPD codon 751 polymorphism, metabolism genes, smoking, and bladder cancer risk. Cancer Epidemiology Biomarkers & Prevention. 2002;11:1004–1011. [Abstract] [Google Scholar]
- Strobl C, Boulesteix AL, Kneib T, Augustin T, Zeileis A. Conditional variable importance for random forests. Bmc Bioinformatics. 2008:9. [Europe PMC free article] [Abstract] [Google Scholar]
- Strobl C, Boulesteix AL, Zeileis A, Hothorn T. Bias in random forest variable importance measures: Illustrations, sources and a solution. Bmc Bioinformatics. 2007:8. [Europe PMC free article] [Abstract] [Google Scholar]
- Struchalin MV, Dehghan A, Witteman JC, van Duijn C, Aulchenko YS. Variance heterogeneity analysis for detection of potentially interacting genetic loci: method and its limitations. BMC Genet. 2010;11:92. [Europe PMC free article] [Abstract] [Google Scholar]
- Takeuchi F, Kobayashi S, Ogihara T, Fujioka A, Kato N. Detection of common single nucleotide polymorphisms synthesizing quantitative trait association of rarer causal variants. Genome Res 2010 [Europe PMC free article] [Abstract] [Google Scholar]
- Tan P, Steinbach M, Kumar V. Introduction to Data Mining. Addison-Wesley; 2006. [Google Scholar]
- Tanck MW, Jukema JW, Zwinderman AH. Simultaneous estimation of gene-gene and gene-environment interactions for numerous loci using double penalized log-likelihood. Genet Epidemiol. 2006;30:645–51. [Abstract] [Google Scholar]
- Tchetgen EJ, Robins J. The semiparametric case-only estimator. Biometrics. 2010;66:1138–44. [Europe PMC free article] [Abstract] [Google Scholar]
- Tchetgen EJT, Kraft P. On the Robustness of Tests of Genetic Associations Incorporating Gene-environment Interaction When the Environmental Exposure is Misspecified. Epidemiology. 2011;22:257–261. [Europe PMC free article] [Abstract] [Google Scholar]
- Tchetgen Tchetgen EJ, VanderWeele TJ. Harvard University Biostatistics Working Paper Series Working Paper. 2012. Robustness of Measures of Interaction to Unmeasured Confounding; p. 89. [Google Scholar]
- Thomas D. Gene--environment-wide association studies: emerging approaches. Nat Rev Genet. 2010a;11:259–72. [Europe PMC free article] [Abstract] [Google Scholar]
- Thomas D. Methods for Investigating Gene-Environment Interactions in Candidate Pathway and Genome-Wide Association Studies. Annual Review of Public Health. 2010b;31:21–36. [Europe PMC free article] [Abstract] [Google Scholar]
- Thomas DC. Case-parents design for gene-environment interaction by Schaid. Genet Epidemiol. 2000;19:461–3. [Abstract] [Google Scholar]
- Thompson WD. Effect modification and the limits of biological inference from epidemiologic data. J Clin Epidemiol. 1991;44:221–32. [Abstract] [Google Scholar]
- Tryon R. Cluster Analysis. McGraw-Hill; New-York: 1939. [Google Scholar]
- Tung L, Gordon D, Finch SJ. The impact of genotype misclassification errors on the power to detect a gene-environment interaction using cox proportional hazards modeling. Hum Hered. 2007;63:101–10. [Abstract] [Google Scholar]
- Tweel I, Schipper M. Sequential tests for gene-environment interactions in matched case-control studies. Stat Med. 2004;23:3755–71. [Abstract] [Google Scholar]
- Tzeng JY, Zhang DW, Pongpanich M, Smith C, McCarthy MI, Sale MM, Worrall BB, Hsu FC, Thomas DC, Sullivan PF. Studying Gene and Gene-Environment Effects of Uncommon and Common Variants on Continuous Traits: A Marker-Set Approach Using Gene-Trait Similarity Regression. American Journal of Human Genetics. 2011;89:277–288. [Europe PMC free article] [Abstract] [Google Scholar]
- Uher R. Gene-environment interaction: overcoming methodological challenges. Novartis Found Symp. 2008;293:13–26. discussion 26–30, 68–70. [Abstract] [Google Scholar]
- Umbach DM, Weinberg CR. Designing and analysing case-control studies to exploit independence of genotype and exposure. Stat Med. 1997;16:1731–43. [Abstract] [Google Scholar]
- Umbach DM, Weinberg CR. The use of case-parent triads to study joint effects of genotype and exposure. Am J Hum Genet. 2000;66:251–61. [Europe PMC free article] [Abstract] [Google Scholar]
- van der Sluis S, Dolan CV, Neale MC, Posthuma D. A general test for gene-environment interaction in sib pair-based association analysis of quantitative traits. Behav Genet. 2008;38:372–89. [Europe PMC free article] [Abstract] [Google Scholar]
- Van Lishout F, Cattaert T, Mahachie John M, Gusareva E, Urrea V, Cleynen I, Théatre E, Charloteaux B, Calle M, Wehenkel L, Van Steen K. An efficient algorithm to perform multiple testing in epistasis screening. 2011. [Europe PMC free article] [Abstract] [Google Scholar]
- Van Steen K. Travelling the world of gene-gene interactions. Briefings in Bioinformatics. 2012;13:1–19. [Abstract] [Google Scholar]
- Vansteelandt S, Demeo DL, Lasky-Su J, Smoller JW, Murphy AJ, McQueen M, Schneiter K, Celedon JC, Weiss ST, Silverman EK, Lange C. Testing and estimating gene-environment interactions in family-based association studies. Biometrics. 2008;64:458–67. [Abstract] [Google Scholar]
- Vercelli D. Gene-environment interactions in asthma and allergy: the end of the beginning? Current Opinion in Allergy and Clinical Immunology. 2010;10:145–148. [Europe PMC free article] [Abstract] [Google Scholar]
- Visscher PM, Yang J, Goddard ME. A commentary on ‘common SNPs explain a large proportion of the heritability for human height’ by Yang et al. Twin Res Hum Genet. 2010;13:517–24. [Abstract] [Google Scholar]
- Wakefield J, De Vocht F, Hung RJ. Bayesian mixture modeling of gene-environment and gene-gene interactions. Genet Epidemiol. 2010;34:16–25. [Europe PMC free article] [Abstract] [Google Scholar]
- Wang LY, Lee WC. Population stratification bias in the case-only study for gene-environment interactions. Am J Epidemiol. 2008;168:197–201. [Abstract] [Google Scholar]
- Wang WY, Barratt BJ, Clayton DG, Todd JA. Genome-wide association studies: theoretical and practical concerns. Nat Rev Genet. 2005;6:109–18. [Abstract] [Google Scholar]
- Weinberg CR, Umbach DM. Choosing a retrospective design to assess joint genetic and environmental contributions to risk. Am J Epidemiol. 2000;152:197–203. [Abstract] [Google Scholar]
- Whittemore AS. Assessing environmental modifiers of disease risk associated with rare mutations. Hum Hered. 2007;63:134–43. [Abstract] [Google Scholar]
- Wild CP. Complementing the genome with an “exposome”: The outstanding challenge of environmental exposure measurement in molecular epidemiology. Cancer Epidemiology Biomarkers & Prevention. 2005;14:1847–1850. [Abstract] [Google Scholar]
- Willis-Owen SA, Valdar W. Deciphering gene-environment interactions through mouse models of allergic asthma. J Allergy Clin Immunol. 2009;123:14–23. quiz 24–5. [Abstract] [Google Scholar]
- Witte JS, Gauderman WJ, Thomas DC. Asymptotic bias and efficiency in case-control studies of candidate genes and gene-environment interactions: basic family designs. Am J Epidemiol. 1999;149:693–705. [Abstract] [Google Scholar]
- Wong MY, Day NE, Luan JA, Chan KP, Wareham NJ. The detection of gene-environment interaction for continuous traits: should we deal with measurement error by bigger studies or better measurement? Int J Epidemiol. 2003;32:51–7. [Abstract] [Google Scholar]
- Wong MY, Day NE, Luan JA, Wareham NJ. Estimation of magnitude in gene-environment interactions in the presence of measurement error. Stat Med. 2004;23:987–98. [Abstract] [Google Scholar]
- Wray NR, Purcell SM, Visscher PM. Synthetic Associations Created by Rare Variants Do Not Explain Most GWAS Results. Plos Biology. 2011:9. [Europe PMC free article] [Abstract] [Google Scholar]
- Wright AF, Carothers AD, Campbell H. Gene-environment interactions--the BioBank UK study. Pharmacogenomics J. 2002;2:75–82. [Abstract] [Google Scholar]
- Wu C, Hu Z, He Z, Jia W, Wang F, Zhou Y, Liu Z, Zhan Q, Liu Y, Yu D, Zhai K, Chang J, Qiao Y, Jin G, Liu Z, Shen Y, Guo C, Fu J, Miao X, Tan W, Shen H, Ke Y, Zeng Y, Wu T, Lin D. Genome-wide association study identifies three new susceptibility loci for esophageal squamous-cell carcinoma in Chinese populations. Nat Genet. 2011;43:679–84. [Abstract] [Google Scholar]
- Wu X, Jin L, Xiong M. Mutual information for testing gene-environment interaction. PLoS One. 2009;4:e4578. [Europe PMC free article] [Abstract] [Google Scholar]
- Wyszynski DF, Diehl SR. The mother-only method (MOM) to detect maternal gene--environment interactions. Paediatr Perinat Epidemiol. 2001;15:317–8. [Abstract] [Google Scholar]
- Yang J, Benyamin B, McEvoy BP, Gordon S, Henders AK, Nyholt DR, Madden PA, Heath AC, Martin NG, Montgomery GW, Goddard ME, Visscher PM. Common SNPs explain a large proportion of the heritability for human height. Nat Genet. 2010;42:565–9. [Europe PMC free article] [Abstract] [Google Scholar]
- Yang Q, Khoury MJ. Evolving methods in genetic epidemiology. III. Gene-environment interaction in epidemiologic research. Epidemiol Rev. 1997;19:33–43. [Abstract] [Google Scholar]
- Yoshida M, Koike A. SNPInterForest: A new method for detecting epistatic interactions. Bmc Bioinformatics. 2011:12. [Europe PMC free article] [Abstract] [Google Scholar]
- Yu K, Wacholder S, Wheeler W, Wang Z, Caporaso N, Landi MT, Liang F. A flexible bayesian model for studying gene-environment interaction. PLoS Genet. 2012;8:e1002482. [Europe PMC free article] [Abstract] [Google Scholar]
- Zhai R, Zhao Y, Liu G, Ter-Minassian M, Wu IC, Wang Z, Su L, Asomaning K, Chen F, Kulke MH, Lin X, Heist RS, Wain JC, Christiani DC. Interactions between environmental factors and polymorphisms in angiogenesis pathway genes in esophageal adenocarcinoma risk: A case-only study. Cancer. 2011;118:804–11. [Europe PMC free article] [Abstract] [Google Scholar]
- Zhang L, Mukherjee B, Ghosh M, Gruber S, Moreno V. Accounting for error due to misclassification of exposures in case-control studies of gene-environment interaction. Stat Med. 2008;27:2756–83. [Abstract] [Google Scholar]
- Zhang Y, Jiang B, Zhu J, Liu JS. Bayesian models for detecting epistatic interactions from genetic data. Ann Hum Genet. 2011;75:183–93. [Abstract] [Google Scholar]
- Zhang Y, Liu JS. Bayesian inference of epistatic interactions in case-control studies. Nat Genet. 2007;39:1167–73. [Abstract] [Google Scholar]
- Zuk O, Hechter E, Sunyaev SR, Lander ES. The mystery of missing heritability: Genetic interactions create phantom heritability. Proceedings of the National Academy of Sciences of the United States of America. 2012;109:1193–1198. [Europe PMC free article] [Abstract] [Google Scholar]
Full text links
Read article at publisher's site: https://doi.org/10.1007/s00439-012-1192-0
Read article for free, from open access legal sources, via Unpaywall:
https://europepmc.org/articles/pmc3677711?pdf=render
Citations & impact
Impact metrics
Article citations
The interaction of genetics and physical activity in the pathogenesis of metabolic dysfunction associated liver disease.
Sci Rep, 14(1):17817, 01 Aug 2024
Cited by: 0 articles | PMID: 39090170 | PMCID: PMC11294342
Bayesian Approaches in Exploring Gene-environment and Gene-gene Interactions: A Comprehensive Review.
Cancer Genomics Proteomics, 20(6suppl):669-678, 01 Dec 2023
Cited by: 1 article | PMID: 38035701 | PMCID: PMC10687732
Review Free full text in Europe PMC
Statistical methods for gene-environment interaction analysis.
Wiley Interdiscip Rev Comput Stat, 16(1):e1635, 05 Oct 2023
Cited by: 0 articles | PMID: 38699459 | PMCID: PMC11064894
Precision pharmacological reversal of strain-specific diet-induced metabolic syndrome in mice informed by epigenetic and transcriptional regulation.
PLoS Genet, 19(10):e1010997, 23 Oct 2023
Cited by: 0 articles | PMID: 37871105 | PMCID: PMC10621921
Social determinants of health and selection bias in genome-wide association studies.
World Psychiatry, 22(1):160-161, 01 Feb 2023
Cited by: 2 articles | PMID: 36640412 | PMCID: PMC9840488
Go to all (86) article citations
Similar Articles
To arrive at the top five similar articles we use a word-weighted algorithm to compare words from the Title and Abstract of each citation.
Invited commentary: from genome-wide association studies to gene-environment-wide interaction studies--challenges and opportunities.
Am J Epidemiol, 169(2):227-30; discussion 234-5, 20 Nov 2008
Cited by: 99 articles | PMID: 19022826 | PMCID: PMC2727257
Current Challenges and New Opportunities for Gene-Environment Interaction Studies of Complex Diseases.
Am J Epidemiol, 186(7):753-761, 01 Oct 2017
Cited by: 103 articles | PMID: 28978193 | PMCID: PMC5860428
Molecular genetic gene-environment studies using candidate genes in schizophrenia: a systematic review.
Schizophr Res, 150(2-3):356-365, 02 Oct 2013
Cited by: 45 articles | PMID: 24094883
Review
Quick assessment for systematic test statistic inflation/deflation due to null model misspecifications in genome-wide environment interaction studies.
PLoS One, 14(7):e0219825, 18 Jul 2019
Cited by: 5 articles | PMID: 31318927 | PMCID: PMC6638962
Funding
Funders who supported this work.
NCI NIH HHS (1)
Grant ID: R21 CA165920
NIDDK NIH HHS (1)
Grant ID: R21 DK084529
NIEHS NIH HHS (1)
Grant ID: T32 ES007142