Abstract
Background
Previous studies have demonstrated that serological markers can assist in diagnosing inflammatory bowel disease (IBD). In this study, we aim to build a diagnostic tool incorporating serological markers, genetic variants, and markers of inflammation into a computational algorithm to examine patterns of combinations of markers to (1) identify patients with IBD and (2) differentiate patients with Crohn's disease (CD) from ulcerative colitis (UC).Methods
In this cross-sectional study, patient blood samples from 572 CD, 328 UC, 437 non-IBD controls, and 183 healthy controls from academic and community centers were analyzed for 17 markers: 8 serological markers (ASCA-IgA, ASCA-IgG, ANCA, pANCA, OmpC, CBir1, A4-Fla2, and FlaX), 4 genetic markers (ATG16L1, NKX2-3, ECM1, and STAT3), and 5 inflammatory markers (CRP, SAA, ICAM-1, VCAM-1, and VEGF). A diagnostic Random Forest algorithm was constructed to classify IBD, CD, and UC.Results
Receiver operating characteristic analysis compared the diagnostic accuracy of using a panel of serological markers only (ASCA-IgA, ASCA-IgG, ANCA, pANCA, OmpC, and CBir1) versus using a marker panel that in addition to the serological markers mentioned above also included gene variants, inflammatory markers, and 2 additional serological markers (A4-Fla2 and FlaX). The extended marker panel increased the IBD versus non-IBD discrimination area under the curve from 0.80 (95% confidence interval [CI], ±0.05) to 0.87 (95% CI, ±0.04; P < 0.001). The CD versus UC discrimination increased from 0.78 (95% CI, ±0.06) to 0.93 (95% CI, ±0.04; P < 0.001).Conclusions
Incorporating a combination of serological, genetic, and inflammation markers into a diagnostic algorithm improved the accuracy of identifying IBD and differentiating CD from UC versus using serological markers alone.Free full text

Combined Serological, Genetic, and Inflammatory Markers Differentiate Non-IBD, Crohn’s Disease, and Ulcerative Colitis Patients
Abstract
Background
Previous studies have demonstrated that serological markers can assist in diagnosing inflammatory bowel disease (IBD). In this study, we aim to build a diagnostic tool incorporating serological markers, genetic variants, and markers of inflammation into a computational algorithm to examine patterns of combinations of markers to (1) identify patients with IBD and (2) differentiate patients with Crohn’s disease (CD) from ulcerative colitis (UC).
Methods
In this cross-sectional study, patient blood samples from 572 CD, 328 UC, 437 non-IBD controls, and 183 healthy controls from academic and community centers were analyzed for 17 markers: 8 serological markers (ASCA-IgA, ASCA-IgG, ANCA, pANCA, OmpC, CBir1, A4-Fla2, and FlaX), 4 genetic markers (ATG16L1, NKX2-3, ECM1, and STAT3), and 5 inflammatory markers (CRP, SAA, ICAM-1, VCAM-1, and VEGF). A diagnostic Random Forest algorithm was constructed to classify IBD, CD, and UC.
Results
Receiver operating characteristic analysis compared the diagnostic accuracy of using a panel of serological markers only (ASCA-IgA, ASCA-IgG, ANCA, pANCA, OmpC, and CBir1) versus using a marker panel that in addition to the serological markers mentioned above also included gene variants, inflammatory markers, and 2 additional serological markers (A4-Fla2 and FlaX). The extended marker panel increased the IBD versus non-IBD discrimination area under the curve from 0.80 (95% confidence interval [CI], ±0.05) to 0.87 (95% CI, ±0.04; P < 0.001). The CD versus UC discrimination increased from 0.78 (95% CI, ±0.06) to 0.93 (95% CI, ±0.04; P < 0.001).
Conclusions
Incorporating a combination of serological, genetic, and inflammation markers into a diagnostic algorithm improved the accuracy of identifying IBD and differentiating CD from UC versus using serological markers alone.
The inflammatory bowel diseases (IBDs) are chronic inflammatory disorders of the gastrointestinal (GI) tract, the most common of which are Crohn’s disease (CD) and ulcerative colitis (UC). The etiologies of the IBDs are unknown, but evidence suggests that genetic predisposition combined with environmental exposures lead to inappropriate intestinal immune response to the enteric microbiota, resulting in characteristic inflammatory lesions of the gut. A diagnosis of IBD is complex and based on a combination of clinical examination, imaging, endoscopy with histopathology, and laboratory testing. In cases where the diagnosis is uncertain, serological markers can provide adjunctive information. In the early 1990s, anti-Saccharomyces cerevisiae antibodies (ASCA, antibodies to mannan on the surface of S. cerevisiae) and antineutrophil cytoplasmic antibody, perinuclear pattern (pAN-CA), were reported as specific serological markers in patients with IBD. ASCA is detected mainly in patients with CD. pANCA is more common in patients with UC, although it is also associated with left-sided colitis or UC-like CD (reviewed by Bossuyt1 and Targan et al2). Other serological markers have provided additional diagnostic information. These include antibodies to the bacterial protein OmpC3 and to the bacterial flagellins, including CBir12 and the recently described FlaX and Fla2.4 Generally, these antibodies may be of pathophysiologic significance as they represent systemic evidence of immune reactivity to specific components of the enteric microbiota.5
In addition to the antimicrobial antibodies typically associated with IBD, recent evidence suggests that other markers, including IBD-associated genetic variants and molecules involved in angiogenesis and inflammation, may provide additional information to help better identify and classify IBD. Over 100 IBD genetic susceptibility loci have been identified through genome-wide association studies.6,7 At least 30% of the loci are shared between CD and UC, whereas others are associated with only CD or UC.6 These genes play roles in a wide range of processes including development, innate immunity, adaptive immunity autophagy, and barrier functions.8
Several markers associated with chronic inflammation, angiogenesis, and cell adhesion have been reported to be upregulated in the serum and intestinal mucosa of patients with IBD. Expression of these markers may arise from remodeling of the microvasculature in the inflamed intestine, and ongoing angiogenesis may aggravate the inflammatory process, contributing to the pathology of IBD. In the gut, the cellular adhesion molecules ICAM-1 and VCAM-1 facilitate the binding and infiltration of leukocytes at inflammatory sites.9,10 Leukocytes then produce mediators such as vascular endothelial growth factor (VEGF), an angiogenic cytokine that potentiates vascular permeability and stimulates blood capillary growth.11 VEGF is elevated in the tissue and serum of patients with IBD.12–14 In addition, soluble ICAM-1 is elevated in patients with IBD with active disease.
To assist in the diagnostic evaluation of patients with suspected IBD, clinicians may benefit from a tool that can accurately (1) distinguish patients with IBD from patients with other GI disorders and (2) differentiate CD from UC. Given the heterogeneity of IBD, it is unlikely that any single marker or class of markers could successfully achieve both of these goals. However, a diagnostic tool that examines the patterns among multiple classes of markers may have valuable diagnostic potential. Machine learning algorithms are programs that have been used to incorporate information from multiple biomarkers to aid in the development of diagnostic instruments.15,16 Using multimarker patterns, such programs can be trained with a data set of marker values and known diagnoses to recognize different disease states in patients. The trained algorithm can then be used to classify patients into distinct disease states based on measurements of the individual’s marker levels. The aim of this study was to build a diagnostic tool that detects patterns among a combination of 3 different classes of markers to identify patients with IBD and to differentiate patients with CD from UC.
MATERIALS AND METHODS
Study Population
A cross-sectional study design was used to develop and validate the diagnostic test. Blood samples were drawn from patients between 1 and 10 years after the date of disease diagnosis. Samples were obtained from 8 academic and 37 community medical practices in North America. A total of 1520 patient samples were collected, including 900 patient samples with IBD (572 CD and 328 UC), 437 non-IBD GI disease controls, and 183 healthy controls. The GI disease controls included patients with irritable bowel syndrome (n = 167), chronic hepatitis (n = 42), chronic constipation (n = 16), functional dyspepsia (n = 1), gastroesophageal reflux disease (n = 133), celiac disease (n = 31), diverticulitis (n = 38), microscopic colitis (n = 3), and pancreatitis (n = 6). Healthy controls included patients who did not have any known inflammatory disorders or any diagnosed GI disorder, although colonoscopy or visualization was not required. The cohort included blood samples obtained from patient sample banks from Mount Sinai Hospital, Toronto, Canada (n = 298), and the University of North Carolina, Chapel Hill (n = 84). Study protocols were institutional review board approved for each site. Subjects were diagnosed with CD or UC based on a combination of standard criteria that included clinical symptoms, endoscopy, histopathology, video capsule, and/or radiographic studies. Extensive clinical information was not available for all patients. Additional information, such as the most recent symptoms, the Harvey–Bradshaw Index (n = 334), partial Mayo index (n = 184), smoking history (n = 518), CD location and behavior (n = 425), UC location (n = 183), and medication use (n = 900) were also available for a subset of patient samples. For the purpose of this study, the cohort was split into a “training” set of 1083 subjects and a “validation” set of 437 subjects.
Serological Markers
Serum concentrations of anti-CBir1, anti-OmpC, ASCA-IgA, ASCA-IgG, ANCA, anti-A4-FlaX, and anti-Fla2 antibodies were measured by a standardized enzyme-linked immunosorbent assay (ELISA) using a Freedom EVO 200 liquid-handling robot (Tecan, Männedorf, Switzerland) and has been described elsewhere.17–19 ELISA units per milliliter were calculated from a 5-parameter, logistic-derived 6-point standard curve derived from standards prepared from a pool of sera. Testing for perinuclearstaining antineutrophil cytoplasmic antibodies (pANCA) was performed by immunofluorescence staining of neutrophils, as previously described.20
Genotyping
Single-nucleotide polymorphism (SNP) genotyping on serological samples was performed by Prometheus Laboratories (San Diego, CA). The genotyping consisted of testing the following SNPs: SNP rs2241880 in the ATG16L1 gene, SNP rsl0883365 in the NKX2–3 gene, SNP rs3737240 in the ECM1 gene, and SNP rs744166 in the STAT3 gene. An allelic discrimination PCR method was used including 2 target-specific oligonucleotide sequences as PCR primer pairs and 2 allele-specific TaqMan probes for each assay (Applied Biosystems, Foster City, CA). Genotyping assays were performed on an ABI 7500 FAST Real-Time PCR system (Applied Biosystems). Patients were considered positive if they were homozygous for the risk allele.
Inflammatory Markers
Human VEGF levels were measured following the manufacturer’s procedure for the human VEGF ELISA Kit (Thermo Fisher Scientific, Waltham, MA). Concentrations were calculated using 4-parameter logistic curve-fitting software for the standard curve. Samples were tested for C-reactive protein (CRP), serum amyloid A (SAA), intracellular adhesion molecule 1 (ICAM-1), and vascular cell adhesion molecule 1 (VCAM-1) using a Meso Scale Discovery (MSD; Gaithersburg, MD) MULTI_SPOT Vascular Injury II Assay according to the manufacturer’s instructions and analyzed on an MSD SECTOR Imager.
Statistics on Single Markers
The assay results for the serological markers (anti-A4-Fla2, anti-A4-FlaX, anti-CBir1, anti-OmpC, ASCA-IgA, ASCA-IgG, and ANCA) and the inflammatory markers (VEGF, ICAM-1, VCAM-1 CRP, and SAA) were converted into quartiles. Patients were considered positive if their biomarker measurement was equal to or above the third quartile for that marker's pooled measured values. For genetic markers (ATG16L1, NXK2–3, ECM1, and STAT3), patients were considered positive if they were homozygous for the risk allele. Univariate analyses, Mann–Whitney U tests for continuous markers, or Fisher’s exact tests for binary variables were used to assess the single marker significance for non-IBD versus IBD and CD versus UC.
Marker Selection
The initial marker selection was made by screening the previously described biomarkers with the potential to discriminate between IBD versus non-IBD or CD versus UC. One hundred and twelve potential biomarkers were assessed on an independent discovery cohort made of 1000 IBD and non-IBD samples (data not shown). Univariate analyses, Mann-Whitney U tests for continuous markers, or Fisher’s exact tests for binary variables were used to assess the significance of the markers for non-IBD versus IBD and CD versus UC. Statistically significant markers were selected and used during the algorithm training phase to build the model. Markers selected for use in the diagnostic test were either significant in univariate analyses or significantly enhanced the discriminatory power of the diagnostic algorithm. A final set of 17 markers was selected based on statistical significance and contribution to the Random Forest model: 8 serological markers (anti-A4-Fla2, anti-A4-FlaX, anti-CBir1, anti-OmpC, ASCAIgA, ASCA-IgG, pANCA, and ANCA), 5 inflammatory markers (VEGF, ICAM-1, VCAM-1, CRP, and SAA), and 4 genetic markers (ATG16L1, NXK2–3, ECM1, and STAT3).
Diagnostic Algorithm Development
The diagnostic algorithm was built using data from the 1083 patients in the training set. All markers were used as continuous variables, except pANCA and the 4 SNPs, which were converted to binary (0 or 1) values. The algorithm included “Random Forests,” computational classifiers used in machine learning. A Random Forest is a collection of thousands of decision trees, each addressing the same classification problem but with a different randomized selection of instances used for each tree.21 In addition, a different random subset of predictive variables is considered at each node of a tree. Individual patients were considered the “instances” and the biomarkers were the “predictive variables,” so the Random Forests were trained to classify IBD disease status based on biomarker measurements. The final algorithm consisted of 2 Random Forests, one to classify patients as IBD or non-IBD and a second to classify patients with IBD as either CD or UC. Filtering rules were developed to identify the small group of patients with IBD that possessed a biomarker pattern consistent with IBD but were “inconclusive” in the ability to distinguish CD from UC due to the presence of both CD and UC markers. The “inconclusive” rule was defined using the third-quartile upper boundaries from the training set. Samples were considered “inconclusive” if (1) they returned positive ANCA and detectable pANCA results and at least 1 positive of the flagellin markers CBir1, anti-A4-FlaX, or anti-Fla2 or (2) detectable pANCA and at least 2 positives among the flagellin markers CBir1, anti-A4-FlaX, or anti-Fla2. In the training set, 68 of 1083 (6.3%) patients were labeled “inconclusive”; of these 68 patients, 30 (44%) patients were initially diagnosed with CD and 38 (56%) patients were initially diagnosed with UC. In the validation set, 32 of 437 (7.3%) patients were labeled “inconclusive”; of these 32 patients, 15 (47%) patients were initially diagnosed with CD, 16 (50%) patients were initially diagnosed with UC, and 1 (3%) patient was initially diagnosed with hepatitis.
The use of heterozygous and homozygous alleles in the Random Forest method showed that the predictive capability of the model was not as strong as the predictive performance obtained with homozygous allele alone. Therefore, the authors selected a model allowing homozygous individuals to be considered positive for the risk allele for each gene/SNP.
To calculate statistics for expected performance, the training set was resampled repeatedly. For each resampling iteration, a forest was built based on data from a randomly selected 2/3 of the patients in the training set and then tested on data from the remaining 1/3 of training set patients. After settling on the algorithm and on the biomarkers for each classification, the final Random Forests were built using the entire training set (refined for the CD versus UC Random Forest). Cutoffs for tuning sensitivity and specificity were selected based on these final forests’ performances on the validation data.
The importance of each biomarker in each Random Forest was calculated using the default Random Forest method, which determined the drop in each decision tree’s correct predictions after randomly scrambling the measures for the biomarker in question among out-of-bag samples. The reported importance score was the average drop (over all decision trees) for a specific biomarker divided by its variance. All analyses were performed using the R statistical programming language (R Development Core Team, 2008).
RESULTS
Study Cohort
The demographic characteristics of the IBD patient cohort (n = 900) are shown in Table 1. The median age of IBD diagnosis was 35 years for patients with CD and 30 years for patients with UC, with median disease duration of 7 years and 6 years, respectively. The majority of patients with IBD were diagnosed between ages 17 and 40 years using the Montreal Classification,22 and the majority of patients with IBD had CD (63.5%). Disease severity in patients with CD was assessed using the Harvey–Bradshaw Index,23 with 66.5% of patients with CD classified as being in remission. The severity of UC disease was assessed using the partial Mayo index,24 with 51.1% of patients with UC classified as being in remission. Medications were used during course of disease by a subset of patients with IBD (Table 1). The drug categories are not mutually exclusive. The non-IBD patient cohort was composed of 29.5% healthy volunteers, 24.2% noninflammatory controls, 19.3% inflammatory controls, and 26.9% with irritable bowel syndrome (Table 2). The median disorder duration ranged from 4 to 6 years, and the majority of patients with a disorder were diagnosed between ages 17 and 40 years. The demographics characteristics of the non-IBD patient cohort (n = 620) are shown in Table 2.
TABLE 1
Demographics of Patients with IBD in the Study Cohort
Demographics | CD (n = 572) | UC (n = 328) |
---|---|---|
Gender (% female) | 54.00 | 50.60 |
Smoking history (n = 518), % | 39.22 | 32.61 |
Median age at blood draw (range) (n = 900), yr | 35 (18–66) | 38 (18–65) |
Median IBD duration (range) (n = 816), yr | 7 (<1–44) | 6 (<1–50) |
Age at diagnosis (Montreal classification) (n = 890), % | ||
A1 (≤6 yr) | 15.60 | 10.00 |
A2 (≥17 and ≤40 yr) | 68.40 | 64.40 |
A3 (>40 yr) | 16.00 | 25.60 |
CD location (Montreal classification) (n = 425), % | ||
L1 | 21.37 | N/A |
L2 | 49.65 | N/A |
L3 | 20.24 | N/A |
L4 | 0.24 | N/A |
L1 + L4 | 1.18 | N/A |
L2 + L4 | 4.71 | N/A |
L3 + L4 | 2.35 | N/A |
UC location (Montreal classification) (n = 183), % | ||
E1 | N/A | 24.59 |
E2 | N/A | 33.88 |
E3 | N/A | 41.53 |
Behavior (Montreal classification) (n = 425), % | ||
B1 | 45.18 | N/A |
B1p | 1.58 | N/A |
B2 | 32.22 | N/A |
B2p | 1.23 | N/A |
B3 | 22.59 | N/A |
B3p | 9.81 | N/A |
Harvey–Bradshaw index (n = 334), % | ||
Remission (<5) | 66.50 | N/A |
Mild (5–7) | 17.40 | N/A |
Moderate/severe (8–33) | 16.20 | N/A |
Partial Mayo index (n = 184), % | ||
Remission (≤2) | N/A | 51.10 |
Mild (3–5) | N/A | 31.50 |
Moderate/severe (6–12) | N/A | 17.40 |
Medications taken during course of disease (n = 900), % | ||
Remicade | 33.00 | 14.30 |
Humira | 16.80 | 2.40 |
Cimzia | 5.60 | 0.00 |
Azathioprine | 26.20 | 18.60 |
N/A, not applicable.
TABLE 2
Demographics of Non-IBD Controls in the Study Cohort
Demographics | Healthy Volunteers (n = 183) | Noninflammatory Non-IBD Controls (Chronic Constipation; Functional Dyspepsia; GERD) (n = 150)a | Irritable Bowel Syndrome (n = 167) | Inflammatory Non-IBD Controls (Celiac Disease, Diverticulitis, Hepatitis, Microscopic Colitis, Pancreatitis) (n = 120)b |
---|---|---|---|---|
Gender (% female) | 65.6 | 65.3 | 83.8 | 55.8 |
Smoking history (%) | 16.4 | 42.0 | 35.3 | 39.2 |
Median age at blood draw (range), yr | 31 (18–66) | 48 (19–65) | 46 (20–65) | 52 (20–65) |
Median disorder duration (range), yr | N/A | 6 (0–40) | 5 (0–52) | 4 (0–39) |
Age at diagnosis (%), yr | ||||
≤16 yr | N/A | 4.0 | 6.0 | 0.8 |
≥17 and ≤40 yr | N/A | 45.3 | 55.7 | 30.8 |
>40 yr | N/A | 50.7 | 38.3 | 68.3 |
N/A, not applicable.
Overview of Individual Biomarker Characteristics
Serological, inflammatory, and genetic characteristics of the study population are shown in Table, Supplemental Digital Content 1, http://links.lww.com/IBD/A106. The frequency of anti-ASCA-IgA and ASCA-IgG serum positivity was 52.6% and 48.4% in patients with CD and 7.9% and 9.8% in patients with UC, respectively. OmpC positivity was found in 37.4% of patients with CD, in 22.0% of patients with UC, and 15.2% of non-IBD controls. The frequency of serum reactivity for anti-A4-Fla2, anti-CBir1, and anti-FlaX was 44.4%, 39.5%, and 44.4% in patients with CD and 15.9%, 17.4%, and 16.5% in patients with UC, respectively. Antibody levels were significantly different (P < 0.0001) between patient populations for all of the serological markers analyzed. ANCA and pANCA presence was significantly higher in the UC population at 62.8% and 61.6%, respectively, than in the CD (24.1% and 21.0%) and non-IBD (6.3% and 3.2%) populations (see Table, Supplemental Digital Content 1, http://links.lww.com/IBD/A106). The frequency of VEGF, ICAM-1, and VCAM-1 serum reactivity of IBD patients was 29.7%, 29.6%, and 29.2%, respectively, and 18.2%, 18.5%, and 19.0%, respectively, in non-IBD patients. All 3 of these inflammatory markers were significantly higher in the IBD patient population compared with the non-IBD population (P ≤ 0.005): however, no significant differences were observed between the CD and UC population (see Table, Supplemental Digital Content 1, http://links.lww.com/IBD/A106). CRP and SAA were detected in 33.9% and 37.6% of the patients with CD, respectively, and in 24.1% and 25.6% of the patients with UC, respectively. Non-IBD samples showed 17.3% and 13.1% CRP and SAA reactivity, respectively. CRP and SAA levels were significantly different between IBD versus non-IBD and CD versus UC patient populations (P ≤ 0.001, see Table, Supplemental Digital Content 1, http://links.lww.com/IBD/A106). For ATG16L1 and NKX2–3 homozygous risk alleles were present in 31.4% and 29.0% of IBD patients, respectively; this compared with 23.5% and 19.7% of non-IBD patients, respectively. The selective utility of these markers was demonstrated by the Random Forests algorithm ranking, which demonstrated that the ATG16L1 and NKX2–3 homozygous variant alleles were significantly associated with IBD (P < 0.001 and P < 0.0001, respectively) (see Table, Supplemental Digital Content 1, http://links.lww.com/IBD/A106).
Further analysis of the frequency of anti-A4-Fla2, anti-CBir1, and anti-FlaX (flagellins) reactivity in patients with CD demonstrated a complex relationship of positivity for these antibodies (Fig. 1 A). Approximately 46% of the CD population was negative for all 3 antibodies. Thus, the remaining 54% of patients with CD, in combination, reacted to at least 1 antigen. Approximately 32% of the patients with CD were reactive to all 3 antigens. The frequency of the serological markers ASCA-IgA, ASCA-IgG, OmpC, and all flagellins combined is shown in Figure 1B. Of the 83% of the CD population that reacted to at least 1 antigen, 16.8% of the patients were reactive to all of the markers. Similarly, the frequency of the acute inflammatory markers CRP and SAA is shown in Figure 2A for the CD and UC (IBD) population combined. Approximately 57% of the IBD population was negative for CRP and SAA. Approximately 42% of the IBD population was positive for at least 1 of these markers, and 22.3% were positive for both CRP and SAA. Figure 2B shows the interaction of serum reactivities for all of the inflammatory makers. Of the 65% of patients with CD that were positive for at least 1 of the 5 markers, 11.2% were reactive to all 5.
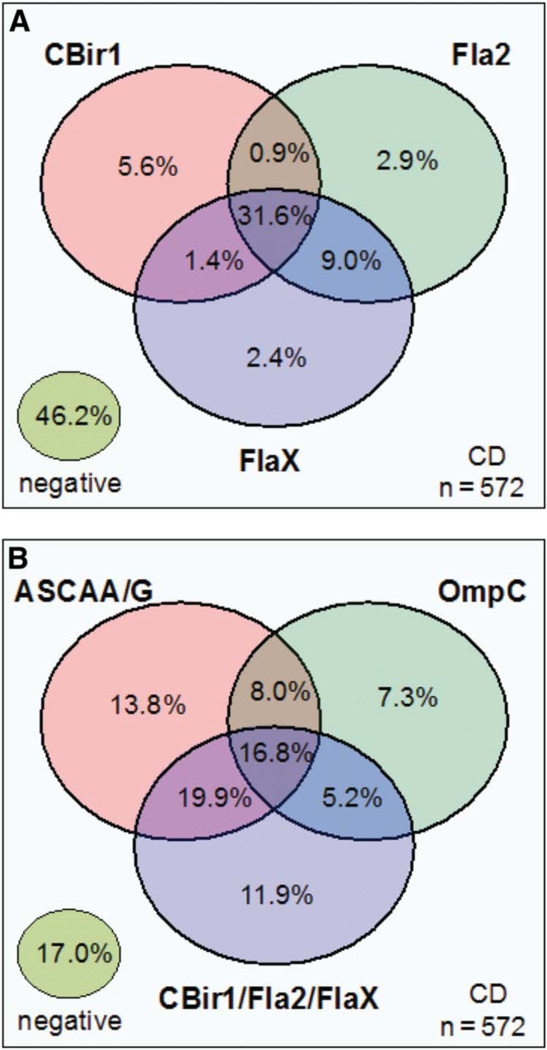
Venn diagram describing the relationships between serological markers in the CD cohort (n = 572). The percentage of positive patients for each marker or combination of markers is shown. A, The relationship between anti-CBirl, anti-A4-Fla2, and anti-FlaX. B, The relationship between ASCA-lgA/ASCA-lgG, OmpC, and the combination of anti-CBirl/anti-A4-Fla2/anti-FlaX.
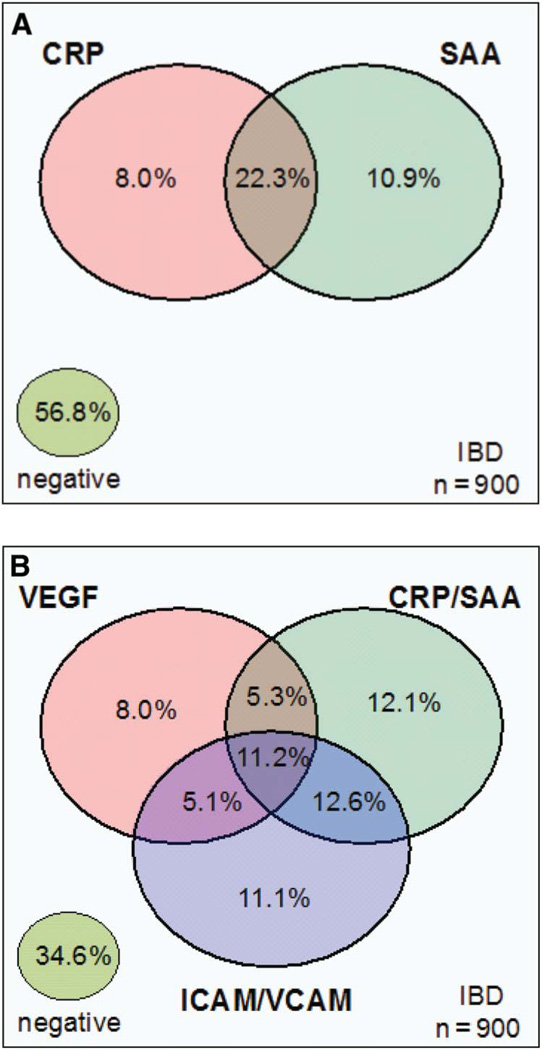
Venn diagram describing the relationships between the inflammatory markers. The percentage of positive patients for each marker or combination is shown. A, The relationship between CRP and SAA in the IBD cohort (n = 900). B, The relationship between the combination CRP/SAA, VEGF, and the combination of ICAM-1/VCAM-1 in the IBD cohort (n = 900).
The Use of Multiple Serological, Inflammatory, and Genetic Markers to Differentiate Disease Phenotype
Serological and inflammatory markers were used to differentiate between IBD and non-IBD phenotypes. Zero markers or 1 positive serological marker was significantly associated with the non-IBD phenotype, whereas the association of 2 or more positive serological markers was significantly associated with the IBD phenotype (P < 0.05) (Fig. 3A). Similarly, a combination of 2 or more positive inflammatory markers was significantly associated with the IBD phenotype, whereas the absence of inflammatory markers was significantly associated with the non-IBD phenotype (Fig. 3B). Subjects with fewer than 2 against 2 or more positive genetic markers were analyzed by disease status (non-IBD or IBD) using a 2-by-2 contingency table (see Table, Supplemental Digital Content 2, http://links.lww.com/IBD/A107). IBD subjects had significantly greater odds of belonging to the “2 or more” genetic marker group compared with non-IBD subjects (odds ratio, 1.53; 95% confidence interval [CI], 1.20–1.95, P = 0.0004).
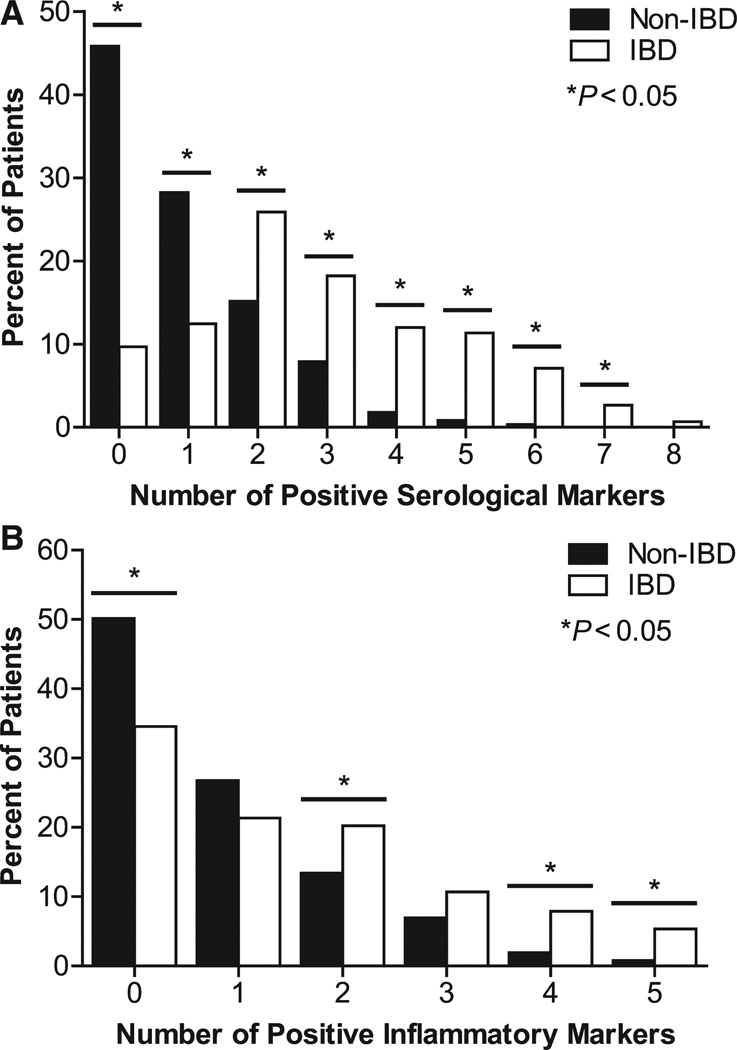
Differentiation between IBD and non-IBD patients was enhanced with biomarker combinations. A, The relationship between the number of positive serological markers (ASCA-lgA, ASCA-IgG, ANCA, anti-OmpC, anti-CBirl, anti-A4-Fla2, and anti-FlaX) and the percentage of IBD and non-IBD patients. B, The relationship between the number of positive inflammatory markers (CRP, SAA, VEGF, ICAM-1, and VCAM-1) and the percentage of IBD and non-IBD patients. A patient was considered positive if their biomarker measurement was equal to or above the third quartile for that marker’s measures.
The Use of a Random Forests Algorithm Using Serological, Inflammatory, and Genetic Markers to Differentiate Between IBD Versus Non-IBD and CD Versus UC
Area under the curve (AUC) values were derived using receiver operating characteristic analysis to plot true positive versus false-positive rates of decision criteria in the Random Forests model, thereby acting as a measure of each biomarker’s discriminating capacity (Fig. 4). A receiver operating characteristic curve was generated for each biomarker and for the serological, genetic, and inflammatory biomarker panels. The discriminating capacity considerably increased when the entire marker panel was used compared with individual markers (Fig. 4). AUCs were used to examine relative differences between chance (0.5) and the test performance. Using this metric, the IBD versus non-IBD Random Forests showed 95% more discrimination potential than ASCA-IgA alone ([0.871 – 0.5]/[0.690 – 0.5] = 1.95). The CD versus UC Random Forests had 36% more discrimination potential than did ASCA-IgA alone ([0.929 – 0.5]/[0.816 – 0.5] = 1.36) (data not shown). The sensitivity and specificity of the algorithm correctly classified 73.6% of IBD patients and 89.6% of non-IBD patients, respectively (Table 3). For IBD-predicted patients, the algorithm classified 88.9% of patients with CD and 97.7% of patients with UC correctly (Table 3). Positive predictive values and negative predictive values ranged from 70.5% to 98.9%, providing additional confidence in the sensitivity, specificity, and accuracy of the algorithm (Table 3).
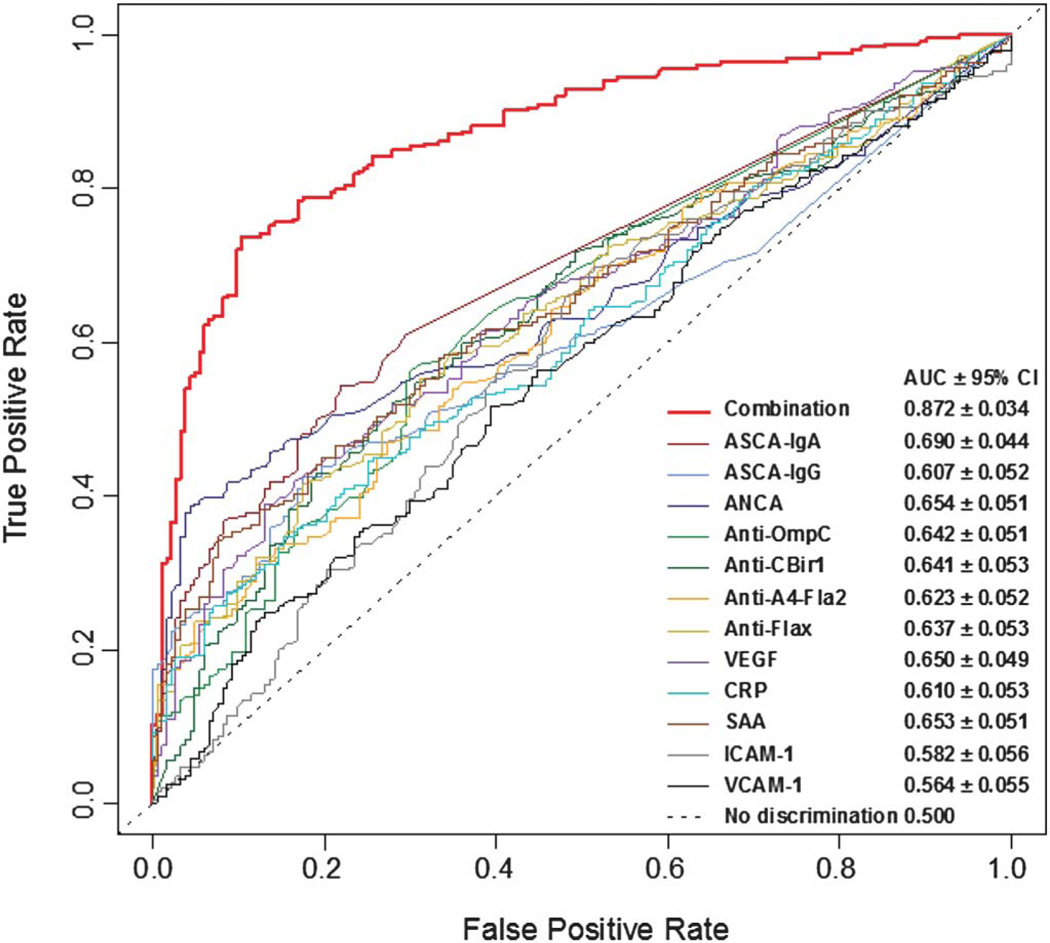
Receiver operating characteristic curves for discriminating IBD and non-IBD patients. AUC is shown for the combination of serological, genetic, and inflammatory markers and for the following continuous markers: ASCA-lgA, ASCA-IgG, ANCA, anti-OmpC, anti-CBirl, anti-A4-Fla2, anti-FlaX, CRP, SAA, ICAM-1, VCAM-1, and VEGF.
TABLE 3
Performance of Biomarkers in Identifying and Confirming IBD, CD and UC Status
IBD Status | Sensitivity (%) | Specificity (%) | PPV (%) | NPV (%) |
---|---|---|---|---|
IBD | 73.6 | 89.6 | 90.8 | 71 |
CD | 88.9 | 81 | 87 | 83.6 |
UC | 97.7 | 83.5 | 70.5 | 98.9 |
PPV, positive predictive value; NPV, negative predictive value.
The application of a Random Forest classifier to define IBD versus non-IBD status used biomarkers that were ranked in order of contribution to the model (see Table, Supplemental Digital Content 3, http://links.lww.com/IBD/A108). Serological markers contributed the most to the Random Forest model, whereas genetic biomarkers and VEGF (0.193) made a lower level of contribution to the Random Forest model.
In a direct performance comparison of the biomarker panel combination of serology, genetics, and inflammation markers against a panel built from the serological biomarkers only (ASCA-IgA, ASCA-IgG, OmpC, CBir1, ANCA, and pANCA) using 373 patient samples, the AUC for the IBD versus non-IBD with the panel combination was 0.87 (95% CI, ±0.04), whereas the AUC for the serological biomarkers was 0.80 (Fig. 5; 95% CI, ±0.05; P = 0.0001). Similarly, the AUC for CD versus UC with the panel combination was 0.93 (95% CI, ±0.04), whereas the AUC for the serological biomarkers was 0.78 (95% CI, ±0.06; P < 0.0001) (Fig. 5). Therefore, in a direct comparison, combining the 3 classes of biomarkers in this novel test provided a significant improvement in the diagnostic performance of the test when compared with a panel that includes serological markers only.
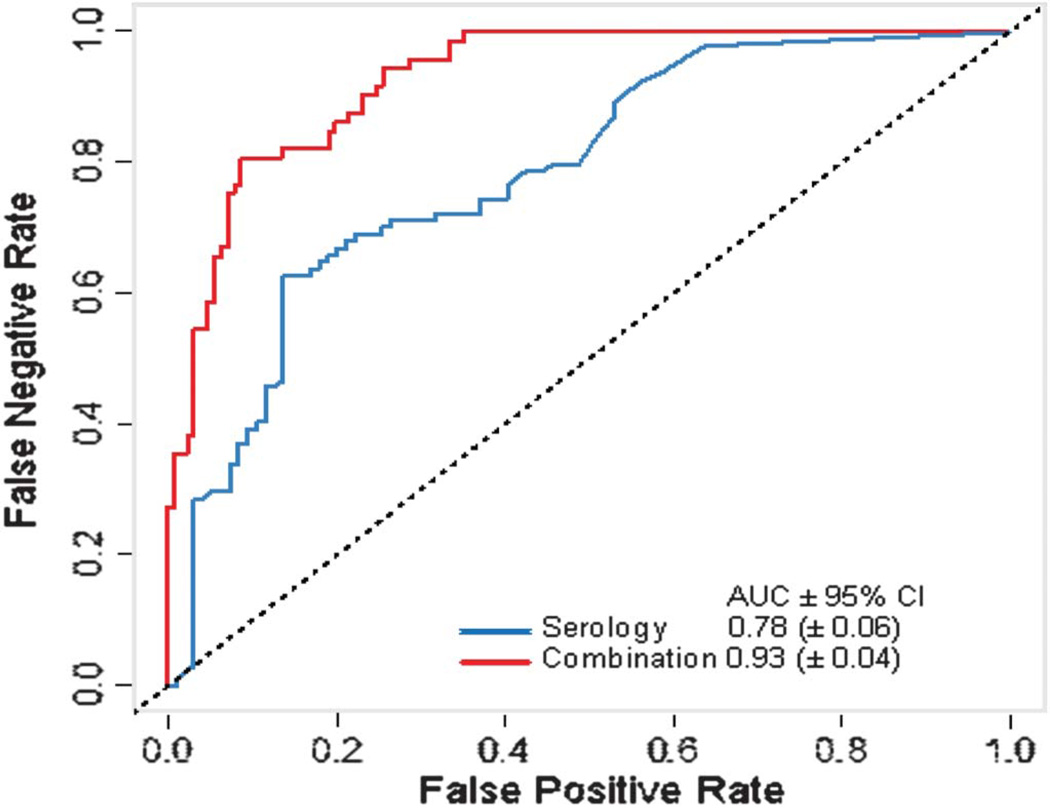
Receiver operating characteristic curves for discriminating patients with CD and UC in a direct performance comparison of the biomarker panel combination of serology, genetics, and inflammation markers against a panel built from the serological biomarkers alone (ASCA-IgA, ASCA-IgG, OmpC, CBirl, ANCA, and pANCA) using 373 patient samples.
DISCUSSION
This study used a combination of serological, inflammatory, and genetic markers to differentiate IBD from non-IBD patients and patients with CD from UC. A cross-sectional sample of 1520 subjects was used to train and validate the test algorithm developed using Random Forests models.21 The performance advantage of using a combination of serological, inflammatory, and genetic markers over algorithms that use only serological markers was demonstrated.
Previous research showed that the serological markers ASCA-IgA, ASCA-IgG, OmpC, CBir1, ANCA, and pANCA are associated with IBD. These markers are also known for their ability to discriminate between CD and UC.2,3,25–27 In this study, 2 recently described serological markers from the flagellin family, A4-Fla2 and FlaX, were introduced. The antibody response against these 2 markers has been shown to be associated with clinical CD phenotypes4 and also to discriminate between IBD versus irritable bowel syndrome,28 highlighting the major role of enteric bacterial flagellins as antigenic stimuli in IBD. In this study, an immune response against A4-Fla2 and FlaX was found in 14.3% of CBir1-negative patients. This result underlines the value of using newly described flagellin markers in conjunction with CBirl to help diagnose IBD and CD.
Inflammatory markers such as CRP are commonly used in IBD management; CRP is an acute-phase protein that correlates very well with disease activity in patients with IBD, although the correlation seems to be higher with CD compared with UC.28–30 However, a CRP response is not observed in some patients with inflammation even in the presence of known active disease.31 SAA is a non-specific acute-phase protein secreted in response to cytokines such as interleukin 1, interleukin 6, and tumor necrosis factor a.32,33 In this study, 10.9% of patients with IBD were both SAA positive and CRP negative, underlining the benefit of using and monitoring both markers. VEGF, a cytokine released by immune cells that potentiates vascular permeability and angiogenesis, is important in wound healing and is elevated in the serum of patients with IBD.11,12 ICAM-1 and VCAM-1 help regulate leukocyte adherence and infiltration to endothelium in inflammatory sites and are both subsequently elevated in patients with IBD.9 The 5 markers used in the inflammatory panel in this study may represent different stages of the inflammatory process and provide additive information that exceeds the information obtained from measuring CRP alone. These inflammatory markers were useful in distinguishing between IBD and non-IBD patients but not between UC and CD when used alone.
A significant issue regarding the use of inflammatory markers is the possibility of their variation over time, for example, it is possible that a patient in deep remission may have normal inflammatory markers. The premise of the clinical utility of the test is that it will be used as an aid in the diagnosis of IBD, and therefore, patients will be symptomatic at the time of use. However, in the study protocol, there was no prerequisite for the patients to have active symptoms at the time of the blood draw, and the discriminatory findings were still significant. These findings suggest that if only symptomatic patients were included in the analysis, which would reflect real-life clinical setting, a stronger association between inflammatory markers and IBD may have been observed.
In this study, only biomarkers that significantly contributed to the Random Forests model were included. A marker was considered a “significant contribution” to the Random Forests when its importance statistic was significantly higher than zero. Simple relationships can be captured in models such as logistic regression, which are often used in multimarker algorithms. An advantage to Random Forests modeling is that the algorithm automatically explores complex multivariates and can capture complexities in the interactions among predictive variables.21 Several significant findings have been noted; for example, the genetic markers STAT3 and ECM1 have been included in the marker selection because of their significant contribution to the Random Forests model. However, STAT3 and ECM1 alone were not able to significantly differentiate between IBD and non-IBD. Therefore, the source of their contribution must be an interaction with additional marker(s). Lunetta et al34 similarly observed that SNPs of interest in genome-wide association studies could be identified more efficiently in multimarker Random Forests screens than in single-marker screens because the algorithm is able to detect such interactions. All of the genetic markers included in this study were previously described in multiple cohort studies as being strongly associated with IBD.35–40
An intermediate stage to the diagnostic algorithm allowed for IBD-predicted subjects to be labeled as “inconclusive.” This selection occurred after the IBD classification of the samples was determined and before the CD and UC classification step. Although pANCA is predominantly associated with UC, a subgroup of patients with CD in the patient cohort used here had detectable pANCA and elevated flagellin levels. These subjects may represent an indeterminate form of IBD (IBD-U) or be characterized as “UC-like colitis,” where the inflammation usually involves the left side of the colon with UC-like features.2,41 This intermediate stage was implemented to prevent miss call for this type of subject because these individuals were not specifically represented in the training and validation cohort. As such, patients with IBD-U were not represented in the study because there was an insufficient number of samples and insufficient prospective follow-up to allow for meaningful analysis.
In this study, the predictive performance of a multimarker panel Random Forests model was greater compared with using individual serological markers (ASCA-IgA, ASCA-IgG, OmpC, CBir1, ANCA, and pANCA) alone to distinguish between IBD versus non-IBD patients and patients with CD versus UC. This approach illustrates the potential of using markers from different platforms to refine and improve their utility in clinical practice. Additional cohort studies will be necessary to confirm the model. Moreover, investigations are currently being conducted to address the question of marker stability over time and also to address marker behavior related to disease activity and treatments.
Supplementary Material
Suppl Table 1
Suppl Table 2
Suppl Table 3
Acknowledgments
Supported by Prometheus Laboratories Inc, San Diego, CA.
S. Lockton, E. Chuang, F. Princen, S. Singh, L. Croner, J. Stachelski, M. Brown, and C. Triggs are employees of Prometheus Laboratories Inc. T. Stockfisch is an employee of Stockfisch Consulting and provided bioinformatics consulting services funded by Prometheus laboratories Inc. S. Plevy and M. S. Silverberg have received consulting fees and research support from Prometheus Laboratories Inc.
Writing support was provided by Anthony Stonehouse and Rebecca Watson. Dr. Stonehouse and Dr. Watson are employees of Watson & Stonehouse Enterprises, LLC.
Footnotes
Supplemental digital content is available for this article. Direct URL citations appear in the printed text and are provided in the HTML and PDF versions of this article on the journal’s Web site (www.ibdjournal.org).
REFERENCES
Full text links
Read article at publisher's site: https://doi.org/10.1097/mib.0b013e318280b19e
Read article for free, from open access legal sources, via Unpaywall:
https://cdr.lib.unc.edu/downloads/9306t6206
Citations & impact
Impact metrics
Article citations
Noninvasive Stool RNA Test Approximates Disease Activity in Patients With Crohn's Disease.
Gastro Hep Adv, 3(8):1079-1086, 30 Jul 2024
Cited by: 0 articles | PMID: 39529640
Enhancing Calprotectin's Predictive Power as a Biomarker of Endoscopic Activity in Ulcerative Colitis: A Machine Learning Use Case.
Biomedicines, 12(3):475, 20 Feb 2024
Cited by: 0 articles | PMID: 38540089 | PMCID: PMC10968359
Serological Biomarker-Based Machine Learning Models for Predicting the Relapse of Ulcerative Colitis.
J Inflamm Res, 16:3531-3545, 21 Aug 2023
Cited by: 3 articles | PMID: 37636275 | PMCID: PMC10455884
Profiling the inflammatory bowel diseases using genetics, serum biomarkers, and smoking information.
iScience, 26(10):108053, 26 Sep 2023
Cited by: 3 articles | PMID: 37841595 | PMCID: PMC10568094
The effect of olsalazine of chinese generic drugs on ulcerative colitis induced by dextran sulfate sodium salt in BALB/c mice.
Acta Cir Bras, 38:e382923, 21 Aug 2023
Cited by: 1 article | PMID: 37610966 | PMCID: PMC10443231
Go to all (74) article citations
Data
Data behind the article
This data has been text mined from the article, or deposited into data resources.
BioStudies: supplemental material and supporting data
SNPs (3)
- (1 citation) dbSNP - rs2241880
- (1 citation) dbSNP - rs3737240
- (1 citation) dbSNP - rs744166
Similar Articles
To arrive at the top five similar articles we use a word-weighted algorithm to compare words from the Title and Abstract of each citation.
Serological markers predict inflammatory bowel disease years before the diagnosis.
Gut, 62(5):683-688, 26 Jul 2012
Cited by: 71 articles | PMID: 22842615
Retrospective evaluation of the clinical utility of serological biomarkers in Chinese patients with inflammatory bowel disease: 2-year clinical experience.
Clin Chem Lab Med, 55(6):865-875, 01 May 2017
Cited by: 2 articles | PMID: 27831916
Familial and sporadic inflammatory bowel disease: comparison of clinical features and serological markers in a genetically homogeneous population.
Scand J Gastroenterol, 37(6):692-698, 01 Jun 2002
Cited by: 46 articles | PMID: 12126248
Differentiating ulcerative colitis from Crohn disease in children and young adults: report of a working group of the North American Society for Pediatric Gastroenterology, Hepatology, and Nutrition and the Crohn's and Colitis Foundation of America.
J Pediatr Gastroenterol Nutr, 44(5):653-674, 01 May 2007
Cited by: 244 articles | PMID: 17460505