Abstract
Free full text

Seven challenges for neuroscience
Summary
Although twenty-first century neuroscience is a major scientific enterprise, advances in basic research have not yet translated into benefits for society. In this paper, I outline seven fundamental challenges that need to be overcome. First, neuro-science has to become “big science” – we need big teams with the resources and competences to tackle the big problems. Second, we need to create interlinked sets of data providing a complete picture of single areas of the brain at their different levels of organization with “rungs” linking the descriptions for humans and other species. Such “data ladders” will help us to meet the third challenge – the development of efficient predictive tools, enabling us to drastically increase the information we can extract from expensive experiments. The fourth challenge goes one step further: we have to develop novel hardware and software sufficiently powerful to simulate the brain. In the future, supercomputer-based brain simulation will enable us to make in silico manipulations and recordings, which are currently completely impossible in the lab. The fifth and sixth challenges are translational. On the one hand we need to develop new ways of classifying and simulating brain disease, leading to better diagnosis and more effective drug discovery. On the other, we have to exploit our knowledge to build new brain-inspired technologies, with potentially huge benefits for industry and for society. This leads to the seventh challenge. Neuroscience can indeed deliver huge benefits but we have to be aware of widespread social concern about our work. We need to recognize the fears that exist, lay them to rest, and actively build public support for neuroscience research. We have to set goals for ourselves that the public can recognize and share. And then we have to deliver on our promises. Only in this way, will we receive the support and funding we need.
Introduction
Twenty-first century neuroscience is a rapidly growing, large-scale scientific enterprise. According to PubMed, the number of published papers with the word “brain” in the title increased from fewer than 3000 per year in 1960 to more than 60000 in 2010 ( Fig. 1 ). The research going into these papers is supported by public funding amounting to more than $7 billion a year, mainly from the USA (about $5.6 billion) and the rest from EU countries and increasingly from other areas of the world.
Yet despite this enormous investment, the number of new drug and other treatments is decreasing and seems to be grinding to a halt. It can be argued that “small science” in neuroscience has failed to harvest our exponentially growing knowledge and turn it into a benefit for society. Neuroscience has also not delivered on many basic promises. After decades of effort, we still have only a very limited understanding of the mechanisms linking brain structure and function at the microscopic level to cognition and behavior or to the large-scale patterns of activity we observe in imaging studies. Neuroscience research has done little to halt the rising tide of brain disease, whose costs may soon reach 10% of world GDP. And it has yet to make a real contribution to computing technology. When a young child recognizes and grasps a furry toy, the child’s brain demonstrates image processing and motor control capabilities beyond those of our most powerful computers. In principle, neuroscience could reveal the biological mechanisms underlying these capabilities – allowing the development of a new generation of brain-inspired computing technology. To date we have failed to do this.
Human beings have a powerful urge to understand their own nature, and a strong practical need to cure brain disease and develop new computing technologies. These demands, combined with the new possibilities opened up by modern ICT and high throughput technology, are driving a rapid transformation of neuroscience in the direction of “big science” and big “data”. The last few years have seen the birth of pioneering efforts such as the Allen Brain Institute’s Brain Atlases, the Human Connectome Project, work at Cold Springs Harbor on the human projectome, the ADNI initiative and our own Blue Brain Project at EPFL. 2013 saw the announcement of the American BRAIN project and the EU-funded Human Brain Project, which I have the privilege to coordinate. Other countries like Canada and China are planning their own initiatives. These projects, and others working in the same direction, have the potential to finally realize the enormous scientific, medical and technological potential of modern neuroscience. But to do so there are still fundamental challenges that need to be overcome. In this paper, I will outline what I believe are the seven most important of these challenges.
Challenge 1. Change the way neuroscience is done
Delivering on the promise of neuroscience is not just a question of research methodology or technology – it implies a change in the structure and practices of our discipline. Big science initiatives in other disciplines such as physics or astronomy or genomics involve large multidisciplinary teams, close collaboration between scientists and engineers, and widespread sharing of data and tools, for example through the deposition of data in public repositories and the use of pre-print servers. In neuroscience, by contrast, most laboratories are relatively small and have only limited access to engineering resources. Despite the emergence of large resources, such as the Allen Brain Atlases, data sharing remains the exception rather than the rule. Attempts to bridge the gaps between different levels of brain organization are hampered by the fragmentation of the discipline into sub-disciplines each with its own journals, conferences, conceptual frameworks, vocabulary and experimental methods.
Neuroscience has the potential to make fundamental contributions to medicine, computing and our understanding of the human condition, but to do so it has to adopt forms of organization and modes of operation better adapted to the needs of big science. The first major challenge is thus to change the way neuro-science is done: to move away from small-scale collaborations towards large teams that bring together the huge range of competences and the technical and financial resources necessary to tackle the “big problems”. We have spent too long waiting for a new Einstein to unify our field. We have to unify it ourselves. The way to do so is to forget about our egos and seriously begin working together.
Challenge 2. Data ladders
The way neuroscience is currently organized has many practical implications for research. Groups working on different levels of brain organization work in different areas of the brain, in different animals, at different ages. Geneticists use mice, studies of neural microcircuitry focus on rats, most of our knowledge of the visual cortex comes from cats, and research on higher cognitive functions uses monkeys or human volunteers. Of course, there are good technical and scientific reasons for this diversity: for instance, most of our current genetic technologies have been developed in mice. However, the lack of a unified strategy has two negative consequences. The first is that there is still not a single area of the brain, in any species, for which we have data spanning all its different levels of organization. This means we have no way of identifying or experimentally manipulating the biological mechanisms linking lower and higher levels. The second negative consequence is that we lack the data to correlate observations in one species with observations in another. In particular, we are missing the systematic knowledge we would need to extrapolate results from animal experiments to humans – where many kinds of experiment are technically or ethically impossible. The second major challenge is thus to create “data ladders” – interlinked sets of data providing an increasingly complete picture of a single area of the brain at different levels of organization (molecules, cells, microcircuits, brain areas, etc.) with “rungs” linking the descriptions for homologous areas in humans and other species ( Fig. 2 ). Creating such ladders is an example of what we can achieve if we can transform the organization and practices of neuroscience along the lines outlined in challenge 1.
Challenge 3. Predictive neuroscience
“Big neuroscience” faces challenges that are even harder than the challenges addressed by previous “big science” projects. Consider, for instance, the Human Genome Project. The goal was to measure the approximately 3 billion base pairs of the human genome – a huge but not intractable challenge. The problem facing neuroscience is much larger – and harder to define. To completely characterize the human brain, we would need to measure more than 80 billion neurons, and more than 80 trillion synapses each with its own characteristic structure and electrophysiological behaviors, not to mention their subcellular structures and the innumerable molecular interactions that regulate their development and behavior.
Instead of measuring every individual neuron and each individual synapses – an impossible task – we could characterize the pathways between specific types of neuron. But even then the problem remains extremely hard. Our own studies of the cortical column show that a single column contains more than a thousand such pathways. But after decades of research we have detailed characterizations of just twenty – at a cost of about one million dollars per pathway. Given these numbers, it is evident that we will never be able to measure each of these pathways experimentally. The alternative is to predict the value of key parameters from data that is more readily available. For instance, we have recently published a technique that makes it possible to reliably predict the characteristics of synaptic pathways from the composition of a particular area of the brain – the number of cells belonging to different neuron types – and from 3D reconstructions of their morphology ( Hill et al., 2012).
This is only an example of what is possible. The literature shows that we can apply predictive strategies to many different levels of brain organization. Examples of work in this area include a recently published algorithm that can synthesize a broad range of neuron dendritic morphologies ( Cuntz et al., 2010), algorithms to generate specific motifs in network connectivity ( Song et al., 2005), and algorithms to predict synaptic strength based on network architecture ( Perin et al., 2011). In another area of research, recent work has demonstrated that biophysical models of neurons’ electrophysiological properties can successfully predict ion channel distributions and densities on the cell surface ( Hay et al., 2011).
By combining these predictions with cellular composition data, it is possible to predict protein maps for neural tissue. Finally, predictive methods can help to resolve one of the most important challenges for modern neuro-science, namely the classification and categorization of different types of cortical interneurons ( Ascoli et al., 2008). A recent model uses gene expression data to predict type, morphology and layer of origin with over 80% accuracy ( Khazen et al., 2012). The same model reveals rules for the combinatorial expression of ion channel genes.
The third challenge facing neuroscience is to develop these strategies further – in the Human Brain Project we are applying them to sixteen different prediction problems. Only in this way can we obtain the data we need to model and simulate the brain.
Challenge 4. Simulating the brain
How does the brain compute? What are the computational principles that allow it to model, predict, perceive and interact with the outside world? How does the brain implement these principles? To answer these fundamental questions, we need data ladders – but data and correlations among data sets are not enough. What we need to identify are causal mechanisms: for example we need to understand the way neurotransmitters and hormones modulate neural activity, synaptic transmission and plasticity, or, at a higher level, the way the brain “binds” information from multiple visual areas to form a unified picture of the world.
The classical way to establish causation is through experimental manipulation of living brains or tissue samples combined with simultaneous measurements of the response. But experiments in humans and animals are technically difficult, expensive and often cannot answer the questions we need to ask. For ethical and technical reasons, most invasive techniques are impossible to use on humans. Non-invasive imaging methods lack the spatial and temporal resolution to probe detailed neuronal circuitry. Working in animals, there are technical limitations on how many experiments we can perform and how much information we can extract from each experiment.
In other words, neuroscience is in a position similar to that of cosmology or climatology – sciences in which opportunities for experiments are strictly limited. In each of these disciplines, researchers investigate causal mechanisms, not by manipulating a physical system (it is hard to manipulate the cosmos!) but by building computer models of the system and manipulating the models in in silico experiments. Obviously, every model needs to be validated. But once it has been demonstrated that it effectively replicates a particular class of experimental observation, it becomes a new class of experimental tool.
Simulation offers huge advantages to neuroscience. There are no limitations on what we can record: so long as a parameter is represented in the model, we can measure it. Potentially, simulation allows us to record from millions or billions of neurons at a time. There are also no limits on the number of manipulations we can perform: with simulation we can perform systematic studies, unthinkable in animals or in tissue samples. Another advantage is that experiments are perfectly replicable – simulation models are not affected by the variability present even in the best-designed biological experiments. Simulation makes it possible, for the first time, to build bridges between different levels of brain organization. The cortical column we are modeling in the Blue Brain Project represents just one pixel in an image coming from an fMRI study. By modeling and manipulating multiple columns, we can begin to understand the low-level mechanisms underlying the higher-level patterns of activity we observe in our imaging studies.
At the time of writing, we can create cellular-level models of a few tens of cortical columns in the brain of a juvenile rat. But in the next ten years, the Human Brain Project will develop first draft cellular-level models of whole rodent brains and eventually of the whole human brain. With the development of human brain models, simulation will begin to show its full potential. If we want to study the low-level biological mechanisms responsible for human cognitive capabilities and the breakdown of these mechanisms in disease, we will not be able to use the same invasive approaches we use in animals. In silico manipulations and recordings will become our main experimental tool.
Realizing the potential of simulation calls for major technological innovation in high-performance computing. Detailed simulations of the brain have huge memory footprints. Thus, simulating the human brain will require new computer memory hardware, and new ways of managing very large volumes of memory. Very large brain simulations will require new numerical techniques making it possible to efficiently solve huge numbers of differential equations. We will need multi-scale simulation techniques making it possible to simulate some “regions” in greater detail than others. We will need to simulate aspects of the brain that are not yet included in our models: plasticity, the rewiring and pruning of neural circuitry, the role of neuromodulation, glia cells and the vasculature. Researchers performing in silico experiments will require virtual instruments equivalent to the physical instruments they use in the lab – virtual microscopes, virtual imaging technology. Implementing such instruments will require new forms of interactive supercomputing and supercomputer visualization.
Finally, to fulfill the promise of brain simulation we will need the ability to study how the brain gives rise to behavior. In other words, we have to “close the loop”, simulating how a model brain can control a body interacting with the physical world. Thus, we will need to simulate not just the brain, but also the body, the interface between the brain and the body, the physical world the body inhabits, and their interactions.
Each of these tasks will require radically new hardware and software. Thus, the fourth challenge for neuroscience goes beyond neuroscience. To simulate the brain, we first have to develop the hardware and software we need to do so.
Challenge 5. Classifying and simulating diseases of the brain
According to a recent report, nearly one-third of the citizens of the EU will be affected by psychiatric or neurological disease (anxiety, mood disorders, neurodegenerative disease, etc.) at least once in their life. The cost of brain disease to the European economy has been estimated at nearly Eur 800 billion per year, accounting for 25% of the total direct costs of healthcare (costs borne by national health services, insurance companies, and patients’ families) and a very considerable indirect cost (lost working days for patients and their carers) ( Gustavsson et al., 2011). Meanwhile, the cost of developing new CNS drugs is rising exponentially, largely due to high failure rates in phase III clinical trials. As a result, pharmaceutical companies are shutting down their neuroscience research labs and shifting their resources to other, more profitable areas of medicine and the rate of drug discovery is falling ( Fig. 3 ) ( Abbott, 2011). Many of the drugs we currently use date back to the 1980s and 1990s or even to the 1950s. Almost none are curative.
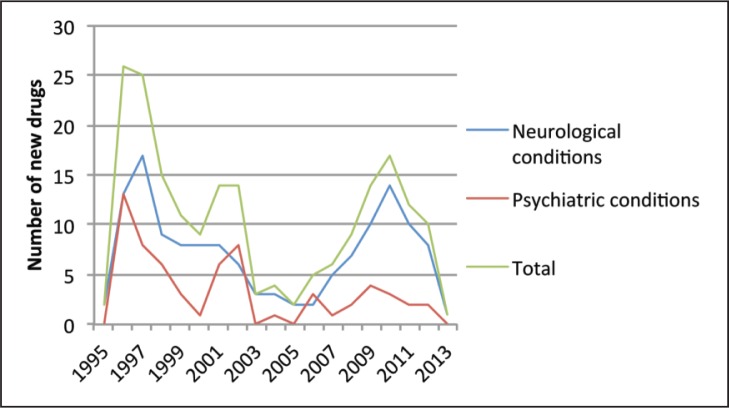
Number of new drugs registered by the FDA for sale in the USA (calculated from data provided by http://www.centerwatch.com/drug-information/fda-approvals/).
Neurological and psychiatric disease begins with an initial change in the brain – sometimes triggered by events in the patient’s external environment – followed by a cascade of knock-on effects. The fundamental reason it is so difficult to diagnose and treat brain disease is that we lack an adequate understanding of these cascades. As a result, most brain diseases are diagnosed not in terms of objective biological markers such as those we use to diagnose cancer or cardiovascular disease but by cognitive and behavioral symptoms, grouped into syndromes. This creates a severe risk of misdiagnosis – autopsy studies suggest that as many as 20% of cases of Alzheimer’s disease are misdiagnosed ( Beach et al., 2012). It also means many diseases (including Alzheimer’s) are diagnosed at late stages in which they have already caused irreversible damage. Meanwhile, pharmaceutical companies are forced to invest in drug candidates without fully understanding their mechanism of action or their potential side effects. Critically, there is currently no drug for the central nervous system (CNS) for which we have a full picture of its impact at all relevant levels of biological organization, from genes, ion channels and receptors through to cells, circuits, and the whole brain. Poor diagnostic methods and the lack of reliable biomarkers make it hard for researchers to select drug targets and candidate molecules, hard to choose patients for trials, and hard to measure outcomes. In these conditions, it is not surprising that trials of CNS drugs are long, require large numbers of participants and have higher rates of failure than trials for other indications. Worse, trials often fail to identify potentially valuable drugs that are effective only for a subgroup of patients ( Pangalos et al., 2007).
The fifth challenge facing neuroscience is to help resolve this impasse. To do so, we need to characterize the way diseases of the brain modify the structure and function of the brain at its different levels of organization. As in studies of the healthy brain, the first step will be to gather the data we need – in this case, very large sets of genetic data, lab results, imaging, and clinical observations from patients with the broadest possible range of pathologies. The data we need exists: hospital archives store vast volumes of data about patients; clinical trials and long-term longitudinal studies have accumulated enormous databases. However, we still need technical, legal and organizational solutions to federate this data and make it available to researchers, while simultaneously satisfying legitimate concerns about privacy and data protection.
The second step will be to analyze and cluster the data – identifying groups of patients whose biological data show common patterns, e.g. mutations in the same genes, similar patterns of gene expression, similar modifications in the large features of neuroanatomy or brain activity, as detected by imaging. The discovery of common patterns will make it possible to develop objective classifications of diseases, allowing physicians to diagnose diseases of the brain in terms of their unique biological signatures. New diagnostic methods, based on these classifications, will ensure that patients receive the therapies best adapted to their conditions, providing new opportunities for personalized medicine. The new methods will also make it easier for pharmaceutical companies to select participants for clinical trials and to measure the outcomes. In a longer-term perspective, they will make it possible to modify models of the healthy brain to reproduce the biological signatures of disease. In medicine as in neuroscience, brain modeling and simulation will allow in silico experiments that are ethically or technically impossible with any other technique. In particular, simulation will enable researchers to systematically explore alternative intervention strategies before embarking on costly animal studies and clinical trials. Neuroscience has the potential to accelerate drug discovery, reduce failure rates in trials and cut the cost of CNS drug discovery. It is a moral imperative that it realize that potential.
Challenge 6. From the brain to brain-inspired technology
The human brain is the world’s most sophisticated information processing machine, yet it operates on computational principles that seem to be completely different from those of conventional computing technology. These principles – which we have still to properly understand – allow it to solve computational problems that are difficult or intractable with current computing technology, all while consuming about 30W of power. They allow it to learn new skills without explicit programming. They ensure that it can operate reliably even when many of its components fail. These are highly desirable characteristics for future generation computing technologies.
In the coming years, neuroscience will learn more and more about the brain’s unique ability to model and predict the outside world, about the basic computational principles underlying this ability, and about the biological mechanisms implementing these principles at different levels of brain organization. In particular, experimental research combined with modeling and simulation will help us to distinguish aspects of neurobiology that are essential to the brain’s computational and cognitive capabilities from details that are not directly relevant to brain function. The sixth challenge for neuroscience is to translate this fundamental knowledge into brain-inspired technologies, directly inspired by the architecture of the brain.
There are many problems we will need to solve. Some are engineering issues. Future “neuromorphic” computing systems will contain millions and ultimately billions of artificial neurons. Implementing such systems will require new hardware technologies; designing, configuring, testing and using them will require new software. But many of the key issues have a direct tie to neuroscience. Given the limitations of our hardware technology, we will need techniques that allow us to simplify our brain models, while conserving the functionality we wish to replicate in technology. This will require a deep theoretical understanding of the way the brain implements its computational principles. Knowledge of the cognitive architectures underlying capabilities such as visual perception can help us to design computing systems offering functionality completely absent in current systems.
These are the challenges. The prize at stake is a completely new category of computing technology, with potentially huge benefits for industry and society.
Challenge 7. Working with society
Every neuroscientist is aware of widespread social concern about our work. There is concern about our research methods. Animal experimentation is increasingly unpopular – not just among activists and extremists. Proposals to re-use clinical data in research arouses concerns about privacy and consent. Perhaps more critically, many sectors of public opinion are frightened by claims about what we are likely to achieve. For many, understanding the brain is one more step in the “disenchantment of the world”. What would it mean for our perceptions of ourselves as human beings if we finally understood the biological mechanisms underlying human decision-making, human emotions, our perceptions of beauty, our sense of right and wrong? What would it mean for our concepts of free will and moral responsibility? What would it mean for our system of criminal law? We have to recognize that these are deeply rooted concerns.
Other fears focus on possible technological applications of neuroscience results – some real, some imaginary. For instance, media reports of experiments in “mind-reading” and transcranial magnetic stimulation have raised concerns that future technologies could be used to probe or manipulate people’s inmost thoughts. Work on the neural correlates of violent or other forms of deviant behavior raises the specter of preventative legal measures against citizens deemed to be at high risk – before they have committed a crime.
In computing, the idea of brain simulation and of brain-inspired computing technologies has led to speculations about new forms of artificial intelligence, more powerful than human intelligence. More realistically, the possibility of a new category of neuromorphic computing technology with strong disruptive potential raises a broad range of concerns, from worries about military applications, and applications for mass surveillance, to questions concerning the impact on industry and employment.
Some of the issues raised by pundits and the media are due to a misapprehension of the current state of neuroscience and technology. However, the fears to which they give rise are absolutely real.
The seventh, vital challenge for neuroscience is to recognize these fears, lay them to rest, and actively build public support for neuroscience research. For neuroscience to deliver on its promise it is not enough that society tolerates what we are doing – leaving us alone in our laboratories. We need society’s active support. This means we have to take public concerns seriously – even when they seem to be irrational or ill-founded. This means we should not denigrate our opponents. It means we should work hard to educate the public about our goals, methods and results.
However, first and foremost it means we have to set goals for ourselves that the public can recognize and share. And then we have to deliver on our promises. Only if we do this, engaging and informing the public, will we receive the support and funding we need to address the other challenges I have outlined in this paper.
References
- Abbott A . Novartis to shut brain research facility . Nature . 2011 ; 480 : 161 – 162 . [Abstract] [Google Scholar]
- Ascoli GA , Alonso-Nanclares L , Anderson SA , et al. Petilla terminology: nomenclature of features of GABAergic interneurons of the cerebral cortex . Nat Rev Neurosci . 2008 ; 9 : 557 – 568 . [Europe PMC free article] [Abstract] [Google Scholar]
- Beach TG , Monsell SE , Phillips LE , et al. Accuracy of the clinical diagnosis of Alzheimer disease at National Institute on Aging Alzheimer Disease Centers, 2005–2010 . J Neuropathol Exp Neurol . 2012 ; 71 : 266 – 273 . [Europe PMC free article] [Abstract] [Google Scholar]
- Cuntz H , Forstner F , Borst A , et al. One rule to grow them all: a general theory of neuronal branching and its practical application . PLoS Comput Biol . 2010 ; 6 : e1000877 . [Europe PMC free article] [Abstract] [Google Scholar]
- Hay E , Hill S , Schürmann F , et al. Models of neocortical layer 5b pyramidal cells capturing a wide range of dendritic and perisomatic active properties . PLoS Comput Biol . 2011 ; 7 : e1002107 . [Europe PMC free article] [Abstract] [Google Scholar]
- Hill SL , Wang Y , Riachi I , et al. Statistical connectivity provides a sufficient foundation for specific functional connectivity in neocortical neural microcircuits . Proc Natl Acad Sci U S A . 2012 ; 109 : E2885 – E2894 . [Europe PMC free article] [Abstract] [Google Scholar]
- Khazen G , Hill SL , Schürmann F , et al. Combinatorial expression rules of ion channel genes in juvenile rat (Rattus norvegicus) neocortical neurons . PLoS One . 2012 ; 7 : e34786 . [Europe PMC free article] [Abstract] [Google Scholar]
- Pangalos MN , Schechter LE , Hurko O . Drug development for CNS disorders: strategies for balancing risk and reducing attrition . Nat Rev Drug Discov . 2007 ; 6 : 521 – 532 . [Abstract] [Google Scholar]
- Perin R , Berger TK , Markram H . A synaptic organizing principle for cortical neuronal groups . Proc Natl Acad Sci U S A . 2011 ; 108 : 5419 – 5424 . [Europe PMC free article] [Abstract] [Google Scholar]
- Song S , Sjöström PJ , Reigl M , et al. Highly nonrandom features of synaptic connectivity in local cortical circuits . PLoS Biol . 2005 ; 3 : e68 . [Europe PMC free article] [Abstract] [Google Scholar]
- Gustavsson A , Svensson M , Jacobi F , et al. Cost of disorders of the brain in Europe 2010 . Eur Neuropsychopharmacol . 2011 ; 21 : 718 – 779 . [Abstract] [Google Scholar]
Articles from Functional Neurology are provided here courtesy of CIC Edizioni Internazionali
Citations & impact
Impact metrics
Article citations
Review of the Brain's Behaviour after Injury and Disease for Its Application in an Agent-Based Model (ABM).
Biomimetics (Basel), 9(6):362, 14 Jun 2024
Cited by: 0 articles | PMID: 38921242 | PMCID: PMC11202129
Review Free full text in Europe PMC
Flagship Afterthoughts: Could the Human Brain Project (HBP) Have Done Better?
eNeuro, 10(11):ENEURO.0428-23.2023, 14 Nov 2023
Cited by: 3 articles | PMID: 37963651 | PMCID: PMC10646882
Determining Commonalities in the Experiences of Patients with Rare Diseases: A Qualitative Analysis of US Food and Drug Administration Patient Engagement Sessions.
Patient, 17(1):25-37, 13 Oct 2023
Cited by: 0 articles | PMID: 37833521
Convergence of Artificial Intelligence and Neuroscience towards the Diagnosis of Neurological Disorders-A Scoping Review.
Sensors (Basel), 23(6):3062, 13 Mar 2023
Cited by: 7 articles | PMID: 36991773 | PMCID: PMC10053494
Review Free full text in Europe PMC
Neuroscience Knowledge and Endorsement of Neuromyths among Educators: What Is the Scenario in Brazil?
Brain Sci, 12(6):734, 02 Jun 2022
Cited by: 0 articles | PMID: 35741619 | PMCID: PMC9221520
Go to all (29) article citations
Similar Articles
To arrive at the top five similar articles we use a word-weighted algorithm to compare words from the Title and Abstract of each citation.
A unified platform to manage, share, and archive morphological and functional data in insect neuroscience.
Elife, 10:e65376, 24 Aug 2021
Cited by: 9 articles | PMID: 34427185 | PMCID: PMC8457822
The future of Cochrane Neonatal.
Early Hum Dev, 150:105191, 12 Sep 2020
Cited by: 5 articles | PMID: 33036834
Discovery and integrative neuroscience.
Clin EEG Neurosci, 36(2):55-63, 01 Apr 2005
Cited by: 7 articles | PMID: 15999900
Review
A repository based on a dynamically extensible data model supporting multidisciplinary research in neuroscience.
BMC Med Inform Decis Mak, 12:115, 08 Oct 2012
Cited by: 4 articles | PMID: 23043673 | PMCID: PMC3560115