Abstract
Free full text

Genetic variation of a bacterial pathogen within individuals with cystic fibrosis provides a record of selective pressures
Associated Data
Abstract
Advances in sequencing have enabled the identification of mutations acquired by bacterial pathogens during infection1-10. However, it remains unclear whether adaptive mutations fix in the population or lead to pathogen diversification within the patient11,12. Here, we study the genotypic diversity of Burkholderia dolosa within people with cystic fibrosis by re-sequencing individual colonies and whole populations from single sputum samples. Extensive intra-sample diversity reveals that mutations rarely fix within a patient's pathogen population—instead, diversifying lineages coexist for many years. When strong selection is acting on a gene, multiple adaptive mutations arise but neither sweeps to fixation, generating lasting allele diversity that provides a recorded signature of past selection. Genes involved in outer-membrane components, iron scavenging and antibiotic resistance all showed this signature of within-patient selection. These results offer a general and rapid approach for identifying selective pressures acting on a pathogen in individual patients based on single clinical samples.
Two opposing models of within-patient bacterial evolution have been proposed: a “dominant lineage” model, in which beneficial mutations drive superior lineages to dominate in the population, and a “diverse community” model whereby adaptive lineages rise to intermediate frequency and coexist with other lineages (Fig. 1)11-14. The diversity of within-patient pathogen populations has major implications for drug treatment and resistance7,15,16, for inferring transmission networks8,9,17,18, and for understanding evolutionary processes13,19. Here, to distinguish between these models and to understand the sources of genetic diversity, we compared the genomes of many bacterial cells of the same strain from the same clinical sample.
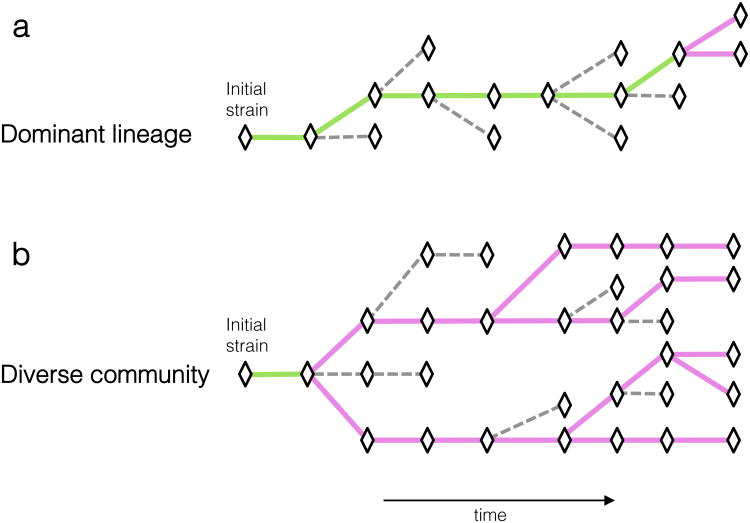
(a) In the dominant-lineage model of within-host evolution, lineages with beneficial mutations sweep to fixation (green lines), eliminating their less fit ancestors or other temporarily arising genotypes (dashed lines). In this model, most observed mutations will be fixed and polymorphic mutations will be rare, representing only recent mutational events (magenta lines). (b) In the diverse community-model, lineages coexist and compete for long stretches of time. In this model, most sampled mutations will be polymorphic.
We focused on chronic infections with Burkholderia dolosa, a rare and deadly opportunistic pathogen that spread among 39 people in with cystic fibrosis (CF) cared for at a single center in Boston starting in the 1990s20,21. The airways of these patients were infected with very similar starting strains, and surviving patients have been colonized for years. A previous retrospective study of single-colony isolates revealed specific B. dolosa genes that evolved under strong selective pressures during the outbreak8. Now, using sputum samples collected during clinical care, we characterize contemporary intraspecies diversity in 5 individuals from this outbreak who have been infected with B. dolosa since the early 2000's.
We used two genomic approaches, colony re-sequencing (Patient 1) and deep population sequencing (Patients 1-5), to identify single nucleotide mutations and their frequencies within single sputum samples. In our colony re-sequencing approach, we isolated dozens of colonies from a clinical sample and analyzed their genomes individually by alignment of reads to a B. dolosa reference genome, AU0158, a strain taken from a different patient in this outbreak. Since each colony originates from a single bacterium, this approach is equivalent to comparing different bacterial cells from the initial clinical sample. In the population sequencing approach22,23, we pooled hundreds of colonies from each clinical sample and sequenced the pool with deep coverage (~450×). We then aligned reads to AU0158 and identified fixed mutations, appearing in all reads, and polymorphisms, appearing in only a fraction of the reads. To remove false positive polymorphic sites caused by systematic sequencing or alignment errors24,25, we developed a set of thresholds and statistical tests that reject polymorphic sites where the mutated and ancestral reads have significantly different properties22,23 (see Supplementary Note). We calibrated this approach using an isogenic control for which we expect no polymorphisms. For validation, we performed both methods on a single sample from Patient 1, comparing diversity among 29 individual colonies to the population sequencing approach (Fig. 2). The population sequencing approach reliably detects polymorphisms where the minor allele frequency is larger than 3%, while decreasing the cost and labor required per sample.
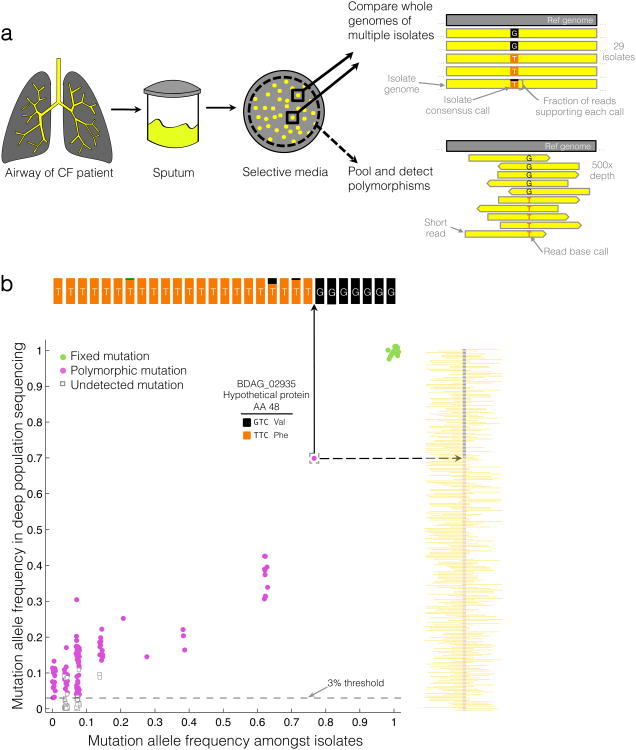
(a) To study within-patient evolution, we cultured sputum samples from patients with cystic fibrosis on selective media. In the colony re-sequencing approach (solid arrows, performed for one patient), we isolated multiple individual colonies from the same single sample, independently called variants for each isolate via alignment of reads, and compared variants among the isolates. In the deep population sequencing approach (dashed arrow, performed for five patients), we pool hundreds of colonies from the same plate and analyze the pool's genomic DNA. We identified positions on the genome where some reads, originating from different colonies on the plate, disagree with an inferred ancestral genome (Online Methods). (b) Allele frequency estimates in the population sequencing (y-axis) versus the colony re-sequencing (x-axis) from the same sputum sample (P1) for each mutated position. Mutations are classified as either fixed (green circles) or polymorphic (magenta circles). Some mutations found in the colony-based approach are sub-threshold in frequency or confidence in the pool-based approach (open squares). Slight jitter is added in the X and Y locations for each point to improve visibility (up to 2% change). As an example, the insets at top and at right display a summary of the raw data at the indicated genomic position. The population sequencing (right) at this position shows 70% aligned reads supporting a T (orange) and 30% supporting a G (black), consistent with the corresponding number of colonies in the individual isolates (22, T; 7, G). Reads from each isolate (top) are mostly of identical calls (all T, or all G). Green indicates a single read in one isolate supporting an A, likely a sequencing error. For further comparison of the two methods, see Supplementary Figure 7.
We found that most mutations that arise during the course of infection do not fix, but remain polymorphic within the patient. The colony re-sequencing approach performed for Patient 1 revealed 188 mutations occurring in some, but not all, isolates and only 10 mutations shared among all isolates. This dominance of polymorphisms, also seen in the population sequencing from the same sample, strongly supports the diverse community model (Fig. 3a-b). Similarly, for the four other patients, population sequencing on single samples identified a preponderance of polymorphisms compared to fixed mutations (≥73% of mutations, Fig. 3b). We found these excesses despite the bias to overestimate mutations fixed during infection; some fixed mutations in a sputum sample might be polymorphic within the patient's airways or have fixed prior to patient colonization (Supplementary Fig. 1).
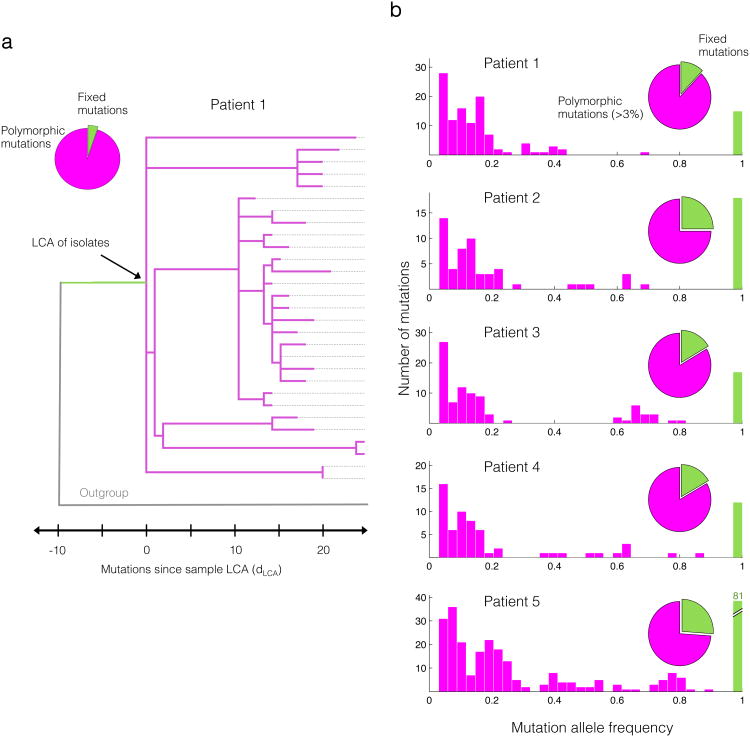
Mutations found in B. dolosa within-patient populations relative to the outgroup are classified as fixed (green), or polymorphic (magenta). An excess of polymorphic versus fixed mutations supports the diverse-community model over the dominant-lineage model. (a) A maximum-parsimony phylogeny of 29 isolates from the same sputum sample (P1) shows the coexistence of diverse sub-lineages separated by many single nucleotide mutations accumulating since the last common ancestor (LCA) of this patient. Each isolate is represented by a dotted line. (b) The diverse-community model is also supported by the distribution of allele frequencies from the population sequencing in 5 patients' samples.
The observed genomic diversity is a reflection of multiple coexisting lineages. Investigating the community structure of B. dolosa within Patient 1, we found a deeply branched phylogeny with 6 lineages separated by at least 5 lineage-specific mutations (Fig. 3a). On average, pairs of isolates from this sample differed by 26 mutations, and, of all 406 possible isolate pairs, only one was identical. Thus, even within a single sputum sample, the population is so diverse that full identity between isolates is extremely rare.
In one patient (Patient 5), the B. dolosa community had many more mutations than other patients' populations (P < 0.05, Grubbs' test for outliers). This excess of mutations is due solely to increased transitions and not transversions, suggesting hypermutation (Supplementary Fig. 2a, P < 0.01, Grubbs' test). A search of the 199 mutated genes unique to Patient 5's population revealed a single mutation involved in DNA repair: a nonsynonymous mutation at a conserved position in mutL, defects of which are known to cause excess transitions26 (Supplementary Fig. 2b). These excess mutations are enriched in synonymous mutations relative to the other patients, further supporting hypermutation (P < .001, Supplementary Fig. 2c). While hypermutation is a common phenotype in many pathogens, hypothesized to accelerate the evolution of antibiotic resistance27-30, it has not been previously described in members of the B. cepacia complex31.
For how long have these diverging lineages coexisted? The time to the last common ancestor (LCA) of each non-hypermutating patient's population32 can be estimated using the number of mutations accumulated since the LCA and the molecular clock previously measured for this outbreak (2.1 SNPs/year8). Given the phylogeny of isolates from Patient 1, we calculated the distribution of the number of mutations since the LCA, dLCA, across the population (Fig. 4a). The mean value of dLCA across isolates, <dLCA>, is 19.6 single nucleotide mutations per genome (95% confidence interval, CI = 18.3-20.8), suggesting that the LCA existed 9.3 years ago (CI = 8.7-9.9). This places the LCA of the isolates from this sample slightly earlier than the first B. dolosa culture from this patient (7.6 years before sample collection), suggesting that the B. dolosa population in Patient 1 has been diverging since, or perhaps before, initial colonization. While the population sequencing approach cannot provide a distribution of dLCA, due to a lack of information regarding linkage between mutations, we can still calculate <dLCA>: it is the sum of the polymorphic mutation frequencies (see Supplementary Note for derivation). Using this approach, the estimated time to LCA for Patient 1's population is 7.9 years. This value is slightly lower than calculated from the clonal re-sequencing approach, likely due to mutations left undetected by our conservative polymorphism caller (see Supplementary Note for discussion of error). For Patients 2 and 4, the time to LCA calculated by this population sequencing approach is several years less than the time since first positive culture, suggesting fixation events sometime during these patients' histories (Supplementary Table 1). For all these patients, we estimate that diverging lineages have coexisted in each of these patients for at least 5 years (Fig. 4b).
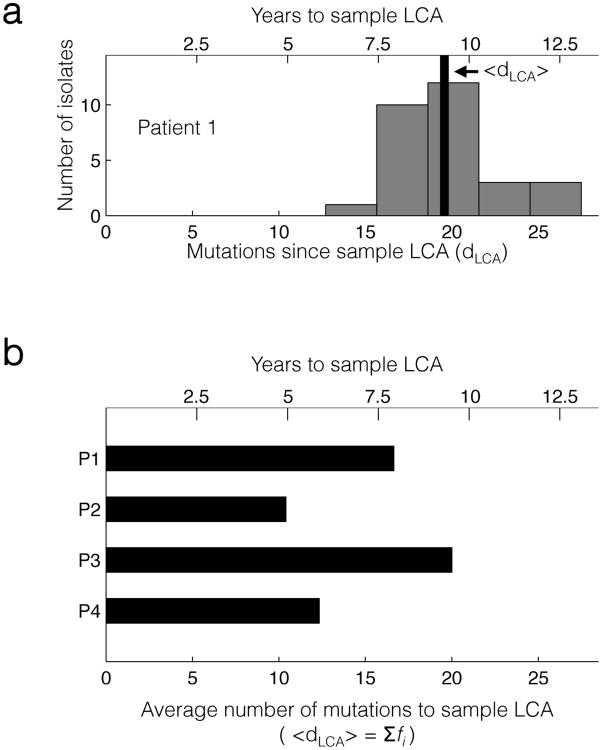
(a) A histogram of the number (dLCA) of single nucleotide mutations found in isolates from Patient 1, relative to their LCA. The black bar indicates the mean value of dLCA across the isolates. (b) The value of <dLCA> from the population sequencing data for patients Patients 1 through 4 (Online Methods). In both panels, the axis at top shows the relationship between dLCA and years to LCA, as calculated via the molecular clock (2.1 SNPs/yr)8.
To explore the drivers of this long-coexisting diversity, we examined the identity of the evolving genes. Interestingly, we found that within each sample, several B. dolosa genes carried 2-4 coexisting polymorphisms (Supplementary Table 2). This clustering is a significant departure from a neutral model given the number of mutations and the distribution of gene lengths (Fig. 5a, P < 0.005 for Patients 1-4; Online Methods). A similar analysis at the operon-level further identified several operons enriched for polymorphisms (Supplementary Table 3 and Supplementary Fig. 3). An enrichment of nonsynonymous mutations in these multi-diverse genes and operons suggests that they are drivers of adaptive change in vivo (dN/dS = 7.0, CI = 2.3-34.9, Fig. 5b). Polymorphisms are thus concentrated within genes undergoing adaptive evolution.
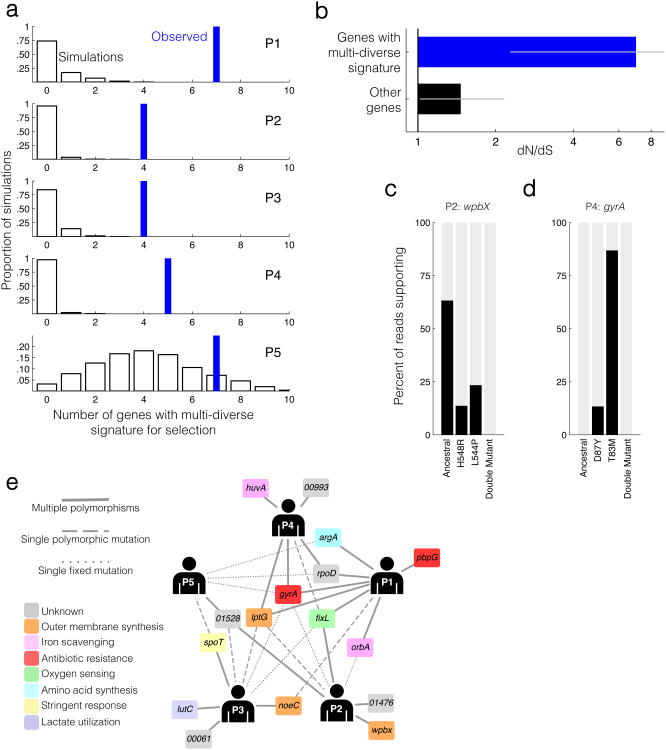
(a) Number of multi-diverse genes observed in samples from Patients 1-5 (P1-P5, blue bars) relative to a null expectation in which diverse sites are randomly distributed across the genome (histogram, 1000 simulations). For P5, the number of multi-diverse genes observed is not significant. (b) The canonical signal for selection, dN/dS, across the set of 16 genes and 3 operons showing a multi-diverse signature in at least one patient (P1-P4, 21 genes total, blue) versus dNdS across the set of genes not showing this signature (black). dN/dS >1 indicates positive selection for amino acid change. Error bars indicate 95% CIs. See Online Methods for details on the calculation of dNdS. (c-d) Linkage between nearby polymorphisms based on jointly overlapping short reads. Percentages of reads supporting the ancestral genotype, each of the single mutants, and the double mutant are plotted. No reads supporting the double mutant were found (c, n=524; d, n=415; See Supplementary Fig. 5 for exception). (e) A network of patients and genes showing a multi-diverse signature at least once in P1-P4. A gene is connected to a patient if it was mutated multiple times (solid line), had a single polymorphic mutation (dashed lined), or single fixed mutation (dotted line) within that patient. Genes closer to the center of the network are mutated in more patients, representing common targets of in vivo pathogen selection, while genes connected to single patients may indicate patient-specific adaptation. Genes are labeled with their closest homolog and predicted biological role. The biological role of rpoD is unclassified because it is recently duplicated in the B. cepacia complex39 (see Supplementary Note, Supplementary Table 2).
To understand why polymorphisms cluster within some genes, we asked if coexisting mutations in the same gene appeared in different lineages or were linked in a double mutant. Examining the single isolate genomes, we found no isolates with doubly mutated genes (Supplementary Fig. 4). Similarly, for the population sequencing, in 10 of 11 cases where polymorphic positions are close enough on the genome to be covered by the same short sequencing reads, we did not find reads that contain both variants (Fig. 5c, Supplementary Fig. 5). In some of these cases, the ancestral genotype is completely purged from the population (Fig. 5d). Thus, diversification is driven by multiple adaptive mutations in the same genes evolving in parallel within individual patients.
These findings provide a new signature of past selective pressures detectable in a single clinical sample; the coexistence of multiple polymorphisms within the same gene in a clinical sample. Sixteen B. dolosa genes display this multi-diverse signature, including genes with homologs involved in outer membrane synthesis, antibiotic resistance, iron scavenging, oxygen sensing, amino acid synthesis, lactate utilization, and stress response. Additionally, some genes with less characterized biological roles display a multi-diverse signature, including two transcriptional regulators with unknown targets in B. dolosa, an uncharacterized glucoamylase, and two genes that encode hypothetical proteins (Supplementary Table 2). A similar signature for selection is seen in three operons, two involved in lipopolysaccharide transport and one containing a two-component regulatory system with unknown targets (Supplementary Table 3). Selection on many of these elements can be rationalized based on the relevance of their annotated functions to conditions to which the bacteria are exposed in the course of the infection. Yet, further investigation will be required to understand the potential roles of some of these genes in antibiotic resistance, fitness, and other aspects of pathogenesis.
We found that many of the selective forces acting on the pathogen are the same across patients (Fig. 5e). Often, genes showing a multi-diverse signature for selection in one patient also carry mutations in other patients (P < 0.002, hypergeometric test). A prominent example is gyrA, a well-studied target of quinolones, which is mutated in all patient populations. Further support for commonality in mutational trajectories across patients emerges from a significant overlap between this list of 16 multi-diverse genes and 17 genes previously found to be under parallel evolution across a larger group of patients, only one of whom (Patient 2) was included in both studies (P < 0.001, hypergeometric test). Thus, the study of a single clinical sample can provide generalizable lists of selective pressures felt within the human body.
Yet, some multi-diverse signatures are patient-specific. A penicillin-binding protein (BDAG_01166, homologous to PBP7) has 3 nonsynonymous mutations in Patient 1, but is not mutated in other patients. Such patient-specific parallel evolution might reflect patient-specific selective pressure or perhaps a fitness benefit dependent upon previously acquired mutations. But these hypotheses are hard to test because the genomic target for a selective force might include more than one gene. For example, four of the five patients' populations have a mutation in a homolog of the histidine kinase fixL (BDAG_01161, known to be under strong selection in these infections8) while the fifth has a mutation in the corresponding response regulator.
To investigate the stability of these multi-diverse signatures for selection, we collected a second sputum sample 14 days after initial sample collection from Patient 2. Three of the four genes with the multi-diverse signature at day 0 show the same pattern at day 14. The absence of the signature in the fourth gene at the later time point does not reflect a relaxation in selection for mutant alleles, but rather incomplete detection of genes under selection; this gene also has abundant nonsynonymous mutants at day 14, concentrated at a single nucleotide position (Supplementary Fig. 6). These results suggest that the multi-diverse signature for selection is relatively stable and that multiple sample collections per patient can increase the sensitivity of the detection.
Our results reject the dominant lineage model of infection, yet demonstrate that these diversifying bacteria adapt under the pressure of natural selection. These observations are consistent with clonal interference: in large asexual populations, multiple beneficial mutations emerge and compete, impeding the ability of these lineages to reach fixation33-35. In addition to large population size (108 cells/mL sputum), the branched structure of the airways may further hinder the capacity of any adaptive lineage to dominate and fix, and the immune system or niche-specific adaptations might directly promote diversity. Diversified by any of these means, lineages may then continue to evolve in parallel against common selective forces.
As B. dolosa adapts to the airways of people with cystic fibrosis, mutations lead to diversification rather than fixation and replacement. Though it is possible that adaptive mutations will lead to fixation more frequently in other infections, there is evidence that, at least in long-term colonization, diversity might be common14,36-38. This long-term coexistence of diverse lineages records the genomic history of selection on the pathogen within its host. The ability to rapidly read off within-patient evolutionary history from the genotypic diversity within a single clinical sample may greatly accelerate the ability to survey selective pressures acting on bacterial pathogens in vivo – shifting from an epidemic level investigation to a single-patient paradigm.
Online Methods
Study cohort and sample collection
An epidemic clone of B. dolosa infected and colonized 39 individuals with cystic fibrosis in the Boston area over a 20-year period21. We studied B. dolosa intrapatient diversity in 5 surviving individuals still infected with B. dolosa. All subjects were male, had homozygous ΔF508 mutations, had not received lung transplants, were between 21 and 35 years of age, and had been colonized for between 7 and 10 years at the time of sample collection (see Supplementary Table 1). Longitudinal microbial isolates from Patient 2 were also included in a previous retrospective study (patient J in reference 8).
For Patient 1, both the colony re-sequencing and deep population sequencing approaches were performed on a single sputum sample (P1). For Patient 2, population deep sequencing was performed on each of two sputum samples (P2 and P2T), collected 14 days apart. Between collections, Patient 2 was treated for a pulmonary exacerbation, including a change in antibiotic regimen, but his condition did not improve and B. dolosa density did not decrease. For Patients 3-5, population sequencing was performed on a single sputum sample from each patient (P3-P5).
Expectorated sputum samples were collected at Boston Children's Hospital after written informed consent was obtained under protocols approved by the Institutional Review Boards at Boston Children's Hospital and Harvard Medical School. Samples were liquefied with dithiothreitol40 and stored at −80°C in 20% glycerol. B. dolosa was cultured from frozen samples. For population sequencing, a plate with 5,000 to 30,000 small colonies was chosen from a serial dilution. See Supplementary Note for more details on sample preparation.
Illumina sequencing
Genomic DNA was extracted using MoBio UltraClean Microbial DNA Isolation Kit per the manufacturer's instructions. Genomic libraries were constructed and barcoded using the Illumina-compatible Epicentre Nextera DNA Sample Prep Kit and following manufacturer's instructions (PCR amplification in the Nextera preparation does not introduce false positive polymorphisms, see Supplementary Note). Genomic libraries were sequenced on the Illumina HiSeq 2000 by Partners HealthCare Center for Personalized Genetic Medicine. Individual colonies were sequenced using single-end, 50 base-pair (bp) reads and pooled samples were sequenced using paired-end, 50bp reads. Reads were aligned to the B. dolosa draft genome AU0158 (GenBank accession number AAKY00000000, see URLs), belonging to an isolate recovered from patient zero of the outbreak. AU0158 consists of 233 contigs on 3 scaffolds (B. dolosa has 3 chromosomes). Standard approaches were used for read filtering and alignment (Supplementary Note). See Supplementary Table 4 for coverage statistics.
Mutation identification, colony re-sequencing
An outgroup of 3 outbreak strains (A-0-0, G-9-8, and N-12-6d-$, previously sequenced8) was included in the analysis to identify mutations fixed among the 29 isolates from P1. We considered genomic positions at which at least one pair of isolates was discordant on the called base and both members of the pair had FQ scores less than −40 (FQ scores are produced by SAMtools41; lower values indicate agreement amongst reads). Genomic positions for which multiple isolates had multiple calls per isolate were discarded (likely duplication not represented in the reference). A best call was forced for each isolate (Supplementary Table 5) and the list of concatenated SNPs was inputted into the dnapars program in PHYLIP v3.6942. The resulting phylogeny was visualized the tree using Figtree (Fig. 3b).
Mutation identification, deep population sequencing
Fixed mutations within each patient's population were called using the same procedure as individual isolates, with a stricter quality score threshold (FQ < -282). Custom MATLAB scripts and SAMtools-produced pileup files were used to summarize all calls and their related quality scores at each genomic position (e.g. base quality, mapping quality, tail distance; see Supplementary Note). Using the isogenic control, multiple isolates from Patient 1, and an interactive MATLAB environment that enabled investigation of the raw data, we developed a set of filters to identify true-positive polymorphic positions with minor allele frequency above 3% (Supplementary Table 6). Thresholds were chosen to minimize false positives. See Supplementary Note and Supplementary Figs. 7-8 for description of filters and sensitivity analysis.
Estimation of <dLCA>
For the colony-based approach, dLCA was calculated for each isolate as the number of mutations received by that isolate normalized by the size of the callable genome. For this approach, the callable genome is the set of genomic positions with FQ score < -40. The confidence interval for <dLCA> presented for this approach is calculated according to a Poisson distribution. For the pool-based approach, <dLCA> was calculated as the sum of the mutation frequencies at each polymorphic position called within that population, normalized by the size of the callable genome (see Supplementary Note). For the pool-based approach, we define the callable genome as the set of positions that met the chosen thresholds for coverage, average base quality, average mapping quality, and average tail distance for each strand, irrespective of nucleotide call. See Supplementary Fig. 6b and the Supplementary Note for a discussion of sources of error in estimating <dLCA> and time to LCA.
Detection of parallel evolution within patients
We define genes with a multi-diverse signature of selection as genes for which within the same sputum sample there were multiple polymorphisms and multiple polymorphisms per 2000bp (to account for the fact that long genes are more likely to be mutated multiple times by chance). To determine whether the number of genes showing this signature was a significant departure from what expected in a neutral model, we performed for each sputum sample 1000 simulations in which we randomly shuffle the polymorphisms found across the callable genome, and calculate how many genes show the signature of selection (Fig. 5a).
This analysis was repeated at the operon and pathway levels, using the free version FgenesB to identify operons and subsystem annotations provided by SEED43 as pathways (see Supplementary Figure 3). As in the gene analysis, we considered operons and pathways to have a signature for selection if they had both multiple polymorphisms and multiple polymorphisms per 2000 nucleotides with the same patient.
dN/dS
Mutations were classified as nonsynonymous (N) or synonymous (S) according to annotations provided in genbank file. For open reading frames in draft genome without a provided frame, we used BLAST and RefSeq to identify the most likely reading frame in the neighborhood of the found mutations. For each dNdS calculation, we used the particular spectrum of mutations observed to calculate the expected N/S (e.g. A->C mutations are 10.6 times more likely to cause an N than G->A mutations). The observed value of N/S was divided by this expectation to get dN/dS. Confidence intervals and p-values were calculated according to binomial sampling. The dNdS reported Fig. 5b groups together the mutations found in genes and operons under selection; the same calculation for only genes gives a dN/dS of 5.9 (95% CI = 1.9-29.6).
Parallel evolution across patients
We used the hypergeometric distribution to assess the significance of overlap between gene sets. Of 225 B. dolosa genes mutated in P1-P4, only 16 showed the multi-diverse signature for selection within patients and only 29 genes were mutated in multiple of these patients (fixed or polymorphic), yet 7 genes are in common between these lists (P=.0015) Similarly, 13 of these 225 genes were also found on a list of 17 genes evolved in parallel across patients in a previous study8. These 13 genes were enriched in the 16 genes under selection in in this study (5 gene overlap, P=.0009). When this analysis was repeated without mutations from P2 (Patient 2 also included in retrospective study), 11 of the 189 mutated genes were found in the previous study and 13 genes show a multi-diverse signature for selection. The overlap between these lists of 11 and 13 genes is smaller but still significant (4 genes; P=.0035).
Supplementary Material
1
2
3
Acknowledgments
We are grateful to Jean-Baptiste Michel and members of the Kishony lab for insightful discussions and support, to the team at Partners HealthCare Center for Personalized Genetic Medicine (PCPGM) for Illumina sequencing, to Laura Williams and Adam Palmer for discussions and technical assistance, and to Ylaine Gerardin, Justin Meyer, Laura Stone, and Rebecca Ward for their comments on the manuscript. T.D.L. and G.P.P. were supported in part by grants from the Cystic Fibrosis Foundation (LIEBER12H0 to T.D.L and PRIEBE1310 to G.P.P). This work was funded in part by the US National of Institutes of Health (GM081617 to R.K.), the New England Regional Center of Excellence for Biodefense and Emerging Infectious Diseases (NERCE; AI057159 to R.K.) and Hoffman-LaRoche.
Footnotes
Accession numbers: SRP030656
Author Contributions: TDL, AJM, GPP and RK designed the study. AJM and TRM collected clinical samples. KBF, TRM, AJM and GPP conducted chart review and provided medical information. TDL performed experiments. TDL, IY and RK wrote the sequence analysis scripts. TDL and RK analyzed the data. TDL, AJM, GPP and RK interpreted the results and wrote the paper.
URLs: Burkholderia dolosa sequencing project, http://www.broadinstitute.org/annotation/genome/burkholderia_dolosa
References
Full text links
Read article at publisher's site: https://doi.org/10.1038/ng.2848
Read article for free, from open access legal sources, via Unpaywall:
https://www.ncbi.nlm.nih.gov/pmc/articles/PMC3979468
Citations & impact
Impact metrics
Citations of article over time
Alternative metrics
Smart citations by scite.ai
Explore citation contexts and check if this article has been
supported or disputed.
https://scite.ai/reports/10.1038/ng.2848
Article citations
Drivers of diversification in fungal pathogen populations.
PLoS Pathog, 20(9):e1012430, 12 Sep 2024
Cited by: 0 articles | PMID: 39264909 | PMCID: PMC11392411
Review Free full text in Europe PMC
Genomic evidence of <i>Escherichia coli</i> gut population diversity translocation in leukemia patients.
mSphere, 9(10):e0053024, 04 Oct 2024
Cited by: 0 articles | PMID: 39365076 | PMCID: PMC11520291
Evolution of evolvability in rapidly adapting populations.
Nat Ecol Evol, 8(11):2085-2096, 11 Sep 2024
Cited by: 2 articles | PMID: 39261599
Fast and accurate variant identification tool for sequencing-based studies.
BMC Biol, 22(1):90, 22 Apr 2024
Cited by: 1 article | PMID: 38644496 | PMCID: PMC11034086
Native microbiome dominates over host factors in shaping the probiotic genetic evolution in the gut.
NPJ Biofilms Microbiomes, 9(1):80, 14 Oct 2023
Cited by: 0 articles | PMID: 37838684 | PMCID: PMC10576824
Go to all (165) article citations
Data
Data behind the article
This data has been text mined from the article, or deposited into data resources.
BioStudies: supplemental material and supporting data
Nucleotide Sequences
- (1 citation) ENA - SRP030656
Similar Articles
To arrive at the top five similar articles we use a word-weighted algorithm to compare words from the Title and Abstract of each citation.
Genetic signature of bacterial pathogen adaptation during chronic pulmonary infections.
Nat Genet, 46(1):5-6, 01 Jan 2014
Cited by: 3 articles | PMID: 24370741
Parallel bacterial evolution within multiple patients identifies candidate pathogenicity genes.
Nat Genet, 43(12):1275-1280, 13 Nov 2011
Cited by: 263 articles | PMID: 22081229 | PMCID: PMC3245322
Long-term colonization of the cystic fibrosis lung by Burkholderia cepacia complex bacteria: epidemiology, clonal variation, and genome-wide expression alterations.
Front Cell Infect Microbiol, 1:12, 02 Dec 2011
Cited by: 42 articles | PMID: 22919578 | PMCID: PMC3417363
Insights into the role of extracellular polysaccharides in Burkholderia adaptation to different environments.
Front Cell Infect Microbiol, 1:16, 15 Dec 2011
Cited by: 31 articles | PMID: 22919582 | PMCID: PMC3417362
Review Free full text in Europe PMC
Funding
Funders who supported this work.
NCATS NIH HHS (1)
Grant ID: UL1 TR000170
NIAID NIH HHS (1)
Grant ID: U54 AI057159
NIGMS NIH HHS (2)
Grant ID: GM081617
Grant ID: R01 GM081617