Abstract
Free full text

Genome Properties and Prospects of Genomic Prediction of Hybrid Performance in a Breeding Program of Maize
Associated Data
Abstract
Maize (Zea mays L.) serves as model plant for heterosis research and is the crop where hybrid breeding was pioneered. We analyzed genomic and phenotypic data of 1254 hybrids of a typical maize hybrid breeding program based on the important Dent × Flint heterotic pattern. Our main objectives were to investigate genome properties of the parental lines (e.g., allele frequencies, linkage disequilibrium, and phases) and examine the prospects of genomic prediction of hybrid performance. We found high consistency of linkage phases and large differences in allele frequencies between the Dent and Flint heterotic groups in pericentromeric regions. These results can be explained by the Hill–Robertson effect and support the hypothesis of differential fixation of alleles due to pseudo-overdominance in these regions. In pericentromeric regions we also found indications for consistent marker–QTL linkage between heterotic groups. With prediction methods GBLUP and BayesB, the cross-validation prediction accuracy ranged from 0.75 to 0.92 for grain yield and from 0.59 to 0.95 for grain moisture. The prediction accuracy of untested hybrids was highest, if both parents were parents of other hybrids in the training set, and lowest, if none of them were involved in any training set hybrid. Optimizing the composition of the training set in terms of number of lines and hybrids per line could further increase prediction accuracy. We conclude that genomic prediction facilitates a paradigm shift in hybrid breeding by focusing on the performance of experimental hybrids rather than the performance of parental lines in testcrosses.
HYBRID breeding was pioneered in maize (Shull 1908) and plays an ever increasing role in other globally important field (Duvick 1999) and vegetable crops (Silva Dias 2010). Maize has also served as a model species for research in heterosis, the phenomenon behind the success of hybrid varieties, for which the genetic mechanisms have been elusive (Duvick 1999; Lippman and Zamir 2006). In recent years, evidence emerged for the importance of (pseudo-)overdominance in the manifestation of heterosis in maize (Lippman and Zamir 2006; Schön et al. 2010) and the particular role of the centromeres in this process (Gore et al. 2009; McMullen et al. 2009). Today, the availability of high-density marker data and whole-genome regression methods developed in the context of genomic prediction (Meuwissen et al. 2001) allows us to revisit this hypothesis by studying key genome properties such as allele frequencies and linkage phases.
Consistency of linkage phases between quantitative trait loci (QTL) and markers is a key prerequisite for pooling of diverse breeds and germplams to increase sample size for genetic studies and transferability of their results to different populations (De Roos et al. 2008). Weber et al. (2012) used whole-genome estimates of marker effects of several cattle breeds to investigate across-breed marker–QTL linkage phase consistency. Such a study is still missing for maize and other important crops. For optimum exploitation of heterosis, the parental inbred lines of maize hybrids are taken from genetically distant pools of germplasm, called heterotic groups (Melchinger and Gumber 1998). Comparing the profiles of marker effects of both heterotic groups would be of great interest for better understanding the genetic basis of heterosis and choice of models for genomic prediction (Technow et al. 2012).
With the advent of doubled-haploid technology in many species, fully homozygous inbred lines can be generated rapidly, at low cost, and in great numbers (Wedzony et al. 2009). This leads to a vast expansion of the number of potential hybrids. For example, with only 1000 lines generated in each heterotic group every year, the number of potential hybrids reaches 1 million. Because producing and testing a substantial fraction of these in field trials is impossible, prediction of hybrid performance is of tremendous importance for hybrid breeding (Bernardo 1996).
Genomic prediction (Meuwissen et al. 2001), originally devised for prediction of breeding values, involves a “training set” of individuals that have been both genotyped and phenotyped and a “candidate set” of untested individuals, for which only genotypic information is available (Jannink et al. 2010). The genotypic values of the candidates are then predicted either from their genomic relationship to the training set individuals or from marker effects estimated in the training set. Genomic prediction of hybrid performance came into focus recently, with studies exploring its prospects in maize (Maenhout et al. 2010; Massman et al. 2013), sunflower (Reif et al. 2013), and wheat (Zhao et al. 2013). However, the low number of markers or the low number of parental lines and phenotyped hybrids used in these studies allowed only preliminary inferences about the prospects of genomic prediction in commercial hybrid breeding programs of ordinary size.
Optimal composition of training sets is crucial for successful application of genomic prediction (Rincent et al. 2012; Windhausen et al. 2012). For hybrid prediction, a critical question is how many hybrids per inbred line, i.e., crosses with lines from the opposite heterotic group, should be included in the training set. With a given budget for phenotyping of training set hybrids, the number of hybrids per line limits the total number of inbred lines that can be tested. The number of hybrids per line and the total number of lines and hybrids in the training set can affect the prediction accuracy. These important factors were not investigated in previous studies.
Technow et al. (2012) showed in a simulation study that the Bayesian whole-genome regression method BayesB (Meuwissen et al. 2001) is a powerful alternative to genomic best linear unbiased prediction (GBLUP), first used by Maenhout et al. (2010) for genomic prediction of hybrid performance. Zhao et al. (2013) later compared both methods, using a wheat data set of very limited size. Thus, conclusive results on the comparative performance of GBLUP and BayesB in real data sets are still missing.
Our objectives were to (i) investigate differences among chromosomal regions in linkage disequilibrium and linkage phases, allele frequencies, and marker effects of the parental heterotic groups; (ii) examine the prospects of genomic prediction of hybrid performance for an important heterotic pattern in maize; (iii) investigate the effects of the size of the training set and of its composition in terms of the number of lines and the number of hybrids per line on prediction accuracy; and (iv) compare the prediction accuracy achieved by prediction methods GBLUP and BayesB. We therefore analyzed high-density genomic and phenotypic data of 1254 hybrids, collected over the last decade in a typical maize hybrid breeding program based on the Dent × Flint heterotic pattern.
Materials and Methods
Phenotypic data
Our phenotypic database comprised grain yield (GY) (in quintals per hectare) and grain moisture content (GM) (in percent) of 1254 maize single-cross hybrids generated and tested over the last decade within the breeding program of the University of Hohenheim. The hybrids represent an incomplete factorial between 123 Dent and 86 Flint inbred lines, with each Dent line involved in 10 (range 2–56) and each Flint line in 15 (range 1–102) hybrid combinations, on average. A schematic view of the factorial is shown in Supporting Information, Figure S1.
The data were collected in 14 years (1999–2012) and across 20 locations in Southern Germany, providing 131 environments. The field design used at each location was an α-lattice with two to three replications and incomplete block sizes of five. In total, data of 24,925 field plots were available.
On average, 95 hybrids, produced from 15 Dent and 11 Flint lines, were tested each year. The number of years in which a hybrid was tested ranged from 1 to 9, with an average of 1.2. Of all hybrids, 182 were tested in multiple years. The average number of years a line served as parent of one or several hybrids was 1.6 (range 1–9) for Dent lines and 1.8 for Flint lines (range 1–10).
Analysis of genomic data
All parental inbred lines were genotyped with the Illumina MaizeSNP50 BeadChip (Ganal et al. 2011). We removed all markers missing or heterozygous in >5% of the inbred lines. Remaining missing (0.2%) or heterozygous (0.3%) marker genotypes were replaced with the most frequent allele. A total of 35,478 markers were subsequently available for further analysis. The marker data are provided in File S1, File S2, and File S3.
Overall pairwise linkage disequilibrium (LD) between markers on the same chromosome was computed as r2, separately for the Dent and Flint group, using only markers with a minor allele frequency (MAF) ≥ 0.025 in the respective group (24,242 markers for the Dent lines and 23,450 for the Flint lines). To diminish the confounding effect of varying marker density on regional LD patterns along the chromosomes, we reduced the marker density to ~4 markers per megabase (Mb), with a spacing of ~0.25 Mb, resulting in 4958 markers available for analysis within the Dent and 4929 within the Flint heterotic group. Nevertheless, some density differences could not be completely eliminated. This was because in some instances, no segregating markers could be found in the desired intervals. We then divided all chromosomes into bins of 5Mb width and computed the average pairwise LD, measured as r2, between all markers in the bin. For each bin, we also determined the proportion of marker pairs with the same linkage phase, i.e., same sign of the r statistic in Dent and Flint (Technow et al. 2012), and the correlation between the r values of both groups. For this, 4397 markers with a MAF ≥ 0.025 in each group were used.
MAF patterns along the chromosomes were investigated using a similar approach. Again we used consecutive bins of 5Mb width and computed the average MAF for each bin in the sets of Dent and Flint lines as well as the average absolute difference between the reference allele frequencies in the two groups. These investigations were carried out using all 35,478 markers. The allele that had highest frequency across the combined set of Dent and Flint lines was defined as the reference allele.
Variance components and adjusted means
We used a two-stage analysis for estimation of variance components and adjusted entry means that closely followed Bernardo (1996) and Massman et al. (2013). Two-stage analysis is commonly used for analyzing plant breeding field trials and delivers in most cases results similar to those of considerably more complex one-stage approaches (Möhring and Piepho 2009). Its main advantage is the strongly reduced computational burden when numbers of genotypes and environments are large.
In the first stage, hybrid × environment means y were calculated with a standard α-lattice design analysis to adjust for the effects of the field design in these environments. In the second stage, we fitted the model
where vector y contained the phenotypic observations of the hybrids in the 131 environments obtained in stage one, β was the vector of fixed effects of environments, and X was the corresponding design matrix.
The design matrices ZD and ZF associated the random general combining ability (GCA) effects of the parental Dent lines (gD) and Flint lines (gF), respectively, to the observations of the hybrids in y. ZS was the design matrix of the random specific combining ability (SCA) effects (s) for specific Dent × Flint hybrid combinations in y. The residuals were represented by vector e. The covariance matrix of gD was
The genomic relationship matrix GD was computed according to VanRaden (2008) as
Let D and D* denote any two Dent lines and F and F* any two Flint lines. For a given pair of single crosses (D × F) and (D* × F*), the element of S was the product
The variance components were estimated for the whole data set, using the EM algorithm for restricted maximum likelihood described by Henderson (1985) and adapted for variance component estimation in factorials by Bernardo (1996). The entry-mean heritability was computed as
GBLUP
The performance of untested hybrids was predicted by GBLUP with the formula
BayesB
Our BayesB-type model for the performance of the ith hybrid corresponded to model S2 of Technow et al. (2012):
Here, the linear predictor of the performance of the ith hybrid is denoted as μi and β0 is a common intercept. The row vectors
The elements of the matrices MD and MF code the presence or absence of the reference allele in the gametes produced by the parental Dent and Flint lines as 1/2 and −1/2, respectively. In contrast to MD and MF, which code the genotypes of parental gametes, matrix D directly reflects the genotypes of the single-cross hybrids, coding heterozygous genotypes as 1 and homozygous genotypes as 0. For example, if the allele contributed by the Dent parent was “C” and that by the Flint parent “T”, and T had the higher allele frequency, then the corresponding elements of MD, MF, and D were −1/2, 1/2, and, 1, respectively.
Additive effects were estimated only for markers with a MAF ≥ 0.025 within the set of tested inbred line parents of the respective heterotic group and dominance effects only for markers with a MAF ≥ 0.025 in at least one of the groups. We reduced the marker density to ~10 markers per megabase to facilitate computations. Using higher marker densities did not improve prediction accuracies, as far as we could see. In total, additive effects were estimated for mD = 7500 markers of the Dent parental lines and for mF = 6500 markers of Flint parental lines, on average. The average number of markers for which dominance effects were estimated was mDF = 8900.
Prior specifications as well as the Gibbs-sampling strategy were identical to those in Technow et al. (2012). The same uninformative prior distribution, a Gamma distribution with α = β = 0.1, was used for the scale parameter S2. However, the hyperparameters ν and π were set to constant values, as in the original BayesB implementation of Meuwissen et al. (2001). Parameter ν was set to 4.001 for all types of marker effects, and π was chosen such that the number of markers fitted was 500, on average; e.g., for dominance effects (1 − πDF)mDF = 500.
Three independent Gibbs-sampling chains were run for 75,000 iterations, of which the first 74,000 iterations were discarded as burn-in. Using a higher number of iterations and chains did not improve prediction accuracy. The posterior means of marker effects were used to predict the performance of untested hybrids according to model (2).
For investigating the genetic architecture, namely the distribution and properties of marker effects, we fitted model (2), using all 1254 hybrids. The marker density was further reduced to ~1 marker per megabase, or 1617 markers used in total. This was done mainly to counter potential problems with likelihood identifiability that can occur when the number of effects is much larger than the sample size (Gianola 2013). All markers used segregated in each set of parental inbred lines with MAF ≥ 0.025. Thus, all three types of marker effects (additive effects for Dent and Flint and dominance effects) were estimated for each marker. For each trait, we ran 24 independent Gibbs-sampling chains for 1,000,000 iterations. We discarded the first 990,000 iterations as burn-in and afterward stored only samples from every 10th iteration. The posterior means of the marker effects were used as their point estimates.
Evaluation of prediction accuracy
The cross-validation procedure for estimating prediction accuracy was stratified by the parental lines (Figure 1). Let D = {1, 2,…
,
123} and F = {1, 2,
…
,
86} denote the entire set of Dent and Flint lines, respectively, and let the entire set of available hybrids be denoted by Π = {(i, j) | i
D, j
F, with hybrid combination i × j among the 1254 single-crosses evaluated}. As a first step, we sampled a subset DT of ND Dent lines from D and a subset FT of NF Flint lines from F. Then we sampled a random subset ΠT of NH training set hybrids from all hybrids for which both the Dent and Flint parents were elements of DT and FT, respectively. The constraint here was that for all i
DT and j
FT,
ΠT for the ith Dent line, and likewise
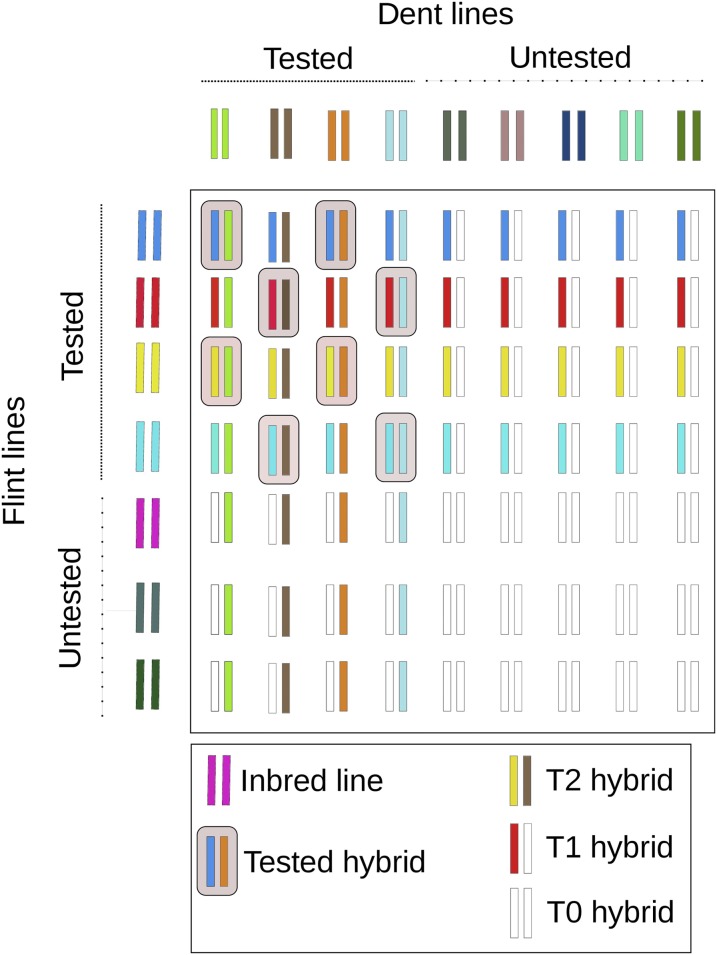
Schematic visualization of the strategy for distinguishing the tested hybrids in the training set and T2, T1, and T0 hybrids in the validation set.
For investigating the influence of NH, we varied NH between 150 and 450 in steps of 50 but kept ND constant at 90 and NF at 53. The latter restriction guaranteed that both the required number of training set hybrids and sufficiently sized candidate groups were available for all values of NH. The number of T2 hybrids necessarily decreased with increasing NH; for NH = 450, its average was still 119. The numbers of T1 and T0 hybrids were on average 557 and 128, respectively. With increasing NH, the average number of hybrids per Dent line
For investigating the influence of the number of parental lines used in the training set, we set ND to 70 and 110, respectively, and NF to 33 and 73, respectively, while keeping NH constant at 200. Here, the value NH = 200 ensured that the groups of T2, T1, and T0 hybrids had a sample size of at least 20 hybrids each for all values of ND and NF. When ND = 70 and NF = 33, the average numbers of hybrids per line were
Table 3
Method | GY | GM | ||||||
---|---|---|---|---|---|---|---|---|
T2 | T1 | T0 | T2 | T1 | T0 | |||
GBLUP | 3.05 (70) | 6.18 (33) | 0.90 (0.05) | 0.83 (0.02) | 0.75 (0.06) | 0.93 (0.03) | 0.77 (0.03) | 0.61 (0.07) |
1.82 (110) | 2.75 (73) | 0.88 (0.02) | 0.83 (0.05) | 0.75 (0.17) | 0.89 (0.02) | 0.79 (0.05) | 0.64 (0.21) | |
BayesB | 3.05 (70) | 6.18 (33) | 0.90 (0.05) | 0.83 (0.02) | 0.75 (0.06) | 0.92 (0.03) | 0.77 (0.03) | 0.59 (0.09) |
1.82 (110) | 2.75 (73) | 0.88 (0.02) | 0.83 (0.04) | 0.75 (0.18) | 0.87 (0.02) | 0.78 (0.05) | 0.63 (0.23) |
The size of the training set was held constant at NH = 200. The values refer to the mean (standard deviation) over 10,000 and 100 cross-validation runs with the prediction methods GBLUP and BayesB, respectively, for grain yield (GY) and grain moisture (GM). For T2, T1, and T0 group hybrids, two, one, and zero parents, respectively, were tested in other combinations in the training set.
The prediction accuracy rA was computed separately for each group of hybrids by dividing the correlation of predicted and observed values (“predictive ability”) by
All analyses were carried out in the R statistical software environment (R Development Core Team 2012).
Results
Analysis of genomic data
From all 35,478 markers analyzed, 18.0% were monomorphic in the set of Dent lines, 20.5% in the set of Flint lines, and 8.5% in both. Excluding monomorphic markers, the median MAF in the Dent pool was 0.19 and that in the Flint pool was 0.12. Marker densities were lowest in pericentromeric regions, where particularly low MAFs were found (Figure 2, A and B). The largest absolute differences between the allele frequencies in the Dent and Flint heterotic groups were also found in pericentromeric regions (Figure 2C), indicating different fixation of alleles in these regions between the two groups.
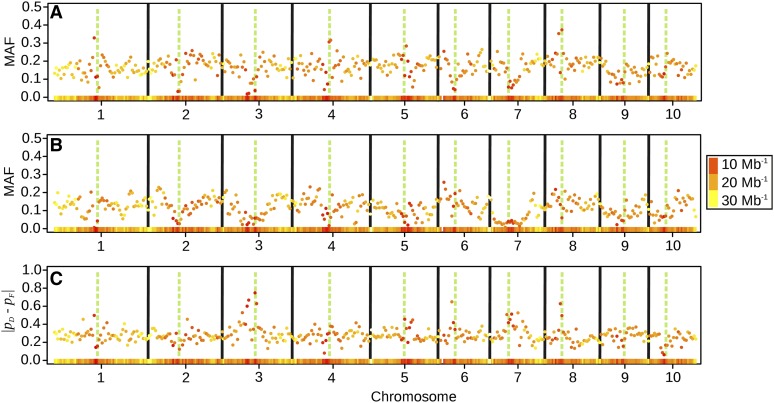
(A and B) Average minor allele frequency (MAF) of SNP within consecutive bins of 5-Mb width along the chromosomes, for Dent lines (A) and Flint lines (B). (C) Average absolute difference of reference allele frequency between Dent and Flint lines in the same 5-Mb bins. The different colors of the points and the heat map in the bottom of each subplot indicate the marker density within the bin (Mb−1). The green, dashed vertical bars indicate the physical positions of the centromeres, and the solid, black bars separate the chromosomes.
The LD in relation to physical distance reached very high median values ~0.33 for markers in close proximity (<0.125 Mb), with considerable proportions of the marker pairs exhibiting r2 values >0.8 (Figure 3). It then decayed to median r2 values ~0.10 for marker pairs with distances of ~3 Mb. The decrease in LD then continued, however, less pronounced, such that even at distances of 15 Mb, the median r2 was still ~0.05 (data not shown).
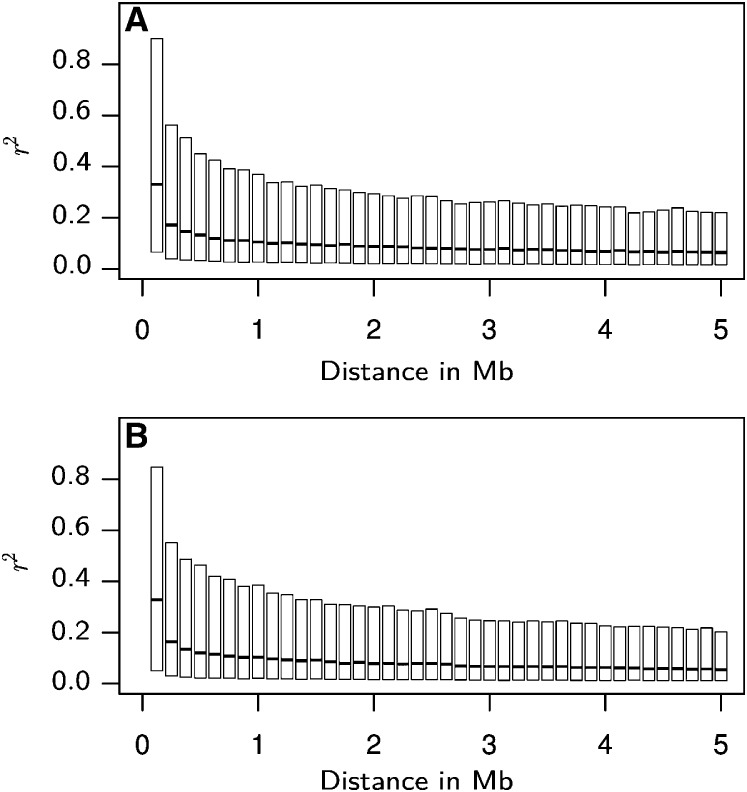
(A and B) Boxplots of pairwise LD, measured as r2, between markers on the same chromosomes, with distances in megabases (Mb), for the set of Dent (A) and Flint (B) lines. Marker pairs were binned according to physical distance, each bin corresponding to an interval of 0.125 Mb.
Pericentromeric regions displayed considerably elevated levels of regional LD (Figure 4, A and B). In many cases, the average pairwise r2 values in pericentromeric regions were more than four times higher than those in distal chromosome regions. Also the proportion of markers with the same sign of the r linkage statistic was higher in pericentromeric regions (Figure 4C). Here, the proportion could reach 100%, whereas in distal regions of the chromosomes it was ~50% (the value indicating independence of Dent and Flint linkage phases). Similar trends were observed for the regional correlation of r between groups, which was generally positive and high in pericentromeric regions but around zero outside of these (Figure S2).
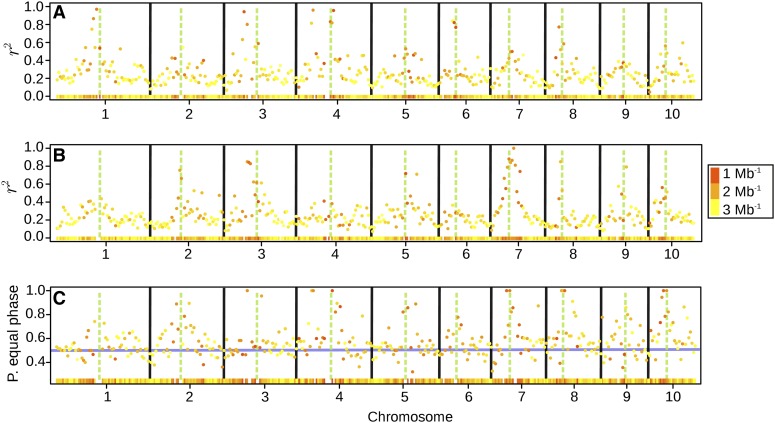
(A and B) Average pairwise LD (measured as r2) within consecutive bins of 5-Mb width along the chromosomes, for Dent lines (A) and Flint lines (B). (C) Proportion of marker pairs with equal linkage phase (equal sign of r statistic) between Dent and Flint lines in the same 5-Mb bins. The different colors of the points and the heat map in the bottom of each subplot indicate the marker density within the bin (Mb−1). The green, dashed vertical bars indicate the physical positions of the centromeres, and the solid, black bars separate the chromosomes. The horizontal line in C indicates the value of 0.50.
Estimated marker effects:
The number of markers with sizeable estimated additive effects was much larger than the number of markers with sizeable dominance effects (Figure S3 and Figure S4). Additive and dominance marker effect estimates were in equal proportions negative and positive. We did not observe a strong accumulation of large additive or dominance marker effects in any particular genomic region or chromosome.
The additive marker effects estimated for Dent (uD) were overall not consistent with those for Flint (uF). The rank correlation between additive marker effects for Dent uD and Flint uF was close to zero for both traits, but when restricted to markers within 12.5 Mb of the centromeres, the correlation was 0.385 (P = 0.195 × 10−5) and 0.200 (P = 0.015) for GY and GM, respectively (Figure 5).
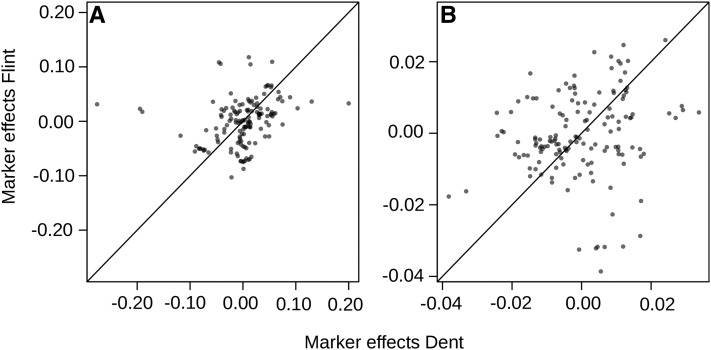
Scatterplot of posterior means of additive effects of markers located within 12.5 Mb of the centromeres in the Dent and Flint lines, estimated simultaneously with BayesB, using a subset of 1617 markers in total and all 1254 hybrids. Marker effects shown are for grain yield (A) and grain moisture content (B).
For GY, markers with strong additive effects for both Dent and Flint were encountered in the first quarter of chromosome 1 and in the last quarters of chromosomes 4 and 7 (Figure S3). The squared correlation between the predicted genotypic values and adjusted entry means was 0.85 and 0.94 within the training set for GY and GM, respectively.
Variance components and heritabilities:
For both traits, estimates of
Table 1
H2 | ||||||
---|---|---|---|---|---|---|
GY (q ha−1) | 32.79 | 28.12 | 8.44 | 179.00 | 12.17 | 0.87 |
GM (%) | 2.58 | 2.59 | 0.40 | 3.70 | 7.15 | 0.96 |
q ha−1, quintals per hectare.
Prediction accuracies:
Prediction methods GBLUP and BayesB resulted in very similar prediction accuracies (Table 2 and Table 3). Our presentation of prediction accuracy results therefore applies to both methods, if not mentioned otherwise.
Table 2
Method | NH | GY | GM | ||||||
---|---|---|---|---|---|---|---|---|---|
T2 | T1 | T0 | T2 | T1 | T0 | ||||
GBLUP | 150 | 1.69 | 2.85 | 0.87 (0.03) | 0.82 (0.03) | 0.75 (0.08) | 0.88 (0.02) | 0.77 (0.04) | 0.63 (0.10) |
200 | 2.25 | 3.80 | 0.89 (0.03) | 0.84 (0.03) | 0.76 (0.08) | 0.91 (0.02) | 0.79 (0.03) | 0.64 (0.10) | |
250 | 2.81 | 4.75 | 0.90 (0.03) | 0.85 (0.03) | 0.77 (0.08) | 0.92 (0.02) | 0.80 (0.03) | 0.64 (0.10) | |
300 | 3.37 | 5.71 | 0.91 (0.03) | 0.85 (0.02) | 0.78 (0.08) | 0.93 (0.02) | 0.80 (0.03) | 0.65 (0.10) | |
350 | 3.93 | 6.65 | 0.92 (0.03) | 0.86 (0.02) | 0.78 (0.07) | 0.94 (0.02) | 0.81 (0.03) | 0.65 (0.09) | |
400 | 4.49 | 7.61 | 0.92 (0.03) | 0.86 (0.02) | 0.78 (0.07) | 0.94 (0.02) | 0.81 (0.03) | 0.65 (0.10) | |
450 | 5.06 | 8.55 | 0.92 (0.04) | 0.86 (0.02) | 0.78 (0.07) | 0.95 (0.02) | 0.81 (0.03) | 0.65 (0.10) | |
BayesB | 150 | 1.69 | 2.85 | 0.86 (0.03) | 0.82 (0.03) | 0.76 (0.07) | 0.87 (0.03) | 0.76 (0.04) | 0.62 (0.11) |
200 | 2.25 | 3.80 | 0.88 (0.03) | 0.83 (0.03) | 0.76 (0.09) | 0.89 (0.02) | 0.78 (0.03) | 0.64 (0.10) | |
250 | 2.81 | 4.75 | 0.90 (0.03) | 0.84 (0.02) | 0.75 (0.09) | 0.91 (0.02) | 0.79 (0.03) | 0.62 (0.09) | |
300 | 3.37 | 5.71 | 0.91 (0.03) | 0.85 (0.02) | 0.77 (0.08) | 0.92 (0.02) | 0.79 (0.03) | 0.63 (0.11) | |
350 | 3.93 | 6.65 | 0.91 (0.03) | 0.85 (0.02) | 0.78 (0.07) | 0.93 (0.02) | 0.80 (0.03) | 0.64 (0.10) | |
400 | 4.49 | 7.61 | 0.92 (0.03) | 0.86 (0.02) | 0.78 (0.07) | 0.93 (0.02) | 0.80 (0.03) | 0.64 (0.10) | |
450 | 5.06 | 8.55 | 0.92 (0.04) | 0.86 (0.02) | 0.78 (0.07) | 0.93 (0.02) | 0.80 (0.03) | 0.64 (0.09) |
For both traits and across all levels of NH, the prediction accuracy was highest for T2 hybrids, followed by T1 and T0 hybrids (Table 2). Prediction accuracies of GY were higher than those of GM for T1 and T0 hybrids but the opposite was true for T2 hybrids.
The prediction accuracy rA increased with increasing NH similarly for both traits (Table 2). The increase in rA was strongest for the T2 hybrids, followed by T1 and T0 hybrids. For example, the average increase in rA from NH = 150 to NH = 450 was 0.06 for T2 hybrids, 0.04 for T1 hybrids, and 0.025 for T0 hybrids. For the T2 and T1 hybrids the accuracy still increased in the higher range of NH, while for T0 hybrids the rA values did not increase further above NH = 300.
Keeping NH constant, but increasing ND and NF, decreased the prediction accuracy for T2 hybrids for both traits (Table 3). The difference in rA between the high ND and NF scenario and the low ND and NF scenario was 0.02 (GY) and 0.04 (GM). For GM, rA of the T1 and T0 hybrids increased with increasing ND and NF (difference 0.03). Altering ND and NF had no effect on rA values of T0 and T1 hybrids for GY.
Discussion
Consistency of linkage phases and marker effects across heterotic groups
Establishing separate training sets of sufficient size for small breeds in animal breeding or for different germplasm groups in plant breeding is generally too expensive. In this situation, pooling data sets from several germplasm groups can increase the power of genomic selection, as demonstrated by Technow et al. (2013) for disease resistance in maize. In cattle breeding, too, augmenting training sets with individuals from other breeds increased prediction accuracy to some extent (De Roos et al. 2009; Hayes et al. 2009; Erbe et al. 2012; Weber et al. 2012).
Habier et al. (2007, 2013) showed by simulation and theory that genomic prediction methods such as GBLUP and BayesB can exploit information from pedigree relationships, cosegregation, and LD for prediction. Owing to the long separation of cattle breeds and heterotic groups in maize, respectively, pedigree relationships and cosegregation can be ruled out as sources of information shared across groups, leaving only LD.
For major cattle breeds (De Roos et al. 2008) and for the Dent and Flint heterotic groups in maize (Technow et al. 2013), linkage phases between SNP markers were indeed similar across breeds and heterotic groups, respectively. We confirmed the latter result and could further show that the consistency of linkage phases is highest in pericentromeric regions of the maize genome.
However, LD between markers is not necessarily a good indicator for LD between markers and QTL, especially when the latter have a much lower minor allele frequency than the former (Yang et al. 2010). To investigate the consistency of marker–QTL LD and linkage phases across breeds, Weber et al. (2012) compared marker effect estimates of several cattle breeds, because a high similarity of marker effect profiles across breeds would reflect consistency in marker–QTL LD. They found the similarity to be low and concluded that LD between markers and QTL did not persist across breeds.
Factorial crosses between lines of two heterotic groups represent an ideal material for comparison of estimated additive marker effects of each group without confounding by different genetic backgrounds and environments. This is because each genotype of a single-cross hybrid represents a perfect combination of the two parental genomes without recombination.
In our study, additive marker effects estimated simultaneously for Dent and Flint were generally not consistent across these groups. However, we observed that there is a considerable consistency of marker effects in pericentromeric regions, in particular for GY. We therefore hypothesize that the increase in prediction accuracy observed by Technow et al. (2013) when combining Dent and Flint lines in a training set was mostly attributable to the pericentromeric regions of the genome, where linkage phases between markers and QTL are consistent across Flint and Dent. Regional differences in LD were also observed for cattle breeds (Sargolzaei et al. 2008). Thus, similar to maize, increases in prediction accuracy from pooled multibreed training sets might be driven by particular genomic regions with high linkage phase consistency across breeds.
An alternative approach to pooling for incorporating information from different breeds or germplasm groups was proposed by Brøndum et al. (2012). They described how genome position-specific priors for estimation of marker effects in one dairy cattle breed can be derived from marker effects estimated in a different breed. Using these genome position-specific priors increased prediction accuracy within each breed. The method of Brøndum et al. (2012) does not require consistent linkage phase between breeds but only identical QTL positions. However, in this way priors can be specified only for marker effect shrinkage parameters. If marker–QTL linkage phases are consistent across populations, as seems to be the case in pericentromeric regions of maize, priors could be derived for the marker effects themselves, too. This could be achieved, for example, by changing the prior mean of the marker effect from zero to the posterior mean of the marker effect estimated in the other breed, population, or heterotic group.
Estimation of population-specific marker effects for genomic prediction of crossbreds and single-cross hybrids
There are many parallels between hybrid breeding in crops like maize and crossbreeding in livestock production. In simulation studies on genomic prediction with training sets consisting of crossbred individuals (Ibánez-Escriche et al. 2009; Zeng et al. 2013) or single-cross hybrids (Technow et al. 2012), it was found that genomic prediction models that fitted specific marker effects for the parental populations (i.e., purebred breed or heterotic group) had little or no advantage over simpler models that assumed marker effects to be the same across parental populations. One explanation the authors gave for this was that the linkage phase consistency across populations was sufficiently high at high marker densities. In addition, the authors argued that the strongly increased dimensionality of those models prevented them from efficiently capturing remaining across-population differences in marker effects. Knowing in which genomic regions marker–QTL linkage phases are consistent or not could also be used for developing models that estimate population-specific marker effects only where necessary. This would reduce the dimensionality of these models and might mitigate some of the problems associated with it.
We also observed that fitting marker effects to be the same across heterotic groups delivered virtually the same prediction accuracy as model (2) in which specific marker effects were estimated for Dent and Flint (results not shown). This seems to contradict our observation that marker effects are consistent only in pericentromeric regions. However, as is discussed later in detail, prediction of hybrid performance is mostly driven by the presence of close relatives in the training set, in particular for T2 and T1 hybrids. As shown by Habier et al. (2007), BayesB can capture such pedigree relationships, particularly when many markers are fitted. Capturing pedigree relationships with markers does not require physical linkage between them and the QTL (Habier et al. 2013). Consistency of marker–QTL linkage phase might therefore not be mandatory for accurate predictions when close relatives are present in the training set.
Hill–Robertson effect and heterosis
It is known that recombination is suppressed in the pericentromeric regions of maize chromosomes (Gore et al. 2009; Schnable et al. 2009; Ganal et al. 2011; Bauer et al. 2013) and while gene density is comparably low in these regions (Schnable et al. 2009), they still contain a considerable portion of genes (Gore et al. 2009). The Hill–Robertson effect (Hill and Robertson 1966; Felsenstein 1974) describes the influence of recombination on selection efficiency. This effect predicts a buildup of repulsion-phase linkage between QTL alleles when recombination is suppressed (McVean and Charlesworth 2000). One consequence of repulsion-phase linkage is pseudo-overdominance, because additive QTL effects cancel out. Based on the Hill–Robertson effect, McMullen et al. (2009) hypothesized that the strongly suppressed recombination in pericentromeric regions of maize results in pseudo-overdominance and is therefore a major cause of heterosis. Larièpe et al. (2012) mapped dominance QTL in Dent × Flint crosses for important agronomic traits, using a North Carolina III design, and found a large proportion of QTL with (pseudo-)overdominance in pericentromeric regions. Schön et al. (2010) observed the same for Stiff-Stalk Synthetic × Non-Stiff-Stalk crosses. They concluded that pseudo-overdominance in pericentromeric regions led to differential fixation of QTL alleles in each heterotic group. We found the largest allele frequency differences between Dent and Flint in pericentromeric regions and therefore conclude that also for the Dent × Flint heterotic pattern differential fixation in pericentromeric regions takes place.
As allele frequencies in opposite heterotic groups drift apart during reciprocal recurrent selection (Labate et al. 1999), the ratio of SCA variance to GCA variance decreases (Reif et al. 2007) and dominance effects are increasingly absorbed into the population mean or become inseparable from additive effects (i.e., when QTL are fixed in one group but still segregate in the other). In particular, QTL with strongly positive (pseudo-)dominance or (pseudo-)overdominance effects are expected to be affected by differential fixation. The dominance effects of these QTL increase the “baseline” heterosis of the Dent × Flint heterotic pattern but are not detectable with statistical means in our set of Dent × Flint interpool hybrids. This can explain why dominance marker effects had positive and negative signs in almost equal proportions even though dominance effects for grain yield in maize are expected to be mostly positive (Schön et al. 2010). It also explains the absence of any noticeable accumulation of major dominance marker effect estimates in pericentromeric regions.
Comparison of prediction methods
GBLUP and BayesB achieved nearly identical prediction accuracies. Both GY and GM are considered to be highly polygenic traits, based on QTL mapping results (Schön et al. 2004; Huang et al. 2010). Several authors found in simulation studies and for real data sets that GBLUP models were superior to or equally well performing as Bayesian whole-genome regression methods for such traits (Zhong et al. 2009; Hayes et al. 2010; Clark et al. 2011; Kärkkäinen and Sillanpää 2012; Technow and Melchinger 2013; Wimmer et al. 2013). Daetwyler et al. (2010) arrived at the same conclusion based on theoretical results. Zhao et al. (2013) compared several methods for genomic prediction of grain yield of wheat hybrids and also found that GBLUP delivers the same or slightly higher prediction accuracy than BayesB. Thus, no substantial differences between both methods are expected for prediction of hybrid performance for traits like GY and GM.
If the effects of single QTL in polygenic traits vary considerably in size, adaptively shrinking Bayesian whole-genome regression methods could potentially outperform GBLUP. Furthermore, we hypothesize that BayesB could have an advantage for prediction of performance of T0 hybrids from lines distantly related to the parents of the training set hybrids, because then prediction accuracy would mainly come from short-range LD, which is not captured optimally by GBLUP (Habier et al. 2013).
Bayesian whole-genome regression methods can suffer from a lack of likelihood identifiability, when the number of markers is much larger than the size of the training set (Gianola 2013). This can lead to computational and convergence problems in Gibbs sampling (Gelfand and Sahu 1999). As reported by Technow and Melchinger (2013), nonidentifiability can impair prediction accuracy. Our BayesB model for prediction of hybrid performance fits up to three effects per marker, thereby exacerbating the problem. Consequently, Bayesian whole-genome regression methods require larger sizes of the training set for realizing a potential advantage.
Technow et al. (2012) confirmed in a simulation study that BayesB can achieve slightly higher prediction accuracy than GBLUP under a polygenic trait architecture, with a training set comprising 800 hybrids. Assembling large training sets is possible even for moderately sized breeding programs, like the one of the University of Hohenheim. With our data set, for example, a training set of 1254 hybrids could have been assembled, albeit without the possibility of performing a thorough cross-validation. Nonetheless, given the considerably greater computational demands of Bayesian whole-genome regression methods, GBLUP seems to be a very pragmatic and robust method for genomic prediction of hybrid performance for polygenic traits.
Prediction accuracy of T2, T1, and T0 hybrids
We confirmed the sizeable differences in prediction accuracy between T2, T1, and T0 hybrids found in the simulation study of Technow et al. (2012). The same was observed by Maenhout et al. (2010) and Schrag et al. (2010), who compared only T1 and T0 hybrids. These differences can be explained by the different numbers of parents of the hybrids that are also parents of training set hybrids (i.e., two, one, and zero for T2, T1, and T0 hybrids, respectively); the more that are shared, the higher the accuracy that can be expected (Technow et al. 2012). The paramount importance of pedigree relationships relative to other potential sources of accuracy like LD between markers and QTL was convincingly substantiated by Wientjes et al. (2012). In the human genetics context, De Los Campos et al. (2013) have derived an upper limit for the prediction accuracy that is a function of the accumulated relationship between individuals in the training and testing sets, respectively. The importance of close relatives for achieving highly accurate predictions was also observed in an animal breeding context (Legarra et al. 2008; Habier et al. 2010).
Because of the rapidly expanding arrays of genotyped lines, the number of T1 and T0 hybrids will eclipse the number of T2 hybrids. For example, if 1000 lines are available per heterotic group, of which 100 are parents of hybrids in the training set, the number of T1 hybrids reaches 180,000 and the number of T0 hybrids a staggering 810,000, while there are “only” 10,000 T2 hybrids (minus those in the training set). Thus, by sheer numbers, the best hybrids are most likely found among T1 and T0 hybrids. However, owing to the lower prediction accuracies, it will be more difficult to identify them, compared to identifying superior T2 hybrids. Breeders are unlikely to rely solely on genomic predictions when selecting potential hybrids for commercialization. Rather, genomic prediction will be employed as an initial stage in a multistage selection scheme, involving field testing of the most promising experimental hybrids. The number of experimental hybrids that can be tested in such a manner is limited by budget constraints. For practical application of genomic prediction, it is therefore important to investigate how the preselection of hybrids should be informed by the different prediction accuracies observed in the three groups.
In an earlier study on genomic prediction of hybrid performance for GY and GM, Massman et al. (2013) also found high prediction accuracies with training set sizes comparable to ours. The most likely explanation for the high prediction accuracies generally observed is that both H2 and the realized relationships among parental lines tend to be very high in commercial maize breeding programs. For example, our high estimates of H2 were for both traits in close agreement to those of Schrag et al. (2006) and Massman et al. (2013), the latter of which analyzed data from a U.S. corn-belt breeding program. Massman et al. (2013) also found similarly high pairwise realized relationships to those in our study (details not shown). For prediction of breeding values under an additive genetic model, a trait with high H2 is expected to have higher rA values than a trait with low H2 (Daetwyler et al. 2010). In our study, however, the rA values observed for GM, which had a considerably higher H2 than GY, were higher than rA for GY only for T2 hybrids, but lower for T1 and T0 hybrids. Interestingly, Massman et al. (2013) reported exactly the same findings, with GM having higher rA values than GY for T2 hybrids but lower values for T1 hybrids (there were no T0 hybrids in their study). Regional differences in LD, as found in our study, are a possible explanation why the relationship between heritability and prediction accuracy differs strongly among traits (Habier et al. 2013). We hypothesize that the contribution of information from LD to the prediction accuracy differs between T2, T1, and T0 hybrids. Regional differences in LD, therefore, can also explain why the relationship between heritability and prediction accuracy is inconsistent not only among traits, but also between T2, T1, and T0 hybrids.
Composition of training set
Prediction accuracy increased with increasing training set size NH, as expected (Table 2). However, the increase was relatively small, even when NH was tripled. This is in contrast to studies on genomic prediction of additive breeding values in plant breeding, where tripling NH could double the accuracy (Asoro et al. 2011; Technow et al. 2013). One explanation for this is the already rather high level of prediction accuracy reached. On the other hand, accuracy increased for T2 hybrids more than for T0 hybrids, even though their prediction accuracy was already higher for small NH.
The key point is that increasing NH for constant ND and NF does not eliminate the weakness of limited sampling of different GCA effects from each parental germplasm pool. It only increases the number of crosses
Nonetheless, increasing the number of hybrids
Increasing the number of tested lines ND and NF while keeping NH constant decreased the number of hybrids per line
An increase of 40 lines and a 34% and 53% decrease in
Habier et al. (2010) studied the role of pedigree relationships on accuracy of genomic prediction in German Holstein cattle. They found that prediction accuracy decreased only slightly when the training set size was halved as long as the number of close relatives per validation set individual remained constant. In a study on genomic prediction in maize, Albrecht et al. (2011) also observed that the drop in prediction accuracy was small when the training set size was halved. This was most likely a consequence of the presence of close relatives, too, because in their cross-validation scheme, an individual in the validation set had in most cases several full sibs in the training set. These studies demonstrate the disproportional importance of the closest relatives for prediction accuracy. Per definition, hybrids from the T2 and T1 groups always have very close relatives in the training set that share 50% of their genome. However, for hybrids from the T0 group, too, the maximum genomic relationships (i.e., estimated from marker data) to hybrids in the training set remained virtually unchanged by varying NH or ND and NF (results not shown). This is a consequence of the high degree of relationship between the inbred lines in a closed, medium-sized breeding program. The rather small differences in prediction accuracy between the various scenarios investigated reflect the presence of close relatives that determined prediction accuracy. A comparison of the prediction accuracies of our genomic methods with those of pedigree-based methods (Bernardo 1996) could be used to quantify the contribution of pedigree relationships to the prediction accuracy. However, different from the situation in animal breeding, pedigrees of our lines were often incomplete and rarely extended more than two generations. A simulation study, in which pedigree relationships are known without error and maximum relationships can be varied, might help to clarify the role that close relatives have in determining the accuracy with which hybrid performance can be predicted.
Estimates of rA showed a larger variation for the T0 group compared to the T2 and T1 groups. The lower variability for the T1 and T2 groups can be explained by the guaranteed presence of close relatives in the training set. The maximum relationship of T0 group hybrids with hybrids in the training set fluctuates between replications (even though it is high and similar between scenarios, on average). However, technical limitations, such as sampling constraints and different sizes of validation groups, most likely also contributed to the observed differences.
Back to the basics: a paradigm shift
Shull (1908), the inventor of hybrid breeding, recognized that a field (i.e., population) of maize is a mixture of many unique hybrids. Based on this, he defined the tasks of a maize breeder as (a) identifying the best hybrid and (b) reproducing it on a large scale. However, the classical approach of hybrid breeding with recurrent selfing has put great weight on the identification of inbred line parents with superior per se and testcross performance. Testing of experimental hybrids was carried out only in the very last stage of each breeding cycle, when the genetic variability was already largely exhausted. Genomic prediction of hybrid performance allows focusing on single-cross hybrids from the very beginning. At the same time, doubled-haploid technology and high-throughput genotyping facilitate direct capturing and genetic characterization of vast arrays of lines from each heterotic group. Together, these technologies enable a paradigm shift in hybrid breeding and direct implementation of Shull’s groundbreaking ideas for the first time.
Acknowledgments
We acknowledge the excellent technical assistance of the staff members of the maize breeding program of the University of Hohenheim, especially Dietrich Klein, in conducting part of the field trials for this study. We are indebted to the group of Ruedi Fries, from Technische Universität München, for the SNP genotyping of the parental lines. We also thank the three anonymous reviewers of the initial version of the manuscript for their very helpful input and suggestions. This research was funded by the German Federal Ministry of Education and Research within the AgroClustEr “Synbreed—Synergistic plant and animal breeding” (FKZ:0315528d).
Footnotes
Communicating editor: A. Charcosset
Literature Cited
- Albrecht T., Wimmer V., Auinger H.-J., Erbe M., Knaak C., et al. , 2011.
Genome-based prediction of testcross values in maize. Theor. Appl. Genet. 123: 339–350. [Abstract] [Google Scholar]
- Asoro F. G., Newell M. A., Beavis W. D., Scott M. P., Jannink J.-L., 2011.
Accuracy and training population design for genomic selection on quantitative traits in elite North American oats. Plant Genome 4: 132–144. [Google Scholar]
- Bauer E., Falque M., Walter H., Bauland C., Camisan C., et al. , 2013.
Intraspecific variation of recombination rate in maize. Genome Biol. 14: R103. [Europe PMC free article] [Abstract] [Google Scholar]
- Bernardo R., 1996.
Best linear unbiased prediction of maize single-cross performance. Crop Sci. 36: 50–56. [Abstract] [Google Scholar]
- Brøndum R. F., Su G., Lund M. S., Bowman P. J., Goddard M. E., et al. , 2012.
Genome position specific priors for genomic prediction. BMC Genomics 13: 543. [Europe PMC free article] [Abstract] [Google Scholar]
- Clark S., Hickey J. M., van der Werf J. H., 2011.
Different models of genetic variation and their effect on genomic evaluation. Genet. Sel. Evol. 43: 18. [Europe PMC free article] [Abstract] [Google Scholar]
- Daetwyler H. D., Pong-Wong R., Villanueva B., Woolliams J. A., 2010.
The impact of genetic architecture on genome-wide evaluation methods. Genetics 185: 1021–1031. [Europe PMC free article] [Abstract] [Google Scholar]
- de Los Campos G., Vazquez A. I., Fernando R., Klimentidis Y. C., Sorensen D., 2013.
Prediction of complex human traits using the genomic best linear unbiased predictor. PLoS Genet. 9: e1003608. [Europe PMC free article] [Abstract] [Google Scholar]
- de Roos A. P. W., Hayes B. J., Spelman R. J., Goddard M. E., 2008.
Linkage disequilibrium and persistence of phase in Holstein-Friesian, Jersey and Angus cattle. Genetics 179: 1503–1512. [Europe PMC free article] [Abstract] [Google Scholar]
- de Roos A. P. W., Hayes B. J., Goddard M. E., 2009.
Reliability of genomic predictions across multiple populations. Genetics 183: 1545–1553. [Europe PMC free article] [Abstract] [Google Scholar]
- Duvick D., 1999.
Heterosis: feeding people and protecting natural resources, pp. 19–29 in The Genetics and Exploitation of Heterosis in Crops, edited by Coors J., Pandey S., editors. CSSA, Madison, WI. [Google Scholar]
- Erbe M., Hayes B. J., Matukumalli L. K., Goswami S., Bowman P. J., et al. , 2012.
Improving accuracy of genomic predictions within and between dairy cattle breeds with imputed high-density single nucleotide polymorphism panels. J. Dairy Sci. 95: 4114–4129. [Abstract] [Google Scholar]
- Felsenstein J., 1974.
The evolutionary advantage of recombination. Genetics 78: 737–756. [Europe PMC free article] [Abstract] [Google Scholar]
- Ganal M. W., Durstewitz G., Polley A., Bérard A., Buckler E. S., et al. , 2011.
A large maize (Zea mays L.) SNP genotyping array: development and germplasm genotyping, and genetic mapping to compare with the B73 reference genome. PLoS ONE 6: e28334. [Europe PMC free article] [Abstract] [Google Scholar]
- Gelfand A. E., Sahu S. K., 1999.
Identifiability, improper priors and Gibbs sampling for generalized linear models. J. Am. Stat. Assoc. 94: 247–253. [Google Scholar]
- Gianola D., 2013.
Priors in whole-genome regression: the Bayesian alphabet returns. Genetics 194: 573–596. [Europe PMC free article] [Abstract] [Google Scholar]
- Gore M. A., Chia J.-M., Elshire R. J., Sun Q., Ersoz E. S., et al. , 2009.
A first-generation haplotype map of maize. Science 326: 1115–1117. [Abstract] [Google Scholar]
- Habier D., Fernando R. L., Dekkers J. C. M., 2007.
The impact of genetic relationship information on genome-assisted breeding values. Genetics 177: 2389–2397. [Europe PMC free article] [Abstract] [Google Scholar]
- Habier D., Tetens J., Seefried F.-R., Lichtner P., Thaller G., 2010.
The impact of genetic relationship information on genomic breeding values in German Holstein cattle. Genet. Sel. Evol. 42: 5. [Europe PMC free article] [Abstract] [Google Scholar]
- Habier D., Fernando R. L., Garrick D. J., 2013.
Genomic-BLUP decoded: a look into the black box of genomic prediction. Genetics 194: 597–607. [Europe PMC free article] [Abstract] [Google Scholar]
- Hayes B. J., Bowman P. J., Chamberlain A. C., Verbyla K., Goddard M. E., 2009.
Accuracy of genomic breeding values in multi-breed dairy cattle populations. Genet. Sel. Evol. 41: 51. [Europe PMC free article] [Abstract] [Google Scholar]
- Hayes B. J., Pryce J., Chamberlain A. J., Bowman P. J., Goddard M., 2010.
Genetic architecture of complex traits and accuracy of genomic prediction: coat colour, milk-fat percentage, and type in Holstein cattle as contrasting model traits. PLoS Genet. 6: e1001139. [Europe PMC free article] [Abstract] [Google Scholar]
- Henderson C., 1973.
Sire evaluation and genetic trends. J. Anim. Sci. 1973: 10–41. [Google Scholar]
- Henderson C., 1985.
Best linear unbiased prediction of nonadditive genetic merits in noninbred populations. J. Anim. Sci. 60: 111–117. [Google Scholar]
- Hill W. G., Robertson A., 1966.
The effect of linkage on limits to artificial selection. Genet. Res. 8: 269–294. [Abstract] [Google Scholar]
- Huang Y.-F., Madur D., Combes V., Ky C. L., Coubriche D., et al. , 2010.
The genetic architecture of grain yield and related traits in Zea maize L. revealed by comparing intermated and conventional populations. Genetics 186: 395–404. [Europe PMC free article] [Abstract] [Google Scholar]
- Ibánez-Escriche N., Fernando R. L., Toosi A., Dekkers J. C. M., 2009.
Genomic selection of purebreds for crossbred performance. Genet. Sel. Evol. 41: 12. [Europe PMC free article] [Abstract] [Google Scholar]
- Jannink J.-L., Lorenz A. J., Iwata H., 2010.
Genomic selection in plant breeding: from theory to practice. Brief. Funct. Genomics Proteomics 9: 166–177. [Abstract] [Google Scholar]
- Kärkkäinen H. P., Sillanpää M. J., 2012.
Back to basics for Bayesian model building in genomic selection. Genetics 191: 969–987. [Europe PMC free article] [Abstract] [Google Scholar]
- Labate J., Lamkey K., Lee M., Woodman W., 1999.
Temporal changes in allele frequencies in two reciprocally selected maize populations. Theor. Appl. Genet. 99: 1166–1178. [Google Scholar]
- Larièpe A, Mangin B, Jasson S, Combes V, Dumas F.
et al, 2012.
The genetic basis of heterosis: multiparental quantitative trait loci mapping reveals contrasted levels of apparent overdominance among traits of agronomical interest in maize (Zea mays L.). Genetics 190: 795–811. [Europe PMC free article] [Abstract] [Google Scholar]
- Legarra A., Robert-Granié C., Manfredi E., Elsen J.-M., 2008.
Performance of genomic selection in mice. Genetics 180: 611–618. [Europe PMC free article] [Abstract] [Google Scholar]
- Lippman Z. B., Zamir D., 2006.
Heterosis: revisiting the magic. Trends Genet. 23: 60–66. [Abstract] [Google Scholar]
- Maenhout S., De Baets B., Haesaert G., 2010.
Prediction of maize single-cross hybrid performance: support vector machine regression vs. best linear prediction. Theor. Appl. Genet. 120: 415–427. [Abstract] [Google Scholar]
- Massman J. M., Gordillo A., Lorenzana R. E., Bernardo R., 2013.
Genomewide predictions from maize single-cross data. Theor. Appl. Genet. 126: 13–22. [Abstract] [Google Scholar]
- McMullen M. D., Kresovich S., Villeda H. S., Bradbury P., Li H., et al. , 2009.
Genetic properties of the maize nested association mapping population. Science 325: 737–740. [Abstract] [Google Scholar]
- McVean G. A., Charlesworth B., 2000.
The effects of Hill-Robertson interference between weakly selected mutations on patterns of molecular evolution and variation. Genetics 155: 929–944. [Europe PMC free article] [Abstract] [Google Scholar]
- Melchinger A. E., Gumber R. K., 1998.
Overview of heterosis and heterotic groups in agronomic crops, pp. 29–44 in Concepts and Breeding of Heterosis in Crop Plants, edited by Lamkey K. R., Staub J. E., editors. CSSA, Madison, WI. [Google Scholar]
- Meuwissen T. H. E., Hayes B. J., Goddard M. E., 2001.
Prediction of total genetic value using genome-wide dense marker maps. Genetics 157: 1819–1829. [Europe PMC free article] [Abstract] [Google Scholar]
- Möhring J., Piepho H.-P., 2009.
Comparison of weighting in two-stage analysis of plant breeding trials. Crop Sci. 49: 1977–1988. [Google Scholar]
- R Development Core Team, 2012
R: A Language and Environment for Statistical Computing. R Development Core Team, Vienna. [Google Scholar]
- Reif J. C., Gumpert F.-M., Fischer S., Melchinger A. E., 2007.
Impact of interpopulation divergence on additive and dominance variance in hybrid populations. Genetics 176: 1931–1934. [Europe PMC free article] [Abstract] [Google Scholar]
- Reif J. C., Zhao Y., Würschum T., Gowda M., Hahn V., 2013.
Genomic prediction of sunflower hybrid performance. Plant Breed. 132: 107–114. [Google Scholar]
- Rincent R., Laloe D., Nicolas S., Altmann T., Brunel D., et al. , 2012.
Maximizing the reliability of genomic selection by optimizing the calibration set of reference individuals: comparison of methods in two diverse groups of maize. Genetics 192: 715–728. [Europe PMC free article] [Abstract] [Google Scholar]
- Sargolzaei M., Schenkel F. S., Jansen G. B., Schaeffer L. R., 2008.
Extent of linkage disequilibrium in Holstein cattle in North America. J. Dairy Sci. 91: 2106–2117. [Abstract] [Google Scholar]
- Schnable P. S., Ware D., Fulton R. S., Stein J. C., Wei F., 2009.
The B73 maize genome: complexity, diversity, and dynamics. Science 326: 1112–1115. [Abstract] [Google Scholar]
- Schön C. C., Utz H. F., Groh S., Truberg B., Openshaw S., et al. , 2004.
Quantitative trait locus mapping based on resampling in a vast maize testcross experiment and its relevance to quantitative genetics for complex traits. Genetics 167: 485–498. [Europe PMC free article] [Abstract] [Google Scholar]
- Schön C. C., Dhillon B. S., Utz H. F., Melchinger A. E., 2010.
High congruency of QTL positions for heterosis of grain yield in three crosses of maize. Theor. Appl. Genet. 120: 321–332. [Abstract] [Google Scholar]
- Schrag T. A., Melchinger A. E., Sørensen A. P., Frisch M., 2006.
Prediction of single-cross hybrid performance for grain yield and grain dry matter content in maize using AFLP markers associated with QTL. Theor. Appl. Genet. 113: 1037–1047. [Abstract] [Google Scholar]
- Schrag T. A., Möhring J., Melchinger A. E., Kusterer B., Dhillon B. S., et al. , 2010.
Prediction of hybrid performance in maize using molecular markers and joint analyses of hybrids and parental inbreds. Theor. Appl. Genet. 120: 451–461. [Abstract] [Google Scholar]
- Shull G. H., 1908.
The composition of a field of maize. J. Hered. os-4: 296–301. [Google Scholar]
- Silva Dias J. C., 2010.
Impact of improved vegetable cultivars in overcoming food insecurity. Euphytica 176: 125–136. [Google Scholar]
- Stuber C., Cockerham C., 1966.
Gene effects and variances in hybrid populations. Genetics 54: 1279–1286. [Europe PMC free article] [Abstract] [Google Scholar]
- Technow F., Melchinger A. E., 2013.
Genomic prediction of dichotomous traits with Bayesian logistic models. Theor. Appl. Genet. 126: 1133–1143. [Abstract] [Google Scholar]
- Technow F., Riedelsheimer C., Schrag T. A., Melchinger A. E., 2012.
Genomic prediction of hybrid performance in maize with models incorporating dominance and population specific marker effects. Theor. Appl. Genet. 125: 1181–1194. [Abstract] [Google Scholar]
- Technow F., Bürger A., Melchinger A. E., 2013.
Genomic prediction of northern corn leaf blight resistance in maize with combined or separated training sets for heterotic groups. G3 3: 197–203. [Europe PMC free article] [Abstract] [Google Scholar]
- VanRaden P. M., 2008.
Efficient methods to compute genomic predictions. J. Dairy Sci. 91: 4414–4423. [Abstract] [Google Scholar]
- Weber K. L., Thallman R. M., Keele J. W., Snelling W. M., Bennett G. L., et al. , 2012.
Accuracy of genomic breeding values in multibreed beef cattle populations derived from deregressed breeding values and phenotypes. J. Anim. Sci. 90: 4177–4190. [Abstract] [Google Scholar]
- Wedzony, M., B. Forster, I. Zur, E. Golemiec, M. Szechynska-Hebda et al., 2009
Progress in doubled haploid technology in higher plants, pp. 1–33 in Advances in Haploid Production in Higher Plants, edited by A. Touraev, B. Forster, and S. Jain. Springer-Verlag, Dordrecht, The Netherlands. [Google Scholar]
- Wientjes Y. C. J., Veerkamp R. F., Calus M. P. L., 2012.
The effect of linkage disequilibrium and family relationships on the reliability of genomic prediction. Genetics 193: 621–631. [Europe PMC free article] [Abstract] [Google Scholar]
- Wimmer V., Lehermeier C., Albrecht T., Auinger H.-J., Wang Y., et al. , 2013.
Genome-wide prediction of traits with different genetic architecture through efficient variable selection. Genetics 195: 573–587. [Europe PMC free article] [Abstract] [Google Scholar]
- Windhausen V. S., Atlin G. N., Hickey J. M., Crossa J., Jannink J.-L., et al. , 2012.
Effectiveness of genomic prediction of maize hybrid performance in different breeding populations and environments. G3 2: 1427–1436. [Europe PMC free article] [Abstract] [Google Scholar]
- Yang J., Benyamin B., McEvoy B. P., Gordon S., Henders A. K., et al. , 2010.
Common SNPs explain a large proportion of the heritability for human height. Nat. Genet. 42: 565–569. [Europe PMC free article] [Abstract] [Google Scholar]
- Zeng J., Toosi A., Fernando R. L., Dekkers J. C. M., Garrick D. J., 2013.
Genomic selection of purebred animals for crossbred performance in the presence of dominant gene action. Genet. Sel. Evol. 45: 11. [Europe PMC free article] [Abstract] [Google Scholar]
- Zhao Y., Zeng J., Fernando R., Reif J. C., 2013.
Genomic prediction of hybrid wheat performance. Crop Sci. 53: 802–810. [Google Scholar]
- Zhong S., Dekkers J. C. M., Fernando R. L., Jannink J.-L., 2009.
Factors affecting accuracy from genomic selection in populations derived from multiple inbred lines: a barley case study. Genetics 182: 355–364. [Europe PMC free article] [Abstract] [Google Scholar]
Articles from Genetics are provided here courtesy of Oxford University Press
Full text links
Read article at publisher's site: https://doi.org/10.1534/genetics.114.165860
Read article for free, from open access legal sources, via Unpaywall:
https://www.genetics.org/content/genetics/197/4/1343.full.pdf
Citations & impact
Impact metrics
Article citations
One Hundred Years of Progress and Pitfalls: Maximising Heterosis through Increasing Multi-Locus Nuclear Heterozygosity.
Biology (Basel), 13(10):817, 12 Oct 2024
Cited by: 0 articles | PMID: 39452126 | PMCID: PMC11504056
Review Free full text in Europe PMC
Genomic prediction of yield-related traits and genome-based establishment of heterotic pattern in maize hybrid breeding of Southwest China.
Front Plant Sci, 15:1441555, 09 Sep 2024
Cited by: 0 articles | PMID: 39315371 | PMCID: PMC11416964
Optimizing selection based on BLUPs or BLUEs in multiple sets of genotypes differing in their population parameters.
Theor Appl Genet, 137(5):104, 15 Apr 2024
Cited by: 0 articles | PMID: 38622324 | PMCID: PMC11018695
Portability of genomic predictions trained on sparse factorial designs across two maize silage breeding cycles.
Theor Appl Genet, 137(3):75, 07 Mar 2024
Cited by: 0 articles | PMID: 38453705 | PMCID: PMC11341662
Genomic prediction in multi-environment trials in maize using statistical and machine learning methods.
Sci Rep, 14(1):1062, 11 Jan 2024
Cited by: 4 articles | PMID: 38212638 | PMCID: PMC10784464
Go to all (103) article citations
Data
Data behind the article
This data has been text mined from the article, or deposited into data resources.
BioStudies: supplemental material and supporting data
Similar Articles
To arrive at the top five similar articles we use a word-weighted algorithm to compare words from the Title and Abstract of each citation.
Genomic prediction of hybrid performance in maize with models incorporating dominance and population specific marker effects.
Theor Appl Genet, 125(6):1181-1194, 26 Jun 2012
Cited by: 85 articles | PMID: 22733443
Genomic prediction of northern corn leaf blight resistance in maize with combined or separated training sets for heterotic groups.
G3 (Bethesda), 3(2):197-203, 01 Feb 2013
Cited by: 54 articles | PMID: 23390596 | PMCID: PMC3564980
Linkage disequilibrium with linkage analysis of multiline crosses reveals different multiallelic QTL for hybrid performance in the flint and dent heterotic groups of maize.
Genetics, 198(4):1717-1734, 29 Sep 2014
Cited by: 53 articles | PMID: 25271305 | PMCID: PMC4256782
Biochemical analyses of inbreds and their heterotic hybrids in maize.
Prog Clin Biol Res, 344:639-664, 01 Jan 1990
Cited by: 1 article | PMID: 2203058
Review