Abstract
Free full text

Genomics of immune diseases and new therapies
Abstract
Genomic DNA sequencing technologies have been one of the great advances of the 21st century – decreasing in cost by 7 orders of magnitude and opening up new fields of investigation throughout research and clinical medicine. Genomics coupled with biochemical investigation has allowed the molecular definition of a growing number of new genetic diseases that reveal new concepts of immune regulation. Also, defining the genetic pathogenesis of these diseases has led to improved diagnosis, prognosis, genetic counseling, and, most importantly, new therapies. We highlight the investigational journey from patient phenotype to treatment using the newly defined XMEN disease caused by the genetic loss of the MAGT1 magnesium transporter. This disease illustrates how genomics yields new fundamental immunoregulatory insights as well as how research genomics is integrated into clinical immunology. At the end, we discuss two other recently described diseases: PASLI (PI3K dysregulation) and CHAI/LATAIE (CTLA-4 deficiency) that show journeys from unknown immunological diseases to new precision medicine treatments using genomics.
“In almost all things, what they contain of useful or applicable nature is hardly perceived unless we are deprived of them, or they become deranged in some way.”
William Harvey (1657)
Introduction
Medical genetics became a medical subspecialty in the 1950’s with the hope that understanding the genetic basis of disease would accelerate diagnosis, prognosis, and treatments (1). The first genetic (primary) immunodeficiency, Bruton’s agammaglobulinemia, together with the first successful etiological therapy, intravenous immunoglobulin replacement, were defined in 1952 (2). However, four decades passed before the genetic and molecular technologies advanced enough to permit the identification of the causative gene, Bruton tyrosine kinase, BTK (3, 4). Within the past 5 years, new technologies for the investigation of genes has resulted in a cornucopia of newly defined immunodeficiencies and immunoregulatory diseases and an explosion of knowledge on human immune regulation (5, 6).
Genomics is the investigation of nucleotide sequence variants in the entire nuclear DNA content of an organism and their effect on phenotype. Up until recently, the molecular state of the genome in a patient’s cell was essentially unknowable. Next generation sequencing (NGS) technologies have now made it routine. Molecular genetic analysis in humans has also been accelerated by new techniques that allow biochemical and molecular analyses of human genetic defects with a rigor approaching that previously possible only in model organisms. These include efficient cell cultivation, improved electroporation techniques to introduce DNA into cells, and the ability to alter gene expression with interfering RNA or, more recently, genomic editing with techniques such as the clustered regularly interspaced short palindromic repeats (CRISPR)/Cas9 system (7). Gene mapping and candidate gene analyses for primary immunodeficiencies have been largely replaced with NGS (5, 6). Computational approaches have streamlined the identification and validation of causal gene variants. While cross-validation of human disease genes with genetically altered mice, a species that shares 99% of its genes with humans, is a mainstay of immunology, there are many examples in which human disease cannot be modeled in the mouse, such as caspase-8 deficiency or CTLA-4 haploinsufficiency (8, 9). Thus, we are now technologically capable of diving into an ocean of 3 billion nucleotides with a reasonable hope of swimming back to the surface with pearls of fundamental knowledge about the healthy and diseased immune system. This essay is about our experience making such deep sequence explorations.
Approaches to study the genetic contribution to disease
The first DNA sequence of a human genome introduced the possibility that genomics could yield new insights into cellular regulation and disease mechanisms (10). However, knowledge about function required comparative genomics in which DNA sequence variations between different individuals, especially those with specific diseases, were investigated. Early on, cost prevented the wide deployment of DNA sequencing, so alternative genomic screening strategies were developed. The hypothesis emerged that common single nucleotide polymorphisms (SNP) would correlate with common diseases (the common SNP-common disease hypothesis) (11). Relatively inexpensive hybridization chips were used to probe common well-defined SNPs in order to correlate predominant allele frequencies with a variety of diseases including most common autoimmune diseases, a procedure called genome wide association (GWA). For the SNPs on these chips, MHC class II alleles consistently showed significant and reproducible linkage disequilibrium with immunological diseases, but other associations showed only slight linkage (12). Specifically, very few SNPs in the coding portion of the genome had a large effect for any disease (11–13). Another complication of GWA studies is that many SNPs correlated with disease were in non-coding regions, had modest contribution to disease, and were not medically actionable (11–13). This was puzzling and left a large part of the inheritance inferred from other analyses, such as twin studies, unexplained. Also, these data were hard to interpret since they were based on statistical correlation, and determining whether they actually caused disease, though finally established in some cases, was difficult (11, 12). For certain complex diseases, GWA studies suggest that a group of gene variants, each with a small effect, act in concert to produce the disease phenotype (11–13).
As the cost of sequencing DNA dropped precipitously and access to NGS technology became widely available, emphasis shifted to direct gene sequence investigation. Currently genomic investigation uses NGS of exonic DNA captured by hybridization chips, “the exome”, or the entire nuclear DNA of a cell from a human subject, “the genome.” The study of individual exomes and genomes, now increasing exponentially and including a panoply of disease cohorts, yielded a big surprise. Every human genome sequenced contains a surprisingly immense number of rare variants compared to the reference genome and these are usually private, meaning that they are restricted to the immediate blood relatives of the family, parents, siblings, and children (14). Hence, the genomic “software” for every newborn is unique with many variants – generally bugs but occasionally improvements – that affect the cellular programs. Furthermore, in contrast to the weak effects of common SNPs, rare single nucleotide variants (SNVs) and copy number variants (CNVs) can have highly penetrant and deleterious effects on phenotype (14). Single nucleotide changes that are called SNPs are found at frequencies greater than 1% in a population and those with frequencies less than that, usually exceedingly low or unique to a single individual, are called SNVs. How can we interpret the difference in disease heritability between common SNPs and rare SNVs? Any DNA change arising in a family with strong deleterious effects would be eliminated from the population as it is passed on to successive generations because of decreased reproductive fitness. Thus, common SNPs would have been selected for weak disease effects in order to be successfully transmitted within the population. A caveat to this assertion is that common SNPs could not have been selected against by recent specific environmental influences such as drugs, toxins etc. and therefore, some may still have unexpectedly potent deleterious effects. On the other hand, most rare variants would be limited to one or a small number of generations in a family depending on how severely it affects reproductive fitness. The concept of rare, family-restricted variants that have severe phenotypes has been called “clan genomics” and pursuit of these variants has had a major impact on genomic investigation of diseases (14). Rare variation with large effect sizes is likely contributing a significant proportion to the “missing heritability” of complex traits and disease (14, 15).
Why have an astronomical number of private variants (thousands or more per genome) with low minor allele frequencies (MAFs), been uncovered in human genomic sequences around the world. This is likely because of our rapid evolutionary success and the dramatic increase of human populations in a comparatively short span of evolutionary time (16). There may also be increasing retention of disease susceptibility variants that formerly would have been selected out but now persist because of better health through hygiene and medicine. The other feature of clan genomics is homozygosity of private variants through consanguinity. Early in human history, certain isolated hunter-gatherer clans likely practiced a high level of endogamy, causing homozygous retention of private variants through consanguinity (17). As small populations moved or suffered from disease or starvation, the gene pool would be subject to genetic drift and founder effects through population bottlenecks. Even today, this tendency to consanguinity has persisted through social, economic, or religious forces. Large regions of the world, comprising roughly 10% of humanity, have frequent consanguineous reproduction that retains and promotes homozygosity of private SNVs, especially deleterious loss-of-function (LOF) mutants, without dispersing them throughout the regional or national population (16, 17).
Powerful and evolving NGS technologies, now provide the principal approach to identifying disease-associated SNVs and short (< 50 base pairs (bp)) insertions and deletions (Indels) (5, 6, 15, 18, 19). Whole genome sequencing of paired ends (WGS), comparative genomic hybridization (CGH) arrays, and chromosome analysis can identify structural variations (SVs, defined as > 50 bp) including CNVs and more complex genomic rearrangements (18, 19). Individual genomes may harbor nearly 10,000 SNVs, affecting almost 2% of the genome, which will be an important source of disease variants (18–20). These new genomic technologies provide an unbiased method to identify gene mutations in Mendelian, monogenic, and de novo inheritance patterns. Not all monogenic disorders can be considered Mendelian, since de novo gene variants that are congenital but not inherited, and by definition private, cause important disease phenotypes (18, 20). Family-based WGS studies have estimated that each individual’s genome contains many germline de novo mutations (DNMs) and that these are an important source of disease mutations (20–22). These mutations, found in the affected proband but not in the parents or siblings, are potentially more deleterious because they have not been subject to natural selection. DNA sequence analysis of selected cell populations can also uncover somatic mutations (SM) such as FAS gene mutations that are present in a subpopulation of CD4-, CD8- T cells but not in the germline of certain autoimmune lymphoproliferative syndrome (ALPS) patients (23). Somatic reversion can also ameliorate disease phenotype by “correcting” the genetic defect in appropriate cell lineages (24).
In consanguineous families, NGS of affected individuals together with parents and other affected or unaffected relatives combined with homozygosity mapping can efficiently identify disease-associated variants. Non-consanguineous, large multigenerational, and multiplex pedigrees can also be used to identify rare inherited variants. NGS has caused immunology research to emphasize forward genetics, in which a gene(s) variant is sought to explain a specific phenotype, usually a disease. Forward genetics utilizing NGS provides the best unbiased approach to correlating genotypes with phenotypes and has already added a substantial number of new entities to over 250 genetic diseases of the immune system (25). The new technology has also uncovered thousands of disease-associated human gene variants (14, 18, 19, 25). As more comprehensive datasets are assembled, correlating gene variants with various immunological pathologies and infection susceptibility will reveal the genetic landscape of immune diseases.
NGS data coupled with bioinformatics analyses have become increasingly valuable for diagnosing known genetic disorders but are generally not adequate on their own to conclusively implicate new genetic variant(s) in disease pathogenesis (26). When computational approaches do succeed, it is usually when there is a clearly defined clinical phenotype that occurs in multiple unrelated individuals and maps to the same gene in which nucleotide changes cause similar biochemical effects (27, 28). Additional biochemical and molecular validation is essential. This is because there are a large number of variants but the availability of affected samples for rare diseases is often limited. Also, there are surprisingly many severe LOF variants found in ostensibly normal people, almost 100 genes per individual with 20 being homozygous (29). The problem of inadequate validation has been documented in population studies showing that hundreds of variants reported in the human gene mutation database as disease alleles and lacking adequate biochemical validation could be found in normal individuals (15, 26, 30). Although statistical and bioinformatics tools will improve, the most convincing association of gene variants with disease will be based on molecular and biochemical validation in human cell lines or experimental organisms such as mice, zebrafish, or others (26, 31). Validation requires addressing three major issues: How does the nucleotide alteration affect the protein biochemically? How does the gene mutation fit a specific model of inheritance? This can be complicated by reduced penetrance or expressivity of the genetic change. Finally, how does the specific biochemical change derange the cellular pathways in a manner that potentially explains the observed phenotype/disease? As described below, validation is greatly simplified when a “cellular” phenotype that clearly relates to disease manifestations can be defined.
In the remainder of this review, we will walk through the genomic process of disease gene discovery starting with patient phenotype and ending with the molecular definition of a new disease and its therapy. We will use the example of identifying specific mutations in the MAGT1 gene as causative of “X-linked immunodeficiency with magnesium defect, Epstein-Barr virus (EBV) infection, and neoplasia” (XMEN) disease to illustrate the gene discovery process (32). These examples will be in italics. Knowing the identity of the XMEN disease gene offered new theoretical and basic insights into magnesium regulation as well as a direct etiological therapy for the most important consequence of the disease, uncontrolled active EBV infection (33, 34). At the end of the review, we will describe two additional diseases for which knowing the specific gene defect led directly to specific improvements in therapy for life-threatening immune diseases.
Clinical and laboratory phenotype
The process begins with the chief complaint that the patient brings to medical attention. It can be severe or, as was the case of XMEN patients, a mild increase in susceptibility to childhood infections (32). In the realm of immune disease, the phenotype often relates to deficiencies in immune responses manifested by viral, bacterial, fungal, or parasitic infections (2, 8, 35, 36). Conversely, it can involve overactive immune responses leading to cellular or antibody-mediated autoimmunity or atopic disease such as asthma (37, 38). Immune homeostasis can also be deranged with the accumulation of immune cells and/or the distortion of immune subsets. Often, there are combinations of these abnormalities that defy obvious explanation due to the complex web of regulatory interactions in immune responses, for example, severe immunodeficiency with excessive IgE (37). Reasons to believe an immune abnormality is primarily genetic include early-onset, occurrence in a multiplex family, marked severity of disease compared to the normal population, and a characteristic “syndrome” that emerges in unrelated families and cannot be attributed to environmental causes. Clinical lab examination, depending on the depth of analysis available, can uncover abnormal lymphocyte subsets and basic functional assays in B and T cell activation and proliferation. The collective clinical data becomes a foundation of the phenotype upon which the research lab builds.
The first two XMEN patients were brothers who had a clinical history of mild immunodeficiency with repeated but generally self-limited respiratory and gastrointestinal infections (32). Overall, the boys were developmentally normal and were otherwise apparently healthy on clinical exam. Clinical laboratory investigation revealed the fact that the boys had strikingly elevated EBV levels that ranged between 100,000 and 1 million copies per mL blood compared to normal individuals who are often completely negative but can occasionally have up to 800 copies per mL (34). Thus, the clinical phenotype, mild infectious history and astronomical levels of EBV in developmentally normal males, was defined by these analyses. Two affected males in the same family suggested a genetic basis.
Because of the general availability of cells in immune diseases through blood sampling, more elaborate tests can focus on defining the functional defects characteristic of the disorder. A key goal is to define a “cellular phenotype” as a benchmark of the disease. This clarifies the genetic model and provides a molecular confirmation of candidate genetic variants. Later, it can be used as a diagnostic test for individuals suspected of having the disease. Immunophenotyping by flow cytometry for lymphocyte subsets can document derangements in lymphoid development or survival, proliferation, and differentiation. Though this cannot survey tissue-resident immune cells, it can assess many distinct cell types.
In the case of XMEN patients, flow cytometry revealed a modest decrease in peripheral naïve CD4 T cells that inverted the CD4:CD8 ratio and was accompanied by a decreased number of CD31+ recent thymic emigrants (32). These alterations were mild, however, and would not have been expected to cause severe infectious consequences, especially the chronic active EBV infection. Functional analyses were more revealing by showing that both CD4 and CD8 T cells were impaired in upregulating activation markers including CD69, CD25, and CD95 after T cell receptor (TCR) cross-linking (32). Interestingly, this deficit was overcome by using a combination of second messenger mimics (phorbol ester and ionomycin), implying a defect in proximal TCR signaling. By contrast, the patient B cells were normal in number and response to B cell receptor and toll-like receptor stimulation (32).
Modes of inheritance
With the cellular and clinical phenotype in hand, NGS was a logical approach to deciphering XMEN disease, but evaluating SNVs depends on the presumptive mode of inheritance determined from the family pedigree (18, 19, 25, 26). The principal modes are derived from classic Mendelian recessive (both alleles abnormal and skips generations) and dominant (one allele abnormal and affects successive generations) concepts as well as the possibility of haploinsufficiency, X-linked disorders, and de novo occurrence of mutations. In current databases of Mendelian monogenic immune diseases, autosomal recessive (AR) disorders are 3–4 times more common than autosomal dominant (AD) diseases partly because they were the easiest to solve using classic approaches (25). Immunodeficiencies due to AR LOF mutations can be caused by homozygous alleles or two different LOF alleles (biallelic or compound heterozygous) (37, 38). In countries with high consanguinity, AR disorders involve LOF alleles that are usually homozygous. AD disorders are increasingly being reported and arise mainly in two genetic forms: activating - in which the variant allele has hyperactive function; and dominant-interfering - in which the variant allele impairs the function of the normal allele. AD disease, the other side of the Mendelian coin, have been increasingly identified because NGS can uncover causative mutations in small pedigrees or large numbers of unrelated people even with marked variations in penetrance. PASLI disease (p110δ-activating mutation causing senescent T cells, lymphadenopathy and immunodeficiency, also known as activated PI3K-δ syndrome, APDS) is an AD disease due to heterozygous mutations that upregulate phosphoinositol-3′ kinase activity due to changes either in the gene encoding the leukocyte-restricted p110δ enzymatic subunit or the ubiquitous p85α regulatory subunit (27, 28, 39, 40). How the enzymatic “gain of function” (GOF) causes lymphocyte proliferation, differentiation, and senescence with metabolic changes that prevent adequate immune responses will be described in greater depth below. The second type of AD mutations is dominant-interfering (DI) (41, 42). These mutations usually produce an anomalous protein that interferes with the function of the normal protein. For example, in ALPS, apoptosis signaling is inhibited by heterozygous alleles encoding defective FAS proteins that bind to the normal protein and hold it hostage in defective receptor complexes (41, 42). Similarly, in AD autoimmune polyendocrine syndrome (APS), mutations in the plant homology domain 1 (PHD1) generate defective AIRE proteins that sequester the normal AIRE protein in abortive transcriptional complexes in nuclear speckles (43). Rarely there can be genetic gain-of-function mutations that lead to immunodeficiency which some have called autosomal dominance of the “third” kind (44). One specialized Mendelian mode of inheritance, combining heterozygosity and LOF, is called haploinsufficiency (HI), for “half sufficiency” because the normal phenotype depends on full diploid gene dosage and a single defective allele reduces the amount of protein by half (45). These behave genetically like AD alleles. For example, patients with “CTLA-4 haploinsufficiency with autoimmune infiltration” (CHAI) disease have heterozygous, germline LOF mutations in CTLA4 (9, 46). These decrease CTLA-4, resulting in abnormal T cell infiltration and damage to organs such as the lung, brain, and bowel (9). Another class of mutations involves the X chromosome, termed X-linked recessive (XLR). These predominantly affect males who inherit hemizygous defective alleles from their mothers. There are several highly penetrant immunological XLR disorders including X-linked SCID, IPEX, and XMEN diseases (32, 47, 48). These resemble recessive mutations because they are inherited through an unaffected mother. Finally, other important classes of mutations that are not, strictly speaking, Mendelian, are DNMs, found in the proband but absent in parents and siblings. The DNA replication error rate is estimated to be 1 per 108 base pairs, generating 30–100 DNMs in each generation and serving as the source for unique private SNVs. These increase with paternal age and can also be mosaic by occurring post-zygotically (20, 49, 50). De novo mutations can be experimentally identified by NGS of trios comprising the parents and affected child (50, 51). A variant type of DNM occurs in somatic cells. SM usually contribute to genetic disease by conferring a proliferative or survival advantage as well as an abnormal phenotype in the affected cells. Cancer can be thought of as the chief example of this.
For XMEN disease, the occurrence of disease in two boys suggested the possibility of an XLR mode of inheritance. This hypothesis was supported by the skewing of X chromosome inactivation in the unaffected mother such that nearly all of her hematopoietic cells showed inactivation of the X chromosome that she transmitted to both boys (32). This provided strong prima facie evidence for a mutation on the X chromosome that was deleterious to her hematopoietic cells (hence only cells with the normal X chromosome could be found) and responsible for X-linked disease in her sons. Solution hybridization reagents targeting the X chromosome were used to prepare exons from the boys’ and the mother’s DNA for NGS. Sequencing of the captured DNA revealed a 10-base-pair deletion in a magnesium transporter gene called MAGT1 located on the X chromosome (32).
Phenotype variation
In reverse genetics, inbred mouse and rat strains aid the genetic engineering of disease models because it reduces background variation and generates consistent phenotypes. Although 10% of the world is consanguineous, modern human populations are generally highly outbred (16). Thus, in humans, the phenotypes of gene mutations can be highly variable because they shine through heterogeneous genomic atmospheres. This genetic admixing, together with environmental influences, makes it difficult, particularly with small numbers of patients with a given gene variant, to precisely define disease phenotype. Coupled to this vast tumult in gene mixing, human genetics has a powerful phenotype detection mechanism provided by the medical and research professions that globally detect rare and variable phenotypes. This has increased the pace of gene discovery and validation but also revealed how disease associated with a specific gene can present in many different guises.
In human genetics, variation associated with a specific genotype, particular for disease genes, was classically described in two ways: penetrance and expressivity (52–55). Penetrance refers to whether or not a phenotype, in a sort of all or none fashion, was present in individuals with the same gene mutation. Expressivity was used to describe pleiotropy associated with specific gene mutations. With the dizzying array of human conditions associated with genomic variations, the difference between these two concepts has been blurred. Penetrance probably best describes syndromes in which there is a highly stereotypical set of disease features where not everyone with a specific gene mutation gets the disease. For example, in ALPS, healthy parents harboring a dominant-interfering FAS mutation can pass the mutation to a child who develops a clinically significant disease (41). In this situation, where unaffected individuals harbor identical gene mutations to those with full-blown clinical disease, one would say the penetrance is incomplete. Mathematically, penetrance measures the proportion of affecteds in a given population of individuals with a specific genotype (55). In ALPS (AD) and CHAI disease (HI), penetrance ranges around 50 to 60% (9, 41, 45). No molecular explanation currently exists for this surprisingly low penetrance. It is also important to distinguish incomplete penetrance of a disease-causing variant from risk alleles for complex diseases that are identified in GWA studies and cannot, on their own, serve as a primary cause of disease. Variations in expressivity are ubiquitous; essentially every genetic disease associated with a specific gene shows variation in the severity and type of clinical manifestation. As a consequence, this term is used less often in contemporary genomics.
There are a multitude of causes for variation in penetrance and expressivity (Figure 1) (52–55). Some identified causes intrinsic to the genome are: the molecular nature of the gene mutation and the degree to which it is damaging to protein function; additional mutations either in the same gene (cis) or other genes (trans); inter-individual gene expression differences even between alleles; gene dosage; digenic disorders in which there are actually two obligatory causative mutations; sex dependence; age; imprinting; somatic mosaicism, and epigenetic modification. Of special significance are “modifier” genes (see below) that are often unlinked to the primary disease-causing gene but critical determinants of the genetic “atmosphere” that influences penetrance and expressivity. For example, low expression levels of the affected gene can lead to a more severe abnormal phenotype and this can be determined by genetic background (55). In addition there are extrinsic causes due to environmental influences on the affected individual (52–55). A good example of this is genetic susceptibility to viral pathogens or mycobacteria that is silent until exposure to the specific pathogen causes severe disease; individuals who never encounter the pathogen will have zero disease penetrance (56, 57). Differences in penetrance and expressivity create disease disguises that make it difficult to implicate a specific gene variant as the cause of a genetic disease. Violations of the mode of inheritance and inconsistencies in the pedigree, uncertainty about which disease characteristics are the most relevant, and confounding effects from disorders that are actually due to a different gene variant are significant challenges for genomic detective work. Only with further investigation of the specific genotype and phenotype, especially the accumulation of more patients with the same genotype and phenotype does the empiric association between the two become convincing.
There are approximately two dozen XMEN patients from different countries and ethnic backgrounds, and the clinical and immunological phenotype is remarkably consistent. Although females can be carriers, there are no known males harboring silent MAGT1 mutations, perhaps indicating that the disease is 100% penetrant on the cellular level with variable age of apparent symptom onset (34). As noted above, patients showed a decrease in CD4+ T cells, mild immunodeficiency, and a severe loss of immunity against EBV. Accompanying lab findings include a reduction in activation markers such as CD25, and a reduced level of NKG2D on CD8+ T cells and natural killer cells. Yet, how these features fit together to explain a mechanism of disease was a puzzle.
Genotype variation and bioinformatics
Once the genome has been interrogated by CGH arrays or NGS, variations can be defined by comparing the patient sequence to the standard reference assembly as well as family members (5, 6, 15, 25, 51, 58–60). As alluded to above, a large number of SNVs will be identified which leads to the problem of which variants are potentially disease-causing. Exonic sequences will reveal alterations in the coding sequences of particular genes. WGS can also be used to detect alterations in non-coding regions in human disease (58–61). However, the definition of disease-causing alterations in non-coding regions is still in its infancy because it involves casting a searchlight across immense stretches of the genome with complicated regulatory structures that are still only dimly perceived. Even within coding regions, analysis is challenging because there are thousands of variants, many private, to be sifted through (14, 26). The problem was documented in the first analyses of the thousand exome project (26, 30). Even considering only severe LOF variants such as nonsense, indels, and splice site-disrupting SNVs, it was estimated that genomes from healthy humans could contain up to 100 LOF variants of which 20 would be homozygous (29). A similar analysis of missense mutations from this data set showed that 281–515 missense substitutions were predicted to be protein-damaging and LOF (see below) and between 40 and 85 would be homozygous (30). Further compounding the problem was the fact that a shockingly large number (hundreds) of variants in the human disease mutation database were found in normal people and that the original publication of these variants had no biochemical validation of disease involvement (30). Thus, NGS interpretation is prone to false-positive disease correlations (26, 30). To surmount these problems, recommendations for variant interpretation emphasize weighing multiple lines of evidence. When available, statistical arguments across many families is certainly strongly supportive, but difficult to deploy (26). But this is difficult to deploy. Most disease phenotypes are very rare and it is difficult, without the guidance of an identified gene, to assemble a well-defined patient group. For more common conditions, general and less specific phenotypes are binned together (e.g., common variable immunodeficiency) and later prove to have multiple genes involved, thereby defying a uniform approach (62, 63). Indeed, in defining over a dozen new diseases, our program has not been able to use a statistical study design.
The approaches we prefer to use are: 1) judicious choice of candidate variants by a stratification process (51, 58, 60); and 2) biochemical and molecular validation. These have the virtues of proven success and providing a molecular understanding of disease that benefits treatment and prognosis. There are a variety of software tools that are continuously evolving to stratify gene candidates. We will discuss the guiding principles for variant evaluation and will leave the reader to consult with bioinformatics experts for specific recommendations for genomics projects. The stratification of candidate variants is essentially a guesstimate of the probability that a given variant is disease-causing generally using a population of affecteds that is too small to allow a statistical correlation. First, it is crucial to ensure that the variant is not a sequencing or annotation error (51). NGS technologies are high-throughput approaches designed to generate bulk but not complete sequence information and a sequence variant can be most accurately described if it has been redundantly sequenced in the dataset, usually expressed as the read number at that site or “fold coverage.” 30 is a good number; 100 is better, but beyond that is probably unnecessary (51). Medium-sized indels and CNVs can be hard to judge with confidence. Large CNVs or rearrangements will require CGH arrays or chromosome banding analyses. Even with good coverage, once a variant becomes highly suspicious, it should be confirmed by conventional Sanger sequencing on a separate sample from the patient.
Another initial consideration is whether or not the mutated gene is expressed in cell types with an abnormal phenotype relevant to the disease. It is generally safe to assume that the altered gene must be expressed in the affected cells, especially if there is a strong cellular phenotype. Further filtering of gene candidates can then be prioritized considering the presumptive mode of inheritance. Depending on the clarity of the clinical phenotype, the pedigree provides the roadmap for navigating the mutant allele among affecteds and unaffecteds (58). Extensive NGS has shown that nucleotide variation is not uniformly distributed in the genome and a variant further gains significance if it falls in a conserved region of the genome, indicating that the function of that region does not tolerate much variation (20). Conversely, certain types of genes (a random example of this would be olfactory receptors) frequently harbor LOF mutations and are an unlikely cause of immune disease (58). Also important is whether the MAF shows that the variant is unique or very rare in the variant databases (ExAC, dbSNP, etc.). There is no precise cutoff but < 0.1% could be allowed since the reported SNV universe is so vast and covers so many different groups of normal and diseased individuals that there is a chance that the variant would have been reported. For example, heterozygous carriers of AR mutant alleles will be present in “normal” control populations because they will have no disease. Nonetheless, a high MAF decreases the chance a variant is disease-causing. Together these strategies can drastically shrink the list of possibilities from thousands to less than 100 (47, 51, 56, 58–60).
The common assumption for a disease-causing variant is that it will have a severe deleterious effect on gene function (26, 31, 51, 58, 64–67). Largely due to the wide use of exome sequencing, private variants with big effects have been located mainly in protein-coding regions of the genome. These currently account for about 85% of all Mendelian disease variants (63). Generally, nonsense mutations together with deletions (large > small) and splice variants, especially if they are frame-shifting, are more likely to be deleterious then missense mutations (26, 29). Base changes that simply substitute an alternate codon for the same amino acid, termed synonymous, are usually ignored. The problem then becomes how do you tell if a non-synonymous change is likely to be deleterious just by looking at it. Fortunately, ingenious software has been developed to consider various parameters of the non-synonymous change to determine if it is likely to be damaging to the protein (64–67). These include whether the mutation is in an evolutionarily conserved residue, whether it is a marked chemical change, e.g., substituting an acidic residue for a basic residue, whether it affects buried residues, whether it induces a steric clash between amino acids, and how it affects surface electrostatics, among other considerations. Generally, if the functional or conserved parts of the protein structure are drastically compromised, then it is judged to be likely damaging to the protein. Widely used examples of these programs, which are constantly evolving, are SIFT, PROVEAN, CUPSAT, PolyPhen2, MutPred, MutationTaster, GERP and PhyloP, and CADD and variations thereof (64–67). Studies have shown that these programs have great value in prioritizing candidate disease variants (64, 66). Also facilitating disease variant identification are databases that aggregate reference exome data (e.g., http://exac.broadinstitute.org/). These techniques can reduce the candidate variant pool usually to one or a handful of possibilities.
The search for a pathogenic variant in XMEN disease was simplified to a great extent by the hypothesis that the gene was X-linked based on the mother’s skewed X chromosome inactivation (32). However, when the variants were prioritized, no apparently protein-damaging candidate variant emerged that was present in both boys but heterozygous in the mother. The absence of a suitable variant raised a suspicion about the coverage of genes on the X chromosome by the sequencing analysis. In fact, the software was designed to exclude mismatches over 2 bp as erroneous sequences and would miss larger deletions because they would be consigned to the “junk pile” of sequences from the sequencing run. If such an exclusion had occurred, then there would be genes covered in the mother that had no coverage in the children. In fact, two genes met this criterion. When the reads were examined carefully, two non-overlapping partial sequences of the MAGT1 gene were found that were separated by a 10 bp gap. If this 10 bp region was removed from the X chromosome reference sequence and the boys’ sequences were reanalyzed, then a large number of sequences would be recovered from the software’s “junk pile” of discarded sequences, showing a 10 bp deletion in the genomic DNA of both boys (and heterozygous in the mother) that spanned the gap between sequences that had passed the quality control filter. Thus, by examining the “coverage” of genes, a problem with the software filters was detected and overcome. Further analysis of the deletion showed that it spanned an intron-exon junction causing a frameshift and premature stop codon in the protein (32). This variant was predicted by bioinformatics to be a deleterious mutation.
Biochemical validation
The critical final step in the journey and, unfortunately, often the longest and most labor-intensive, is validation (26, 68, 69). This is the bottleneck in genomics research that connects DNA sequencing and computational analysis to clinical application (Figure 2). This includes both making an argument for causality and developing a molecular understanding of the disease mechanism. If a strong cellular phenotype has been defined, then cellular knockouts or knockdowns using CRISPR, siRNA, or shRNA to decrease expression of the gene or transfection of the mutant allele into normal cells might yield data that the gene variant can recapitulate the cellular phenotype associated with disease (7, 8, 28, 32, 40, 68, 70). Also, introducing a normal gene version or knocking out a dominant variant might be sufficient to correct the abnormal phenotype in the patient cells (28, 32, 71). It is crucial to carry out biochemical investigations of the putative mutant protein since protein levels correlate poorly with mRNA in human cells (72). If a cellular phenotype is not apparent or the disease involves a developmental process, say, the differentiation of T cells, then more elaborate investigations are needed (26, 69, 73). Mouse models often reflect the cardinal features of human genetic diseases but are often incomplete (74). Moreover, they may not reflect complicated human genetic disease mechanisms such as haploinsufficiency (9). Researchers can also use emerging new technology to induce pluripotent stem cells (iPS) as a renewable source of cells to test gene variants. iPS can be differentiated into relevant cell populations in vitro, allowing gene experimentation in a physiological context (57, 68). These approaches can build the case that the variant is potentially disease-causing. The discoveries may then be ultimately confirmed by more patients and research as well as a corresponding phenotype in a relevant animal model (26, 68, 73).
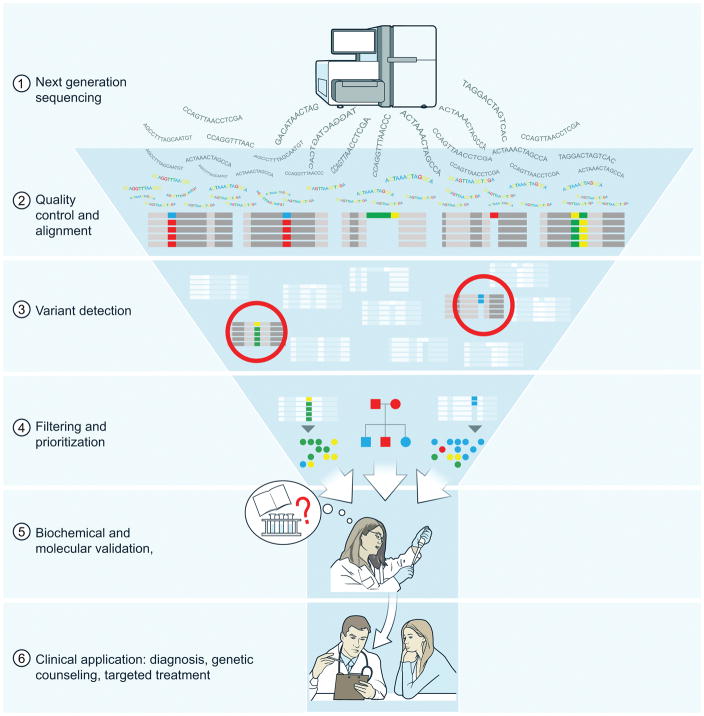
The bottleneck to genetic disease discovery is the biochemical and molecular validation of candidate disease variants obtained through next-generation DNA sequencing. Although computational methods can reduce and prioritize variants, further validation through direct experiments is required. The benefits of clinical application can only pertain once validation has been carried out.
Validation of the first XMEN mutation was guided by a predicted premature stop codon in exon 7 (out of 10), a situation that typically leads to nonsense-mediated decay of the mRNA and accounts for loss of protein in roughly 30% of genetic diseases (75). Indeed, in both affected boys, the mRNA was found to be depleted by polymerase chain reaction of cDNA, and a protein blot showed absence of the MAGT1 protein (32). A strong cellular phenotype in the form of defective TCR but not B cell receptor (BCR) signaling was observed in the patient cells and could be recapitulated by knocking down the MAGT1 protein in normal T cells. Furthermore, artificial expression of a normal MAGT1 gene transferred into patient T cells was able to correct the activation defect. Also, background literature on the gene indicated that it was a magnesium transporter, so Mg2+ levels and mobilization in immune cells were evaluated after stimulating T cells through the TCR. These investigations showed that there was a rapid TCR-gated Mg2+ flux that was required for a rapid and optimal Ca2+ flux. Further biochemical experiments revealed that the Mg2+ influx rapidly activated phospholipase C gamma 1 (PLCγ1) which cleaves phosphoinositide lipids into inositol triphosphate (IP3) and diacylglycerol (DAG) (32). The reduction of IP3 and DAG generation explained the blunted Ca2+ flux and impaired protein kinase C θ phosphorylation, respectively. Together, these defects short-circuited the induction of nuclear factors such as NFAT and NF-κB that control the transcriptional program underpinning normal T cell activation. Other pathways of TCR signaling involving p38 and ERK were Mg2+ independent, indicating an uncoordinated but not completely deficient molecular orchestration of signaling circuits needed for a healthy T cell response. Correspondingly, the patients have a relatively minor immunodeficiency. Experiments also showed that BCR activation of the orthologous enzyme PLCγ2, does not involve any Mg2+ flux, explaining why the number of B cells and their function in XMEN patients was the same as healthy subjects (32). Thus, the molecular validation of the MAGT1 gene was consistent with the patients’ cellular phenotype.
Determining the disease mechanism for a specific variant or the gene itself may be straightforward or may involve substantial investigation. This is the art of experimental immunology, creatively combining cellular, molecular, and biochemical analyses with an understanding of disease manifestations. The central guidepost is the patient phenotype as defined by astute clinicians. It is very helpful if a mouse model is available since many cell populations can be interrogated and well-established autoimmune disease models are available. Also, viruses, bacteria, and parasites can be used to test immune responses. If one can connect the mutant to well-studied molecular pathways, then there will be more reagents and fundamental knowledge to speed up the investigation. However, if the disease-implicating evidence from the genetics and the cellular phenotype is strong, then it would be a mistake to shy away from gene mutations that occur in little known pathways or “don’t make sense.” This may yield immunological insights with the greatest novelty and impact, but the investigative journey could be long and frustrating. Genomics is an unbiased exploration of genotype-phenotype interactions – so it has extraordinary potential to take the investigator into new uncharted territory.
The hallmark of XMEN disease is uncontrolled EBV infection that creates a strong susceptibility to B cell lymphoma even in childhood (33, 34). This selective pathogen susceptibility was difficult to explain on the basis of a relatively mild TCR signaling defect. Further analyses of antiviral mechanisms revealed a severe reduction of the NK cell activating receptor, NKG2D, which was observed in numerous XMEN patients who suffered uncontrolled EBV infection. Moreover, B cells infected with EBV showed increased expression of specific ligands that mark the cells for cytolytic destruction via NKG2D. With a deficit of NKG2D, these infected cells became invisible to anti-viral immunity and escaped elimination. MAGT1 deficiency reduced intracellular basal free Mg2+, which impaired proper surface expression of NKG2D. Remarkably, adding excess Mg2+ to XMEN lymphocytes reversed the intracellular deficiency, resulting in rescue of NKG2D surface expression and cytolytic function against EBV-infected targets (34).
If we stop for a moment and ponder our journey through the incredibly powerful new genomic technologies, we realize that human genetic variation in disease is a gold mine for insight into molecular function. The depredations of immune function caused by genetic mutations have the potential to instruct us to how the immune system operates. As pointed out above, careful work must be done to be sure that the variant under investigation isn’t “fool’s gold.” However, the full value of striking gold isn’t achieved until it is worked into a lustrous understanding of an immune mechanism. Currently in medical genetics, we believe that there is an overemphasis on generating DNA sequence from patients and insufficient emphasis on the biochemical work to understand the molecular and pathophysiological importance of gene variants that are detected. We have argued that if one assumes that 200 families with unknown genetic immunological diseases, for instance parent/child trios, will generate 200 terabytes of DNA sequence data and this will require 1–2 bioinformatics specialists to generate candidate variants, then it will likely require 10 to 12 biochemists and immunologists to biochemically validate the causative variants (69). However, many clinical sequencing groups have not prioritized biochemical efforts so that the mutant proteins can be convincingly validated or investigated in sufficient depth to understand the molecular mechanisms of disease and possibly identify therapeutic strategies. Hence, in-depth functional validation and biochemical studies are essential future goals for human genetic studies. Further, the study of the immune system has the unique and powerful advantage of being accessible for study and treatment, much more so than in cardiology, neurology, ophthalmology, etc. This level of effort will fashion the genetic “gold” first into amulets of understanding and then, hopefully, into better diagnosis and treatment.
Genetic etiology of disease and complex adaptive system theory
In the pre-NGS era of medical genomics, diseases were divided into Mendelian, meaning monogenic (or possibly digenic, but not more), and complex, meaning polygenic (13, 63, 76). As we pointed out above, even Mendelian diseases reflect the contribution of multiple genes. Conversely, NGS has revealed that genetic diseases heretofore thought to be polygenic – autism, mental retardation, and others, are actually heterogeneous collections of individuals with private, highly penetrant deleterious mutations (20, 77). Immunogeneticists have discovered that common variable immunodeficiency (CVID) can be explained in the same way (63). It is likely that rare, highly penetrant mutations may account for other immunological diseases including the common autoimmune conditions. This is where the nascent field of systems biology can play an influential role. By assembling the affected genes in a common phenotype into a regulatory network, systems analyses may be able to place new variants (private, highly deleterious and penetrant) into a logical framework of disease (78).
Understanding the effect of genes on cellular phenotype requires a model for understanding how the linear one-dimensional information in DNA generates a three-dimensional dynamic system. The protein product of a disease gene does not work in isolation but is part of a large interactive system (76). A useful concept for understanding genetic interactions is to view the cell as a complex adaptive system (CAS) (79). A CAS is a collection of diverse elements that self-organizes according to simple rules into a “system.” Although analogous to many different interactome models, a CAS focuses on defining features that enable the system to adapt to new and unpredictable circumstances. Modeling has shown that CAS can generate large intricate systems with spectacularly complex behavior that surpasses the action of individual parts in surprising and unexpected ways (80). Intuitively, this seems to be an appropriate way to model how molecules form the structures and systems of immune cells.
CAS are known to exhibit three key properties: robustness, adaptability, and “tipping.” Robustness refers to stability in the face of unpredictable perturbations, such as genetic alterations, through a network of interacting elements (81). Functionally speaking, robustness – the tendency of the system to remain stable – and adaptability – internal real-time changes (adaptations) to preserve function – are two sides of the same coin. To be clear, adaptability here does not refer to Darwinian adaptations through natural selection, but immediate adjustments in biochemical responses in the life of a single cell or organism to the effects of mutations under different cellular conditions. NGS has shown that individual human genomes tolerate a large number of private deleterious mutations in individuals that appear to be healthy (29, 30). How the CAS in different individuals can adapt to LOF or otherwise disease-causing changes to robustly preserve function is the key to understanding disease pathogenesis. Recent examples of adaptability to genetic loss or severe environmental stress on cells have been described (82, 83). In these instances, the random occurrence of new mutations and the unpredictability of pathogens and the environment, means that there can be no foreordained plan of adaptations to achieve robustness in the system. These same issues underlie any explanation of how penetrance and expressivity can vary so greatly even to the extent that relatives of patients harboring the same genetic defect can remain healthy (41). From the perspective of a CAS, the wide variation in the parts of the genetic system: gene families, allelic differences, polymorphisms and rare variants promotes rather than detracts from robustness. This diversity of components may permit the system to adapt in real time. For any given gene, the complexity of the system is built upon gene families and non-synonymous allelic differences that will create different protein homologues which could be further diversified by post-translational modifications to create a network of similar protein forms. Unlike the classical notion of “one gene-one protein” which implies a solitary gene function, the diversity of protein forms we now know can exist for any gene fits a CAS that uses different forms under distinct conditions of metabolism, invading pathogens, or immune processes to enable the system to operate most effectively.
In a similar vein, the interaction of a network of different genes, often referred to “modifiers”, could achieve robustness by allowing adaptability to genetic defects. This model is very different than modeling the cell as a machine or an electronic circuit where each part is specifically engineered to play a specific role under controlled operating conditions (conceptually the same as the one gene-one protein concept). Machines can be highly complicated but lack the diversity that is crucial to manifesting complex adaptive behavior. When unexpected severe circumstances arise, a machine breaks but a CAS adapts (79). The other CAS characteristic, “tipping”, refers to what happens when the various indirect parts begin to negatively synergize. Here the interconnectedness of the system destroys robustness. Similar to an icy interstate at night where drivers realize too late that they can’t control their cars and one after another slide into a disastrous pile up. Events like lymphoid cancers or apoptosis may reflect tipping of the cellular CAS. As the broad genetic landscape is expressed into the cell interactome, it will be fascinating to understand how the cellular CAS adapts to genetic and environmental perturbation to preserve health or “tip” into disease. Inbred mouse models may provide valuable insights into human disease by allowing experimental evaluation of different alleles and interacting genes in controlled systems (76).
Genetic changes affect the cellular CAS in ways that belie the traditional paradigm of Mendelian versus complex genetic diseases. Moreover, understanding how these primary determinants of disease have molecular effects on the south will also give great insights into disease phenotype (76). Sometimes a variety of different genes affect the same critical component of the system and adaptability fails. For example, a variety of mutant alleles of several receptor and cytokine genes that all interfere with production or response to interferon-γ, cause the clinical entity “Mendelian susceptibility to mycobacterial disease” (MSMD) (56). On the other hand, the same gene can account for two entirely different diseases. Job’s Syndrome, which features impaired Th17 differentiation, immunodeficiency, abnormally elevated IgE, and connective tissue abnormalities is caused by germline dominant-interfering STAT3 variants (84). By contrast, STAT3 heterozygous GOF variants have been identified in patients with lymphoproliferation and early-onset autoimmunity (85–87). A similar dichotomy has also been found in diseases related to STAT1 (88).
Although we have argued that the adaptability inherent in cells can create a continuum between Mendelian and complex disorders, the ends of the continuum can still be clearly defined. For Mendelian disorders, specific gene identification is achieved by each mutation being necessary and sufficient, within the bounds of penetrance and expressivity considerations, for the disease. Generally the mutations are deleterious to protein function, rare, and occur with a mode of inheritance pattern consistent with the disease phenotype (22, 31, 51). The mutation reveals the relevant biological pathway and explains the phenotype. By contrast, complex polygenic diseases, which could include multiple sclerosis, type I diabetes, and rheumatoid arthritis, etc., have disease variants that do not have the same properties as Mendelian disease variants. The associated variants in complex diseases are neither necessary nor sufficient, not rare, and often not in the protein-coding regions of the genome (12–14). Typically, they are noncoding and located in regulatory regions. Nevertheless, the definition of genes involved in Mendelian disorders will likely expose critical pathways that are involved in complex diseases (89). Classic studies have shown that the pathways detected in monogenic disorders, such as hypercholesterolemia, later prove to be valuable targets for pharmaceutical intervention for more common related abnormalities (90).
Clinical diagnosis and incidental genetic findings
The use of NGS for identifying gene variants has now started to be an accurate and cost-effective method that complements traditional molecular diagnostic tests in clinical genomics (91, 92). However, once variant implication in disease moves from the research laboratory into clinical medicine, then the DNA sequencing lab must adhere to the Clinical Laboratory Improvement Amendment (CLIA) requirements and other legal and ethical considerations pertain (93). In order to report a variant as the etiology of a disease, the lab must have a high degree of confidence in the variant assessment (91, 92). As the catalogue of pathogenic variants grows and bioinformatics tools improve, it will be increasingly possible to identify disease-causing variants accurately.
However, WES or WGS generate large amounts of data that extend beyond a specific set of gene candidates for immunological diseases and provide a wealth of genomic information that is potentially important for the health of the patient. Potential risk alleles, spanning a wide range of diseases, was found in every healthy individual in a reanalysis of data from the 1000 exome data set (94). For example, an NGS study carried out to discover a gene mutation causing an immune disease might reveal a breast cancer susceptibility allele. Thus, the medical genetics community has begun to focus on how to integrate WES/WGS into medical care by enabling the reporting to physicians of actionable variants (95). However, many issues regarding how to integrate this process into medical care have been raised (96). Databases of clinically important variants such as ClinVar and Online Mendelian Inheritance in Man (OMIM) will allow access to collected genomic information. This will require ongoing epidemiological analysis of variants at the level of the laboratory and hospital. It will also create a need for allied genetic counselors who can interpret the genomic information and help physicians provide appropriate diagnostic and prognostic information for the patients. The Center for Disease Control and Prevention (CDC) has established a working group for developing standards for the use of NGS in clinical diagnosis (97).
Targeted therapy, precision medicine, and common immune diseases
An important aspiration in the exploration of the genetic basis of immunological disease is that it would provide a molecular approach to new therapeutics. This has been termed precision medicine (98). In the pre-NGS era, bone marrow transplantation was the mainstay of treatment for genetic diseases of the immune system. We believe that the future of genomics will provide two types of new therapies: 1) direct molecular interventions based on the pathway of the mutant protein and 2) genome editing combined with lymphocyte or hematopoietic stem cell transplantation (HSCT). Several recent examples show how gene identification has led directly to new therapeutic concepts for immune diseases. Identification in a group of patients with atopy, immunodeficiency, autoimmunity, and neurocognitive deficits of LOF mutations in phosphoglucomutase 3 (PGM3), which is an essential enzyme for the production of UDP-GlcNAc is one such example (38, 99, 100). How the defective protein glycosylation selectively alters immunity is unknown, but addition of GlcNAc to cells from the PGM3 patients restored intracellular UDP-GlcNAc levels (38). Clinical studies are underway to determine if exogenous non-diabetogenic sugars could be used therapeutically in these patients to ameliorate the immune disorders (38). Although HSCT is life-saving, sugar therapy might be useful as a simple, safer, and inexpensive therapy, especially if the patient is not a good transplant candidate (100). Below we recount additional cases of etiological treatments derived from gene identification in XMEN, CHAI/LATAIE, and PASLI diseases.
After the initial discovery of XMEN disease, it wasn’t clear that a direct etiological treatment was possible. The major pathogenesis of XMEN disease appeared to be due to the failure to control a single pathogen, EBV. Magnesium is an obligatory element in all organisms and its utilization is tightly regulated (101). The most important biological form of magnesium is the divalent cation Mg2+, which is a cofactor for many metabolic reactions including all ATP-requiring kinase reactions, glycolysis, and nucleic acid enzymology. In addition to a defect in TCR signaling, MAGT1 deficiency also substantially decreases cytosolic free basal Mg2+ to less than 50% of normal. While studying the biochemical mechanism of reduced NKG2D expression, which results in uncontrolled EBV infection, it was determined that the decrease in free basal Mg2+ was the cause for reduced expression of NKG2D (33). Further experimentation revealed that the reduction of surface NKG2D was due to ubiquitinylation and degradation. Importantly, it was also found that the free basal Mg2+ in the cell was in equilibrium with extracellular Mg2+. This led to a series of experiments demonstrating that culturing T or NK cells from XMEN patients in supraphysiological concentrations of Mg2+ (> 1 mM), restored free basal Mg2+ to normal levels and rescued the surface expression and function of NKG2D (33). This then provided the rationale for therapeutic Mg2+ supplementation for the XMEN patients. Indeed, chronic Mg2+ supplementation resulted in a significant decrease in the fraction of EBV-infected B cells and blood levels of EBV (33, 34). The hope is that Mg2+ supplementation, a treatment suggested directly by the gene identity, will be a simple, low-cost way to improve anti-viral immunity, suppress EBV, and avoid lymphoma in XMEN patients and perhaps other patients with chronic active EBV.
CHAI and LATAIE disease
In a cohort of patients with severe immune dysregulation and solid organ lymphocytic infiltration, we identified one subset of patients with an AD mode of inheritance and another subset with an AR mode of inheritance. Molecular investigation revealed heterozygous, LOF mutations in CTLA4 in the patients with AD disease, which we named “CTLA-4 haploinsufficiency with autoimmune infiltration” (CHAI) disease (9, 46). In patients that looked very similar but lacked CTLA4 gene mutations, we detected biallelic (homozygous or compound heterozygous) LOF mutations in the lipopolysaccharide–responsive vesicle trafficking, beach- and anchor-containing (LRBA) gene causing recessive disease, which we termed “LRBA deficiency with autoantibodies, Treg defects, autoimmune infiltration, and enteropathy” (LATAIE) (102–104). Both subsets of patients share the predominant characteristics of autoimmune cytopenias, lymphocytic infiltration of multiple non-lymphoid organs, lymphadenopathy/splenomegaly, and hypogammaglobulinemia. There was variable expressivity in clinical presentation, in which the severity of the mutation appears to play a role. The clinical phenotype ranged from severe, life-threatening interstitial lung disease, enteropathy, autoimmune cytopenias, and CVID to an ALPS-like phenotype (i.e., autoimmune cytopenias with lymphadenopathy and/or splenomegaly) with no other autoimmune manifestations. The fact that a very similar clinical picture resulted from mutations in two different genes with two different modes of inheritance raised the critical question of how the two molecular pathways intersected.
CTLA-4 is a critical immune tolerance checkpoint molecule. It is a cell surface receptor protein on activated T lymphocytes that binds and controls access to the CD80 and CD86 co-stimulatory molecules that are expressed on the surface of antigen presenting cells (APCs) with which they are interacting. By constraining co-stimulation, it acts as a checkpoint by down-regulating T cell responses. The importance of this checkpoint control is demonstrated by Ctla4 homozygous knockout mice that develop fatal, multiorgan lymphocytic infiltration and destruction (105, 106). Almost a carbon copy, patients with CHAI disease develop extensive and destructive lymphocytic infiltrates in multiple organs, mainly the gut, lungs, and/or brain (9, 46). However, discovering the gene causing CHAI disease was complicated by two factors. First, it resulted from an AD-HI mode of inheritance rather than AR. Second, the penetrance was incomplete. WES was performed with DNA from affected and unaffected family members and analyzed using an AD model. All CHAI individuals had the CTLA4 mutation, but not all mutation-positive individuals in the family had disease even though all mutation-positive individuals had reduced CTLA-4 protein. Only after finding multiple families with mutations and biochemically demonstrating that the mutations impaired CTLA-4 protein function or expression was CTLA4 concluded to be the disease causative gene.
In addition to conventional T cells, CTLA-4 is a key inhibitory receptor that is constitutively expressed on regulatory T cells (Treg). In certain contexts, CTLA-4 is crucial for proper Treg suppressive function and limits costimulation by multiple molecular mechanisms, e.g., by negative signaling, by competing with the costimulatory molecule CD28 for the shared ligands CD80 and CD86, or by removing these ligands from APCs through trans-endocytosis (107, 108). Consistent with a failure of these functions of CTLA-4, we and others found that patient T cells were hyper-proliferative and their Tregs had an abnormal phenotype and were specifically impaired in suppressive function (9, 46). Surprisingly, several patients had low antibody levels rather than high, as would have been expected from the CTLA-4 null mice, thus illustrating the potential limitations of using mouse models for predicting human disease phenotype (106). CHAI patients also had elevated numbers of anergic or exhausted CD21lo B cells that are putatively autoreactive since they are often enriched for self-reactive BCRs and are found at increased levels in autoantibody-mediated autoimmune diseases (109, 110). Overall, the clinical presentation of the patients revealed a critical role for CTLA-4 in regulating both B and T cell homeostasis.
These findings still left unresolved the pathogenesis of AR LATAIE disease. LRBA mutations were first discovered to be disease-causative in 2012 by genetic linkage analysis of several consanguineous families with hypogammaglobulinemia and autoimmunity, followed by sequencing of positional candidate genes (104). Shortly thereafter, other patients with LRBA deficiency were identified by NGS (102, 103). All mutations caused loss of LRBA protein; however, the molecular function of LRBA or its role in the immune system were initially unknown. A study of the biochemical basis of LATAIE disease not only revealed both the function of LRBA and why LATAIE patients resembled CHAI patients, but also revealed targeted therapies (111). LATAIE patients with life-threatening interstitial lung disease, which was refractory to conventional immunosuppressants, were treated with abatacept (a CTLA4-immunoglobulin fusion protein) for several years before their genetic etiology was known. With this treatment, the patients’ autoimmune conditions and lung function dramatically improved. A clue to the connection between this remarkable treatment effect and LRBA mutations was the tantalizing homology of LRBA to vesicle trafficking molecules (112–114). This link led to the hypothesis that LRBA plays a key role in controlling protein trafficking of CTLA-4, which is known to be tightly regulated through recycling vesicles (111, 115). Consistent with this hypothesis, loss of LRBA, both in LATAIE patients and other experimental settings, was found to cause a profound post-translational loss of CTLA-4 protein.
CTLA-4 is stored in intracellular vesicles and cycles, after T cell stimulation, to the cell surface where it must reside to perform its inhibitory function (115, 116). LRBA was shown to bind to CTLA-4 and, in its absence, CTLA-4 transited to lysosomes and was rapidly degraded. Inhibition of lysosomes with the drug chloroquine could rescue the loss of CTLA-4 in LRBA-deficient cells. Thus, LRBA appears to protect CTLA-4 from lysosomal degradation and therefore helps maintain intracellular pools of CTLA-4 for rapid mobilization to the cell surface for inhibitory function. Understanding the biochemical link between LRBA and CTLA-4 also explained why abatacept was such an effective treatment for the LRBA-deficient patients. By traveling through the blood to sites of T cell activation and providing a therapeutic cap on costimulation by blocking CD80 and CD86, abatacept could make up for the loss of endogenous CTLA-4 on the surface of conventional and regulatory T cells. Altogether, the discovery of CHAI and LATAIE, both autoimmune diseases resulting from CTLA-4 deficiency, emphasizes how critical this molecule is for immune tolerance and homeostasis, since reduction of CTLA-4 or loss of a single allele can throw the immune system out of balance. It also provides a vivid illustration of how research on HI genetic disorders can lead to a greater understanding of immunologic processes.
Uncovering the genetic and molecular etiology of CHAI and LATAIE disease provided novel insights into immune regulation as well as a rational basis for precision medicine treatments for the diseases. The lymphoproliferation and autoimmunity in CHAI and LATAIE diseases both stem from a loss of CTLA-4. Thus, treatment with abatacept as a CTLA-4 replacement therapy, clinically demonstrated to be effective in LATAIE disease, could also be effective for CHAI disease and perhaps other autoimmune diseases involving insufficient CTLA-4. Additionally, LRBA was found to regulate the turnover and degradation of CTLA-4 in lysosomes. Since the lysosomal inhibitor chloroquine could prevent lysosomal loss of CTLA-4 in LRBA-deficient cells, it or its pharmacologically safer alternative hydroxychloroquine could also be effective in treating CHAI or LATAIE diseases by boosting the levels of CTLA-4 protein. Interestingly, hydroxychloroquine is already used as a therapy for rheumatoid arthritis and lupus (117–119), so it is worth investigating whether its efficacy may be partly attributed to an augmentation of CTLA-4. If it were proven to be effective, hydroxychloroquine would be a very inexpensive alternative to abatacept. In summary, knowing not only the genes, but their biochemical and molecular roles in the cell, suggest molecular theories on which to base precision medicines that can have dramatic efficacy for previously undefined diseases.
PASLI disease
Multiple patients were identified who all suffered from an AD immunodeficiency syndrome with recurrent sinopulmonary infections, immunoglobulin synthesis defects, predisposition to EBV and/or CMV viremia, and lymphoproliferative disease (27, 28, 120–122). NGS of such patients revealed a first group of these individuals that carry mutations in the PIK3CD gene encoding the leukocyte-enriched p110δ subunit of phosphatidylinositol-4′,5′-bisphosphate 3′-kinase (PI3K) (27, 28, 120–122). In unrelated families, one of three germline, heterozygous mutations resulting in amino acid substitutions N334K, E525K, or E1021K, were identified. Intriguingly, the former two substitutions align precisely with hyperactivating N345K and E545K substitutions in p110α, resulting from somatic mutations in tumor cells (27, 28). Molecularly, these two changes have been shown to disrupt inhibitory contacts between the regulatory p85α protein and the catalytic p110 proteins of the PI3K complex will Each of these mutations would be expected to augment enzymatic activity. This would explain the dominance of the heterozygous mutant allele. The recurrence of such mutations strongly suggest that they reveal amino acid vulnerabilities in enzyme activity regulation. We named this specific constellation of disease features associated with this genetic etiology p110δ-Activating mutations causing Senescence, Lymphadenopathy, and Immunodeficiency (PASLI, the disease has also been called Activated PI3K-δ Syndrome, or APDS) (27, 28). Since the initial descriptions, approximately 100–150 patients have been identified with PIK3CD mutations causing PASLI disease, and the initial list of 3 mutation sites has been expanded to mutations in 5 DNA sites that cause specific amino acid substitutions that increase enzyme activity. The prevalence of heterozygous, GOF mutations in PIK3CD suggests that p110δ-hyperactivating substitutions represent a significant contributor to disease within the population of immunodeficient lymphoproliferative individuals.
Shortly after discovery of heterozygous PIK3CD mutations, a second set of patients with a similar clinical phenotype, i.e., PASLI disease, were shown to harbor heterozygous splice site mutations in the ubiquitously expressed PIK3R1 gene (39, 40). PIK3R1 encodes the binding partner for p110δ called p85α that ensures stability, localization, and regulation of the p110δ protein. Notably, all the patients (approximately 30–40 individuals identified to date) shared an identical splice site mutation that results in skipping of exon 11 and production of an in-frame transcript encoding a protein lacking important residues in the inter-SH2 domain of p85α. Loss of these residues alters binding of the p85α regulatory subunit to the p110δ catalytic subunit such the former still guides stability and localization but cannot regulate p110δ enzyme activity, resulting in PI3K hyperactivation. Given the similar end result in molecular effect, i.e., hyperactivation of PI3K due to unrestrained p110δ enzyme activity, it is apparent why the clinical and cellular phenotypes within the immune system would be similar in patients with p110δ and p85α alterations. However, unlike p110δ, p85α expression is not restricted to the immune system. Despite ubiquitous p85α expression, these patients with PIK3R1 mutations do not show dramatic non-immune phenotypes due to p110α or p110β hyperactivation. This remains an active area of investigation and may shed light on unique features of the association between p85α and p110δ (as opposed to p110α or p110β). Patients with PIK3CD or PIK3R1 mutations can be referred to as PASLI-CD or PASLI-R1, respectively.
PI3Ks are a family of crucial signaling enzymes that transduce signals from tyrosine kinase receptors and G protein-coupled receptors by phosphorylating the hydroxyl group of the 3′ position of the inositol ring of phosphatidylinositol. This leads to the generation of phosphatidylinositol 3-phosphate (PI(3)P), phosphatidylinositol (3,4)-bisphosphate (PI(3,4)P2), and phosphatidylinositol (3,4,5)-trisphosphate (PI(3,4,5)P3). The last of these products, typically formed in the inner leaflet of the plasma membrane, serves as a docking site for phosphoinositide-binding protein domains such as the pleckstrin homology (PH) domain. There are two types of class I PI3K molecules that are activated by different stimuli, and these have the same heterodimeric structure comprising one 110 kD enzymatic subunit and a regulatory subunit that can vary in size. The class IA PI3K molecules, which include p110δ and p85α are activated by receptor tyrosine kinases to phosphorylate phosphatidylinositol (4,5)-bisphosphate (PI(4,5)P2) at the 3-position, generating the PI(3,4,5)P (123). The generation of PI(3,4,5)P3 at the membrane initiates signaling by attracting the PH domain-containing proteins, including phosphoinositide-dependent kinase-1 (PDK1) and protein kinase B (PKB), also known as AKT. These stimulate further pathways downstream including the mammalian target of rapamycin (mTOR) kinase, which promotes cell growth, proliferation, and survival. Hence, activating mutations in class IA PI3K genes cause lymphoproliferation through the well-known proliferative mTOR pathway, but why this is associated with immunodeficiency loomed large as a key to understanding the pathogenesis and devising a new way to treat disease.
Investigation at the cellular level revealed that PI3K and mTOR hyperactivation in PASLI patients created imbalances in the subsets of T and B lymphocytes. On the T cell side, PASLI patients show a marked reduction in naïve T cells and a corresponding increase in effector-type T cell subsets that have become replicatively and functionally senescent with short telomeres and expression of the senescence marker CD57 on CD8+ T cells (28). Increased glucose uptake in the diseased T cells betrayed metabolic changes driven by PI3K/mTOR signaling that contributed to precocious effector cell maturation and led to most T cells converting into terminally differentiated cells that failed to function adequately (28, 40). On the B cell side, PASLI patients show defective B cell development with a preponderance of CD10+ transitional B cells and reduced frequency of CD27+ memory B cells. Functionally, patient B cells fail to properly secrete class switched immunoglobulins, particularly those specific to polysaccharide antigens. By histological analysis, PASLI patients have prominent germinal centers that lack a mantle zone (28). Thus, putting the cell surface signaling in overdrive caused the lymphocytes to differentiate abnormally.
The knowledge gained by the genetic and biochemical characterization of these patients immediately provided new therapeutic concepts. Fortunately, the mTOR kinase downstream of PI3K-AKT has been successfully targeted in vivo by the FDA-approved immunosuppressant rapamycin. The defects in PASLI disease provided a clear rationale for using PI3K/mTOR inhibitors to treat this disorder. Indeed, in an initial evaluation of the efficacy of rapamycin therapy in one patient, the data suggest that blocking this intrinsic drive toward effector differentiation at least partially restores the balance of naïve, memory, and effector CD8 T cells and markedly improves lymphadenopathy and splenomegaly (28). A larger cohort of patients treated with rapamycin are being studied (G. Uzel, personal communication). Thus, a counterintuitive therapeutic – treating an immunodeficiency with an immunosuppressant – becomes a rational targeted approach once the biochemical mechanism is clear.
Although rapamycin therapy holds great promise for an immediately useful treatment of PASLI disease, mTOR is only one pathway that is stimulated by activated AKT. The others, including NF-κB, GSK3, FOXO, and MDM2, will not be directly affected by rapamycin, so the effects of hyperactive PI3K would not be expected to be fully reversed. Consequently, the ideal therapy would be to directly and specifically target p110δ with an inhibitor to restrain activity to the normal range in PASLI patients. Specific targeting of PI3K isoforms has been the subject of intense research in the cancer field due to the high frequency with which this pathway is upregulated by direct mutation, gene amplification, or upstream receptor activation (124). Indeed, a p110δ-specific inhibitor called Idelalisib (formerly CAL101 or GS1101) has shown promise as a therapy for chronic lymphocytic leukemia (CLL) and is now FDA approved for use in combination with rituximab for relapsed CLL (125, 126). We anticipate that directly inhibiting the source of augmented signaling in PASLI patients will maximize clinical benefit by enabling production of new B and T lymphocytes that escape the PI3K-driven development and differentiation defects.
Disease summary
The distinguishing characteristics of XMEN, PASLI, and CHAI/LATAIE disease are listed in Table 1 below.
Table 1
CVID | ALPS | CAEBV | XMEN | PASLI | CHAI/LATAIE | |
---|---|---|---|---|---|---|
Sinopulmonary infections | Yes | Yes, sometimes with bronchiectasis | Yes, if hypogamm | |||
Ig defects | Yes | High IgM | Often hypogamm | |||
Lymphoproliferative disease | Yes | Yes | Yes | |||
Viremia | Variable | No | EBV | EBV | Often CMV and/or EBV | |
EBV+ B cell lymphoma risk | No | No | Yes | Yes | Yes | |
Lymphocyte tissue infiltration | No | No | No | No | Yes Mucosal nodules, sometimes obstructive | Tissue infiltration |
Expanded cell population | Variable | CD3+CD4/8−(B220+) | CD3+CD8+ | CD3+CD8+ (low CD4:CD8) CD8+NKG2D− | CD3+CD8+ CD3+CCR7− CD8+CD57+ CD20+CD10+ | More memory T cells and CD21lo B cells |
Disease gene product | Variable | FAS, FASL, caspase 10, N-Ras | Variable | MAGT1 | p110δ or p85α | CTLA4 or LRBA |
Conclusions
With the dramatic drop in the cost of NGS, our data banks are bulging to the breaking point with DNA sequences. It is clear that bioinformatics, while enormously powerful, is not adequate on its own to accurately assess the medical significance of most new gene variants. This requires biochemical and molecular validation, which is slow, expensive, and labor intensive (Figure 2). However, our hope is that we have made the case in this essay that investing the time and resources for the appropriate biochemical investigation of gene variants yields important fundamental insights into immunology as well as new, targeted therapies for genetic diseases. We believe that technological advances provide an optimistic outlook for understanding the genetics of immune disorders. NGS and other molecular technologies have greatly expedited the identification of disease-causing variants. As of February 2015 in Online Mendelian Inheritance in Man, 2,937 genes have been associated with 4,163 Mendelian phenotypes, but the genes underlying ~50% of all known Mendelian phenotypes are still undiscovered and an untold number of additional Mendelian phenotypes have yet to be defined. We have found that an integrative approach to genomics with biochemical, molecular, and cellular examination of the phenotype defined in the clinic is most effective to understand pathways in the healthy and diseased immune system. We have provided examples of how causative gene identification in human disorders is already leading to unexpected new therapeutic interventions with less cost and morbidity than traditional HSCT. Another exciting frontier is the development of new technologies in genome engineering that could be used to directly correct the DNA lesion in patient cells. This will be more readily deployable in immunology and other hematopoietic disorders than for solid organ diseases. If the major effect of the gene defect is in cells of hematopoietic origin, then severe defects can be potentially corrected with genome editing of hematopoietic stem cells that could be used for autologous HSCT. If the defect is somewhat less severe, then alternative strategies such as correction of somatic cells, such as T cells, followed by re-infusion of the altered cells could be employed.
The ultimate success of clinical genomics will require further integration of knowledge at the molecular and clinical levels longitudinally. Also, well-defined genetic entities can help defining the prognosis of immunological diseases, the response to medical intervention, and appropriate genetic counseling. This will require organizational efforts on the clinical side including understanding the sociology of interaction with the medical delivery system, electronic medical records that can be integrated with genomics and medical research, and incentivization of medical professionals to record phenotypic features according to the pathophysiology of the disease. At some point in the future, medical genetics may cease to be an independent specialty and evolve into an integrated part of medical practice.
Acknowledgments
We apologize to colleagues whose work we could not cite or could only superficially discuss due to space limitation. We thank our clinical colleagues Gulbu Uzel, Michael Jordan, and Helen Matthews. We also thank Helen Su and Morgan Similuk for critically reading the manuscript and Ryan Kissinger for figure preparation. We thank Bill Paul for his leadership and enthusiastic support of this research program. This work was supported by the Intramural Research Program of the NIAID, NIH.
References
Full text links
Read article at publisher's site: https://doi.org/10.1146/annurev-immunol-041015-055620
Read article for free, from open access legal sources, via Unpaywall:
https://europepmc.org/articles/pmc5736009?pdf=render
Citations & impact
Impact metrics
Citations of article over time
Alternative metrics
Smart citations by scite.ai
Explore citation contexts and check if this article has been
supported or disputed.
https://scite.ai/reports/10.1146/annurev-immunol-041015-055620
Article citations
Cross-population applications of genomics to understand the risk of multifactorial traits involving inflammation and immunity.
Camb Prism Precis Med, 2:e3, 31 Jan 2024
Cited by: 1 article | PMID: 38549844 | PMCID: PMC10953767
Review Free full text in Europe PMC
Immune Diseases: Challenges, Hopes and Recent Achievements.
Pharmaceuticals (Basel), 17(1):97, 11 Jan 2024
Cited by: 0 articles | PMID: 38256930 | PMCID: PMC10821122
Effect of NLRP3 inflammasome genes polymorphism on disease susceptibility and response to TNF-α inhibitors in Iraqi patients with rheumatoid arthritis.
Heliyon, 9(6):e16814, 01 Jun 2023
Cited by: 5 articles | PMID: 37332933 | PMCID: PMC10275785
Integrative multi-omics approaches to explore immune cell functions: Challenges and opportunities.
iScience, 26(4):106359, 09 Mar 2023
Cited by: 7 articles | PMID: 37009227 | PMCID: PMC10060681
Review Free full text in Europe PMC
An Updated Review on MSMD Research Globally and A Literature Review on the Molecular Findings, Clinical Manifestations, and Treatment Approaches in China.
Front Immunol, 13:926781, 18 Jul 2022
Cited by: 4 articles | PMID: 36569938 | PMCID: PMC9774035
Review Free full text in Europe PMC
Go to all (32) article citations
Similar Articles
To arrive at the top five similar articles we use a word-weighted algorithm to compare words from the Title and Abstract of each citation.
CHAI and LATAIE: new genetic diseases of CTLA-4 checkpoint insufficiency.
Blood, 128(8):1037-1042, 14 Jul 2016
Cited by: 70 articles | PMID: 27418640 | PMCID: PMC5000841
Review Free full text in Europe PMC
An Update on XMEN Disease.
J Clin Immunol, 40(5):671-681, 26 May 2020
Cited by: 35 articles | PMID: 32451662 | PMCID: PMC7369250
Review Free full text in Europe PMC
The role of MAGT1 in genetic syndromes.
Magnes Res, 28(2):46-55, 01 Jun 2015
Cited by: 5 articles | PMID: 26422833
Review
Defective glycosylation and multisystem abnormalities characterize the primary immunodeficiency XMEN disease.
J Clin Invest, 130(1):507-522, 01 Jan 2020
Cited by: 45 articles | PMID: 31714901 | PMCID: PMC6934229
Funding
Funders who supported this work.
Intramural NIH HHS (1)
Grant ID: Z01 AI000565-11