Abstract
Free full text

Metabolic pathways of lung inflammation revealed by high-resolution metabolomics (HRM) of H1N1 influenza virus infection in mice
Abstract
Influenza is a significant health concern worldwide. Viral infection induces local and systemic activation of the immune system causing attendant changes in metabolism. High-resolution metabolomics (HRM) uses advanced mass spectrometry and computational methods to measure thousands of metabolites inclusive of most metabolic pathways. We used HRM to identify metabolic pathways and clusters of association related to inflammatory cytokines in lungs of mice with H1N1 influenza virus infection. Infected mice showed progressive weight loss, decreased lung function, and severe lung inflammation with elevated cytokines [interleukin (IL)-1β, IL-6, IL-10, tumor necrosis factor (TNF)-α, and interferon (IFN)-γ] and increased oxidative stress via cysteine oxidation. HRM showed prominent effects of influenza virus infection on tryptophan and other amino acids, and widespread effects on pathways including purines, pyrimidines, fatty acids, and glycerophospholipids. A metabolome-wide association study (MWAS) of the aforementioned inflammatory cytokines was used to determine the relationship of metabolic responses to inflammation during infection. This cytokine-MWAS (cMWAS) showed that metabolic associations consisted of distinct and shared clusters of 396 metabolites highly correlated with inflammatory cytokines. Strong negative associations of selected glycosphingolipid, linoleate, and tryptophan metabolites with IFN-γ contrasted strong positive associations of glycosphingolipid and bile acid metabolites with IL-1β, TNF-α, and IL-10. Anti-inflammatory cytokine IL-10 had strong positive associations with vitamin D, purine, and vitamin E metabolism. The detailed metabolic interactions with cytokines indicate that targeted metabolic interventions may be useful during life-threatening crises related to severe acute infection and inflammation.
influenza virus infections continue to cause annual epidemics with high morbidity and mortality in humans and animals. Infection by the H1N1 virus that caused the 2009 pandemic induces oxidative stress and stimulates pro-inflammatory signaling pathways, which determine disease severity and outcomes (5, 16, 38, 50). Major cellular redox control systems dependent upon thioredoxin (Trx)-1 and glutathione were disrupted by infection of mice with H1N1 2009 pandemic virus (16). Influenza virus infection causes endocrine and neurochemical changes (12) and has systemic effects on multiple organs at various times following infection. Oxidative stress signaling sequentially causes translocation of Trx1 to nuclei, enhanced a transcriptional factor, nuclear factor (NF)-κB activation, and excessive production of inflammatory cytokines, interleukin (IL)-1, IL-6, and tumor necrosis factor (TNF)-α (16). Previous studies have identified interactions and molecular markers in the host immune responses to viral infection using proteomics and transcriptomics approaches (4, 31, 32, 42, 59, 63). Such knowledge of the molecular and cellular signaling events provide an understanding of the host response that contributes to disease severity and mortality.
Improved understanding of host metabolic responses to infection could provide the basis for metabolic support to ameliorate life-threatening effects. A recent study by Cui et al. (8) showed that more than 100 metabolites were differentially expressed in serum, lung and bronchoalveolar lavage fluid from influenza virus infection in a mouse model; they noted that many of these metabolites were associated with regulation of pulmonary surfactant system. Thus, in principle, maintenance of surfactant could provide a protective therapeutic strategy. Another recent study reported metabolic pathways associated with obesity and influenza virus infection (44). Obese mice induced by high-fat diet and genetic modification showed greater mortality, lung inflammatory responses, and excess lung damage in response to influenza virus infection compared with lean control mice. During infection, distinct metabolic disruptions in lung tissue and urine were detected via fatty acid, phospholipids, and nucleotides (44, 56). These studies have established the utility of metabolomics to identify metabolic changes associated with influenza and health risks, which could be used to identify other potential therapeutic targets and also developed for real-time evaluation of the severity of response.
High-resolution metabolomics (HRM) (7, 17–19, 57) can provide detailed metabolic information of the dynamics of influenza virus and host interaction at molecular levels in cellular metabolism. In the present study, we used HRM analysis of lung tissue samples from mice infected with H1N1 influenza virus to test for correlations of metabolites and metabolic pathways with lung cytokine levels. The analyses followed a workflow in which significant metabolites were identified by HRM [liquid chromatography (LC) with ultra-high resolution mass spectrometry (MS)] and partial least squares-discriminant analysis (PLS-DA). Top metabolic features were then annotated and mapped to metabolic pathways. Results show that influenza virus infection significantly alters lung tissue metabolites in amino acids, purine, pyrimidine, and glycophospholipid pathways. We then performed a metabolome-wide association study (MWAS) with inflammatory cytokines, which we term cytokine-MWAS (cMWAS), to identify specific metabolic associations with cytokine abundances. The results show an array of both highly selective and overlapping metabolic associations with influenza virus-responsive cytokines, indicating that targeted metabolic interventions within these loci may be useful to improve host response after influenza virus infection.
MATERIALS AND METHODS
Influenza Virus Infection of Mice
Pandemic 2009 H1N1 influenza virus (A/California/04/2009) was originally provided by Dr. Richard Webby, grown in 11-day-old embryonated chicken eggs, harvested, titered, and stored at −80°C until use. The infection dose of 50% lethality (LD50) was determined by infecting mice (C57BL/6, Jackson Laboratory) with serial dilutions of virus. C57BL/6 mice (4–5 mo old, n = 11–13) were infected with H1N1 virus (0.8x LD50) intranasally during isoflurane anesthesia (or mock control). Following infection, body weight was monitored daily. While it is often observed that patients with severe influenza viral infections frequently develop pneumonia and die from bacterial superinfections and influenza-associated complication, mice infected with H1N1 influenza virus can be lethal without bacterial superinfection. In this study, all infected mice were housed in isolation cages with a sterile air filter during the entire study and it is not expected that these mice were exposed to exogenous bacteria, which could have resulted in infections. The facility was not germ-free, however, so there remains a possibility for contribution of opportunistic infection by the host microbiome. Infection and monitoring of infected mice were conducted with the approval of Institutional Animal Care and Use Committee of Georgia State University in full compliance with the Committee's guidelines.
Enhanced Pause (PenH) of Pulmonary Functions
Enhanced pause (PenH) values for baseline physiological pulmonary functions were measured before virus infection using a whole body plethysmography (EMKA Technologies) as described in a previous study (27). PenH values were then determined at days 3, 7, and 10 postinfection and presented as percent increases above the baseline; maximal PenH measurements occurred at day 10 postinfection.
Lung Histology and Cytokines
Lung tissue samples were harvested from the mice at 10 days after virus infection and the left lobes of lung tissues treated with 10% of neutral formalin for fixation. The fixed lungs were processed and the lung sections were stained with hematoxylin and eosin as described (26, 33). Photographs were acquired by a microscope (Zeiss Axiovert 100) at 100× magnification and an attached camera (Canon 30D). For numerical assessment of histopathology and pneumonia in airways, blood vessels and interstitial spaces were scored on a scale of 0–5 by blinded observers with the severity scoring system as previously described (11, 26). The right lobes of the lung tissues were collected at 10 days postinfection and extracts were prepared by grinding and flow through a cell strainer. For measuring cytokine levels in lung extracts, IL-1β, IL-6, IL-10, and TNF-α and IFN-γ ready-set-go kits (eBiosciences) were used by following manufacturer’s instruction.
Analysis of Cellular Redox State
Total protein thiol measurement.
Lung tissues after influenza virus infection or saline treatment were analyzed for protein thiol levels using Ellman’s reagent. Briefly DTNB (1 mM) in 100 mM potassium phosphate buffer with 1 mM EDTA at pH 7.5 was added to the cellular proteins that were obtained by protein precipitation using 10% trichloroacetic acid and resolubilization. Total protein thiols were measured at an absorbance of 412 nm (ϵ = 14,150 M−1·cm−1) following 5 min incubation in the dark with lysate-free buffer as a blank. Final values were normalized to the protein content (6).
Redox state of cysteine/cystine (EhCySS).
Immediately after excision, 20–30 mg lung tissue was derivatized following the procedures as described previously (14, 15, 28). Briefly, tissue was placed in 500 μl of ice cold lysis buffer (5% perchloric acid, 0.1 M boric acid and 10 µM γ-glutamylglutamate in dH2O), sonicated, and centrifuged, and 300 μl of supernatant was collected. Then 50 mM iodoacetic acid was added to the supernatant followed by derivatization with dansyl chloride. Samples were then analyzed by HPLC with fluorescence detection for quantitation of cysteine (Cys) and cystine (CySS). Concentration of Cys and CySS was used to calculate redox potential of Cys/CySS (EhCySS) using the Nernst equation.
Sample Preparation for High-Resolution Mass Spectrometry
For metabolomics analysis, mice were euthanized by isoflurane 10 days post-infection; 20–30 mg of lung tissue was extracted by homogenization in 15 µl/mg of 2:1 acetonitrile:water containing a mixture of stable isotope-labeled internal standards (17). Samples were incubated on ice for 30 min, then centrifuged to remove precipitates. Supernatants were stored at −80oC until LC/MS analysis.
High-Resolution Mass Spectrometry and Data Preprocessing
For acquisition of lung tissue extract HRM data, each sample was analyzed with three technical replicates, and data were averaged before statistical analyses. For each sample, 5 µl aliquots were injected and analyzed by liquid chromatography with Fourier transform mass spectrometry (Dionex Ultimate 3000, Q-Exactive HF, Thermo Fisher) with HILIC chromatography/positive electrospray ionization (ESI) mode and resolution of 120,000 (54). A quality control pooled reference plasma sample (referred to as Q-Std3) was included at the beginning and end of each analytical batch of 20 samples for normalization and post hoc quantification (19); a pooled lung extract was also used to monitor data acquisition and quality. Spectral mass to charge (m/z) features were acquired in scan range 85–1,275 m/z. Raw data files were converted to centroided .mzXML with ProteoWizard, and data were extracted using XCMS (53). Triplicate injections were averaged, filtered for less than 70% non-missing values across all samples and 80% non-missing values in at least one group, log2 transformed, and quantile normalized (18, 19). Selection of significantly different metabolic features between two conditions was obtained based on limma with FDR threshold of 0.05. For pathway analyses, metabolites with raw P < 0.05 were used.
Metabolite Annotation and Identification
Metabolites were annotated by matching the accurate mass m/z for adducts commonly formed under positive ESI conditions to the METLIN (https://metlin.scripps.edu/index.php) and Kyoto Encyclopedia of Genes and Genomes (KEGG) (http://www.genome.jp/kegg/pathway.html) databases using a mass error threshold of 10 ppm (relative m/z error × 106). Identities of several metabolites of interest associated with tryptophan and kynurenine pathway (e.g., tryptophan, kynurenine, hydroxyindolacetic acid, indole), nucleotide metabolism (inosine, AMP, uridine, cytidine), and phospholipid metabolism [sphinganine, sphingosine, serine, phosphatidylethanolamine (PE)] were confirmed by coelution with standards and ion dissociation (MS/MS) spectra (17–19). Other annotations are supported by pathway enrichment and metabolic correlations to improve annotation confidence (36), especially when authentic reference standards were not available and/or the abundance was too low for ion dissociation (MS/MS) analysis.
Metabolic Pathway Analysis Using Kyoto Encyclopedia of Genes and Genomes (KEGG) and mummichog
Quantitatively different metabolites were visualized in KEGG metabolic pathway analysis (http://www.genome.jp/kegg/pathway.html#metabolism). Pathway enrichment and module analysis were performed for significant metabolic features (m/z) using mummichog. Pathways that have P value of <0.05 are presented. Plots of intensities for selected metabolites in significant pathways are provided to compare mock-infected control and infected groups. Mummichog pathway analysis was similarly performed to determine pathways corresponding to the metabolome associated with each cytokine cluster.
Metabolome-wide Association Study with Inflammatory Cytokines (cMWAS)
Integrative analysis of cytokine data with metabolomics was performed using Partial Least Squares Regression (PLSR) and network() function implemented in R package mixOmics (21, 35). R package plsgenomics was used to determine the optimal number of latent components. The network() function in the mixOmics package was used to generate the association matrix and visualize the associations (21). Student’s t-test was used to evaluate the statistical significance of correlations.
Expression Levels of Genes Associated with cMWAS
Lung mRNA was isolated and hybridized to Affymetrix MoGene ST 2.0 chips for gene analysis. The resulting .CEL file data were transformed by Robust Multi-Array (RMA, from the R package ‘oligo’) and gene names were assigned to the resulting transcript cluster IDs by NetAffx Batch Query (http://affymetrix.com). Metabolic pathways of interest that had been identified by mummichog (see materials and methods) were searched in the Small Molecule Pathway Database (http://smpdb.ca/), which gives gene symbols connected to the pathways of interest. Such genes were isolated from the RMA data, and those meeting a significant t-test (P < 0.05) criteria were listed in the supplement.
RESULTS
H1N1 Influenza Virus Infection Stimulates Pathogenesis and Oxidative Stress in Mice
Previously studied phenotypic disease manifestations of the 2009 pandemic H1N1 influenza virus in mice (48) were used for characterization of health outcomes in this study to ensure an appropriate model for examining metabolic associations using the HRM approach. H1N1 virus-infected mice were monitored daily for changes in body weight and reached maximal weight loss at day 10 postinfection [mock control (n = 11), 102 ± 0.6%; H1N1 (n = 13), 77.9 ± 1.7%, Fig. 1A]. PenH values (representing lung function) at days 0, 3, 7, and 10 after infection showed that physiological pulmonary dysfunction was highest at day 10 (mock control, 100 ± 13%; H1N1, 680 ± 85%, Fig. 1B). Mice were euthanized at day 10 postinfection for histopathology (Fig. 2). Results showed inflammation around the airways, blood vessels, and interstitial spaces as indicted by arrows in a representative image of an H1N1 virus treated lung (H1N1, Fig. 2A). Blinded scoring confirmed significant inflammation around the airways (Fig. 2B), blood vessels (Fig. 2C), and interstitial spaces (Fig. 2D). The phenotypes of infiltrating immune cells into the lung tissues were identified by multi-color flow cytometry and showed 10- to 100-fold increase in inflammatory cells compared with control, including monocytes, neutrophils, eosinophils, plasmacytoid dendritic cells (DCs), and CD103+ DCs and CD11b+ DCs (data not shown).
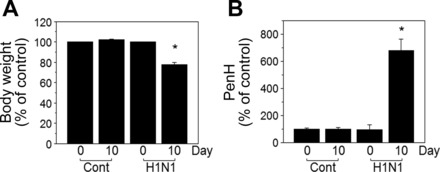
Influenza virus infection of mice induces body weight loss and airway resistance. Groups of mice (n = 11 for mock control, n = 13 for H1N1) were intranasally infected with 2009 H1N1 influenza virus (0.8x LD50). A: body weight loss (% of day 0) at day 10 postinfection [Cont (mean ± SE, day 0, 29.3 ± 0.7 g; day 10, 30.3 ± 0.6 g), H1N1 (mean ± SE, day 0, 29.9 ± 0.8 g; day 10, 23.6 ± 0.7 g)]. B: percentages (%) of PenH values (enhanced pause) at day 10 postinfection as measured by plethysmography of live animals. The data are presented as means ± SE (n = 10–13) and statistical significance was performed by Student’s t-test in GraphPad Prism. *P < 0.001 vs. control group.
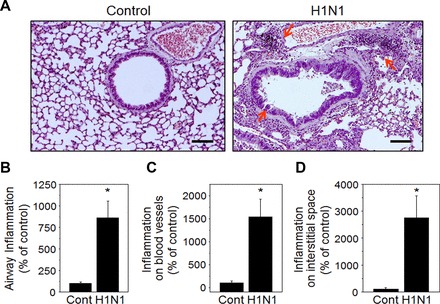
Pulmonary histopathology around the airways, blood vessels, and interstitial spaces. A: lung histology sections stained with hematoxylin and eosin (H and E) at day 10 postinfection. Scale bars indicate 100 µm. Arrows point to airways, blood vessels, and interstitial spaces. B–D: inflammation scoring. Inflammation response on H and E-stained tissue section was scored in airways, blood vessels, and interstitial spaces on a scale of 0 to 5 according to diagnostic criteria. Results (n = 10–13 per group) are presented as means ± SE, and statistical significance was performed by one-way ANOVA with Tukey’s multiple comparisons posttest in GraphPad Prism; *P < 0.001 vs. control group.
Pro- and anti-inflammatory cytokines and oxidative stress markers in lung extracts were also significantly increased (Fig. 3), indicating severe pulmonary inflammation due to H1N1 virus infection. Significant increases of IL-1β, IL-6, IL-10, TNF-α, and IFN-γ in lung extracts from infected mice at day 10 postinfection (Fig. 3, A–E) were observed. H1N1 viral infection of mice resulted in severe disease correlating with pulmonary dysfunction, inflammatory histopathology, high levels of cytokines and oxidative status, implicating metabolic changes associated with disease. Similarly, total protein thiol concentration was decreased by influenza virus infection (control, 610 ± 80; H1N1, 395 ± 100 mmol/mg protein, Fig. 3F) while concentration of the oxidative stress marker cystine (CySS), disulfide of the amino acid cysteine (Cys), was increased (control, 35 ± 3.2; H1N1, 174.1 ± 41.8 μM, Fig. 3G). As an indication of increased level of oxidative stress, the calculated redox potential by the Nernst equation [Eh (mV), control, −116.9 ± 2.9; H1N1, −100.0 ± 4.6, Fig. 3H] using [Cys] and [CySS] was more positive (i.e., oxidized) consistent with elevated oxidative stress by influenza virus infection. These measures established the suitability of the mouse H1N1 virus infection model to determine metabolic associations of infection and cytokine levels.
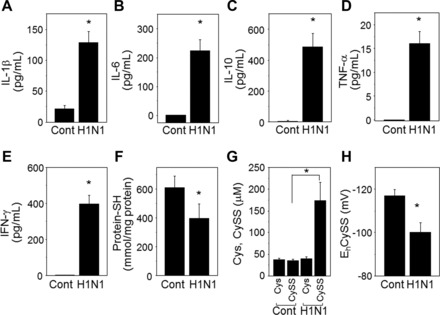
Influenza virus infection induces proinflammatory cytokines and cellular oxidation. Amounts of cytokines extracted from lung tissue of mice at day 10 postinfection (A–E) were determined by using each corresponding cytokine ELISA kit. Results are presented as means ± SE (n = 10–13), and statistical significance was performed by Student’s t-test in GraphPad Prism; *P < 0.001 vs. control group. Levels of cellular oxidation status of lung tissues were determined by total thiol amounts (F) and redox potential of Cys/CySS (G, H). *P < 0.05 vs. control group (n = 7–8 per group).
HRM Identified Lung Metabolic Characteristics Associated with H1N1 Infection
After data extraction and quality filtering, a total of 4,552 m/z ions (metabolic features) were detected in lung tissue extracts from mice at 10 days after challenge with influenza virus (n = 7) or saline (n = 8) (see Supplement 1; note that Supplements 1–5 are available with the online version of this article). For these comparisons, it is important to note that whole lung tissues were analyzed without saline perfusion or other methods to remove blood and immune cells. Partial least-squares discriminant analysis (PLS-DA) showed separation between H1N1 influenza virus infected and control groups by the first two principal components, PC1 (15.6%) and PC2 (17.9%) (Fig. 4A). Three of the top five metabolic features contributing to separation by PLS-DA included m/z ions with accurate mass matches to tryptophan (Trp) metabolites. These included kynurenine (m/z 209.0915, 200 s), a previously confirmed metabolite using this platform (19), and accurate mass matches to its related metabolites, hydroxyindoleacetic acid (m/z 192.0649, 202 s) and methyleneoxindole (m/z 146.0596, 200 s). All three of these metabolites showed increased abundance in infected mouse lung, consistent with extensive literature on altered Trp following infection and discussed in more detail below. The contribution of these metabolites to separation of infected and control mice provided a useful positive control for the metabolomics analysis. The other ions matched a possible dietary sesquiterpenoid (m/z 509.3783, 272 s) and bis-hydroxypropylamine (m/z 151.1437, 228 s), a metabolite of choline produced by bacteria.
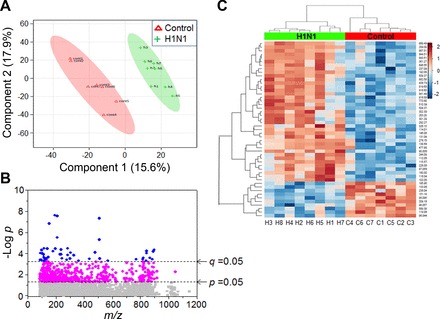
High-resolution metabolomics (HRM)-identified lung metabolic characteristics associated with H1N1 influenza virus infection. Lungs from mice infected with H1N1 influenza virus (green, n = 8) for 10 days or treated with saline control (red, n = 7) were analyzed for HRM. Mass spectral data of 4552 metabolic features (metabolic ions, m/z) obtained from HRM were further examined for partial least-squares discriminant analysis (PLS-DA) to compare between two groups (A). Of 4,552 features, 549 (P < 0.05, magenta + blue symbols) and 49 features (q < 0.05, blue circles) that are significantly different between two groups analyzed by limma and Benjamini and Hochberg false discovery rate (FDR) are shown, respectively (B). C: two-way hierarchical cluster analysis of the 49 metabolic features.
To test for metabolites that significantly differed between lungs from infected and noninfected controls, the 4,552 metabolic features were analyzed using limma. A total of 549 features differed at raw P < 0.05 (magenta + blue, Fig. 4B), and 49 differed using the Benjamini and Hochberg false discovery rate (FDR) procedure (q < 0.05, blue circles, Fig. 4B). Two-way hierarchical cluster analysis of the 49 features for the eight H1N1-infected mice and seven control mice showed three major metabolite clusters (10 decreased metabolites, cluster 1; 39 increased, clusters 2 and 3) that separated the mice into clusters of infected and control mice (Fig. 4C). High-resolution database matching [Supplement 2, level 5 identification (52)] showed that cluster 1 included matches to glycerylphosphorylethanolamine, a precursor for phosphatidylcholine biosynthesis, and multiple metabolites derived from choline breakdown by bacteria, i.e, trimethylamine oxide; amino propanol, trimethylaminoacetone, and bis-(2-hydroxypropyl)amine. A match was obtained for a fatty alcohol, dodecanol, known to affect quorum sensing in Candida albicans (24). Other matches to decreased m/z ions included mitochondria-related metabolites methylniacinamide (metabolic product of niacin and Trp), pyrroline-5-carboxylate, a mitochondrial intermediate in glutamate, ornithine, and proline metabolism, the nonessential amino acid asparagine, and hexaprenyl hydroxybenzoic acid, an intermediate in CoQ biosynthesis.
Of the clusters with increased abundance following infection, cluster 2 contained matches to pyrimidine metabolites (cytidine, cytosine), a dipeptide breakdown product of protein cross-linking (γ-glutamyl-ε-lysine), kynurenic acid (Trp metabolite), and the essential amino acid, threonine. The larger cluster 3 contained matches to eight phosphatidylcholines and several phosphatidylglycerols, phosphatidylethanolamines, and phosphatidylserine. This cluster also included matches to two fatty acids, sphinganine, four sterols (multiple matches to bile acids and vitamin D3 metabolites), and four Trp metabolites.
Metabolic Pathways Affected by Influenza Virus Infection
FDR adjustment protects against inclusion of false positives (Type 1 statistical error) but does this by excluding many true positives (Type 2 statistical error). A statistical approach to minimize this problem involves application of pathway enrichment tools to determine whether the 549 m/z ions that differed at raw P < 0.05 were enriched in metabolites from specific metabolic pathways. As shown in Fig. 5 and Fig. 6, the pathway enrichment program mummichog (36), along with KEGG and MetaCore databases, showed that many amino acids pathways are affected by infection. The major metabolic pathways with −log P values greater than 2.0 include Trp, arginine/proline, lysine, aspartate/asparagine, alanine, urea cycle, vitamin B3/B6, biopterin, pyrimidine, glycine, and histidine (Fig. 5). Trp metabolism had the greatest –log P with an overlap of 27 of 50 ions (P = 0.00367, Fig. 5) associated with the Trp metabolic pathways. Influenza virus infection substantially altered abundance of m/z ions matching 7 key metabolites of Trp regulation including hydroxy-Trp, Trp, formylkynurenine, kynurenine, indole, hydroxyindole acetate, and indole acetaldehyde (Fig. 7). The extensive list of amino acid pathways impacted by infection underscores the serious impact of influenza virus infection on metabolism. It should be noted, however, that some metabolites are common to multiple pathways so that this may overemphasize the breadth of effect.
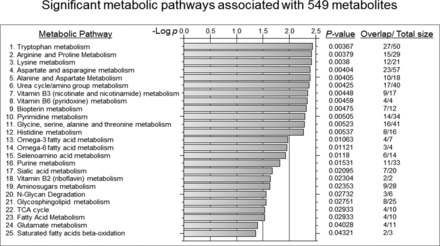
Mummichog-identified metabolic pathways significantly affected by influenza virus infection. The 549 metabolic features were examined for pathway enrichment analysis using mummichog software. The 25 metabolic pathways significantly affected by influenza virus infection are shown by −log P (P < 0.05) and numbers of matched features in a pathway are indicated in overlap/total size.
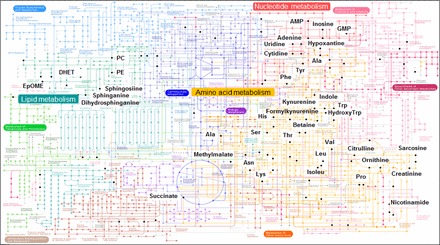
Kyoto Encyclopedia of Genes and Genomes (KEGG). The 549 features were examined for metabolic pathway analysis using KEGG software (http://www.genome.jp/kegg-bin/show_pathway?map01100). Matched features are labeled by black dots and names. Three major pathways affected by influenza infection (amino acid metabolism, nucleotide metabolism, and lipid metabolism) are highlighted.
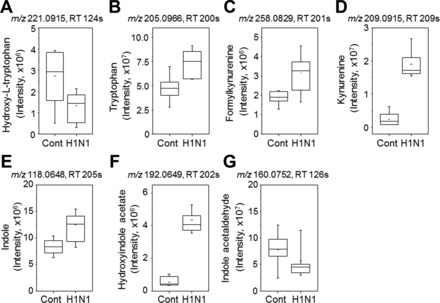
Influenza virus infection-altered lung levels of metabolites associated with Trp metabolism. Influenza virus infection changed lung levels of metabolites of Trp (A, B), kynurenine (C, D), and indole (E–G) compared with mock control. Mass to charge (m/z) and retention time (RT, s) for respective metabolite are indicated (top), and lung amount of each metabolite calculated from peak intensity is shown in whisker plot comparing between 7 mock control and 8 infected mice.
Other central metabolic pathways impacted by H1N1 infection included nucleotide (pyrimidine, purine) and lipid (fatty acid, glycerophospholipids) metabolism (Fig. 6). Matches to metabolites in nucleotide metabolic pathway that were changed due to influenza virus infection included inosine, AMP, hypoxanthine, adenosine, allantoin, uridine, and cytidine (Fig. 8). Metabolites displaying a trend of increased levels in the glycerophospholipids (Fig. 9) pathways after H1N1 influenza virus infection were found to be sphingosine, sphinganine, serine, and phosphatidylethanolamine. These results suggest that influenza virus infection significantly interrupts a range of critical metabolic pathways that might be associated with severity of disease.
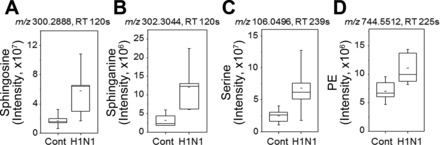
Influenza virus infection-increased metabolites associated with sphingolipid metabolism. The 4 metabolites including sphingosine (A), sphinganine (B), serine (C), and phosphoethanolamine (PE, D) involved in sphingolipid metabolism are significantly elevated by influenza virus infection compared with mock control, and amounts in lung are calculated as described in Fig. 7.
Metabolome-wide Association Study of Pro- and Anti-inflammatory Cytokines
Because morbidity and mortality of influenza virus infection are associated with the extent of cytokine expression, we examined the interaction of metabolic changes with pathological phenotypes by evaluating correlation between abundances of lung cytokines (IL-1β, IL-6, IL-10, TNF-α, IFN-γ) and metabolites (Fig. 10, Supplement 3, Supplement 4). The results of this cytokine-metabolome wide association study (cMWAS) showed that 396 metabolic features were highly correlated (|r| ≥ 0.7) with abundance of at least one cytokine. Most of these metabolites were positively correlated with cytokines, which were significantly increased by influenza virus infection (red edges, Supplement 4). While these findings were very similar to the direct comparisons of infected and noninfected mouse lungs, the further analysis of pathways associated with clusters from the cMWAS provided additional insight.
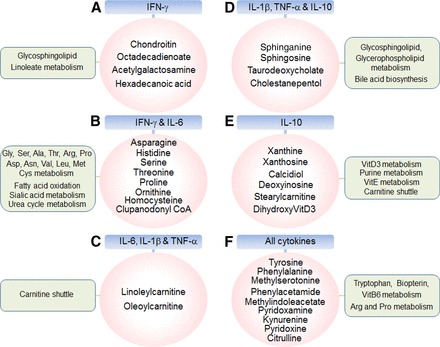
Cytokine-metabolome-wide association study (cMWAS). Relationship between lung metabolites affected by influenza virus infection and five pro- and anti-inflammatory cytokines including interferon (IFN)-γ, IL-6, IL-1β, TNF-α, and IL-10 were examined using partial least squares regression and network function (see materials and methods). Of 396 metabolic features, key metabolites with 6 clusters interacted with cytokines are shown (magenta circle) as follows: A, metabolites correlated with IFN-γ only; B, metabolites correlated with IFN-γ and IL-6; C, metabolites correlated with IL-6, IL-1β, and TNF-α; D, metabolites correlated with IL-1β, TNF-α, and IL-10; E, metabolites correlated with IL-10; F, metabolites correlated with all cytokines. Significant pathways of 6 metabolic clusters analyzed by mummichog pathway enrichment software are also indicated in a box (green). The details of metabolites associated with pathways and cMWAS network are provided in Supplement 3 and Supplement 4, respectively, available with the online version of this article.
The six clusters of cMWAS and significant metabolic features are shown with metabolome correlated with IFN-γ only metabolome (40 metabolic features, m/z) (A), IFN-γ and IL-6 metabolome pathways together (28 metabolic features) (B), IL-6, IL-1β, and TNFα (45 metabolic features) (C), IL-1β, TNF-α, and IL-10 (103 metabolic features) (D), IL-10 only (94 metabolic features) (E), and all cytokines(77 metabolic features) (F) (Fig. 10, Supplement 4). Overall significant pathways associated with inflammatory cytokines are carnitine shuttle (C and E, P = 0.0027), urea cycle/amino group metabolism (B and F, P = 0.0043), glycosphingolipid metabolism (A and D, P = 0.0073), Trp metabolism (F, P = 0.0082), vitamin E metabolism (E, P = 0.012), lipoate metabolism (P = 0.026), vitamin B6 metabolism (F, P = 0.026), Gly/Ser/Ala/Thr metabolism (b, P = 0.033), and Tyr metabolism (F, P = 0.048). Amino acid pathways and carnitine shuttle were associated with two of the largest cytokine clusters. Some of the more distinct associations included negative associations of IFN-γ with metabolites that were enriched for glycosphingolipid and linoleate pathways and altered sialic acid metabolism associated with IFN-γ and IL-6, and bile acid biosynthesis associated with IL-6, TNF-α and IL-10. Alternatively, the anti-inflammatory cytokine IL-10 was positively associated with pathways for vitamin D3 metabolism and vitamin E metabolism as well as purine metabolism. Furthermore, targeted analysis of gene expression was performed to detect genes related to cytokine-associated metabolic pathways (Fig. 10). The analysis revealed pathway-specific changes in gene expression by H1N1 virus infection (Supplement 5) that were consistent with the metabolic changes, including genes related to bile acid, carnitine shuttle and fatty acid, glycerophospholipid, purine, pyrimidine, sphingolipid, tryptophan and urea cycle metabolism.
DISCUSSION
Metabolomics is a rapidly evolving set of approaches to fill a critical gap in systems biology of disease by measuring differences in metabolite abundance and/or flux in biological systems in association or response to disease or experimental perturbation. Mass spectrometry-based metabolomics studies on influenza virus-infected cell lines and animal models (8, 37) and human cerebral spinal fluid (30) have mostly focused on discovery of useful biomarkers, but these studies also provide important information concerning infection and outcome. In particular, the studies show that influenza virus infection causes changes in many amino acid categories and lipid metabolism. Changes in amino acid metabolism may reflect immune response to infection and/or tissue injury and repair, while changes in lipid metabolism suggest that metabolism of surfactant, which is critical for lung function, is disrupted following infection. Thus the findings suggest that more specific understanding of the underlying causes of metabolic disruptions in response to H1N1 infection and their relationships to disease outcomes could provide a basis for metabolic interventions to decrease adverse responses, improving prognosis.
In the present study, we simultaneously examined the lung metabolome and inflammatory markers to obtain detailed information of metabolic associations with inflammation. We confirmed that influenza virus infection induced inflammatory disease markers in mice 10 days after infection, with exacerbated lung dysfunction, severe body weight loss, histopathology, proinflammatory cytokines, and oxidation of redox state (Figs. 1–3).
We then applied powerful non-targeted HRM methods to identify significantly altered metabolites and associated pathways (Figs. 4–9). This unbiased approach confirmed many metabolites and pathways found in previous studies (8, 37, 56), e.g., pyrimidine and purine nucleotide metabolism, lipid metabolism, and amino acid metabolism. Findings in our study at 10 days were comparable to lung tissue metabolomics and histopathology reported at 10 days as part of a larger time course (8), suggesting the kinetics of our experiment and viral titer were similar to that of others. Thus the findings suggest that targeted interventions in these pathways could be considered for therapies to reduce serious illness associated with influenza virus infection. However, further studies in humans, likely utilizing plasma or serum as a common clinical sample (19, 29, 60), are needed to validate whether such interventions may be beneficial. Furthermore, differing infectious or noninfectious inflammatory stimuli such as oleic acid may elicit similar lung injury and inflammation (20) to H1N1, but likely have specific metabolomic signatures.
Among these, elevated levels of metabolites of tryptophan (Trp), kynurenine (kyn), and indole from virus-infected mice warrant attention. Trp metabolism involving these metabolites is a major pathway associated with inflammatory disease (10, 61). Trp is converted to formylkynurenine by indoleamine-pyrrole 2, 3-dioxygenase that is upregulated in response to inflammatory conditions such as atherosclerosis and depression (9, 61, 66). In addition, hydroxyindole acetate is a serotonin metabolite (49), and the level of hydroxyindole acetate was elevated by influenza virus infection in the present study. Serotonin secretion in central nervous systems and spinal cords is associated with psychiatric depression symptoms, unexplained diarrhea, and irritable bowel syndrome disease and inflammation (9, 41, 55, 64). Furthermore, proper generation of aryl hydrocarbon receptor agonists via Trp metabolism is linked to the attenuation of inflammation in a mouse model of colitis (34). Based on results from the previous and the current studies, it is sufficient to state that alteration in Trp metabolism is a marker of inflammation. While cause-effect relationships of Trp metabolic changes and relationships to outcomes remain unclear, management of Trp metabolism through monitoring and selective interventions could potentially be useful to minimize adverse outcomes following infection.
Adenine, a purine base, was detected at a decreased level in mice with inflammatory disease due to influenza virus infection (Fig. 8). Consistently, adenine administration was shown to inhibit the induction of inflammatory cytokines (TNF-α, IL-8) in intestinal epithelial cells as well as inflammatory colitis in a mouse model (13), suggesting that adenine plays a role in maintaining anti-inflammatory homeostasis. Other metabolites also responded to inflammatory disease in previous studies. For example, hypoxanthine that was elevated by influenza virus infection in our study was also detected at higher levels in synovial fluids from patients with rheumatoid arthritis (23). In addition, sphingosine-1-phosphate in sphingolipid metabolism is known as an important mediator of inflammation and cancer (25) and its receptor antagonist has been shown to regulate autoimmune neuroinflammation in multiple sclerosis (47). Together, these data suggest that HRM-determined increased levels of metabolites involved in pathways for purine/pyrimidine nucleotides and lipid metabolism could provide useful biomarkers and/or a basis for metabolic intervention to minimize adverse outcomes from influenza virus infection.
The severe morbidity of influenza is closely related to dysregulation of inflammatory cytokines (58), indicating that the high mortality rate of influenza virus infection might be a consequence of an overactive inflammatory response. This underscores the value of qualitative and quantitative data of cytokines to understand the pathogenesis of viral infection. One must be aware, however, that impaired gas exchange might also affect metabolite levels, and this was not monitored. In the present study, we examined the relationship between cytokines and metabolism through use of cMWAS following influenza virus infection (Fig. 10). Importantly, this association-based method provides biological specificity and meaning to metabolic alterations of H1N1 infection. The results showed associations of 10 metabolic pathways with inflammatory cytokines. Among these, there were distinct clusters of metabolites associated with some cytokines, but more commonly, clusters of metabolites were associated with multiple cytokines. A cluster of 40 metabolites correlated with IFN-γ (A, top cluster of IFN-γ in Fig. 10) and included 32 metabolites that were negatively correlated (Supplement 4). A cluster of 94 metabolites was associated with IL-10 only (E in Fig. 10). Such distinct patterns in cMWAS associations could be due to involvement of different cell types at different time after virus infection. This aspect is supported by the previous studies demonstrating that IFN-γ secreted by T lymphocytes and natural killer cells shortly after infection plays a critical role in regulatory immune response and in the control of viral infection (22, 62). In addition to IFN-γ, IL-10 also plays a key role in controlling inflammation and inhibiting disease in virus-infected mice (39). Metabolites of calcitriol (24,25-dihydroxyvitamin D3), acylcarnitine, and carboxychromanol are correlated with IL-10 in cMWAS, supporting the potential immunomodulatory roles of vitamin-D3 (1–3, 43), vitamin E, and carnitine. Accumulating data support a critical role of vitamin-D3 in IL-10 production in human cells via interacting with their receptors (1–3, 43).
IL-1β and TNFα may respond to and coordinate similar metabolic pathway changes, according to high overlap in cMWAS associations. We found that metabolites regulating bile acid biosynthesis were also highly correlated with cytokines including IL-1β and TNFα. The previous study by Lu et al. (40) showed that peroxisomal multifunctional enzyme type 2 (HSD17B4) in bile acid biosynthesis pathway, which converts dihydroxy-cholestenoyl-CoA to trihydroxy-beta-cholestanoyl-CoA, is regulated by NF-κB, suggesting that bile acid biosynthesis is closely associated with inflammation mechanisms. Although syntheses of bile acids occur mostly in the liver by P450 (CYP7A1)-mediated cholesterol oxidation via the classic pathway (46, 51), elevated levels of genes and metabolites of bile acid biosynthesis in lung were shown in association with pulmonary diseases such as pulmonary arterial hypertension and lung transplantation (45, 65). Consistently, targeted analysis of transcriptomics data show that genes involved in this pathway including cytochrome P450 family 7 (Cyp7b1, 1.2-fold, P < 0.01), and cholesterol 25-hydroxylase (Ch25h, 1.4-fold, P < 0.01) are increased in lungs of H1N1-infected mice (Supplement 5). Further studies with multiple time points after infection and cell type-dependent analysis will be needed to provide more detailed information.
In summary, HRM-identified metabolic features and pathways with simultaneous measures of inflammatory cytokines provide profound resources to generate metabolic associations with inflammation (cMWAS). Such interactive analysis improves our understanding of complex inflammatory diseases, validates potential metabolic biomarkers for inflammation, and further suggests that metabolic features identified by cMWAS could be therapeutic targets in controlling outcome from inflammatory disease such as influenza.
Perspectives and Significance
This HRM analysis provides an unbiased characterization of metabolism following influenza infection in mice and confirms previous findings of profound effects on tryptophan metabolism and many other changes in amino acid, lipid, and nucleotide metabolism. Importantly, the results show both overlapping and distinct associations of metabolites and metabolic pathway effects with specific cytokines. The results imply that selective inhibition of metabolic pathways and/or supplementation of intermediates in response to cytokine profiles may provide an approach to stabilize metabolism and protect against adverse outcomes of excessive cytokine activation during severe infection.
GRANTS
This study was supported by National Institute of Environmental Health Sciences Grants R01-ES-023485 (D. P. Jones and Y.-M. Go) and R21-ES-025632 (DPJ and Y.-M. Go), National Institutes of Health Grant S10-OD-018006 (D. P. Jones), National Institute of Allergy and Infectious Diseases Grants R01-AI-105170 (S.-M. Kang), R01-AI-093772 (S.-M. Kang), and R21-AI-119366 (S.-M. Kang), and Cystic Fibrosis Foundation CHANDL16F0 (JDC).
AUTHOR CONTRIBUTIONS
J.D.C., X.H., E.-J.K., S.P., Y.-T.L., M.L.O., and J.F. performed experiments; J.D.C., X.H., E.-J.K., S.P., Y.-T.L., J.F., K.U., S.-M.K., and Y.-M.G. analyzed data; J.D.C., X.H., E.-J.K., Y.-T.L., J.F., K.U., S.-M.K., D.P.J., and Y.-M.G. interpreted results of experiments; J.D.C., X.H., E.-J.K., S.P., Y.-T.L., K.U., and Y.-M.G. prepared figures; J.D.C., X.H., Y.-T.L., S.-M.K., D.P.J., and Y.-M.G. edited and revised manuscript; J.D.C., X.H., E.-J.K., S.P., Y.-T.L., M.L.O., J.F., K.U., S.-M.K., D.P.J., and Y.-M.G. approved final version of manuscript; S.-M.K., D.P.J., and Y.-M.G. conception and design of research; Y.-M.G. drafted manuscript.
ACKNOWLEDGMENTS
We thank Drs. Sushma K. Cribbs (MD, Pulmonary Medicine, Emory University), Annette Esper (MD, Pulmonary Medicine, Emory University), and Shuzhao Li (PhD, Director of Immunometabolomics, Emory University) for providing comments about the manuscript.
REFERENCES
Articles from American Journal of Physiology - Regulatory, Integrative and Comparative Physiology are provided here courtesy of American Physiological Society
Full text links
Read article at publisher's site: https://doi.org/10.1152/ajpregu.00298.2016
Read article for free, from open access legal sources, via Unpaywall:
https://www.physiology.org/doi/pdf/10.1152/ajpregu.00298.2016
Citations & impact
Impact metrics
Article citations
Metabolomic Signatures Differentiate Immune Responses in Avian Influenza Vaccine Recipients.
J Infect Dis, 230(3):716-725, 01 Sep 2024
Cited by: 1 article | PMID: 38181048
Propolis Alleviates Acute Lung Injury Induced by Heat-Inactivated Methicillin-Resistant Staphylococcus aureus via Regulating Inflammatory Mediators, Gut Microbiota and Serum Metabolites.
Nutrients, 16(11):1598, 24 May 2024
Cited by: 0 articles | PMID: 38892531 | PMCID: PMC11175110
Multi-omics analysis of the gut microbiome and metabolites associated with the psychoneurological symptom cluster in children with cancer receiving chemotherapy.
J Transl Med, 22(1):256, 09 Mar 2024
Cited by: 3 articles | PMID: 38461265 | PMCID: PMC10924342
Prophylactic and therapeutic mouse models for evaluating immunologic resilience to infection with influenza virus by Immulina® (Part 1).
Phytomedicine, 132:155588, 02 Apr 2024
Cited by: 1 article | PMID: 38908194
Inflammation and Organic Cation Transporters Novel (OCTNs).
Biomolecules, 14(4):392, 25 Mar 2024
Cited by: 2 articles | PMID: 38672410 | PMCID: PMC11048549
Review Free full text in Europe PMC
Go to all (65) article citations
Similar Articles
To arrive at the top five similar articles we use a word-weighted algorithm to compare words from the Title and Abstract of each citation.
Pulmonary immune cells and inflammatory cytokine dysregulation are associated with mortality of IL-1R1 -/-mice infected with influenza virus (H1N1).
Zool Res, 38(3):146-154, 01 May 2017
Cited by: 10 articles | PMID: 28585438 | PMCID: PMC5460083
Polygalasaponin F treats mice with pneumonia induced by influenza virus.
Inflammopharmacology, 28(1):299-310, 24 Aug 2019
Cited by: 5 articles | PMID: 31446589 | PMCID: PMC7102181
A Novel Bifunctional Fusion Protein, Vunakizumab-IL22, for Protection Against Pulmonary Immune Injury Caused by Influenza Virus.
Front Immunol, 12:727941, 24 Aug 2021
Cited by: 6 articles | PMID: 34504501 | PMCID: PMC8421727
Integrative Metabolomics to Identify Molecular Signatures of Responses to Vaccines and Infections.
Metabolites, 10(12):E492, 30 Nov 2020
Cited by: 29 articles | PMID: 33266347 | PMCID: PMC7760881
Review Free full text in Europe PMC
Funding
Funders who supported this work.
Cystic Fibrosis Foundation (CFF) (1)
Grant ID: CHANDL16F0
Division of Intramural Research, National Institute of Allergy and Infectious Diseases (Division of Intramural Research of the NIAID) (1)
Grant ID: AI105170
HHS | NIH | National Institute of Allergy and Infectious Diseases (NIAID) (2)
Grant ID: AI093772
Grant ID: AI119366
HHS | NIH | National Institute of Environmental Health Sciences (NIEHS) (2)
Grant ID: ES025632
Grant ID: ES023485
HHS | National Institutes of Health (NIH) (1)
Grant ID: OD018006
NHLBI NIH HHS (1)
Grant ID: P20 HL113451
NIAID NIH HHS (3)
Grant ID: R01 AI093772
Grant ID: R21 AI119366
Grant ID: R01 AI105170
NIEHS NIH HHS (2)
Grant ID: R21 ES025632
Grant ID: R01 ES023485
NIH HHS (1)
Grant ID: S10 OD018006