Abstract
Background
Children who experience early adversity often develop emotion regulatory problems, but little is known about the mechanisms that mediate this relation. We tested whether general associative learning processes contribute to associations between adversity, in the form of child maltreatment, and negative behavioral outcomes.Methods
Eighty-one participants between 12 and 17 years of age were recruited for this study and completed a probabilistic learning Task. Forty-one of these participants had been exposed to physical abuse, a form of early adversity. Forty additional participants without any known history of maltreatment served as a comparison group. All participants (and their parents) also completed portions of the Youth Life Stress Interview to understand adolescent's behavior. We calculated measures of associative learning, and also constructed mathematical models of learning.Results
We found that adolescents exposed to high levels of adversity early in their lives had lower levels of associative learning than comparison adolescents. In addition, we found that impaired associative learning partially explained the higher levels of behavioral problems among youth who suffered early adversity. Using mathematical models, we also found that two components of learning were specifically affected in children exposed to adversity: choice variability and biases in their beliefs about the likelihood of rewards in the environment.Conclusions
Participants who had been exposed to early adversity were less able than their peers to correctly learn which stimuli were likely to result in reward, even after repeated feedback. These individuals also used information about known rewards in their environments less often. In addition, individuals exposed to adversity made decisions early in the learning process as if rewards were less consistent and occurred more at random. These data suggest one mechanism through which early life experience shapes behavioral development.Free full text

Early adversity and learning: implications for typical and atypical behavioral development
Abstract
Background
Children who experience early adversity often develop emotion regulatory problems, but little is known about the mechanisms that mediate this relation. We tested whether general associative learning processes contribute to associations between adversity, in the form of child maltreatment, and negative behavioral outcomes.
Methods
Eighty-one participants between 12 and 17 years of age were recruited for this study and completed a probabilistic learning Task. Forty-one of these participants had been exposed to physical abuse, a form of early adversity. Forty additional participants without any known history of maltreatment served as a comparison group. All participants (and their parents) also completed portions of the Youth Life Stress Interview to understand adolescent’s behavior. We calculated measures of associative learning, and also constructed mathematical models of learning.
Results
We found that adolescents exposed to high levels of adversity early in their lives had lower levels of associative learning than comparison adolescents. In addition, we found that impaired associative learning partially explained the higher levels of behavioral problems among youth who suffered early adversity. Using mathematical models, we also found that two components of learning were specifically affected in children exposed to adversity: choice variability and biases in their beliefs about the likelihood of rewards in the environment.
Conclusions
Participants who had been exposed to early adversity were less able than their peers to correctly learn which stimuli were likely to result in reward, even after repeated feedback. These individuals also used information about known rewards in their environments less often. In addition, individuals exposed to adversity made decisions early in the learning process as if rewards were less consistent and occurred more at random. These data suggest one mechanism through which early life experience shapes behavioral development.
Introduction
Children who experience high levels of early adversity are very likely to develop emotion regulatory problems that undermine adaptive social development. For example, physical abuse during early childhood is associated with the emergence of negative mental health outcomes including aggressive and oppositional behavior (Vachon, Krueger, Rogosch, & Cicchetti, 2015). Although associations between various forms of adversities and later behavioral problems are well-documented, much is still unknown about which specific processes lead to the range of negative outcomes observed in individuals who have had these negative early life experiences. Recently, many scholars who have been studying this issue have advocated for a new conceptual approach. Rather than focusing on specific outcomes (such as depressive or aggressive disorders), researchers are beginning to examine dimensional measures that broadly affect domains of children’s functioning, such as social competence (Casey, Oliveri, & Insel, 2014; Pollak, 2015a). Success at understanding and adjusting to changing social environments is central for healthy adaptation. To this end, we report here on an experiment designed to test whether general associative learning processes are a viable mechanism through which early adversity has a negative impact on children’s behavioral development.
By associative learning, we refer to processes through which events or stimuli become linked to other events or stimuli. For example, stimuli, such as odors and other sensory information, can become coupled with feelings of value or danger through repeated pairings in conditioning experiments (Watson & Rayner, 1920; Wilson & Sullivan, 1994). This conditioning, which is one form of associative learning, can then influence future behavior across a number of different domains (Rescorla, 1988). Impairments in the ability to form associations with new stimuli, or change previous associations, could hinder the potential of an individual to make predictions about, and appropriately adapt to, their environment (and the individuals within an environment).
Aspects of general learning systems appear to be key components for complex social behaviors (Behrens, Hunt, Woolrich, & Rushworth, 2008). As an example, learning occurring from the absence of an expected negative outcome is associated with increased positive emotions and empathy for others (Hein, Engelmann, Vollberg, & Tobler, 2016). Furthermore, some aspects of learning assessed early in development are predictive of later social functioning. Infants with slower associative learning, indexed by eye-blink conditioning at 1 month of age, had lower joint attention and reduced social contingency detection at 1 year of age (Reeb-Sutherland, Levitt, & Fox, 2012). Examined collectively, studies reporting these kinds of results suggest associative learning may be important for social processes. Individuals with poorer associative learning may be slower to understand contingencies in the environment and this may significantly influence behavior during more complex and fast-changing situations, such as those in social interactions (Hammock & Levitt, 2006).
Many different kinds of studies indicate learning is affected by an organism’s caregiving experience. Seminal work comparing rhesus macaques raised by canine surrogates, in contrast to macaques raised by hobby horses, found that response contingent caregiving powerfully shapes learning behavior. Rhesus macaques raised by canine surrogates were more attentive and responsive to their environments, less likely to be indifferent when confronted with change, and more likely to achieve an adaptive outcome by acting on their environment compared to animals raised with hobby horses (Capitanio, 1985; Mason & Capitanio, 1988; Mason & Kenney, 1974). Similarly, when human mothers interacted with their infants via live video feeds, interactions that were not contingently responsive to the infant’s current behavior led infants to be more disengaged and to have slower rates of associative learning (Bigelow & DeCoste, 2003; Papoušek & Papoušek, 1975). These data suggest that caregiving experiences impact how organisms extract and process information from the environment.
There are multiple potential pathways through which adverse environments might affect associative learning systems. Here, we focus on the premise that some types of early adversity undermine the consistency and predictability of the input that children receive. Abusive parents are often inconsistent in their interactions with their children (Wahler & Dumas, 1986). These parents tend to vacillate between-extreme emotional states of anger and social withdrawal (Bousha & Twentyman, 1984; Lyons-Ruth & Block, 1996). Yet, these social interactions with primary caregivers are the initial basis for children’s learning about the social environment. Early adversity also impacts behavioral and neural systems central to attention, executive functions, and emotion processing, processes that are integral to associative learning (Hanson et al., 2010; Hanson, Nacewicz et al., 2015; Pechtel & Pizzagalli, 2011; Pollak, 2015b).
If associative learning is impaired, children are likely to have difficulties adjusting their behavior in dynamic social interactions. Indeed, past research indicates that children and adolescents who suffer early adversity, such as child abuse, are impaired in the ability to detect important environmental cues and adjust their behavior accordingly. For example, Guyer et al. (2006) used a reward task, in which participants had to choose between two stimuli with different amounts of reward and different probabilities of winning. These investigators found the response time of abused children did not differ with the likelihood of reward, unlike nonmaltreated children, who responded more quickly as the chances of winning a reward increased. A reduced sensitivity to positive stimuli in these youth may emerge due to the less positive and less responsive parenting commonly noted in abusive caregivers. Similarly, in a sample of adults exposed to sexual abuse, Pechtel and Pizzagalli (2013) found maltreated participants showed lower accuracy in a probabilistic learning task. Additional studies (Mueller et al., 2012; Weller & Fisher, 2013) reinforce these results, as investigators have found that individuals exposed to early adversity fail to show sensitivity to important environmental cues, specifically changing rewards (Mueller et al., 2012; Weller & Fisher, 2013).
The present study examined associative learning in a sample of adolescents who had suffered physical abuse and other adverse experiences, as well as a group of adolescents who had not experienced extreme adversities. We hypothesized that adolescents who had suffered abuse would have impaired associative learning compared to nonmaltreated adolescents. Because associative learning reflects a child’s ability to make use of information in their environments to guide subsequent behavior, we also predicted that differences in associative learning would account for the relation between a child’s history of physical abuse and levels of disruptive behavioral problems. Finally, in a series of exploratory analyses, we used mathematical modeling methods to investigate which specific subcomponents of learning, such as decision strategies and learning rates over time, might account for behavioral problems. Based on the often inconsistent parenting practices seen in abusive environments, we predicted abused adolescents would behave as though positive feedback based on their choices was less likely and less consistent. We also predicted that physically abused adolescents would be more variable in their choices and have lower use of information about known rewards (or so called ‘exploitation’).
Method
Participants
Eighty-one participants between 12 and 17 years of age were recruited for this study (mean age = 15.02 years, standard deviation = 1.28 years; 41 female). This sample size was chosen based on past studies from our research laboratory focused on similar between-group comparisons (Shackman & Pollak, 2005), as well as previous work employing our experimental paradigm (van den Bos, Güroğlu, van den Bulk, Rombouts, & Crone, 2009). Recruitment of participants who suffered early adversity occurred via a collaboration with Dane County (WI) Child Protective Services (CPS). CPS sent letters about our research to all families with new cases of substantiated maltreatment. Family names were not released to the laboratory, instead families contacted us directly if they wished to learn more about ongoing research.
Forty-one of these participants had been exposed to physical abuse (mean age = 14.85 years, standard deviation = 1.34 years; 18 female, 23 male; 16 Caucasian, 25 non-Caucasian: African-American, Latino, and multiracial). We verified the children’s experience of maltreatment using both records from the Dane County (WI) Department of Human Services and/or parental self-disclosure of abuse on the Conflict Tactics Scale Parent-Child Version (PC-CTS; Straus, Hamby, Finkelhor, Moore, & Runyan, 1998). The PC-CTS quantifies the occurrence of practices that cause distress or fear in children. Sample items include parent admission of actions such as: ‘Grabbed him/her [their child] around the neck and choked him/her’; ‘Burned or scalded him/her on purpose’; and ‘Threatened him/her with a knife/gun.’ Following the practice employed by a number of research groups, parents with scores >20 were categorized as abusive (Shalev et al., 2012). Thirty-five of the abused participants were identified from Dane County (WI) Department of Human Services, while six were classified as abused based on the PC-CTS. Of the participants identified through Dane County, all of the participants had positive reports of physical abuse. In addition, 11 participants in this group also had reports of neglect and one participant had been sexually abused.
Forty participants without any known history of maltreatment served as a comparison group (mean age = 15.19 years, standard deviation = 1.23 years; 22 female, 18 male; 30 Caucasian Ethnicity; 10 non-Caucasian: African-American, Latino, and multiracial). This comparison group included participants with a score of <10 on the PC-CTS and no Department of Human Services records. All parents and participants gave informed consent/assent for the study and the university IRB approved all procedures. Participants in the two groups did not differ in age (β = −.11, p = .311, η2 = .012) or sex (χ2 = .99, p = .317, η2 = .012). The racial composition of the two groups did differ, with the early adversity group having fewer Caucasian participants (χ2 = 12.04, p < .005, η2 = .148).
Measures
Behavioral functioning
Adolescents and their parents each separately completed portions of the Youth Life Stress Interview (YLSI; Rudolph et al., 2000) to elicit information about the adolescent’s behavior. Graduate-level research staff administered this semistructured interview after extensive training from Dr. Rudolph. During this interview, a series of probes was administered to elicit information from children and parents regarding children’s behavioral problems at school (e.g. problems with teachers, disciplinary actions related to disruptive behavior). A panel of three to six trained raters who did not interact with the family used a 5-point scale based on separate parent and child reports. Interviewers were trained to filter out participants’ subjective responses to probes (e.g. child’s affect) during discussion with this rating team. After parent and child reports were scored individually, a consensual rating was assigned integrating information from both informants. Higher scores reflected more serious behavioral problems. For example, a score of 1.5 reflects a child who was rarely in trouble at school, whereas a score of 4 reflects a child who received frequent detentions at school and was often sent to the principal. High reliability has previously been achieved for ratings measuring functioning in different life domains derived from the YLSI (average intraclass correlation = .96; Rudolph et al., 2000; Rudolph & Hammen, 1999).
General cognitive ability
Participants completed the spatial working memory (SWM) task from the Cambridge Neuropsychological Test Automated Battery (CANTAB; Cambridge Cognition; Cambridge, UK) to assess individual differences in cognitive abilities not tied to associative learning. The CANTAB is computerized for standardized administration and does not require verbal responses. CANTAB data were not collected on three participants due to technical issues. A SWM score was calculated for each participant for the total number of errors during the task and z-transformed based on norms for each subject’s age and gender. Because abused children showed poorer cognitive abilities (β = −.35, p = .005, η2 = .123), this variable was used as a covariate in subsequent analyses.
Procedures
Probabilistic learning task
Participants saw two color drawings of everyday objects (e.g. a bell; a bottle) and were instructed to choose one by pressing a button corresponding to the stimulus on the left or right side (van den Bos, Cohen, Kahnt, & Crone, 2012). Stimuli were presented for a maximum of 2,500 ms and offset after participant response. After their choice, participants received positive or negative feedback for 1,000 ms. Feedback was delivered with two different randomized probabilistic schedules, either AB or CD pairs. In AB pairs, the choice of stimulus A led to positive feedback on 80% of trials and stimulus B led to positive feedback on 20% of trials. In CD pairs, stimulus C led to positive feedback on 70% of trials and stimulus D led to positive feedback on 30% of trials. Feedback was given on every trial, except if no response was given within 2,500 ms. In these cases, the text ‘Too Slow’ was presented on the screen after stimulus offset. Our study design is shown in Figure 1.
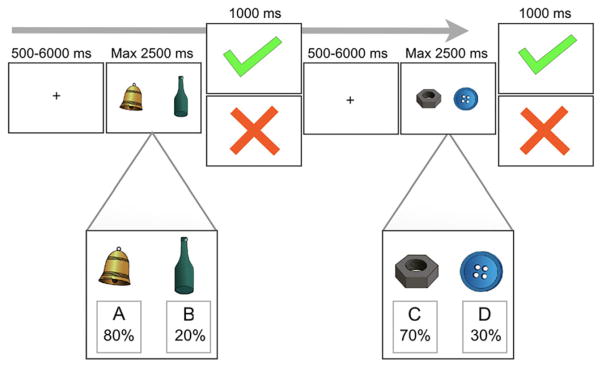
The study design of our probabilistic learning task is detailed in this figure. Participants were presented with two color drawings of everyday objects and instructed to choose one of the two stimuli by pressing a button. Feedback was delivered with two different randomized probabilistic schedules (an 80/20 or 70/30 positive feedback/negative feedback schedule). In the example on the left side, the bell was rewarded on 80% of trials, while the bottle was rewarded on 20% of trials. In the other pairing, on the right side, the bolt was associated with 70% positive feedback, while the button was associated with 30% positive feedback [Colour figure can be viewed at wileyonlinelibrary.com]
Participants were instructed to earn as many points as possible but were also informed that it was not possible to receive positive feedback on every trial. Receiving a positive feedback signal indicated earning of points. Beforehand, each participant completed 50 practice rounds to ensure that they understood the task. Participants completed two runs of 100 trials (50 AB pairs; 50 CD pairs). Each run consisted of different sets of pictures during which participants learned to choose stimuli A and C more often than stimuli B and D. The stimuli were presented in pseudorandom order with a jittered interstimulus interval (minimum = 1,000 ms, maximum = 6,000 ms). Stimuli were presented using E-Prime software (Psychology Software Tools, Pittsburgh, PA) with a screen resolution of 800 × 600 pixels.
Data analyses
Mathematical modeling of learning
To assess subcomponents of associative learning, we fit a reinforcement learning (RL) model to each participant’s behavioral data. This approach is commonly employed in learning and decision-making research with adults (Cohen & Ranganath, 2005; McClure, Berns, & Montague, 2003). Important to our questions of interest, RL models build on a participant’s experiences during an experiment and update decision weights (the likelihood of choosing a given stimulus) over time. A comprehensive summary of our RL methodology is provided in our supplemental materials.
To understand variability in trial-by-trial choices, we modeled the stochasticity of decisions across the experiment (Cohen & Ranganath, 2005). For each participant, this first involves taking the difference between decision weights for opposing stimuli on each trial. This difference is then passed through a sigmoid function (Montague, Hyman, & Cohen, 2004). This mathematical normalization reduces the influence of extreme values (or unexpected choice) in the data without removing them from the dataset. For example, when stimulus pair AB is presented, the probability of choosing stimulus A on trial t is determined by:
In this formula, w represents the decision weight and β accounts for the variability of an individual’s choices. Smaller β values would be indicative of lower ‘exploitation’, the tendency to use known information about the environment. This could also be operationalized as greater variability in choice, as each stimulus set contained one item with a disproportionately higher reward likelihood (e.g. a 70% or 80% rewarded stimulus).
To understand each participant’s expectation of reward, we employed two mathematical modeling approaches and operationalized reward expectation as the starting decision weight for each stimulus at the beginning of the experiment. In line with past work, we first set the decision weights for each stimulus to the same value at the beginning of the experiment. This would indicate that all participants had the same expectation of rewards for each stimulus. In addition, we also modeled this as a free parameter. By explicitly modeling this, it would allow us to capture individual differences in initial expectations of rewards. We used the multivariate constrained minimization function (fmincon) of the optimization toolbox implemented in MATLAB 2012b (MathWorks, Inc., Natick, MA) and a high number of initial decision weights (100 different starting values). This would allow us to arrive at the most reliable model of behavior. Using these different starting points, each individual’s model parameters were tuned to maximize the model fit of an individual’s decision. This was measured by the log likelihood for an individual. The starting decision weights with the maximum fit were selected for the final solution.
Results
Group differences in associative learning performance
To measure associative learning, we calculated the number of highly rewarded stimuli in a pair that each participant chose (that is, the A and C stimuli). We collapsed across stimuli pairs, as consistent with previous reports, performance for AB and CD pairs were highly correlated (van den Bos et al., 2009). To measure learning across the experiment, we created blocks of 20 consecutive trials and summed the number of choices of highly rewarded stimuli in each block. This approach is similar to analytic strategies used in previous studies of adolescent decision-making (Ernst et al., 2003). We then entered participants’ choices for each block into a repeated-measures analyses of variance as the dependent variable, with group (early adversity; comparison group with no such history) and block as independent variables. In this manner, the block variable allowed us to examine associative learning over time with a lower number of choices for highly rewarded stimuli indicating poorer learning.
As predicted, we found adolescents exposed to early adversity were less likely to choose highly rewarded stimuli than comparison adolescents without such exposure (early adversity group mean = 130.92, standard deviation = 30.98; comparison group mean = 154.48, standard deviation = 24.40; F(1,79) = 15.97, p < .001;
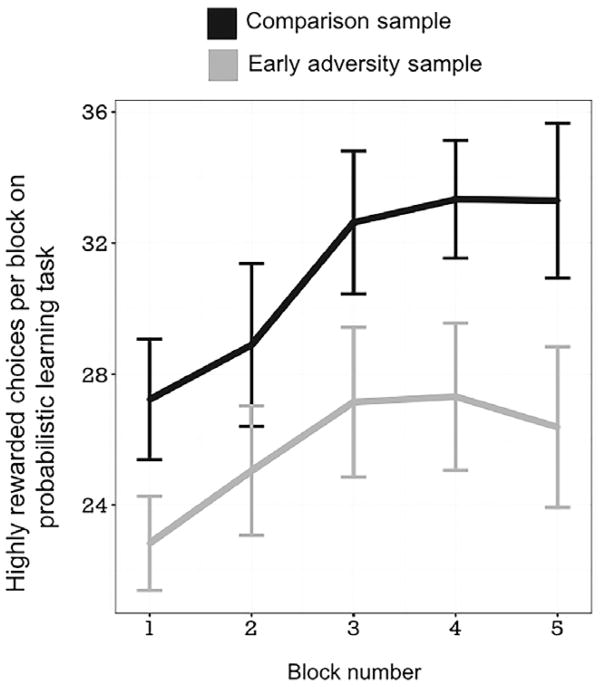
Associative learning performance over the course of the experiment. Total number of choices of highly rewarded stimuli (our measure of associative learning) is shown on the vertical axis, while block is depicted on the horizontal axis. Individuals who had suffered early adversity are displayed as light gray, while participants in the comparison sample are shown in black. Group means and standard error bars are shown. We found adolescents who suffered early adversity had poorer associative learning over time compared to our comparison sample (Group × Block F(4,316) = 2.92, p = .020)
As an additional measure of learning, we also employed linear mixed effects models to calculate the slope of learning across the experiment. We employed similar procedures to those noted above, except we modeled participant as a random factor and included a random intercept for each participant. In addition, we did not include group in these models, instead extracting slopes for the number of highly rewarded stimuli for each participant. We then entered these slopes into regression models with group (early adversity group; comparison group with no such history), age, gender, and general cognitive ability as independent variables. Similar to the analyses noted above, these analyses found group was significantly related to these individual slopes (β = −.438, p < .001; η2 = .19), with adolescents exposed to early adversity having smaller slopes. This pattern can be interpreted as lower associative learning for these youth, as lower values would indicate less change in the number of highly rewarded stimuli over time. All results were similar if regression models did not include general cognitive ability as an additional covariate.
Relations between associative learning and behavioral problems
To determine if associative learning was related to behavioral problems, we constructed linear regression models with the combined number of choices entered as the independent variable and ratings of behavioral problems entered as the dependent variable. Next, we used path analyses to test whether early adversity (X) was associated with behavioral problems (Y) and whether the observed association was mediated by individual differences in associative learning (M). Statistical testing of mediation was done using nonparametric bootstrapping in R, with 95% confidence intervals for indirect (a × b) effects.
Early adversity was associated with higher levels of behavioral problems (YLSI behavioral score – early adversity group mean = 2.15, standard deviation = 1.34; comparison group mean = 1.36, standard deviation = .88; β = .33, p = .003; η2 = .11). Moreover, as shown in Figure 3, individuals with poorer associative learning had more behavioral problems (β = −.45, p < .001; η2 = .2). Again, this effect remained significant when controlling for race (β = −.42, p < .001; η2 = .17). Analyses using slopes of learning yielded similar effects, with smaller slopes being related to greater levels of behavioral problems (β = −.45, p < .001; η2 = .2). All results were similar if we did not include general cognitive ability as an additional covariate.
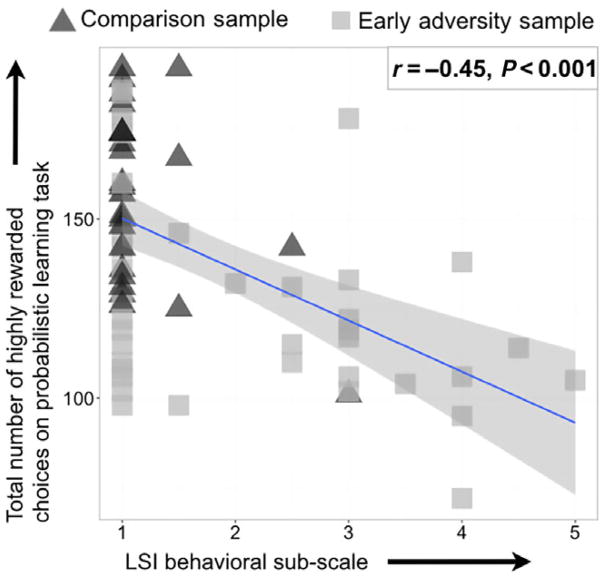
This scatterplot shows the behavioral subscale of the LSI on the horizontal axis and the number of highly rewarded stimuli in our learning task. Higher scores on the behavioral subscale of the LSI indicate greater behavioral problems, while a high number of rewarded choices in the learning task indicate better associative learning. Participants in the comparison sample are shown in black triangles, while individuals who suffered early adversity are displayed as light gray squares. As hypothesized, poorer associative learning was related to greater behavioral problems (β = −.45, p < .001). A similar relation was also seen if only participants who had suffered early adversity were examined (β = −.36, p = .020) [Colour figure can be viewed at wileyonlinelibrary.com]
To test our hypothesized pathway, we examined whether individual differences in associative learning mediated the relation between early adversity and behavioral problems. When associative learning was entered into this model, the relation between adversity and behavioral problems fell to β = .15. In support of our hypothesis, associative learning accounted for 51.4% of the relation between early adversity and behavioral problems (variance mediated by the differences in associative learning = .51; 95% confidence interval = 0.23–1.13, p = .002; η2 = .112; covariates = age, race, gender, and general cognitive ability; shown in Figure 4). Again, analyses using slopes of learning yielded similar effects, with smaller slopes partially mediating the higher levels of behavioral problems seen in youth exposed to early adversity (variance mediated by the differences in associative learning = 0.492; 95% confidence interval = 0.22–0.91, p < .001; η2 = .108; covariates = age, gender, and general cognitive ability). These results were similar if we did not include general cognitive ability as an additional covariate.
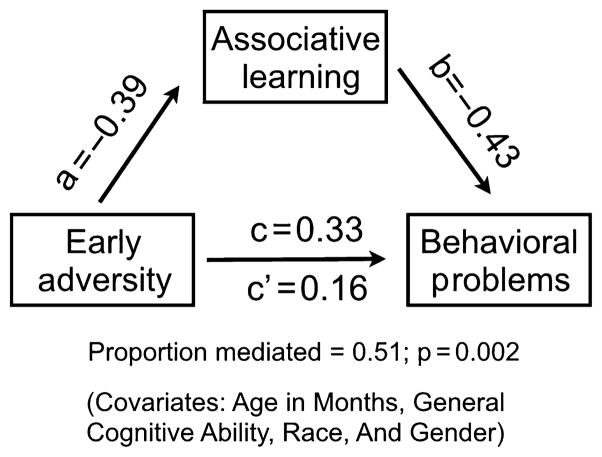
Statistical mediation models are shown in this figure. In line with past reports, we find a significant relation between early adversity and behavioral problems (β = .33, p = .003; the c path). However, this relation becomes nonsignificant when associative learning is added to our nonparametric bootstrapped mediation models (β = .16, p = .16; the c’ path)
Group differences in learning dynamics
Based upon the observed group differences, we sought to better understand how children may be processing and using information from the environment and how such factors may be influenced by early adversity. Because children who had suffered early adversity are often exposed to unpredictable environments, we examined differences in their initial expectations about rewards in the experiment and their ability to consistently use this information. To investigate biases in the decision-making based on the likelihood and consistency of positive feedback during the task, we focused on the decision weights from our mathematical models at the beginning of each experimental run. We tested this parameter for each experimental run separately given that learning in run 1 would likely influence expectations for the stimuli in run 2. Using nonparametric Mann–Whitney statistical tests, we found physically abused adolescents made decisions in a biased way when starting the experiment (early adversity group median = 0.20; comparison median = 0.62; β = −.41, p = .009, η2 = .13; in run 1). Such bias indicates a belief that rewards were less consistent and occurred more at random. At the beginning of the second block, this pattern was no longer present, as this parameter was similar between groups (early adversity group median = .34, comparison group median = .58; β = −.07, t = −1.02, p = .310). Next, to test potential differences in choice variability, we employed linear regression models to compare our groups on the stochasticity parameter (β) derived from our mathematical models. These analyses revealed that adolescents who suffered early adversity used information about known rewards in their environments less often (stochasticity parameter β from our decision-models, early adversity group median = 5.61, comparison median = 7.20; β = −.30, p = .01, η2 = .02). Again, these results were similar if we did not include general cognitive ability as an additional covariate.
Discussion
The goal of this study was to understand if general learning mechanisms could account for the effects of early adversity on the development of behavior problems. We examined a group of adolescents who had experienced physical abuse in their early childhoods. As expected, youths who suffered this early adversity had higher rates of behavioral problems than a community sample of their peers. The data from this study provides new insights into why these behavioral problems arise. First, the participants who had been exposed to early adversity were less able than their peers to correctly learn which stimuli were likely to result in reward, even after repeated feedback. This difficulty discerning and using information from the environment explained the higher levels of behavioral problems in participants exposed to early adversity, and was maintained even after statistically controlling for adolescents’ general cognitive abilities. Second, our study also suggests why individuals exposed to early adversity had difficulty learning associations between stimuli and reward. That is, these individuals used information about known rewards in their environments less often. In addition, individuals exposed to adversity made decisions early in the learning process as if rewards were less consistent and occurred more at random.
Thinking about the broad phenomenology of early adverse environments, children living in such contexts interact with caregivers who often act inconsistently and vacillate between extreme emotional states (Milner & Robertson, 1989). Given these patterns, it may be hard for a child to predict a caregiver’s emotional responses over time. With high variability in caregiver responses, it may be adaptive in that context to presume positive feedback and rewards are less consistent and occur more at random. Indeed, research in decision-making has found that greater variability (operationalized, as lower exploitation) is more optimal in fast-changing, unpredictable environments (Daw, O’Doherty, Dayan, Seymour, & Dolan, 2006). Children who suffered early adversity may then apply this learning in other contexts, failing to use information about rewards structures and instead accessing behavioral patterns commonly deployed in their volatile home environments. As a result of this disregard for environmental cues, these children may be more likely to engage in impulsive and dysregulated behavior that leads to social transgressions and creates stressful contexts outside of the home.
Our results connect to previous studies on early adversity, and also integrate more recent theoretical perspectives underscoring the importance of dimensional measures of well-being. For example, a great deal of past research has concentrated on the links between forms of abuse and conduct disorder (Dodge, Pettit, Bates, & Valente, 1995). Our results suggest general learning processes may explain aggressive and oppositional behavior in adolescence after exposure to such adversity. However, differences in general learning processes may also explain outcomes in other domains of functioning. A number of reports have recently surfaced finding differences in associative learning in adolescents at-risk for and diagnosed with major depression (Forbes & Dahl, 2012). Measures of mood were not available in this sample, but future studies could investigate links between early adversity, learning, and negative affect. Investigating dimensional processes, especially when coupled with strong measures of neurobiology, will be an important step forward in understanding the multitude of negative outcomes seen after different forms of early adversity. This fact may be particularly important given that different forms of early adversity have been found to impact brain areas related to associative learning (Hanson, Hariri, & Williamson, 2015; Hanson et al., 2016). Individual differences in general learning when coupled with information from genes, neural circuits, physiology, and self-report data could aid in understanding the differential impact of early adversity on development.
While adolescents in this study experienced physical abuse during childhood, there is heterogeneity in the characteristics, timing, and duration of this early adversity. In this study, early adversity was theorized to relate to environmental volatility, but we did not directly measure specific characteristics of the home environment. Tying individual differences in environmental features such as specific parenting practices and the child’s perceptions of unpredictability in the home would be particularly powerful moving forward. Having these and other measures, such as richly coded social services reports, could aid in understanding connections between child maltreatment, learning, and negative outcomes. Related to this, general learning systems may be influenced by other forms of adversity, such as early institutionalization or extreme poverty. A starting framework (Humphreys & Zeanah, 2014; Sheridan & McLaughlin, 2014) argues for the difference between deprivation (absence of expected cognitive and social input) and threat (presence of a threat to one’s physical integrity) in early adversity. Discerning between the effects of deprivation and threat, as well as environmental consistency, may aid in cleaner dissociations of neurodevelopmental trajectories of learning processes and the sequelae of early adversity.
The current study has several limitations, which suggest important future directions. First, our study was cross-sectional in nature and therefore prevents us from establishing causal patterns. Longitudinal designs, with additional assessments of multiple neurocognitive functions, could aid in more strongly elucidating the role of general learning in accounting for the negative outcomes seen after early adversity. Second, the task employed here is only one example and form of associative learning. Associative learning encompasses a broad array of behaviors including the receipt of reward, aspects of verbal learning, social learning, and understanding social relationships. While we accounted for a significant amount of variance in behavioral problems, broader assessment of these other related processes could have improved our ability to explain the link between adversity and behavior problems.
Limitations notwithstanding, our study demonstrated that alterations in general forms of learning begin to explain the association between early adversity and disruptive behavioral problems. Our work is grounded in the phenomenology of early adversity and provides a plausible pathway through which volatile caregiving environments could impact behavior. As such, our data suggest that associative learning is an important mechanism in need of further study, as we connect variations in such learning to behavioral problems in adolescents’ everyday lives. Furthermore, we unpacked how specific components of learning, such as bias in choice and use of known information, are shaped by early adversity. This suggests novel targets of investigation to understand how the early environment may create vulnerabilities for later negative outcomes. More broadly, this work begins to move beyond simple descriptive research to understand the ways in which social experience during early adolescence shapes behavioral development. Such a research program, if coupled with strong measures of neurobiology, could have profound implications for the development of interventions for at-risk children and adolescents.
Acknowledgments
This work was supported by the National Institute of Mental Health (MH61285 and MH68858 to S.D.P. and P50-MH84051 and MH43454 to R.J.D.), a National Institute of Drug Abuse Fellowship (DA028087 to J.L.H.), and a core grant to the Waisman Center Intellectual & Developmental Disabilities Research Center from the National Institute of Child Health and Human Development (P30-HD03352). J. L.H. is now in the Department of Psychology at the University of Pittsburgh. The authors have declared that they have no competing or potential conflicts of interest in relation to this article.
Footnotes
Conflict of interest statement: No conflicts declared.
Additional Supporting Information may be found in the online version of this article:
Appendix S1. Additional information on the mathematical modeling of learning.
References
- Behrens TEJ, Hunt LT, Woolrich MW, Rushworth MFS. Associative learning of social value. Nature. 2008;456:245–249. [Europe PMC free article] [Abstract] [Google Scholar]
- Bigelow A, DeCoste C. Sensitivity to social contingency from mothers and strangers in 2-, 4-, and 6-month-old infants. Infancy. 2003;4:111–140. [Google Scholar]
- van den Bos W, Cohen MX, Kahnt T, Crone EA. Striatum-medial prefrontal cortex connectivity predicts developmental changes in reinforcement learning. Cerebral Cortex. 2012;22:1247–1255. [Abstract] [Google Scholar]
- van den Bos W, Güroğlu B, van den Bulk BG, Rombouts SARB, Crone EA. Better than expected or as bad as you thought? The neurocognitive development of probabilistic feedback processing. Frontiers in Human Neuroscience. 2009;3:52. [Europe PMC free article] [Abstract] [Google Scholar]
- Bousha DM, Twentyman CT. Mother-child interactional style in abuse, neglect, and control groups: Naturalistic observations in the home. Journal of Abnormal Psychology. 1984;93:106–114. [Abstract] [Google Scholar]
- Capitanio JP. Early experience and social processes in rhesus macaques (Macaca mulatta): II. Complex social interaction. Journal of Comparative Psychology. 1985;99:133–144. [Abstract] [Google Scholar]
- Casey BJ, Oliveri ME, Insel T. A neurodevelopmental perspective on the research domain criteria (RDoC) framework. Biological Psychiatry. 2014;76:350–353. [Abstract] [Google Scholar]
- Cohen MX, Ranganath C. Behavioral and neural predictors of upcoming decisions. Cognitive, Affective, & Behavioral Neuroscience. 2005;5:117–126. [Abstract] [Google Scholar]
- Daw ND, O’Doherty JP, Dayan P, Seymour B, Dolan RJ. Cortical substrates for exploratory decisions in humans. Nature. 2006;441:876–879. [Europe PMC free article] [Abstract] [Google Scholar]
- Dodge KA, Pettit GS, Bates JE, Valente E. Social information-processing patterns partially mediate the effect of early physical abuse on later conduct problems. Journal of Abnormal Psychology. 1995;104:632–643. [Abstract] [Google Scholar]
- Ernst M, Grant SJ, London ED, Contoreggi CS, Kimes AS, Spurgeon L. Decision making in adolescents with behavior disorders and adults with substance abuse. American Journal of Psychiatry. 2003;160:33–40. [Abstract] [Google Scholar]
- Forbes EE, Dahl RE. Research Review: Altered reward function in adolescent depression: What, when and how? Journal of Child Psychology and Psychiatry. 2012;53:3–15. [Europe PMC free article] [Abstract] [Google Scholar]
- Guyer AE, Kaufman J, Hodgdon HB, Masten CL, Jazbec S, Pine DS, Ernst M. Behavioral alterations in reward system function the role of childhood maltreatment and psychopathology. Journal of the American Academy of Child and Adolescent Psychiatry. 2006;45:1059–1067. [Abstract] [Google Scholar]
- Hammock EA, Levitt P. The discipline of neurobehavioral development: The emerging interface of processes that build circuits and skills. Human Development. 2006;49:294–309. [Google Scholar]
- Hanson JL, Albert D, Iselin AMR, Carré JM, Dodge KA, Hariri AR. Cumulative stress in childhood is associated with blunted reward-related brain activity in adulthood. Social Cognitive and Affective Neuroscience. 2016;11:405–412. [Europe PMC free article] [Abstract] [Google Scholar]
- Hanson JL, Chung MK, Avants BB, Shirtcliff EA, Gee JC, Davidson RJ, Pollak SD. Early stress is associated with alterations in the orbitofrontal cortex: A tensor-based morphometry investigation of brain structure and behavioral risk. Journal of Neuroscience. 2010;30:7466–7472. [Europe PMC free article] [Abstract] [Google Scholar]
- Hanson JL, Hariri AR, Williamson DE. Blunted ventral striatum development in adolescence reflects emotional neglect and predicts depressive symptoms. Biological Psychiatry. 2015;78:598–605. [Europe PMC free article] [Abstract] [Google Scholar]
- Hanson JL, Nacewicz BM, Sutterer MJ, Cayo AA, Schaefer SM, Rudolph KD, … Davidson RJ. Behavioral problems after early life stress: Contributions of the hippocampus and amygdala. Biological Psychiatry. 2015;77:314–323. [Europe PMC free article] [Abstract] [Google Scholar]
- Hein G, Engelmann JB, Vollberg MC, Tobler PN. How learning shapes the empathic brain. Proceedings of the National Academy of Sciences of the United States of America. 2016;113:80–85. [Europe PMC free article] [Abstract] [Google Scholar]
- Humphreys KL, Zeanah CH. Deviations from the expectable environment in early childhood and emerging psychopathology. Neuropsychopharmacology. 2014;40:153–169. [Europe PMC free article] [Abstract] [Google Scholar]
- Lyons-Ruth K, Block D. The disturbed caregiving system: Relations among childhood trauma, maternal caregiving, and infant affect and attachment. Infant Mental Health Journal. 1996;17:257–275. [Google Scholar]
- Mason WA, Capitanio JP. Formation and expression of filial attachment in rhesus monkeys raised with living and inanimate mother substitutes. Developmental Psychobiology. 1988;21:401–430. [Abstract] [Google Scholar]
- Mason WA, Kenney MD. Redirection of filial attachments in rhesus monkeys: Dogs as mother surrogates. Science. 1974;183:1209–1211. [Abstract] [Google Scholar]
- McClure SM, Berns GS, Montague PR. Temporal prediction errors in a passive learning task activate human striatum. Neuron. 2003;38:339–346. [Abstract] [Google Scholar]
- Milner JS, Robertson KR. Inconsistent response patterns and the prediction of child maltreatment. Child Abuse & Neglect. 1989;13:59–64. [Abstract] [Google Scholar]
- Montague PR, Hyman SE, Cohen JD. Computational roles for dopamine in behavioural control. Nature. 2004;431:760–767. [Abstract] [Google Scholar]
- Mueller SC, Hardin MG, Korelitz K, Daniele T, Bemis J, Dozier M, … Ernst M. Incentive effect on inhibitory control in adolescents with early-life stress: An antisaccade study. Child Abuse & Neglect. 2012;36:217–225. [Europe PMC free article] [Abstract] [Google Scholar]
- Papoušek H, Papoušek M. Cognitive aspects of preverbal social interaction between human infants and adults. Ciba Foundation Symposium 33-Parent-Infant Interaction. 1975:241–269. [Abstract] [Google Scholar]
- Pechtel P, Pizzagalli DA. Effects of early life stress on cognitive and affective function: An integrated review of human literature. Psychopharmacology (Berl) 2011;214:55–70. [Europe PMC free article] [Abstract] [Google Scholar]
- Pechtel P, Pizzagalli DA. Disrupted reinforcement learning and maladaptive behavior in women with a history of childhood sexual abuse: A high-density event-related potential study. JAMA Psychiatry. 2013;70:499–507. [Europe PMC free article] [Abstract] [Google Scholar]
- Pollak SD. Developmental psychopathology: Recent advances and future challenges. World Psychiatry. 2015a;14:262–269. [Europe PMC free article] [Abstract] [Google Scholar]
- Pollak SD. Multilevel developmental approaches to understanding the effects of child maltreatment: Recent advances and future challenges. Development and Psychopathology. 2015b;27(4pt2):1387–1397. [Europe PMC free article] [Abstract] [Google Scholar]
- Reeb-Sutherland BC, Levitt P, Fox NA. The predictive nature of individual differences in early associative learning and emerging social behavior. PLoS ONE. 2012;7:e30511. [Europe PMC free article] [Abstract] [Google Scholar]
- Rescorla RA. Behavioral studies of Pavlovian conditioning. Annual Review of Neuroscience. 1988;11:329–352. [Abstract] [Google Scholar]
- Rudolph KD, Hammen C. Age and gender as determinants of stress exposure, generation, and reactions in youngsters: A transactional perspective. Child Development. 1999;70:660–677. [Abstract] [Google Scholar]
- Rudolph KD, Hammen C, Burge D, Lindberg N, Herzberg D, Daley SE. Toward an interpersonal life-stress model of depression: The developmental context of stress generation. Development and Psychopathology. 2000;12:215–234. [Abstract] [Google Scholar]
- Shackman JE, Pollak SD. Experiential influences on multimodal perception of emotion. Child Development. 2005;76:1116–1126. [Abstract] [Google Scholar]
- Shalev I, Moffitt TE, Sugden K, Williams B, Houts RM, Danese A, … Capsi A. Exposure to violence during childhood is associated withtelomere erosion from 5 to 10 years of age: A longitudinal study. Molecular Psychiatry. 2012;18:576–581. [Europe PMC free article] [Abstract] [Google Scholar]
- Sheridan MA, McLaughlin KA. Dimensions of early experience and neural development: Deprivation and threat. Trends in Cognitive Sciences. 2014;18:580–585. [Europe PMC free article] [Abstract] [Google Scholar]
- Straus MA, Hamby SL, Finkelhor D, Moore DW, Runyan D. Identification of child maltreatment with the Parent-Child Conflict Tactics Scales: Development and psychometric data for a national sample of American parents. Child Abuse & Neglect. 1998;22:249–270. [Abstract] [Google Scholar]
- Vachon DD, Krueger RF, Rogosch FA, Cicchetti D. Assessment of the harmful psychiatric and behavioral effects of different forms of child maltreatment. JAMA Psychiatry. 2015;72:1135–1142. [Europe PMC free article] [Abstract] [Google Scholar]
- Wahler RG, Dumas JE. Maintenance factors in coercive mother-child interactions: The compliance and predictability hypotheses. Journal of Applied Behavior Analysis. 1986;19:13–22. [Europe PMC free article] [Abstract] [Google Scholar]
- Watson JB, Rayner R. Conditioned emotional reactions. Journal of Experimental Psychology. 1920;3:1–14. [Google Scholar]
- Weller JA, Fisher PA. Decision-making deficits among maltreated children. Child Maltreatment. 2013;18:184–194. [Europe PMC free article] [Abstract] [Google Scholar]
- Wilson DA, Sullivan RM. Neurobiology of associative learning in the neonate: Early olfactory learning. Behavioral and Neural Biology. 1994;61:1–18. [Abstract] [Google Scholar]
Full text links
Read article at publisher's site: https://doi.org/10.1111/jcpp.12694
Read article for free, from open access legal sources, via Unpaywall:
https://europepmc.org/articles/pmc5474156?pdf=render
Citations & impact
Impact metrics
Article citations
Validation of the Argentine version of the epistemic trust, mistrust, and credulity questionnaire.
PLoS One, 19(10):e0311352, 03 Oct 2024
Cited by: 0 articles | PMID: 39361610 | PMCID: PMC11449354
Neural and immune interactions linking early life stress and anhedonia.
Brain Behav Immun Health, 42:100881, 30 Sep 2024
Cited by: 0 articles | PMID: 39415844 | PMCID: PMC11480252
How adverse childhood experiences get under the skin: A systematic review, integration and methodological discussion on threat and reward learning mechanisms.
Elife, 13:e92700, 16 Jul 2024
Cited by: 2 articles | PMID: 39012794 | PMCID: PMC11251725
Review Free full text in Europe PMC
The effects of trauma on feedback processing: an MEG study.
Front Neurosci, 17:1172549, 02 Nov 2023
Cited by: 0 articles | PMID: 38027493 | PMCID: PMC10651751
Childhood unpredictability and the development of exploration.
Proc Natl Acad Sci U S A, 120(49):e2303869120, 27 Nov 2023
Cited by: 8 articles | PMID: 38011553 | PMCID: PMC10710094
Go to all (56) article citations
Data
Data behind the article
This data has been text mined from the article, or deposited into data resources.
BioStudies: supplemental material and supporting data
Similar Articles
To arrive at the top five similar articles we use a word-weighted algorithm to compare words from the Title and Abstract of each citation.
Ventral striatal activity links adversity and reward processing in children.
Dev Cogn Neurosci, 26:20-27, 15 Apr 2017
Cited by: 16 articles | PMID: 28436832 | PMCID: PMC6987763
The Computational Development of Reinforcement Learning during Adolescence.
PLoS Comput Biol, 12(6):e1004953, 20 Jun 2016
Cited by: 54 articles | PMID: 27322574 | PMCID: PMC4920542
Early deprivation disruption of associative learning is a developmental pathway to depression and social problems.
Nat Commun, 9(1):2216, 07 Jun 2018
Cited by: 45 articles | PMID: 29880851 | PMCID: PMC5992195
Decision-making in the adolescent brain.
Nat Neurosci, 15(9):1184-1191, 28 Aug 2012
Cited by: 285 articles | PMID: 22929913
Review
Funding
Funders who supported this work.
Intellectual and Developmental Disabilities Research Center
NICHD NIH HHS (1)
Grant ID: P30 HD003352
NIDA NIH HHS (1)
Grant ID: F31 DA028087
NIMH NIH HHS (6)
Grant ID: P50 MH084051
Grant ID: P50 MH100031
Grant ID: R01 MH061285
Grant ID: R37 MH043454
Grant ID: R01 MH043454
Grant ID: R01 MH068858
National Institute of Child Health and Human Development (1)
Grant ID: P30‐HD03352
National Institute of Mental Health (4)
Grant ID: MH68858
Grant ID: P50‐MH84051
Grant ID: MH43454
Grant ID: MH61285
National Institute on Drug Abuse (1)
Grant ID: DA028087