Abstract
Study objectives
To improve sleep quality assessment using a single chest-worn sensor by extracting body acceleration and sleep position changes.Methods
Sleep patterns of 21 participants (50.8 ± 12.8 years, 47.8% female) with self-reported sleep problems were simultaneously recorded using a chest sensor (Chest), an Actiwatch (Wrist), and polysomnography (PSG) during overnight sleep laboratory assessment. An algorithm for Chest was developed to detect sleep/wake epochs based on body acceleration and sleep position/postural changes data, which were then used to estimate sleep parameters of interest. Comparisons between Chest and Wrist with respect to PSG were performed. Identification of sleep/wake epochs was assessed by estimating sensitivity, specificity, and accuracy. Agreement between sensor-derived sleep parameters and PSG was assessed using correlation coefficients and Bland-Altman analysis.Results
Chest identified sleep/wake epochs with an accuracy of on average 6% higher than Wrist (85.8% versus 79.8%). Similar trends were observed for sensitivity/specificity values. Correlation between Wrist and PSG was poor for most of the sleep parameters of interest (r = 0.0-0.3); however, Chest and PSG correlation showed moderate to strong agreement (r = 0.4-0.8) with relatively low bias and high precision bias (precision): 9.2 (13.2) minutes for sleep onset latency; 17.3(34.8) minutes for total sleep time; 7.5 (29.8) minutes for wake after sleep onset; and 2.0 (7.3)% for sleep efficacy.Conclusions
Combination of sleep postural/position changes and body acceleration improved detection of sleep/wake epochs compared to wrist acceleration alone. The chest sensors also improved estimation of sleep parameters of interest with stronger agreement with PSG. Our findings may expand the application of wearable sensors to clinically assess sleep outside of a sleep laboratory.Free full text

Improving Sleep Quality Assessment Using Wearable Sensors by Including Information From Postural/Sleep Position Changes and Body Acceleration: A Comparison of Chest-Worn Sensors, Wrist Actigraphy, and Polysomnography
Associated Data
Abstract
Study Objectives:
To improve sleep quality assessment using a single chest-worn sensor by extracting body acceleration and sleep position changes.
Methods:
Sleep patterns of 21 participants (50.8 ± 12.8 years, 47.8% female) with self-reported sleep problems were simultaneously recorded using a chest sensor (Chest), an Actiwatch (Wrist), and polysomnography (PSG) during overnight sleep laboratory assessment. An algorithm for Chest was developed to detect sleep/wake epochs based on body acceleration and sleep position/postural changes data, which were then used to estimate sleep parameters of interest. Comparisons between Chest and Wrist with respect to PSG were performed. Identification of sleep/wake epochs was assessed by estimating sensitivity, specificity, and accuracy. Agreement between sensor-derived sleep parameters and PSG was assessed using correlation coefficients and Bland-Altman analysis.
Results:
Chest identified sleep/wake epochs with an accuracy of on average 6% higher than Wrist (85.8% versus 79.8%). Similar trends were observed for sensitivity/specificity values. Correlation between Wrist and PSG was poor for most of the sleep parameters of interest (r = 0.0–0.3); however, Chest and PSG correlation showed moderate to strong agreement (r = 0.4–0.8) with relatively low bias and high precision bias (precision): 9.2 (13.2) minutes for sleep onset latency; 17.3(34.8) minutes for total sleep time; 7.5 (29.8) minutes for wake after sleep onset; and 2.0 (7.3)% for sleep efficacy.
Conclusions:
Combination of sleep postural/position changes and body acceleration improved detection of sleep/wake epochs compared to wrist acceleration alone. The chest sensors also improved estimation of sleep parameters of interest with stronger agreement with PSG. Our findings may expand the application of wearable sensors to clinically assess sleep outside of a sleep laboratory.
Citation:
Razjouyan J, Lee H, Parthasarathy S, Mohler J, Sharafkhaneh A, Najafi B. Improving sleep quality assessment using wearable sensors by including information from postural/sleep position changes and body acceleration: a comparison of chest-worn sensors, wrist actigraphy, and polysomnography. J Clin Sleep Med. 2017;13(11):1301–1310.
INTRODUCTION
Sleep quality is an important measure of health. In-laboratory polysomnography (PSG) is considered the “gold standard” for measuring sleep quality parameters, such as total sleep time, sleep efficiency, and sleep stages.1 PSG is affected by key limiting factors including high cost, arduous application, intrusiveness to sleep, and the requirement of a sleep laboratory and its dedicated infrastructure.2
There has been growing interest in wearable sensors, such as the wrist actigraph, as potential alternatives to PSG to examine clinically relevant sleep quality parameters. In addition to their user-friendliness and low cost, wearable sensors allow for clinical sleep assessment in the home setting, which may better emulate real conditions than in a sleep laboratory. However, current wearable devices may not be capable of extracting sleep parameters of interest with sufficient accuracy, compared to the gold standard PSG.3 This limits the application of wearable devices for clinical sleep assessment outside of a sleep laboratory setting, in particular for tracking changes in sleep parameters of interest and/or as a function of a sleep disorder.
Among wearable devices for sleep assessment, wrist accelerometer sensors are the most popular sensors because of low cost, ease of wearing, and currently validated algorithms, tailored for acceleration level measured from wrist body location, to extract sleep parameters of interest.4,5 Most wrist accelerometer sensors use the popular method of sleep quality assessment based on measuring body acceleration due to movement within an inertial frame.6–9 Sleep quality parameters of interest are typically estimated based on detection of sleep/wake epochs using body acceleration patterns.
To improve the accuracy of awake/sleep epoch assessment, several signal processing schemes have been proposed.8–10 However, these signal processing methods mainly identify sleep/wake epochs based on inertial acceleration (ie, acceleration due to movement) without taking into account information from static acceleration (ie, acceleration due to gravity). Thus, these methods neglect valuable information related to sleep position and/or changes between sleep positions, measurable from static acceleration, if the sensor is placed on specific body segment (eg, chest, leg, or waist).11,12 This may explain the poor specificity obtained from wrist sensors to identify sleep/ wake patterns, in the range of less than 50% according to the literature.4–6,9 The poor specificity causes overestimation of sleep duration, thus reducing the ability of identifying those with true sleep problems.13,14
Although several studies have explored the accuracy of wearable sensors on different body locations, such as the hip,4,5 these studies often neglect to update the algorithm and/or include information from body postural changes as a sleep parameter of interest, and thus the alternative sensor placement often yielded lower accuracy than wrist-based sensors.
This study describes a proposed novel algorithm based on body acceleration plus body postural changes to estimate sleep parameters of interest using a single chest-worn sensor based on accelerometry. We compared the performance of the chest sensor using the proposed algorithm at home with that of PSG in a sleep laboratory to accurately estimate sleep parameters of interest among a sample of patients who suffer from sleep deprivation. We evaluated the additional benefit of sleep postural changes for sleep assessment by comparing the results from the chest sensor versus Actiwatch, a validated wrist sensor for sleep assessment, using PSG as the gold standard.
METHODS
We have described our algorithm subcomponents and the study design to evaluate accuracy of each subcomponent in the following sections. In brief, to evaluate the accuracy of wearable sensors' assessment of sleep quality, we first tested data on detection of sleep/wake epochs from a chest sensor and wrist sensor as compared to PSG. Then, sleep quality parameters of interest were estimated using a standard approach,4,15 in which detection of sleep/wake epoch is a key ingredient. Finally, Bland-Altman plots were used to examine bias and precision of wearable sensors to evaluate the ability of wearable sensors to assess sleep quality parameters of interest.
Study Population
Participants in this study were recruited from patients referred to the Banner University Medical Center, Tucson Center for Sleep Disorders for evaluation in Tucson, Arizona. The study was approved by the Institutional Review Board (No: 13-0651), and written informed consent according to the principles expressed in the Declaration of Helsinki was obtained from all participants before participation. Participants were informed that they could withdraw from the study at any time without loss of benefits. Participants with a pacemaker or implantable cardioverter defibrillator were excluded.
Clinical Assessment
Participants' basic demographic data were collected at clinical examination prior to the sleep study. As part of in-laboratory clinical sleep quality assessment participants' overall sleep quality was measured using the Pittsburgh Sleep Quality Index (PSQI)16 and presleep and postsleep questionnaires were collected from participants.
Polysomnography
We conducted overnight PSG on the participants according to the standards of the American Academy of Sleep Medicine, to compare the performance of the wrist device and chest device.1 The participants arrived 1.5 hours prior to the start of PSG recording and underwent instrumentation for the electrodes and sensors to record sleep-related data. The following signals were recorded from each participant: 4 electroencephalography channels (1 frontal, 1 occipital, 2 central), 2 electrooculography channels, 2 electromyography channels (mentalis/submentalis, tibialis), nasal airflow, snoring, respiratory efforts (thoracic and abdominal), pulse oximetry, body position, and a single-lead electrocardiograph (ECG). All data were transferred to the station, where the sleep laboratory technician monitored the individuals. The data were collected from 2 PSG devices: Comet-PLUS XL (Grass Technologies, West Warwick, Rhode Island, United States) and Embla S4500 (Embla Systems, Inc., Broom-field, Colorado, United States). The PSG outcomes were documented every 30 seconds for different sleep stages (30-second epochs).
To assess the performance between wrist and chest sensors, we transferred the 30-second epochs to 1-minute epochs by preferring the wake score6,7 because the wrist sensor only provided 1-minute epochs: if in 1 minute, at least 1 of the epochs was wake, we labeled that minute as awake; otherwise it was labeled as sleep according to previous literature.6,7,10
PSG is used to quantify the apnea-hypopnea index (AHI) to assess the severity of sleep apnea syndrome.15,17 The AHI is defined as the average number of apnea or hypopnea events per hour to quantify the apnea severity.15,17 Apnea is identified as the absence of airflow (≥ 90% of baseline) for 10 seconds or longer. In this study, we defined hypopneas as 50% or greater reduction in airflow lasting for 10 seconds or longer with a 3% desaturation or an arousal.15,17 The AHI is classified as: normal (AHI < 5), mild (5 ≤ AHI < 15), moderate (15 ≤ AHI < 30), and severe (AHI ≥ 30) OSA, respectively.15
Wrist Sensor
An Actiwatch-L (CamNtech Ltd, Cambridgeshire, United Kingdom) wrist sensor (Wrist) was attached to the dominant wrist to report sleep/wake epochs (with 1-minute resolution) and sleep parameters. The Actiwatch recorded activity counts, and its software (Action4, Ambulatory Monitoring Inc., Ardsley, New York, United States) reported sleep parameters that were developed and validated in children and the adult population.18 To score 1-minute epochs we used Action4 version 1.16 with the Cole-Kripke setting.7
Chest Sensor
A chest sensor (Chest) (BioPatch ZephyrLife; Annapolis, Maryland, United States) was attached to the sternum between the sternal notch and xiphisternum in patients with 2 standard ECG electrodes. The device can measure ECG (250 Hz), three-dimensional accelerations (100 Hz), temperature (1 Hz), and breathing rate (25 Hz). We used the acceleration data to detect sleep postures. The raw accelerations data were transferred to the MATLAB software (MathWorks Inc., Natick, Massachusetts, United States) for further analysis. Because the temporal synchronization between Wrist, Chest, and PSG was essential,19 we calibrated the timing of Chest and Wrist on the same computer as PSG.
Algorithm
An algorithm for analyzing the accelerations data from the chest sensor was developed using the following steps:
PREPROCESSING: The raw three-dimensional acceleration data were processed with a band-pass filter at cutoff frequencies of 0.1953 Hz and 12.5 Hz to remove the gravity component and high-frequency noise that was not originated from human body movements.20,21 Figure S1 in the supplemental material describes the flowchart of body acceleration estimation. In summary, a vector magnitude (VM) of the filtered acceleration data was calculated for each sample (sample frequency: 100 Hz). The formula for calculating the VM is as follows:

where accV2, accL2, and accF2 are the acceleration value in each direction of vertical, lateral, and frontal, respectively. Then, the maximum value of the VM for each second was calculated.7 To avoid outliers, values below the 5th percentile value (0.02 g) and greater than the 95th percentile value (0.2 g) were replaced with 0.02 g and 0.2 g, respectively.20 Then the outputs were normalized to range from zero to 1 (NmVM). The body acceleration (BAcc) is defined as the sum of NmVM values at every minute (Figure S1).
FEATURE SELECTION: We extracted 43 features from the BAcc: the value itself at every epoch (feature #1); mean, maximum, standard deviation (SD) and natural logarithm of SD of adjacent 1 to 10 minutes at both sides (3, 5, 7,…21-minute window) with the current epoch as the center (features #2–41); the percentage of recording progression at the current epoch (feature #42); the postural transition profile (ie, whether the participants had any sleep position changes or change of posture to upright position [not lying] at the current epoch [feature #43]). The postural transition profile was estimated from a previous validated algorithm.22
FEATURE EXTRACTION: A logistic regression model (conditional backward) was applied to the whole dataset to identify the independent predictors of sleep/wake identification. The dependent variable was wake/sleep for each epoch as identified by PSG. The independent variables were sensor-derived parameters as described previously. Overall, 8,862 epochs were included in the regression model, which included 1,907 awake, and the rest epochs were sleep. Using this model extracted from all measured epochs, a linear regression model from the independent variables was formed per subject named probability of sleep (PS):

where A0 is constant, Xi is independent sensor-derived features identified by the logistic regression model, and Ai is the coefficient of each feature estimated from the model.
To examine the accuracy of the model per subject, we used leave-one-out cross-validation,23 due to a small sample size. In this method, the training dataset includes all participants except one, who is selected as the validation dataset. This process is repeated for each participant so that each individual is selected as a validation subject against the model based on the rest of the participants. For the PS, we devised a threshold (T) to score each epoch as sleep or wake (if PS < T, then sleep, otherwise, wake). Traditionally, the threshold is identified based on accuracy rate (ACCU) as suggested by sleep studies.4,6,7,10,19,20,24 This identification of the threshold method yields the highest accuracy rate. We also used the Matthew correlation coefficients (MCC)25 approach to identify the best threshold, which is insensitive to inter-subject difference for the number of sleep/wake epochs. The chest sensor classification results estimated using ACCU approach was named ChestACCU and the classification results estimated using MCC approach was named ChestMCC.
All of the steps in this section applied to the 1-minute epochs. The same procedures were also performed for the 30-second epochs.
Statistical Analysis
To compare the performance of Chest and Wrist with PSG, we computed sensitivity as the proportion of correctly classified sleep epochs, specificity as the proportion of correctly classified wake epochs, and accuracy as the proportion of correctly classified sleep and wake epochs. These performance parameters were calculated for the entire dataset and across each patient, where the mean ± SD was reported. Then the Student t test was used to compare the performance of Wrist and Chest for each participant. The participants were classified into 2 groups of obese and nonobese based on the body mass index cutoff 30 kg/m2 as recommended by the World Health Organization.26 Between-group differences from point of view of performance (accuracy, sensitivity, and specificity) estimation per individual was examined using the Mann-Whitney U test.
For the sleep parameter analysis, we calculated 4 parameters:27 Sleep onset latency (SOL) was defined as the time difference from lights out until sleep onset. Total sleep time (TST) was defined as actual time spent asleep. Wake after sleep onset (WASO) was defined as total awakening time after sleep onset. Sleep efficiency (SE) was defined as percentage of time difference between sleep onset and final awakening, which was spent asleep. We assessed Pearson correlation with level of significance between PSG and wearable sensors. Based on Cohen strength of correlation,28 3 correlation levels were defined as follows: (1) weak (r: ± 0.10 to ± 0.29), (2) moderate (r: ± 0.30 to ± 0.49), and (3) strong (r: ± 0.50 to ± 1.0) correlation.
Additionally, Bland-Altman plots29 were used to assess absolute agreement (eg, bias and precision) between wearable sensors and PSG for those parameters of interest. A significant correlation was observed between sensor-derived parameters (using either method of estimation) and PSG. Bland-Altman analysis was performed by calculating the mean and SD of difference between sleep parameters reported from the wearable sensors and PSG. The mean difference between PSG and the sensors provided the bias, and the SD provided the precision. In the Bland-Altman plot, we also presented the upper and lower 95% limit of agreement (mean difference ± 1.96 × SD).
The agreement between perceived sleep quality (ie, PSQI score) and objective sleep quality (ie, ChestMCC, ChestACCU, and PSG) was examined using Spearman correlation coefficient (Table S2 in the supplemental material).
Using a binary logistics regression model and assuming PSG output (sleep/wake) as a dependent variable, we applied three models to predict sleep/wake epochs. The first model used was Wrist output, the second model used Chest output (ChestACCU), and the third model used a combination of Wrist and Chest outputs to predict true sleep/wake epochs as identified by PSG. The area under the curve (AUC) was used to compare the performance of each model.
All the analyses were performed with SPSS v.22 (IBM Corporation, Armonk, New York, United States) or MATLAB software.
RESULTS
Participant Characteristics
Twenty-three participants were recruited (50.8 ± 12.8 years, 48% female). Data for 2 participants were excluded due to clinical sleep lab staff error (the sensors were not turned on during recording). Data for 12 participants were recorded by the Embla S4500 system, and data for 11 participants were recorded by the Comet-PLUS XL system. Most participants' PSQI scores were greater than 5 (9.1 ± 4.2), indicating below-average or poor sleep quality. Mean AHI was 22.8 ± 26.5 events/h: 9% normal; 43% mild; 24% moderate; and 24% had severe apnea. A total of 7 patients (35%) had hypersomnia, 2 (9%) had respiratory abnormality, and 1 (5%) had periodic limb movements. On average, participants suffered from obesity, with an average body mass index of 36.7 ± 12.0 kg/m2 and approximately 69.6% were white or Caucasian. The summary of demographic and characteristic information is presented in Table 1.
Table 1
Summary of demographic information, sleep measures, and postural data; n = 21.
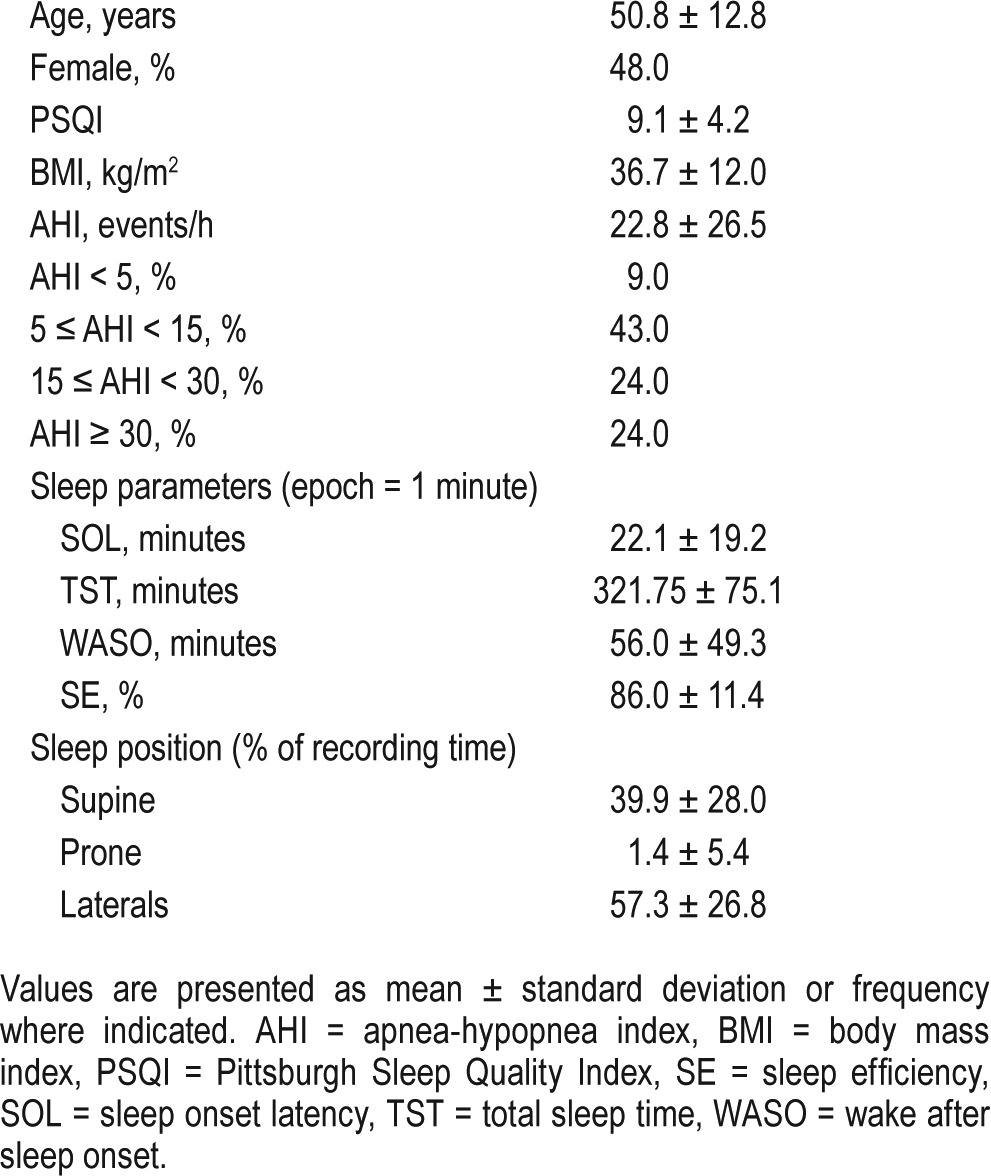
Chest Sensor Independent Variables
Overall, 8,862 epochs were recorded in this study from all participants, out of which 1,907 epochs were awake, and the rest were sleep epochs according to PSG. Out of 43 features, 10 significant independent features were extracted from the chest sensor using the logistic regression model as follows:
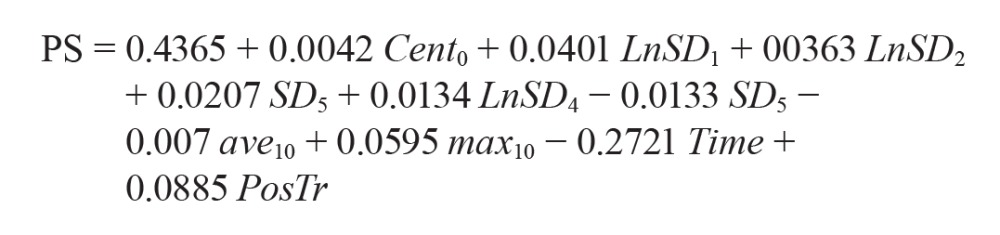
where Cent0 represents the value of body acceleration at each epoch; LnSD1, LnSD2, and LnSD4 represent the natural logarithm of the standard deviation of body acceleration estimated using a window size of 1, 2, and 4 epochs (before and after Cent0), respectively; SD5 and SD10 represent the standard deviation of body acceleration estimated using a window size of 5 and 10 epochs (before and after Cent0), respectively; ave7 represents the average of body acceleration estimated using a window size of 7 epochs (before and after Cent0); max10 represents the maximum value of body acceleration estimated using a window size of 10 epochs (before and after Cent0); Time represents the percentage of recording progression; and PosTr represents the presence of postural transition profile: 0 = no change, 1 = postural transition occurred.
Results From 1-Minute Epoch
The base rate of sleep was 78.0 ± 17.7 percent in our sample calculated from PSG. Table 2 summarizes the performance of Wrist, ChestMCC, and ChestACCU to detect sleep/wake epochs across all detected epochs, as identified by PSG using 1-minute epoch method (Table 2). Overall, Chest had higher performance (sensitivity 91.8% and 94.9; specificity 58.3% and 53.4%; accuracy 84.5% and 85.8% for ChestMCC, and ChestACCU, respectively) than Wrist (sensitivity = 90.9%, specificity = 41.5%, and accuracy = 79.8%). Similar trends were observed where inter-participant performance was estimated (Figure 1). On average the Chest sensitivity was 3.5% higher than Wrist (2% and 5% for ChestMCC and ChestACCU, respectively), and Chest specificity 32% (37% and 27% for ChestMCC and ChestACCU, respectively) and accuracy 6.5% (6% and 7% for ChestMCC, and ChestACCU respectively) were on average higher than Wrist. Across participants, ChestACCU had the highest sensitivity, and its specificity was 27% higher than Wrist (Figure 1). ChestMCC had the highest specificity, and its sensitivity was almost at the same level of Wrist, with half the SD of Wrist. ChestMCC and ChestACCU both had higher accuracy than Wrist, while their SD was lower than Wrist.
Table 2
Comparing different wearable sensors performance with the gold standard for all the participants; n = 21.
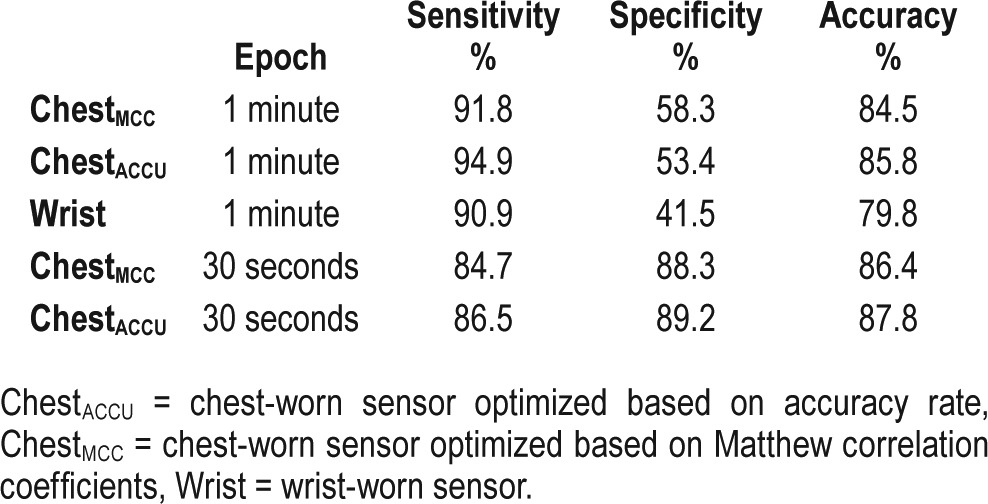
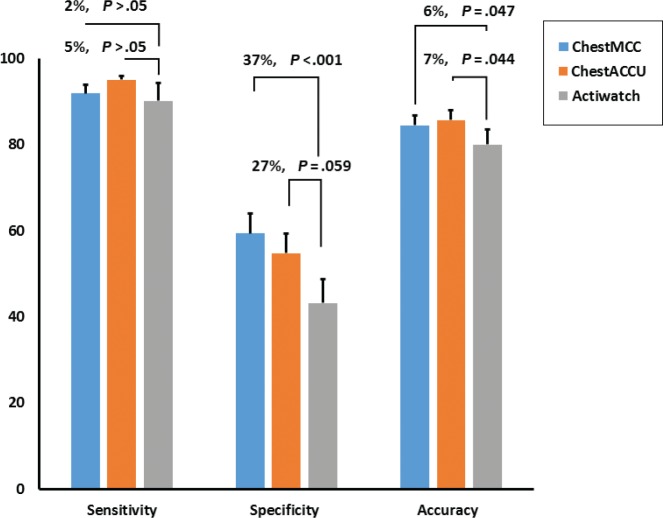
Pairwise comparison of individual sensitivity, specificity and accuracy in the chest sensor and the wrist sensor. Sensitivity represented epochs that the sensor correctly detected as sleep relative to gold standard. Specificity represented epochs that the sensor correctly detected as wake. Accuracy represented the rate of correct epoch detection. The wrist sensor represented by “Actiwatch” label, chest sensor algorithm optimized by Matthew correlation coefficient represented by “ChestMCC”, and chest sensor algorithm optimized by accuracy rate represented by “ChestACCU.”
The pairwise comparison of sensitivity showed no statistically significant difference between Wrist and ChestMCC or ChestACCU. However, there was a significant difference between specificity of Wrist and ChestMCC (37%, P < .001) and a similar trend with ChestACCU (27%, P = .059). The difference between Wrist and ChestMCC or ChestACCU accuracy was statistically significant, with a mean difference of 6% and 7%, respectively (Figure 1).
When participants were classified based on obesity status (obese [n = 7] versus nonobese status [n = 14]), no between-group difference was observed using either methods (ie, ChestMCC and ChestACCU) for accuracy (ChestMCC: P = .468, ChestACCU: P = .817) as well as for sensitivity (ChestMCC approach: P = .094, ChestACCU: P = .143). However, the estimated specificity was significantly lower in the obese group versus the nonobese group using either estimation methods (ChestMCC approach: P = .020, ChestACCU approach: P = .033).
Sleep Parameters of Interest
Using Pearson correlation analysis (Table 3), we evaluated Chest and Wrist sleep parameter results with those of PSG (Figure 2). Wrist sleep parameters, SOL, TST, WASO, and SE, had weak correlation with the gold standard method (P > .05). ChestACCU and ChestMCC were statistically significant and placed in strong correlation range. Both ChestACCU and ChestMCC had moderate correlation with SOL (P > .05). ChestACCU had higher correlation in sleep parameters such as TST, WASO, and SE. Overall, the optimized method based on accuracy had higher correlation (on average 29.4%) than the Matthew correlation coefficients approach in estimating sleep parameters.
Table 3
The Pearson correlation of sleep parameters between wearable sensors and the gold standard.
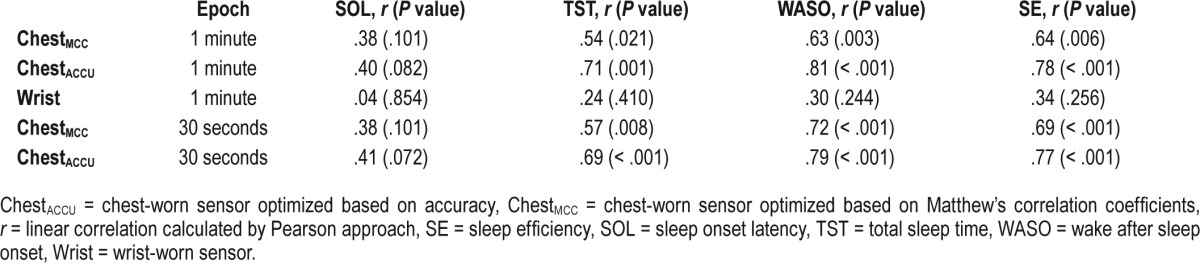
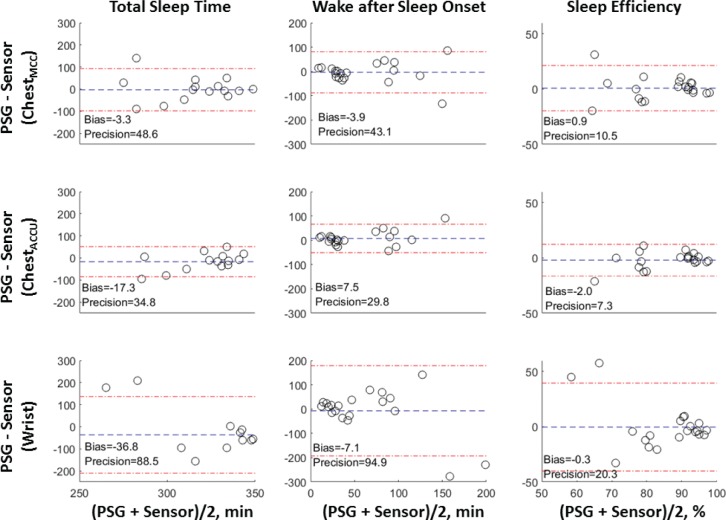
Bland-Altman plot of the sleep parameters (left column = total sleep time, middle column = wake after sleep onset, right column = sleep efficiency). The y-axis shows the difference of between gold standard (PSG) and sensor derived sleep parameters (PSG - Sensor). The x-axis shows the average of gold standard and sensor derived sleep parameters ([PSG + Sensor] / 2). The wrist sensor represented by “Wrist” label, chest sensor algorithm optimized by Matthew correlation coefficient represented by “ChestMCC”, and chest sensor algorithm optimized by accuracy rate represented by ChestACCU”. The blue lines (dash) show the average of the difference or bias. The red lines (dash and dot) show the average ± 1.96 × SD. The SD represents the precision. The lower values of bias and precision, the better agreement with the gold standard.
Using Bland-Altman analysis, we examined the agreement between the results of Chest (ChestACCU, ChestMCC) and Wrist with that of PSG (Figure 2 and Table S1 in the supplemental material). For SOL, there were no signifi-cant correlations between PSG and the sensors; therefore, the Bland-Altman analysis were not reported. The Bland-Altman analysis showed that Wrist overestimated TST by 36.8 minutes (95% CI −88.7 to 0.9, P = .014). When TST was estimated by ChestMCC, a nonsignificant bias value of 3.3 minutes (95% CI −25.5 to 20.0, P > .05) was observed. The value of bias was, however, significant when ChestACCU was used but was still less than Wrist (17.3 min, 95% CI −33.3 to −0.6, P = .043).
The agreement in the WASO parameter in Chest was higher than that in Wrist. Wrist biased WASO by 7.1 minutes (95% CI −54.2 to 35.2, P > .05) whereas the ChestMCC biased WASO by 3.9 minutes (95% CI −24.4 to 16.0, P > .05) and ChestACCU biased WASO by 7.5 minutes (95% CI −6.7 to 21.3, P > .05). Overall, the bias estimate in the ChestMCC or ChestACCU was lower than Wrist and its precision is at least twice as high.
Wrist biased SE by 0.3% (95% CI −9.4 to 9.7, P > .05), ChestMCC biased SE by 0.9% (95% CI −5.3 to 1.6, P > .05), and ChestACCU biased SE by 2.0% (95% CI −3.9 to 6.0, P > .05). The bias in Wrist was lower than that in ChestMCC, but Wrist precision was lower (50%).
In addition, the correlation between PSQI score and wearable-derived as well as PSG-derived parameters were explored, and no significant correlation was observed (Table S2 in the supplemental material).
Chest Sensor: 30-Second Epochs Versus 1-Minute Epochs
We explored the performance of our algorithm for 30-second versus 1-minute epochs. The rate of sensitivity was reduced to approximately 8% in 30-second epochs, but the rate of specificity was increased by 50% in 30-second epochs. The rate of accuracy stayed almost at the same level. The Pearson correlation improved slightly in 30-second epochs. The correlation of sleep parameters was statistically significant (P < .001), except for SOL, which had a moderate correlation (P = .07). The bias estimate in TST increased and the precisions also decreased. The bias in WASO stayed at the same level while the precision decreased. Interestingly, the bias in SE improved along with the precision.
Combining Outcomes from Wrist and Chest Sensors
The AUC for Wrist was 0.671, whereas the AUC for ChestACCU was 0.739 (10.1% improvement). The AUC for the combined model was 0.761, which showed 2.9% improvement in comparison with only Chest.
DISCUSSION
This study explored the value of sleep postural changes, in addition to the standard body acceleration in sleep quality assessment. The results suggest that monitoring sleep postural changes could enhance the accuracy of wake/sleep detection on average by 6% and could especially improve specificity detection, a reported weakness of wearable sensors9,30,31 on average by 15%. Moreover, adding sleep posture in addition to body acceleration enhances the precision and bias for most of the sleep parameters of interest except for SE, when compared with PSG measures.
We used a chest sensor instead of a wrist sensor to estimate postural changes and body acceleration to better emulate central truncal position. We have previously demonstrated that this sensor's chest location was able to discriminate all major postures including sitting, standing, lying, and locomotion,22,32 all of which could provide a more comprehensive quality assessment. In particular, our previous study has demonstrated that sleep posture could be detected with sensitivity and specificity of 95% or higher with a chest accelerometer.22 By identifying sleep posture, the chest sensor can accurately detect exact time in bed (TIB). TIB is of key importance to assess sleep parameters of interest including SE, TST, and SOL.33 However, wrist sensors rely on a time-log from participants to decide the TIB. As described in previous studies, time-logs are limited by user subjectivity and inconsistent adherence to logging information, which increase the likelihood of overestimated or underestimated data.10,30,34 Furthermore, the ability of monitoring daytime postures and physical activities (eg, daytime sitting, standing, lying, and locomotion), which is feasible using a chest-worn sensor, could provide valuable information to assess the effect of day activities and activity patterns (eg, unbroken long sitting bout) on quality of sleeping during the night.11,35–37 Furthermore, the information from sleep posture could assist in providing personalized care such as posture therapy to enhance sleep quality.38
We evaluated 1-minute epochs for the purpose of comparison with Actiwatch. We have also explored the chest sensor's performance with 30-second epochs in comparison with the gold standard, PSG (Table 2). The change in epoch duration did not influence the accuracy in our sample; it only slightly reduced sensitivity on average by 6%. However, the 30-second epochs sharply improved specificity (detecting wake) by up to 88% (or 50% improvement compared to the 1-minute epochs). This could be explained by the fact that most of our participants having sleep disorders also may suffer from bothersome sleep conditions, such as frequent WASO, which may not be detectable by using long segmentation epochs. We found better agreement between Chest and PSG by using 30-second epochs. The increased time resolution with shorter epochs may enhance the ability of sensor-derived parameters to track sleep disorders, which could explain our results. Similarly, the 30-second epochs reduced bias and improved precision for SE assessment compared to that of PSG. This could also be explained by the fact that WASO influences SE, and thus enhancing detection of WASO could improve the performance of measuring SE.
Although the correlation between the wrist-worn sensor and the gold standard in estimating sleep parameters of interest was weak, the chest sensor showed moderate correlation in estimating SOL and strong correlation in detecting TST, WASO, and SE. The amount of Bland-Altman plot biases for the chest sensor for all sleep parameters of interest were less, whereas the chest sensors had higher precision than the wrist-worn sensor.
Overall, the wrist-worn sensor yielded higher bias and poorer precision for measuring sleep quality parameters compared to the chest sensor. The observed biases in the Bland-Altman plots for most of the sleep parameters of interest were close to zero for both wearable systems, indicating that both wrist and chest sensors are accurate to quantify sleep parameters of interest. The precision is, however, noticeably poorer for the wrist sensor compared to the chest sensor for most sleep parameters. It is, however, unclear whether the observed differences are clinically meaningful. Further study is needed to examine whether the proposed method is more sensitive to identify cases with sleep problems and/or track changes in sleep quality as a function of intervention.
No significant correlation was observed between perceived sleep quality (ie, PSQI score) and objective sleep parameters measured using either PSG or wearable-derived parameters in our sample (absolute range: rho = 0.06–0.23, P > .05). The lack of significant correlation between perceived and objective sleep quality in this study could be explained due to the small sample size or homogeneity of the sample (eg, all the participants in this study had poor sleep quality). It could also be due to limitation of self-reported sleep parameters, which may not truly reflect actual sleep quality. This is supported by a study by Buysse et al.39 that found no agreement between PSQI and PSG or between PSQI and actigraphy. Additionally, sleep quality in the current study was assessed in a laboratory setting over a single night, which may not truly reflect the sleep quality in the natural environment of the participant and thus is unrepresentative of perceived sleep quality in general.
When comparing studies where body-worn sensors were used to evaluate sleep quality in patients with sleep apnea,4,5,30 we observed superior performance for wearable sensors that identify sleep/wake based on our proposed approach: combining body acceleration and sleep postural changes (Table 4). Specifically, using 30-second epochs, the chest sensor yielded higher sensitivity and accuracy than the wrist sensor; the chest sensor had comparable levels of specificity with the wrist-worn sensor. Similarly, when using 1-minute epochs, the chest sensor yielded better sensitivity, specificity, and accuracy than that of the wrist sensor.
Table 4
Comparison of previous study performance in identifying sleep/wake epochs using proposed algorithm for the chest-worn sensor.
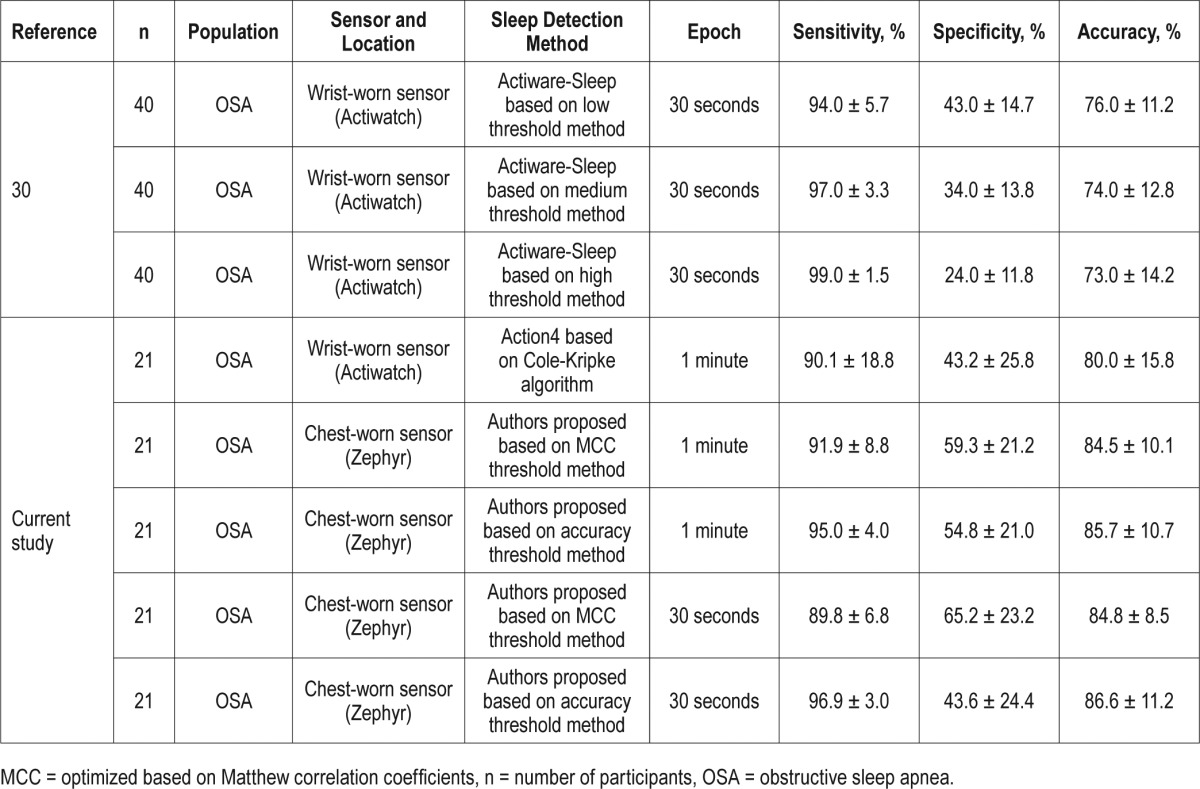
Few studies have explored sensor location other than the wrist to evaluate sleep quality. Two studies used wrist-worn sensors at the hip location and reported poorer accuracy in identifying sleep/wake episodes (Slater et al.5 used the Actigraph sensor and Zinkhan et al.4 used the SOMNOwatch and Actigraph sensors). Neither study used information from sleep postural changes for assessing wake/sleep episodes, thereby reducing accuracy and, in particular, lowering the specificity as compared to that of wrist sensors when worn on the wrist.
If only body acceleration is considered for sleep assessment, the wrist may be the best location for a sensor because of its higher sensitivity to movement while awake. However, adjusting the acceleration threshold and integrating information from sleep postures could enhance performance of sleep assessment algorithms based on wearable sensors. Further study is warranted to determine the ideal sensor placement (eg, hip, ankle, waist, or head) and if the measurement of posture enhances sleep assessment accuracy.
This study also explored whether combining the information from both chest and wrist locations could enhance detection of sleep/wake episodes. The results showed that fusing the outcomes from Wrist and Chest slightly improved detection performance, but improvement is not noticeable in our sample. In other words, rather than wearing multiple sensors, which could be cumbersome, a single chest sensor could suffice for the purpose of assessing sleep parameters of interest.
Compared to other body locations that could provide body posture (eg, hip, thigh, etc.), the chest location may be superior when measuring important physiological parameters, such as heart rate, heart rate variability, and respiration rate. We did not explore these parameters in the context of our study. Another study is needed to examine whether fusion of this information could further enhance the accuracy of sleep detection. In addition, the fusion of these physiological parameters could enable health providers to study sleep patients from multiple angles and provide more personalized care. It has been demonstrated that analyzing the fused data may predict adverse events such as fall risk in an acute care setting.39 Furthermore, fusion of physiological parameters may assist in detection other key sleep-related parameters such as different sleep stages especially stage R sleep, light sleep, and deep sleep.40
Limitations
This study has several limitations. First, the sample size was modest (n = 21), which may not provide sufficient power to identify different abnormal sleep patterns. Thus, in addition to assessing agreement between PSG and sensor-derived parameters, assuming each subject as an independent sample (n = 21), the accuracy of the proposed algorithm to detect wake/ sleep epochs was also estimated considering each individual epoch as an independent sample (n = 17,334 30-second epochs, 20% awake), which produced sufficient sample size to estimate accuracy, sensitivity, and specificity of sleep/wake discrimination. Second, our study did not include healthy subjects without sleep disorders, and therefore our study design and results cannot be generalized beyond tracking sleep quality in older adults with high suspicion of sleep disorders. Furthermore, because of a limitation of Actiwatch, this study was unable to compare the wrist-worn and chest-worn sensors using 30-second epochs. In order to compare the chest-worn sensor with the wrist-worn sensor, the results were downsampled to 1-minute epochs, which may not be sufficient to track sleep fluctuation in parameters such as number of awakenings. To address this limitation, we have also compared the changes in accuracy between 30-second and 1-minute epochs and compared the results with PSG. Third, although the chest sensor allows for capturing physiological parameters that could be beneficial for sleep assessment, this study was limited to data collected from the 3 axial accelerometers. Another study is needed to examine whether the inclusion of other physiological parameters could additionally enhance the accuracy of tracking sleep quality. Fourth, the synchronization between chest sensors and PSG was done by synchronizing the clocks of both systems. This may not be an ideal method for point-to-point comparison between two systems during long-term recording, given the fact that each device uses an independent clock with different sample frequency. This may explain part of the poor correlations observed between sleep parameters of interest extracted from the chest sensor and PSG. Fifth, the algorithm developed for postural information is beneficial and specific to individuals who sleep in a bed where they have freedom to change position. Those with a sleep disorder who sleep elsewhere (eg, sofa, recliner chair) may be restricted from changing position during sleep, and the additional value of postural changes could be negligible. Although the study includes those with sleep problems, the current study design does not have sufficient power to examine changes in accuracy in specific sleep disorders such as predominant insomnia, where subjects may be lying in bed for prolonged periods and not be asleep, and thus just information from body acceleration and sleep positions may not be sufficient to accurately identify sleep/wake status. Furthermore, we assessed the accuracy of the algorithm as a function of sleep position (Figure S3 in the supplemental material). We saw no significant difference between different sleep postures, which represented the robustness of the algorithm. In a future study, we will report the prevalence of different postures in several subgroups of patients to assess posture affects. Finally, this study was conducted in a sleep laboratory that does not represent the natural environment of at-home sleep. However, this may have a limited effect on this study because it was focused on assessing the validity of the proposed algorithm in measuring sleep parameters of interest, and all devices were compared in an identical environmental condition. Another study is warranted to examine whether a chest-worn sensor has enough sensitivity and specificity to accurately and precisely identify those with sleep disorders from healthy controls in their natural sleep environment.
CONCLUSIONS
This study investigates whether a chest sensor capable of extracting body acceleration and sleep postural changes information is more accurate in assessing sleep/wake epochs in subjects with sleep disorders than the more commonly used wrist sensor (Actiwatch), when compared to the gold standard, PSG. Our findings suggest that combining the information from body acceleration and sleep positional changes could enhance detection of sleep/wake epochs and therefore enrich clinical diagnosis of sleep disorders.
DISCLOSURE STATEMENT
Work for this study was performed at University of Arizona; Baylor College of Medicine; Michael E. DeBakey, Veterans Affairs Medical Center. This study was supported in part by a grant from National Institute of health (NIH), National Cancer Institute (Award # 1R21CA190933-01A1), University of Arizona (UA), and Baylor College of Medicine (BCM). The content is solely the responsibility of the authors and does not necessarily represent the official views of NIH, UA, or BCM. The authors report no conflicts of interest.
ACKNOWLEDGMENTS
The authors thank Kimberly Macellaro, PhD, a member of the Baylor College of Medicine Michael E. DeBakey Department of Surgery Research Core Team, for her editorial assistance during the preparation of this manuscript.
REFERENCES
Articles from Journal of Clinical Sleep Medicine : JCSM : Official Publication of the American Academy of Sleep Medicine are provided here courtesy of American Academy of Sleep Medicine
Full text links
Read article at publisher's site: https://doi.org/10.5664/jcsm.6802
Read article for free, from open access legal sources, via Unpaywall:
https://jcsm.aasm.org/doi/pdf/10.5664/jcsm.6802
Citations & impact
Impact metrics
Citations of article over time
Article citations
Deciphering Optimal Radar Ensemble for Advancing Sleep Posture Prediction through Multiview Convolutional Neural Network (MVCNN) Approach Using Spatial Radio Echo Map (SREM).
Sensors (Basel), 24(15):5016, 02 Aug 2024
Cited by: 1 article | PMID: 39124063 | PMCID: PMC11314943
Evaluating reliability in wearable devices for sleep staging.
NPJ Digit Med, 7(1):74, 18 Mar 2024
Cited by: 3 articles | PMID: 38499793 | PMCID: PMC10948771
Review Free full text in Europe PMC
Objectively measured peri-vaccination sleep does not predict COVID-19 breakthrough infection.
Sci Rep, 14(1):4655, 26 Feb 2024
Cited by: 1 article | PMID: 38409137 | PMCID: PMC10897487
Harnessing physical activity monitoring and digital biomarkers of frailty from pendant based wearables to predict chemotherapy resilience in veterans with cancer.
Sci Rep, 14(1):2612, 31 Jan 2024
Cited by: 1 article | PMID: 38297103 | PMCID: PMC10831115
Recent Progress in Long-Term Sleep Monitoring Technology.
Biosensors (Basel), 13(3):395, 17 Mar 2023
Cited by: 3 articles | PMID: 36979607 | PMCID: PMC10046225
Review Free full text in Europe PMC
Go to all (23) article citations
Data
Data behind the article
This data has been text mined from the article, or deposited into data resources.
BioStudies: supplemental material and supporting data
Similar Articles
To arrive at the top five similar articles we use a word-weighted algorithm to compare words from the Title and Abstract of each citation.
Comparison of Commercial Wrist-Based and Smartphone Accelerometers, Actigraphy, and PSG in a Clinical Cohort of Children and Adolescents.
J Clin Sleep Med, 12(3):343-350, 01 Mar 2016
Cited by: 59 articles | PMID: 26446248 | PMCID: PMC4773624
Validation of the Sleep-Wake Scoring of a New Wrist-Worn Sleep Monitoring Device.
J Clin Sleep Med, 14(6):1057-1062, 15 Jun 2018
Cited by: 10 articles | PMID: 29852899 | PMCID: PMC5991960
Validation of a Consumer Sleep Wearable Device With Actigraphy and Polysomnography in Adolescents Across Sleep Opportunity Manipulations.
J Clin Sleep Med, 15(9):1337-1346, 01 Sep 2019
Cited by: 55 articles | PMID: 31538605 | PMCID: PMC6760396
Wrist actigraphy.
Chest, 139(6):1514-1527, 01 Jun 2011
Cited by: 289 articles | PMID: 21652563 | PMCID: PMC3109647
Review Free full text in Europe PMC