Abstract
Free full text

Offer acceptance practices and geographic variability in allocation MELD at transplant
Abstract
Offer acceptance practices may cause geographic variability in allocation model for end-stage liver disease score (aMELD) at transplant and could magnify the effect of donor supply and demand on aMELD variability. To evaluate these issues, offer acceptance practices of liver transplant programs and donation service areas (DSAs) were estimated using offers of livers from donors recovered between January 1, 2016, and December 31, 2016. Offer acceptance practices were compared with liver yield, local placement of transplanted livers, donor supply and demand, and aMELD at transplant. Offer acceptance was associated with liver yield (odds ratio, 1.32; P < 0.001), local placement of transplanted livers (odds ratio, 1.34; P < 0.001), and aMELD at transplant (average aMELD difference, −1.62; P < 0.001). However, the ratio of donated livers to listed candidates in a DSA (i.e., donor-to-candidate ratio) was associated with median aMELD at transplant (r = −0.45; P < 0.001), but not with offer acceptance (r = 0.09; P = 0.50). Additionally, the association between DSA-level donor-to-candidate ratios and aMELD at transplant did not change after adjustment for offer acceptance. The average squared difference in median aMELD at transplant across DSAs was 24.6; removing the effect of donor-to-candidate ratios reduced the average squared differences more than removing the effect of program-level offer acceptance (33% and 15% reduction, respectively). Offer acceptance practices and donor-to-candidate ratios independently contributed to geographic variability in aMELD at transplant. Thus, neither offer acceptance nor donor-to-candidate ratios can explain all of the geographic variability in aMELD at transplant.
Introduction
Due to the controversy surrounding liver allocation, the determinants of geographic disparities in access to liver transplant and, specifically, geographic variability in median allocation model for end-stage liver disease score (aMELD) at transplant are contested. Most proposals assume that geographic variability in deceased donor liver supply and demand is the underlying cause of geographic variability in median aMELD at transplant. However, offer acceptance practices could be an important determinant. For example, below average offer acceptance in kidney transplantation was associated with higher odds of kidney discard and lower odds of local placement, i.e., kidneys transplanted in the recovering donation service area (DSA) (1). A similar association in liver transplantation could artificially depress local donor supply and lead to higher aMELD at transplant than expected based on the local donor supply and demand. However, previous studies of liver offer acceptance practices have not addressed the relationship between offer acceptance and geographic variability in aMELD at transplant.
We investigated several dimensions of the relationship between offer acceptance practices and geographic variability in aMELD at transplant. Specifically:
The association of offer acceptance with liver yield or local placement of recovered and eventually transplanted livers. This is a potential mechanism that could cause DSAs with below average offer acceptance to perform transplants in recipients with higher aMELD than expected.
The association between offer acceptance practices and aMELD at transplant. This association would suggest that offer acceptance practices contribute to geographic variability in aMELD at transplant.
The association between donor supply and demand and aMELD at transplant. While this relationship has been previously observed (2), we specifically evaluated the association after adjusting for offer acceptance practices. If offer acceptance practices confound the geographic disparities caused by donor supply and demand, then the adjusted association between donor supply and demand and aMELD at transplant should attenuate. Otherwise, offer acceptance and donor supply and demand would likely be independent contributors to geographic variability in aMELD at transplant.
The average squared differences of median aMELD at transplant after removing the effect of offer acceptance practices or the effect of donor supply and demand. This helps conceptualize the relative importance of offer acceptance versus donor supply and demand to geographic variability in aMELD at transplant. For example, the component that most reduces the average squared differences contributes more to geographic variability in median aMELD at transplant.
The literature on offer acceptance practices in liver transplantation is sparse with respect to the influence on geographic variability in aMELD at transplant. A better understanding of the relationship between offer acceptance practices and geographic variability in aMELD at transplant will help inform discussions of liver allocation.
Methods
This study used Scientific Registry of Transplant Recipients (SRTR) data. The SRTR data system includes data on all donors, waitlisted candidates, and transplant recipients in the US, submitted by the members of the Organ Procurement and Transplantation Network (OPTN), and has been described elsewhere (3). The Health Resources and Services Administration, US Department of Health and Human Services, provides oversight of the activities of the OPTN and SRTR contractors.
Liver Offer Acceptance Model
Discrete-time survival models estimated the probability of acceptance separately for offers to pediatric or adult candidates from match runs that ended in acceptance, and were estimated with generalized linear models with a logit-link. The time-scale was the number of previous offers, and a semi-parametric baseline hazard function (i.e., the effect of the number of previous offers) ensured a non-zero probability of acceptance for each offer. The survival model for offers to adult candidates was stratified by donor age: ≤ 40 or > 40 years. The offer acceptance model adjusted for donor quality, candidate health, and donor-candidate interactions. The model used match runs for livers recovered between January 1, 2016, and December 31, 2016. The Supplemental Materials provide a thorough description of the offer acceptance model.
The offer acceptance model assumed that each offer to a program within a match run was independent after adjusting for donor and candidate characteristics. This assumption is likely false as numerous anecdotes describe programs declining every offer associated with a donor after receiving the initial offer. Programs that receive offers from unacceptable donors will likely have lower offer acceptance ratios than if they had never received the offers. However, the model adjusts for offer number, and the probability of acceptance typically decreases with higher offer numbers (see Figure S1). Additionally, programs that regularly decline every offer associated with a liver may artificially restrict the donor pool, and aMELD at transplant may therefore be higher. Since this is related to the question of interest, the offer acceptance model should not remove the potential effect.
The descriptive statistics of the offer data were stratified by offer number to emphasize the differences in acceptance practices at different points in the match run. The specific categories were the first offer, offers 2–10 and offers after 10. Frequencies and percentages summarized categorical variables, while means and standard deviations summarized continuous variables.
Estimation of Program- and DSA-Level Offer Acceptance Ratios
The program- and DSA-level offer acceptance ratios were estimated separately from the liver offer acceptance model to alleviate the computational burden associated with the large number of deceased donor liver offers (over 170,000 offers in the cohort). After estimating the liver offer acceptance model, a generalized linear mixed effects model with a logit link estimated the program- and DSA-specific offer acceptance ratios with a random intercept term (4). The offer acceptance ratios accounted for donor and candidate characteristics through an offset term equal to the linear predictors from the liver offer acceptance model.
Metric of Donor Supply and Demand
The metric of donor supply and demand in a DSA was the number of recovered liver donors and the number of candidates on the waiting list in the DSA between January 1, 2016, and December 31, 2016, i.e., donor-to-candidate ratios. This metric focuses on the current state of liver supply and demand and may be subject to historical disparities and/or differential listing practices, which have been implicated in geographic variability in aMELD at transplant (2). Sensitivity analyses considered each combination of incident listings (i.e., listing on or after January 1, 2016), candidates with aMELD ≥ 15, and candidates with aMELD ≥ 20 at any point between January 1, 2016, and December 31, 2016.
Metric of Geographic Disparities in Access to Transplantation
The aMELD of transplant recipients in a DSA was the metric of geographic disparities in access to deceased donor liver transplant. Conceptually, in an allocation system with geographic equity, the aMELD at transplant would be similar across DSAs because the allocation system would not result in greater illness severity and waitlist mortality rate prior to transplant across listing DSAs. Additionally, aMELD at transplant is typically the metric of geographic disparities for the current discussions of liver allocation. The aMELD was assessed for recipients who underwent transplant between January 1, 2016, and December 31, 2016.
Association of Offer Acceptance with Liver Yield and Local Placement
A multiple logistic regression estimated the association of DSA-level offer acceptance ratios (on log base 2 scale) with the likelihood of liver yield and local placement of transplanted livers recovered in the DSA. By placing the offer acceptance ratios on the log base 2 scale, the interpretation of the odds ratios was the increase or decrease in odds for every doubling of the DSA-level offer acceptance ratio. The liver yield analysis used “recovered donors,” i.e., donors from whom any solid organ was recovered, which is the liver yield definition from regulatory review of organ procurement organizations (OPOs). The analysis of local placement used transplanted livers rather than recovered donors because the export status of discarded livers is unknown. For both analyses, the cohort was donors recovered in a DSA with an active liver transplant program between January 1 and December 31, 2016. An active program was required in the DSA to guarantee the existence of the DSA-level offer acceptance ratio.
The logistic regressions adjusted for several donor factors: hepatitis C, hepatitis B, history of hypertension, insulin dependence, diabetes status, mechanism of death, Public Health Service increased infectious risk, sex, blood type, cause of death, circumstance of death, past or current cigarette use, past or current cocaine use, past or current use of other drugs, current alcohol use, history of cancer, cardiac arrest after brain death, history of myocardial infarction, protein in urine, time between support withdrawal and cross-clamp for donation after circulatory death (DCD), recovery outside of the contiguous United States, pO2, pO2/fiO2, serum creatinine, body mass index, liver donor risk index (5), and age. The effects of continuous variables were estimated with splines.
DSA-level offer acceptance ratios were used because recovered livers are allocated at the DSA rather than the program level. Additionally, the effect of program-level offer acceptance on liver yield or local placement is difficult to identify because several programs can receive offers for a given liver and receiving the offer may depend on the acceptance decisions of other programs.
Associations between aMELD at Transplant, Donor-to-Candidate Ratios and Offer Acceptance
Pearson correlation coefficients estimated the program- and DSA-level associations between offer acceptance ratios, donor-to-candidate ratios, and median aMELD at transplant. If offer acceptance ratios are not associated with donor-to-candidate ratios, then the association between donor-to-candidate ratios and aMELD at transplant is likely not confounded by offer acceptance practices. That is, the lack of an association would suggest that offer acceptance and donor-to-candidate ratios are independent contributors to geographic variability in aMELD at transplant. To further investigate the hypothesis, a multiple linear regression estimated the recipient-level association of aMELD at transplant with donor-to-candidate ratios (on the log base 2 scale) before and after adjusting for program-specific offer acceptance ratios. If the association between aMELD at transplant and donor-to-candidate ratios did not meaningful change after adjustment for offer acceptance, then offer acceptance practices likely did not confound the relationship.
To characterize the relative importance of offer acceptance practices and donor-to-candidate ratios on geographic variability in median aMELD at transplant, the effect of offer acceptance and donor-to-candidate ratios was removed with the estimated effects from the multiple linear regression. Specifically, for each recipient, aMELD at transplant was modified by subtracting, for example, the effect of offer acceptance times the program-level offer acceptance ratio of the program listing the recipient. These modified values, which removed the effect of program-level offer acceptance or donor-to-candidate ratios, were aggregated over DSAs. The median adjusted aMELD at transplant across DSAs was illustrated with maps of the United States and the average squared differences were calculated.
All analyses were completed in R v3.3.3. Splines were estimated with the ‘mgcv’ package.
Results
Characteristics of Offers for Deceased Donor Livers (Table 1)
Table 1
Descriptive statistics for deceased donor liver offers across different points in the match run
Offer Characteristics
| |||
---|---|---|---|
First Offer | Offers 2–10 | Offers > 10 | |
| |||
n = 5855 | n = 24,886 | n = 141,659 | |
Acceptance | 1004 (17) | 3560 (14) | 2077 (1) |
Candidate characteristics | |||
![]() | 38 (24) | 46 (23) | 56 (13) |
![]() | 66 (37) | 72 (33) | 83 (22) |
![]() | 3264 (56) | 14945 (60) | 90273 (64) |
![]() | 1062 (18) | 2053 (8) | 4589 (3) |
![]() | 37 (6) | 31 (8) | 21 (8) |
Donor characteristics | |||
![]() | 39 (17) | 41 (18) | 48 (18) |
![]() | 79 (25) | 82 (25) | 87 (24) |
![]() | 924 (16) | 4084 (16) | 22835 (16) |
![]() | 1490 (25) | 6358 (26) | 36524 (26) |
![]() | 5464 (93) | 22452 (90) | 122186 (86) |
![]() | 127 (2) | 815 (3) | 7461 (5) |
![]() | 225 (4) | 1362 (5) | 10084 (7) |
![]() | 39 (1) | 257 (1) | 1928 (1) |
![]() | 374 (6) | 1485 (6) | 8116 (6) |
Note: DCD warm ischemia time is the time between withdrawal of donor support and cross-clamp. Unless otherwise indicated, values are n (%).
DCD, donation after circulatory death; HCV, hepatitis C virus; MELD, model for end-stage liver disease score; PHS, Public Health Service; SD, standard deviation.
As expected, the acceptance rate for the first 10 offers was ≥ 14%, and approximately 1% for later offers. Compared with candidates at later offers, candidates at early offers were younger, weighed less, were more likely to have undergone previous liver transplant, and had higher aMELD. Similarly, donors at early offers were younger, weighed less, and were more likely to have donated after brain death or to have lower DCD warm ischemia time, i.e., minutes between withdrawal of support and cross-clamp.
Association of DSA-Level Offer Acceptance with Liver Yield and Local Placement (Table 2)
Table 2
The association of DSA-specific offer acceptance ratios (on log base 2 scale) with liver yield and local placement of livers recovered in the DSA
Odds Ratio (95% CI) | P | |
---|---|---|
Liver yield | 1.32 (1.21–1.44) | < 0.001 |
Placement | 1.34 (1.24–1.45) | < 0.001 |
CI, confidence interval; DSA, donation service area.
After adjusting for donor factors, DSA-level offer acceptance was strongly associated with liver yield for donors recovered in the DSA and local placement of transplanted livers recovered in the DSA. For example, doubling the DSA-level offer acceptance ratio was associated with 24% higher odds of liver yield (P < 0.001) and 34% higher odds of the liver being transplanted in the local DSA (P < 0.001). Thus, higher offer acceptance was associated with a higher likelihood that donated livers were used and that they were transplanted in the local DSA.
Association between Offer Acceptance and Donor-to-Candidate Ratios (Figure 1)
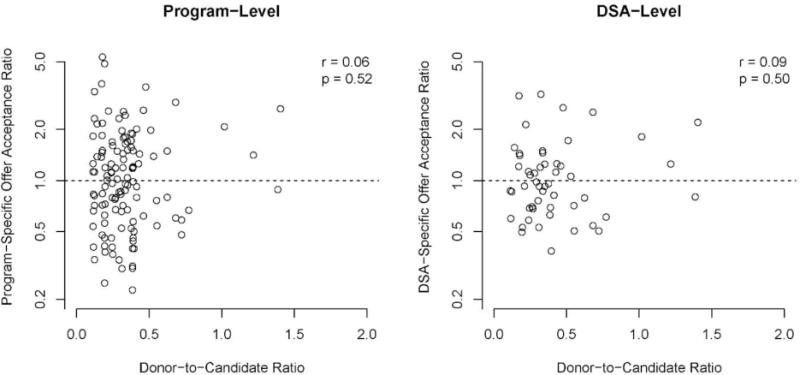
The association between program- and DSA-level offer acceptance and donor-to-candidate ratios. The donor-to-candidate ratios are measured at the DSA level. DSA, donation service area.
Donor-to-candidate ratios in a DSA were not associated with program- or DSA-level offer acceptance ratios (P = 0.52 and 0.50, respectively). That is, programs in DSAs with relatively low donor supply compared with demand did not necessarily have more conservative offer acceptance practices. Conversely, programs in DSAs with relatively high donor supply compared with demand did not have, on average, more aggressive offer acceptance practices.
Association of aMELD at Transplant with Offer Acceptance and Donor-to-Candidate Ratios (Figures 2 and and33)
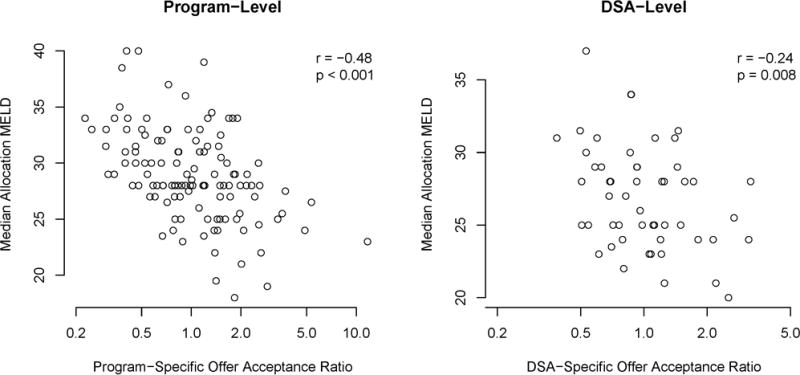
The program- and DSA-level association between offer acceptance and median aMELD. aMeld, allocation model for end-stage liver disease score; DSA, donation service area.
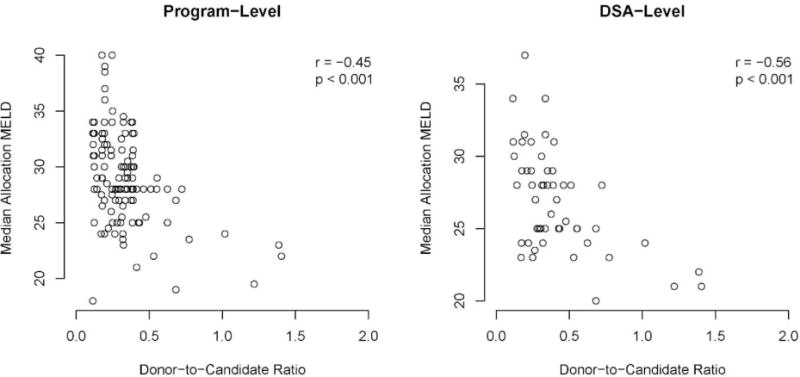
The association between program- and DSA-level median aMELD and the donor-to-candidate ratios. aMeld, allocation model for end-stage liver disease score; DSA, donation service area.
We found an association between program-level offer acceptance and the median aMELD of recipients at the program (r = −0.48; P < 0.001), and an association between DSA-level offer acceptance and the median aMELD of recipients in the DSA (r = −0.24; P = 0.008). Donor-to-candidate ratios in a DSA were associated with the median aMELD of transplant recipients at programs (r = −0.45; P < 0.001) and in DSAs (r = −0.56; P < 0.001). In other words, higher offer acceptance and higher donor-to-candidate ratios were moderately associated with lower median aMELD at transplant.
In the unadjusted recipient-level linear regression (Table 3), doubling the DSA-level donor-to-candidate ratio was associated with a −1.58 average difference in aMELD at transplant (P < 0.001). The association was unchanged after adjusting for program-level offer acceptance ratios (average difference in aMELD at transplant per doubling in donor-to-candidate ratio, −1.57; P < 0.001). That is, program-level offer acceptance practices were not an apparent confounder of the association between donor-to-candidate ratios and aMELD at transplant.
Table 3
The association of DSA-specific donor-to-candidate ratios (on log base 2 scale) with recipient-level allocation MELD at transplant
Adjustment for Offer Acceptance | Odds Ratio (95% CI) | P |
---|---|---|
Unadjusted | −1.58 (−2.16,−1.00) | < 0.001 |
Adjusted | −1.57 (−2.15,−0.99) | < 0.001 |
Note: The association was estimated before and after adjusting for program-level offer acceptance ratios (on log base 2 scale). The interpretation is the average difference in aMELD at transplant per doubling of the donor-to-candidate ratio.
CI, confidence interval; DSA, donation service area; MELD, model for end-stage liver disease score.
Average Squared Differences in Median aMELD at Transplant across DSAs (Figure 4)
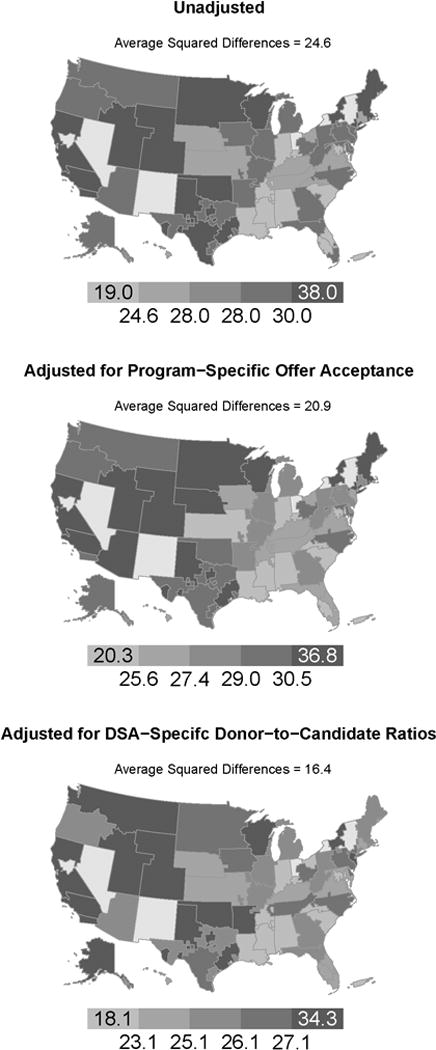
The geographic distribution of the median aMELD of recipients in a DSA. The top map used the unadjusted aMELD, the middle map removed the effect of program-specific offer acceptance practices from aMELD at transplant, and the bottom map removed the effect of donor-to-candidate ratios from aMELD at transplant. aMeld, allocation model for end-stage liver disease score; DSA, donation service area.
The average squared difference in median aMELD at transplant across DSAs was 24.6. The average squared difference in median aMELD at transplant was reduced by approximately 15% to 20.9 after the observed variability in program-level offer acceptance was removed. In contrast, the average squared difference in median aMELD at transplant was reduced by approximately 33% to 16.4 after the observed variability in donor-to-candidate ratios was removed. The variability in DSA-level donor-to-candidate ratios had a larger apparent effect on the variability in median aMELD at transplant than the variability in program-level offer acceptance practices.
Discussion
Variation in offer acceptance practices was associated with liver yield, local placement of transplanted livers, and geographic variability in aMELD at transplant. However, offer acceptance was not associated with donor-to-candidate ratios and did not confound the association of donor-to-candidate ratios with aMELD at transplant. Offer acceptance and donor-to-candidate ratios were therefore independent contributors to geographic variability in aMELD at transplant. Further, offer acceptance practices had a smaller apparent effect than donor-to-candidate ratios on the squared differences in median aMELD at transplant across DSAs. Thus, geographic variability in aMELD at transplant is not entirely explained by only offer acceptance practices or donor-to-candidate ratios.
Reducing program-level variability in offer acceptance practices could have several benefits. First, lower geographic variability in aMELD at transplant could improve equity in access to liver transplant. Second, since offer acceptance of first-ranked liver offers was associated with waitlist mortality (6), reducing variability in offer acceptance could also reduce program-level variability in waitlist mortality. However, reducing program-level variability in offer acceptance practices is not straightforward. Many authors advocate further emphasis on offer acceptance, e.g., in program evaluations, while simultaneously acknowledging that some program-level variability in offer acceptance is expected due to variability in expertise (6;7;8). Further, variability in program-level offer acceptance may be caused by differences in institutional resources available to the program, which may suggest an association between offer acceptance and program volume. Low offer acceptance for high-risk donors may also be appropriate for low-volume liver programs; prior research observed worse posttransplant outcomes in low- compared with high-volume programs (9). Alternatively, poor outcomes of recent transplants and the fear of regulatory review could cause below average offer acceptance practices. Thus, further research should investigate the program-level determinants of offer acceptance and assess the tradeoff of higher offer acceptance and the correspondingly better access to transplant with potentially worse posttransplant outcomes at, for example, small-volume programs.
The association between offer acceptance and liver yield suggests that improving offer acceptance could improve the likelihood of liver yield. However, offer acceptance practices have a nuanced relationship with liver discard. For example, implementation of Share 35 was associated with a lower offer acceptance rate for the first five offers of a match run (10), but the unadjusted and adjusted liver discard rate decreased after implementation of Share 35 (11;12). Thus, a higher offer acceptance rate may not necessarily improve liver yield. Liver yield could instead be a product of offer acceptance for the entire match run rather than for the first five offers. For example, Share 35 may have decreased the likelihood of acceptance during the first five offers but improved offer acceptance rates in other parts of the match run. A broader focus on acceptance may be especially important since 31% of recovered and transplanted livers in 2016 were accepted after the tenth offer and the livers were from lower quality donors, e.g., older and heavier (see Table 1). Additionally, preliminary research found that the relationship between kidney offer acceptance and discard may be modified by donor quality (1). A similar situation could exist for liver yield in different parts of the match run. Further research should investigate the causal mechanisms of the relationship between offer acceptance and liver yield.
The association of offer acceptance with liver yield and local placement of transplanted livers extends a similar association from kidney transplantation (1). For liver transplantation, the association could have attenuated due to the additional emphasis on regional sharing compared with kidney transplantation, e.g., candidates with aMELD ≥ 35 (Share 35). Since non-local programs may have to decline offers for high-aMELD candidates, the increased regional sharing could have separated the likelihood of liver yield for donors recovered in the DSA from the offer acceptance practices of local programs. Further research should investigate the role of broader sharing in the relationship between offer acceptance and liver yield.
The role of OPO performance in geographic disparities in access to transplant is controversial. For example, OPO performance has been criticized as a cause of high median aMELD at transplant for some DSAs. However, due to the association between the offer acceptance practices of programs in a DSA and liver yield of an OPO (i.e., 1 minus liver discard), the median aMELD at transplant in a DSA should not be compared with OPO liver yield without considering the offer acceptance practices of the local transplant programs. These relationships are further complicated by research suggesting that risk tolerance of local programs may influence an OPOs decision to pursue a donor (13). Additionally, liver yield differs by less than 2-fold between the OPOs with the highest and lowest yield (14). In contrast, DSA-level offer acceptance ratios ranged from less than 0.50 to higher than 2.00, i.e., over a 4-fold difference in offer acceptance. Thus, OPO donor yield is unlikely to be a major determinant of geographic disparities due to the small amount of variability and an association with more variable offer acceptance practices that explain some, but not most, geographic variability in median aMELD at transplant.
The donor-to-candidate ratio depends on an OPO’s propensity to recover a given liver and on transplant program listing practices. The former can depend on an OPO’s ability to convert potential donors into actual donors, i.e., the conversion rate. The OPO decision to recover a liver may also be influenced by local programs’ risk tolerance (13). Variability in listing rates is associated with geographic variability in median aMELD at transplant (14;2), and is not fully explained by geographic variability in the burden of liver disease (15). The donor-to-candidate ratio in a DSA may not, therefore, accurately identify the donor supply relative to the burden of liver disease in a DSA. However, any alternative metric of donor supply and demand (e.g., donors per million divided by the liver disease burden in a DSA) would have to be associated with offer acceptance practices to become a dependent contributor to geographic variability in aMELD at transplant. The association of donor-to-candidate ratios with aMELD at transplant and the lack of an association with offer acceptance practices remained in sensitivity analyses that considered incident listings and/or candidates with aMELD ≥ 15 (see Figures S2-S11). Further research could investigate the association of offer acceptance with alternative metrics of donor supply and demand, e.g., alternative definitions of potential donors (16;17), and defining demand as the burden of liver disease in a DSA (15;18).
Our analysis of the relationship is subject to potential limitations. First, the offer acceptance model could only consider offers of eventually accepted livers. This could cause offer acceptance ratios to appear higher than they would if every offer was considered. Second, the study was observational and could be biased by unmeasured risk factors. For example, the analysis considered a recent cohort, and the current variability in aMELD at transplant could be caused by historical variability in offer acceptance that is independent of current offer acceptance practices. Alternatively, if donors with cardiovascular disease were more likely to be recovered in certain DSAs, then the programs in the DSAs could have lower offer acceptance than expected and the yield of recovered livers could be lower than expected. Lastly, the number of liver donors in a DSA may be influenced by OPO performance beyond the effect of liver yield, e.g., donation rate, which could cause lower donor-to-candidate ratios than expected.
In summary, we found that offer acceptance practices were associated with liver yield, local placement of transplanted livers, and aMELD at transplant. Reducing variability in offer acceptance may reduce, but would not eliminate, geographic variability in aMELD at transplant because donor-to-candidate ratios were an independent contributor to such variability.
Supplementary Material
Supp FigS1-11
Figure S1. The estimated effect of offer number up to 100 for the offer acceptance models stratified by pediatric status and donor age for offers to adult candidates. Offer number 1 is the reference level for each model. The effect of offer numbers after 100 are estimated with right-hand linear splines on the log-scale and are omitted due to the sparsity and skewness of the data.
Figure S2. A sensitivity analysis of the association between median aMELD at transplant and the donor-to-candidate ratio. The donor-to-candidate ratio used the number of candidates listed during the cohort period, i.e., incident listings.
Figure S3. A sensitivity analysis of the association between median aMELD at transplant and the donor-to-candidate ratio. The donor-to-candidate ratio used the number of candidates with an aMELD score greater than or equal to 15 at any point during the cohort period.
Figure S4. A sensitivity analysis of the association between median aMELD at transplant and the donor-to-candidate ratio. The donor-to-candidate ratio used the number of candidates with an aMELD score greater than or equal to 20 at any point during the cohort period.
Figure S5. A sensitivity analysis of the association between median aMELD at transplant and the donor-to-candidate ratio. The donor-to-candidate ratio used the number of candidates listed during the cohort period with an aMELD score greater than or equal to 15 at any point during the cohort period.
Figure S6. A sensitivity analysis of the association between median aMELD at transplant and the donor-to-candidate ratio. The donor-to-candidate ratio used the number of candidates listed during the cohort period with an aMELD score greater than or equal to 20 at any point during the cohort period.
Figure S7. A sensitivity analysis of the association between the offer acceptance and donor-to-candidate ratios. The donor-to-candidate ratio used the number of candidates listed during the cohort period, i.e., incident listings.
Figure S8. A sensitivity analysis of the association between the offer acceptance and donor-to-candidate ratios. The donor-to-candidate ratio used the number of candidates with an aMELD score greater than or equal to 15 at any point during the cohort period.
Figure S9. A sensitivity analysis of the association between the offer acceptance and donor-to-candidate ratios. The donor-to-candidate ratio used the number of candidates with an aMELD score greater than or equal to 20 at any point during the cohort period.
Figure S10. A sensitivity analysis of the association between the offer acceptance and donor-to-candidate ratios. The donor-to-candidate ratio used the number of candidates listed during the cohort period with an aMELD score greater than or equal to 15 at any point during the cohort period.
Figure S11. A sensitivity analysis of the association between the offer acceptance and donor-to-candidate ratios. The donor-to-candidate ratio used the number of candidates listed during the cohort period with an aMELD score greater than or equal to 20 at any point during the cohort period.
Supp Info
Acknowledgments
The authors thank SRTR colleague Nan Booth, MSW, MPH, ELS, for manuscript editing.
Financial support: This work was conducted under the auspices of the Minneapolis Medical Research Foundation, contractor for the Scientific Registry of Transplant Recipients, as a deliverable under contract number HHSH250201500009C (US Department of Health and Human Services, Health Resources and Services Administration, Healthcare Systems Bureau, Division of Transplantation). As a US Government-sponsored work, there are no restrictions on its use. The views expressed herein are those of the authors and not necessarily those of the US Government. AKI was partially supported by R01 HS 24527. SEG was partially supported by 1R01DK111233-01 from the National Institute of Diabetes and Digestive and Kidney Diseases (NIDDK).
Abbreviations
aMELD | allocation model for end-stage liver disease |
DCD | donation after circulatory death |
DSA | donation service area |
OPO | organ procurement organization |
OPTN | Organ Procurement and Transplantation Network |
SRTR | Scientific Registry of Transplant Recipients |
Footnotes
Conflicts of interest: The authors have no conflicts of interest to report.
Supplementary Materials
Description of Offer Acceptance Model
References
Full text links
Read article at publisher's site: https://doi.org/10.1002/lt.25010
Read article for free, from open access legal sources, via Unpaywall:
https://aasldpubs.onlinelibrary.wiley.com/doi/pdfdirect/10.1002/lt.25010
Citations & impact
Impact metrics
Article citations
Center expansion of liver transplants using donation after circulatory death organs is associated with reduced overall waitlist mortality.
Clin Transplant, 37(6):e14960, 17 Mar 2023
Cited by: 0 articles | PMID: 36929662 | PMCID: PMC10272092
Heterogeneous donor circles for fair liver transplant allocation.
Health Care Manag Sci, 27(1):20-45, 20 Jul 2022
Cited by: 0 articles | PMID: 35854169 | PMCID: PMC10896798
Tool to Aid Patients in Selecting a Liver Transplant Center.
Liver Transpl, 26(3):337-348, 01 Mar 2020
Cited by: 5 articles | PMID: 31923342 | PMCID: PMC8193801
Scientific Registry of Transplant Recipients program-specific reports: where we have been and where we are going.
Curr Opin Organ Transplant, 24(1):58-63, 01 Feb 2019
Cited by: 16 articles | PMID: 30575617
Review
Go to all (8) article citations
Data
Data behind the article
This data has been text mined from the article, or deposited into data resources.
BioStudies: supplemental material and supporting data
Similar Articles
To arrive at the top five similar articles we use a word-weighted algorithm to compare words from the Title and Abstract of each citation.
Variability in acceptance of organ offers by pediatric transplant centers and its impact on wait-list mortality.
Liver Transpl, 24(6):803-809, 01 Jun 2018
Cited by: 5 articles | PMID: 29506323
Heart and lung organ offer acceptance practices of transplant programs are associated with waitlist mortality and organ yield.
Am J Transplant, 18(8):2061-2067, 09 May 2018
Cited by: 12 articles | PMID: 29673099 | PMCID: PMC6836691
Analysis of Liver Offers to Pediatric Candidates on the Transplant Wait List.
Gastroenterology, 153(4):988-995, 13 Jul 2017
Cited by: 25 articles | PMID: 28711630 | PMCID: PMC6288076
New paradigms for organ allocation and distribution in liver transplantation.
Curr Opin Gastroenterol, 34(3):123-131, 01 May 2018
Cited by: 4 articles | PMID: 29465430
Review
Funding
Funders who supported this work.
AHRQ HHS (1)
Grant ID: R01 HS024527
Health Resources and Services Administration, Healthcare Systems Bureau, Division of Transplantation (1)
Grant ID: HHSH250201500009C
NIDDK NIH HHS (1)
Grant ID: R01 DK111233